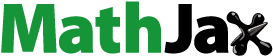
Abstract
The objective of this research is to examine and compare the mean reversion phenomenon in developed and emerging stock markets. An important aim is to measure and compare the speed of mean reversion and half-life of volatility shocks of emerging and developed markets. For this purpose, we have selected five developed and seven emerging markets, and used daily market indices for the period of 1 January 2000 to 30 June 2016. We employed autoregressive conditional heteroskedasticity – Lagrange multiplier (A.R.C.H.-L.M.), generalised autoregressive conditional heteroskedasticity (G.A.R.C.H.) (1, 1), and half-life volatility shock techniques to carry out this research. The results of the study confirmed the mean-reverting process in developed and emerging markets. The South Korean market has the slowest mean reversion, and thus has the highest comparative volatility over a longer period of time. However, the Pakistan stock exchange exhibited the fastest mean reverting process. It is also concluded that the relative volatilities are higher in emerging markets, whereas the comparative volatilities are higher in developed markets. Therefore, it is further concluded that the mean reversion process is much faster in emerging indices except the South Korean and Chinese markets. The study recommends that if investors want higher returns in a shorter period of time then they should invest in emerging markets.
1. Introduction
1.1. Background of the research study
The asset-pricing model has been a paramount feature for the equity prices since the 1970s. The efficient market hypothesis considers asset pricing as a core proposition in financial economics. Thus, this hypothesis provides all the necessary information to the individual and institutional investors regarding the equity prices that also allow an opportunity to gain excessive profit. This model also provides an opportunity for the investors to get better future equity returns. Thus, econometricians try to calculate the actual potential of undervalued stock by using different techniques. The efficient market hypothesis is also described in terms of a random walk; it is very complicated to explore the undervalued company stock and its future performance (Mishra, Citation2017; Tripathy, Citation2017; Wang, Zhang, & Zhang, Citation2015; Zakamulin, Citation2016).
In contrast, mean reversion theory elaborates the investors’ point of view as compared to the random walk. The theory of mean reversion demonstrates that after touching a certain extreme point, the equity prices return back to their mean prices (Arefin & Ahkam, Citation2017; Chi, Dong, & Wong, Citation2016; Lubnau & Todorova, Citation2015; Ribeiro, Cermeño, & Curto, Citation2017). The phenomenon is known as the mean reversion if the equity price has a tendency to come back its original long-term average price after some certain time period (Chaves & Viswanathan, Citation2016; Huang, Citation2017; Huggins & Schaller, Citation2013; Trypsteen, Citation2017). According to Hillebrand (Citation2003), the mean reversion varies in the equity return, following a path that is determined by past reaction to changes. Thus, the financial experts believe that the future prices can be predicted on the basis of past history of equity prices.
1.2. Distinction between mean reversion and random walk
There is a significant difference between the mean reversion and the random walk. This can be seen by drawing the graphs of mean reversion and random walk. As depicted in Figure , in the random walk model, observations start from zero, with each subsequent movement starting from the value of a previous observation. This random deviation means that the model cannot predict the mean of future price on the basis of past historical behaviour of the price.
Figure 1. Random Walk. Source: Huggins and Schaller (Citation2013; p. 19).
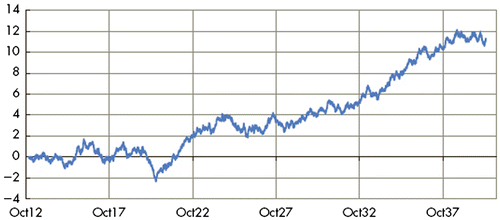
On the other hand, if we observe the Figure , it is clearly demonstrated that the spikes of observations show that every observation has a tendency to revert back its original mean value, and this is known as the mean reversion phenomenon.
Figure 2. Mean Reversion Process. Source: Huggins and Schaller (Citation2013; p. 19).
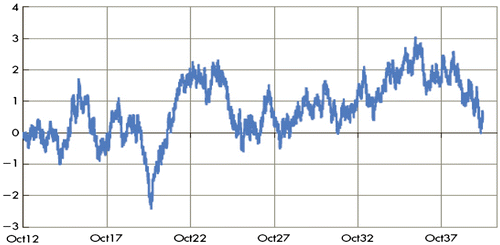
Since the investors’ decision cannot be considered as rational if they simply rely on the market propositions, financial researchers develop a mean reversion process on the basis of well-known anomalies of the finance. Hence, it can be believed that the mean-reverting process is the by-product of risk aversion, expediency prejudice and attraction of lower equity prices (Mohammadi, Citation2017; Al-Hajieh, Citation2017; Oikarinen & Schindler, Citation2015; Tie & Zhang, Citation2016). The foremost factor which creates the mean reversion development is the obtainability prejudice of the market, and investors’ tendency to take their decisions on the basis of available information (Gimpel, Citation2007). The heavy reliance on the current events and information means that investors can overreact, being more speculative on the valuation of a stock. Another important paradigm for the development of mean reversion is the risk aversion when bad news on the market triggers a loss in returns, meaning that investors prefer to retain their equity to prevent further losses. After a certain time period when the bad news passes, equity prices return into profit. The third important reason, which contributes to the development of the mean reversion process, is the attraction of low prices of equities, and investors willing to purchase stocks at lower prices for significant profits (Annaert & Hyfte, Citation2005; Hart, Lence, Hayes, & Jin, Citation2015; Slim, Koubaa, & BenSaïda, Citation2017; Trypsteen, Citation2017; Tsekrekos & Yannacopoulos, Citation2016).
The objective of this research paper is to examine the existence of mean reversion in emerging and developed stock markets. Another important objective is to measure and compare the speed of mean reversion between emerging and developed stock markets, and therefore measure the comparative and relative volatilities and relative market efficiencies of the emerging and developed equity markets. By accomplishing these specified objectives the study intends to help investors to identify the rate at which the prices revert back to their mean value, not only helping in the selection of strategies but also in planning entries and exits for the trade.
2. Substantiation from the literature
Financial researchers and practitioners have investigated different techniques and theories to analyse the behaviour of stocks across the globe. As discussed in the earlier section, the efficient market hypothesis is the oldest proposition. According to that proposition, the equity price reflects all the necessary information which is important for the individual and institutional investors. This proposition does not follow any specific pattern; therefore, the returns cannot be predicted. The random walk is an alternative terminology for the efficient market hypothesis; it is very complicated to explore the undervalued stock, and to predict whether the stock can perform more strongly in the future (Mishra, Citation2017; Tripathy, Citation2017; Wang et al., Citation2015; Zakamulin, Citation2016). According to Al-Hajieh (Citation2017) and Dupernex (Citation2007), this unpredictability no longer exists for the equity returns. In the light of previous theories, several researchers have carried out their studies in order to understand the global financial markets turmoil of 2007–08. They have come to the conclusion that the financial policies were the major reason for that crash (Ahmad, Raheem Ahmed, Vveinhardt, & Streimikiene, Citation2016; İzgi & Duran, Citation2016; Neaime, Citation2015; Slim et al., Citation2017).
The econometricians and financial experts questioned the whole foundation of the efficient market hypothesis assumptions (Mtunya, Ngare, & Nkansah-Gyekye, Citation2016; Trypsteen, Citation2017). Thus, it is important to examine the alternative theories that can predict the market performance in an efficient manner. Keeping in mind the basic idea that ‘what goes up must come back down’, De Bondt and Thaler (Citation1985) proposed the postulate of mean reversion. This theory refers the historic mean values of the stock prices and is known as the mean reverting process. According to this theory that there is a tendency of returns to come back its past mean value after a certain time period. Fama and French (Citation1988) have also carried out a study to confirm the mean reversion phenomenon, and they studied the memory pattern in stock returns. They concluded that the past prices predict the future values of stocks, and this is the proof of memory pattern that supports the postulate of a mean reversion process. According to previous research studies, the mean reversion process can be segregated into long-term and short-term mean reverting processes. If the return of a stock back into its mean value occurs within a one-month time period then it is known as short-term mean reversion process (Chi et al., Citation2016; Hart et al., Citation2015; Ribeiro et al., Citation2017). The flow of research studies regarding the short-term mean reversion has also been increased due to the access of information on a daily basis regarding the investments. Hence, short-term mean reversion was found to be more attractive than the long-term mean reversion process (Chaves & Viswanathan, Citation2016; Huang, Citation2017; Neaime, Citation2015; Wang et al., Citation2015). If the stock underperforms within a one-year time period and after one year it overperforms then this phenomenon is known as the long-term mean reversion. According to the previous literature, long-term mean reversion can be examined by using two different methods, termed as relative mean reversion and absolute mean reversion (Slim et al., Citation2017; Trypsteen, Citation2017).
2.1. Mean reversion in stock prices
The researchers and financial experts have given much attention to distinguish the returns of a market and whether it follows the mean reversion process or the random walk across the globe. If the stock returns follow the mean reversion then it is easy to predict the future returns of the stocks on the basis of past information, which is the most important reason behind these studies (Gulay & Emec, Citation2017). According to Mohammadi (Citation2017), Chaves and Viswanathan (Citation2016), Hart et al. (Citation2015), Tsekrekos and Yannacopoulos (Citation2016), Lubnau and Todorova (Citation2015) and Balvers, Wu, and Gilliland (Citation2000), the mean reversion process provides an opportunity to earn more profit because investors buy stocks at the lowest prices and sell them when they become more profitable by using the constrained trading strategy (Chen, Jiang, & Li, Citation2012). Substantial work was carried out by Neaime (Citation2015) and Hakim and Neaime (Citation2003), who reviewed the mean reversion behaviour of stock markets of Middle East and North African (M.E.N.A.) countries. The results of the studies indicated that the Jordan, Turkey, Egypt and Morroco markets have shown a rapid mean reversion process that also exhibits the stocks’ volatility of these market. Similarly, Kuttu (Citation2017) suggested that the existence of mean reversion process explains the different behaviours of the stock market. Ribeiro et al. (Citation2017), Ahmad et al. (Citation2016), and Kim, Morley, and Nelson (Citation2001) also studied the behaviours of stocks and volatility, and they have also concluded the direct relationship between stock returns and the volatility.
Arefin and Ahkam (Citation2017), Chen et al. (Citation2012), and Balsara, Chen, and Zheng (Citation2007) concluded that the investors should use the constrained trading strategy to get maximum profit because of the mean reversion process. Chaudhuri and Wu (Citation2003) studied the behaviour of stock returns in 17 emerging markets. They indicated that there is an existence of a mean reversion phenomenon, and also calculated the speed of mean reversion by using the half-life method. They concluded that equity returns revert back to their past mean values after 30 months in emerging markets. Poshakwale (Citation2003), Malliaropulos and Priestley (Citation1996), Riaz (Citation2014) and Mustafa and Ahmed (Citation2013) have rejected the hypothesis of random walk and concluded the existence of mean reversion in different financial markets of the world. However, contradicting results and arguments have also been presented in support of a random walk rather than a mean reversion process (Gulay & Emec, Citation2017; Trypsteen, Citation2017). On the basis of the above discussion and previous literature, it is evident that there is still an ongoing debate regarding the equity behaviour as to whether returns follow the mean reversion process or the random walk hypothesis. A few researchers have additionally concluded that the mean reversion process varies market-to-market and region-to-region, and the time factor is also a vital determinant while investigating the mean reversion phenomenon (Kuttu, Citation2017; Slim et al., Citation2017).
3. Data and methodology
3.1. Classification of stock markets
The study undertaken considers important emerging and developed stock indices of the world. We have taken these markets as segregated by the Morgan Stanley Capital International (M.S.C.I.). In this segregation, M.S.C.I. considers different factors such as G.D.P., per capita income, perceived investment risk, local government regulations, foreign ownership restrictions and control on the capital etc. As of 2016, there were seven emerging market indices: B.V.S.P. (Brazil), S.S.E. (China), SENSEX (India), J.K.S.E. (Indonesia), K.O.S.P.I. (South Korea), K.L.S.E. (Malaysia) and K.S.E. 100 (Pakistan). As of 2016, according to the M.S.C.I., five developed equity markets, D.A.X. (Germany), H.S.I. (Hong Kong), Nikkei 225 (Japan), S.&P. 500 and N.A.S.D.A.Q. (United States), have been selected for this research. The selected markets are a good representation of emerging and developed markets because the selected emerging markets are the best performing markets in the recent time period, whereas the considered developed markets are the benchmark for the developed equity markets of the world.
3.2. Data collection
We have collected daily closing readings of stock indices from the Yahoo Finance website. The considered time period is from 1 January 2000 to 30 June 2016. The selection of this period carries a meaningful contribution because in 2007–2008 the global financial crisis has significantly hampered the global financial markets (Covitz, Liang, & Suarez, Citation2013), and all the financial markets have collapsed. Thus, this research incorporates both a pre-crisis period and a post-crisis period, meaning that the considered period for this research will give a better understanding of equity markets’ behaviour. We have selected seven prominent emerging and five developed markets as discussed above.
3.3. Estimation techniques
The analysis was carried out through different statistical techniques such as descriptive statistics, the Augmented Dickey–Fuller (A.D.F.) test, autoregressive conditional heteroskedasticity – Lagrange multiplier (A.R.C.H.-L.M.) and generalised autoregressive conditional heteroskedasticity (G.A.R.C.H.) (1,1) models (Gulay & Emec, Citation2017; Zakamulin, Citation2016). For the estimation of the speed of mean reversion and volatility shocks, we have used a half-life model (Chaudhuri & Wu, Citation2003; Goudarzi, Citation2013; Kuttu, Citation2017; Mohammadi, Citation2017).
3.4. Change in stock exchange indices
The natural log difference approach is used to compute the returns for developed and emerging indices. To substantiate the objective, the following equation is applied in order to calculate the market returns:(1)
(1)
where Equation(1)(1)
(1) SP (t) are the returns of the different equity stocks for the time ‘t’. ‘C t’ and ‘C t-1’ are the returns of the emerging and developed stock markets for the time ‘t’ and ‘t-1’, respectively.
3.5. Unit root test
There are several approaches to test the stationarity of the data time series. However, in our research study, we used the most widely and popular A.D.F. (Dickey and Fuller Citation1981) technique. The following is the generalised form of the equation for this method:(2)
(2)
whereEquation(2)(2)
(2) ‘y’ indicates the time series, ‘t’ is time period, ‘n’ is the optimum number of lags, ‘αo’ is called the constant value and ‘e’ is known as the error term.
3.6. Autoregressive conditional heteroskedasticity
Serial correlation of the heteroskedasticity is also known as the A.R.C.H. effect, which is associated with the relationship within the paradigm of heteroskedasticity. It frequently emerges when there is a clustering in the volatility of a specific variable which is identified by some other variable. The volatility of any financial asset or equity price is essentially the risk factor associated with that asset. The A.R.C.H. effect is the measuring technique to calculate that risk. The generalised form of the A.R.C.H. effect is given as follows:(3)
(3)
Equation (Equation3(3)
(3) ) clearly shows that a white noise error term is associated with zero mean and conditional variance, and follows a normal distribution which depends on one time lagged squared error term. The conditional variance can be defined as ‘the variance of given values of the error term lagged once, twice, thrice etc.’. The equation of conditional variance can be written as follows:
(4)
(4)
whereEquation(4)(4)
(4)
is known as the conditional variance of the white noise error. The revised form of A.R.C.H. effect can be modelled as:
(5)
(5)
Since Equation (Equation5(5)
(5) ) has a single lag on the squared error, it is called an A.R.C.H. (1) process. However, the above equation can be generalised up to ‘p’ lags. Therefore, it will be known as an A.R.C.H. (p) process, and can be expressed as follows:
(6)
(6)
3.7. Testing for the A.R.C.H. effects
Engle (Citation1982) originally proposed the A.R.C.H. effect model, and an A.R.C.H. effect test has a similarity to the LM technique for the measurement of autocorrelation. The following two steps are taken for the calculation:
1. | We use the ordinary least squares to run the regression analysis. In the regression analysis we assemble the squared residuals. | ||||
2. | After collecting the squared residuals, we execute the secondary equation of the regression analysis: |
(7)
(7)
where Equation(7)(7)
(7) ‘u’ is called the square residual, which can be measured by the primary regression model; however, in a secondary regression model, ‘p’ lags are incorporated.
3.8. The G.A.R.C.H. (p, q) model
The generalised A.R.C.H. model is a natural extension of A.R.C.H. besides the A.D.F. and A.R.C.H. models. The generalised A.R.C.H. model can also be used to examine the mean reversion process because it overcomes the shortcomings of the classical A.R.C.H. model. The generalised A.R.C.H. model is denoted as the G.A.R.C.H. process, and in G.A.R.C.H. model we sum up both the A.R.C.H. (α) and G.A.R.C.H. (β) coefficients. In the G.A.R.C.H. model, if the sum of coefficients is less than 1 (α+β<1) then the indices of the time series demonstrate the mean reversion process. If the sum of the A.R.C.H. and G.A.R.C.H. coefficients is equal to 1 (α+β=1) then it is said that the time series of stock markets indices follow the random walk hypothesis. According to Bollerslev (Citation1986), the G.A.R.C.H. model has two distributed lags. The possession of high-frequency effects on lag squared residuals is shown by the first lag; however, the possession of long-run effects of the variance of the lagged values can be captured by the second lag of the G.A.R.C.H. model. The following is the representation of conditional variance:(8)
(8)
where Equation(8)(8)
(8) ‘ψt-1’ is known for the information arrangement accessible at the first lag of time ‘t’. Hence, the generalised expression for the G.A.R.C.H. (p, q) technique can be written as follows:
(9)
(9)
where Equation(9)(9)
(9) ω, α, β are known as the parameters of the G.A.R.C.H. model, ε2t-1 is the first lag of square returns, i.e., A.R.C.H.(1), σt-1 is referred to as the trailing variance, i.e., G.A.R.C.H. (1), and
is known as the conditional variance.
The conditional variance is a function of its own previous values and the squares of the past residuals. Engle (Citation2001) and Bollerslev (Citation1986) have proposed the G.A.R.C.H. (1, 1) model, which can be shown as follows:
(10)
(10)
3.9. Mean reversion and G.A.R.C.H. and A.R.C.H. models
The low or high perseverance in the volatility is usually determined by the G.A.R.C.H. coefficients of a stationary G.A.R.C.H. technique. In a stationary G.A.R.C.H. process, the volatility returns back to its mean at the long-term horizon, and it is a rate calculated by the sum of the G.A.R.C.H. and A.R.C.H. coefficients. In any financial time series, it is usually very close to one. The half-life of the volatility shocks measures the average time periods for the volatility to return back to its mean value in a long run horizon. According to Banerjee and Sarkar (Citation2006), the volatility shock is used for the forecasting of the volatility on a moving average basis. The following is the form of a covariance stationary time series ‘yt’ with an infinite moving average order:(11)
(11)
where ψ0 = 1 and
The impulse response function (I.R.F.) can be detected by plotting ‘ψi’ versus ‘is’. The half-life is sometimes reported by the decay rate of the I.R.F. and it is signified as ‘Lhalf’, and it is the lag at which I.R.F. touches one-half (1/2).
3.10. Half-life of volatility shocks for the stationary G.A.R.C.H. (1, 1) model
The G.A.R.C.H. (1, 1) process provides the basic equation of mean reversion as follows:(12)
(12)
where
Thus, Equation (Equation12(12)
(12) ) is the volatility for unconditional long-term level, and then it can be shown as follows:
(13)
(13)
Now, we can designate Equation (Equation13(13)
(13) ) as the mean reversion rate, and the value of α1+β1 is ~1 as suggested by the most of researchers. It is also important to know that the scale of α1+β1 regulates the mean reverting speed. Thus, we can write the following expression of half-life for the volatility shocks:
(14)
(14)
To estimate the average mean time it proceeds for | ε2t – σ−2 | to reduce by the one-half (1/2). It is important to note that if the value of α1+β1 is closer to 1 then the volatility shocks’ half-life will be the lengthier. According to Banerjee and Sarkar (Citation2006) if the value of α1+β1 > 1 then the G.A.R.C.H. model said to be non-stationary, and the volatility ultimately detonates towards the infinitude.
4. Estimation and results
4.1. Descriptive analysis
Table shows the individual characteristics and properties of the stock markets. According to the results of Table , K.S.E. 100 has the highest returns (20.19% annual) but the risk-return trade-off is also very high. The results of the probability distribution deviation (skewness) indicated that all the emerging and developed markets have a left tail of returns. It further demonstrated that returns distributions are more deviated towards the left except for the returns of N.A.S.D.A.Q. The peak or flatness for all the returns are indicated through kurtosis, and Table exhibited that all the returns series have higher peaks and longer tails because kurtosis values are greater than 3; hence the data series are leptokurtic as well. The higher value of Jarque–Bera and corresponding probabilities (p<0.01) demonstrated that the return series are not normally distributed. Hence the important condition of time series modelling has been met.
Table 1. Characteristic and properties of developed and emerging markets.
4.2. Risk and return model
We have calculated the relative efficiency of emerging stock markets; we can adjust for risk when making a comparison. The mean-variance measure is chosen to examine the efficiencies by employing the following sharp ratio analysis:(15)
(15)
According to the results of the sharp ratio analysis, as shown in Table , the developed markets are relatively more efficient as compared to the emerging markets
Table 2. Sharp ratio analysis and ranking of developed and emerging markets.
4.3. Stationarity of the stock markets indices
The results of the A.D.F. test are shown in Table . These results exhibited that all the return series are stationary at level or order one. Since these results demonstrated that absolute values of A.D.F. are greater than the critical values, this shows that the return series do not have a unit root. It is further concluded that the return series of emerging and developed markets follow the standard assumptions for the asymptotic distribution.
Table 3. Stationarity of stock markets indices.
4.4. The A.R.C.H. effect in stock markets indices
Since we have determined the stationarity of the return series, the next step is to satisfy the two pre-conditions for using the A.R.C.H. and G.A.R.C.H. tests. The first condition is to plot the graphs of the squared residual of the conditional volatility. Figure demonstrates that high volatility periods follow other periods of high volatility, and low volatility periods follow periods of low volatility for the emerging and developed markets’ return series. Thus, the results of Figure confirmed the presence of conditional volatilities in all the return series, and the first condition has been fulfilled.
Figure 3. Conditional variances for emerging and developed stock markets.Table 4 has been edited. Please correct if this is inaccurate. Source: Authors’ calculations.
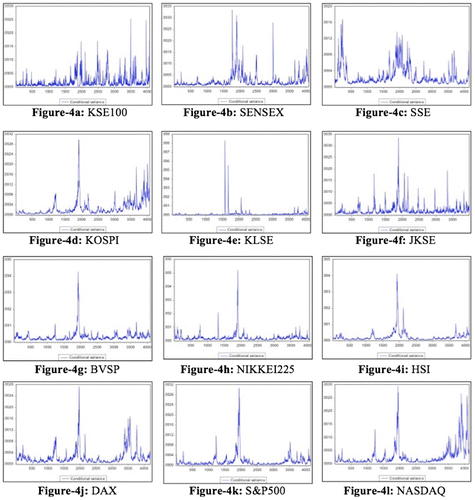
For the second pre-condition, we have employed the A.R.C.H.-L.M. test. The results of Table show that F-statistics for all the returns series are significantly higher and probabilities are less than 0.01. The results indicate the presence of A.R.C.H. effects because the null hypothesis has been rejected. Therefore, A.R.C.H. effects have significant effects. Now, both pre-conditions for using the A.R.C.H. and G.A.R.C.H. models have been satisfied.
Table 4. A.R.C.H. effect in stock markets indices (A.R.C.H.-L.M. test).
4.5. Mean and variance equations for emerging stock indices
Table shows that the higher order of the A.R.C.H. model depicted the higher degree of freedom; therefore, it is better to use G.A.R.C.H. (1,1) as compared to A.R.C.H. In Table , RESID (-1)^2 is known as the A.R.C.H. effect (α), and G.A.R.C.H. (-1) is recognised as (β), representing the previous period squared residual and previous day squared variance, respectively. The results of Table show that both A.R.C.H. and G.A.R.C.H. effects are significant (p<0.01), and we can conclude that the previous day information affects the current volatilities of emerging stock indices’ returns series. The value of R2 for emerging markets indices returns indicate that the internal stuns signified by A.R.C.H. and G.A.R.C.H. coefficients explain only 0.84% (K.S.E. 100), 0.43% (SENSEX), 0.04% (S.S.E.), 0.01% (K.O.S.P.I.), 0.71% (K.L.S.E.), 1.06% (J.K.S.E.) and 0.001% (B.V.S.P.) variations in emerging stock indices. However, the significant variation remained unexplained. Finally, the results of Table show the mean reverting process because the sum of coefficients is less than one (α+β<1), and this is the condition for stationarity or mean reversion (Bollerslev, Citation1986).
Table 5. Mean and variance equations for emerging stock indices.
4.6. Mean and variance equation for developed stock indices
The results of Table again show that both A.R.C.H. and G.A.R.C.H. effects are significant (p<0.01), demonstrating that previous day information affects the current volatilities of developed stock indices’ returns series. The value of R2 for developed market indices returns indicates that the internal shocks suggested by A.R.C.H. and G.A.R.C.H. coefficients only explain 0.02% (Nikkei 225), 0.18% (H.S.I.), 0.13% (D.A.X.), 0.45% (S.&P. 500) and 0.04% (N.A.S.D.A.Q.) variations in developed stock indices.
Table 6. Mean and variance equations for developed stock indices.
4.7. Mean reversion process in the stock indices
Table shows that the sum of G.A.R.C.H. (1,1) parameters and the sum of A.R.C.H. and G.A.R.C.H. coefficients is less than one (α+β<1) for all the emerging and developed markets. Therefore, these results confirmed the mean reverting process, and stock prices revert back to their historical value after a certain time period. It is noted that the higher values of the sum of A.R.C.H. coefficient (α) and G.A.R.C.H. coefficient (β) lead to higher volatility, and slow the mean reverting process. It is evident from Table that the K.O.S.P.I. has the slowest mean reversion with the highest comparative volatility. In contrast, the K.S.E. 100 has the fastest mean reversion, but its comparative volatility is the lowest as compared to other markets.
Table 7. Mean reversion process in emerging and developed markets.
We have calculated the speed of mean reversion by the half-life method, and the results are shown in Table . The results demonstrate that the K.O.S.P.I. has the highest sum of A.R.C.H. and G.A.R.C.H. coefficients (0.997), and thus it takes 306.62 days to revert back to half of its mean, which is the longest period as compared to other emerging and developed markets. In contrast, the K.S.E. 100 has the lowest value of α+β = 0.959, as it takes only 16.95 days to revert back to half of its mean, which is the shortest period as compared to other emerging and developed markets.
Table 8. Speed of mean reversion process in stock indices.
Table shows the results of the speed of mean reversion and ranking of the mean reverting process among emerging and developed stock markets. Since the K.S.E. 100 index displays that the returns reverted back to half of its mean value within 17 days, this suggested that investors of the K.S.E. 100 index must open a position at 0 days and must close after the 34th day. The K.O.S.P.I. has the slowest mean reversion process, and it takes 307 days to revert back to half of its mean value. Therefore, the investors of the K.O.S.P.I. should open at 0 days and must close after 614th day. Thus, the K.O.S.P.I. provides maximum leverage to the investors to operate as compared to the K.S.E. 100, which provides the least time period to operate freely.
Table 9. Speeds and ranking of mean reversion.
4.8. The significance of the A.R.C.H. and G.A.R.C.H. effects
Table depicts the summary of the A.R.C.H. and G.A.R.C.H. affects for emerging and developed stock markets. The results indicate that both the A.R.C.H. and G.A.R.C.H. effects are significant (p<0.01). It is further concluded from the results that all the emerging and developed markets observe a mean reverting process because the sum of A.R.C.H. and G.A.R.C.H. is less than 1.
Table 10. Significance of A.R.C.H. and G.A.R.C.H. effects.
5. Discussions and conclusions
The results of the study indicate that all 12 emerging and developed stock markets have exhibited the presence of the mean reversion process. The results of the study demonstrate that the developed markets are more stable and less volatile as compared to the emerging markets; moreover, the developed equity markets observe the slowest mean reversion with the highest comparative volatility against the emerging markets. It is further concluded from the results that all the emerging and developed markets observe a mean reverting process because the sum of A.R.C.H. and G.A.R.C.H. is less than one. The previous literature has also found the same conclusion (Ahmad et al., Citation2016; Chi et al., Citation2016; Hart et al., Citation2015; Mishra, Citation2017; Tripathy, Citation2017; Wang et al., Citation2015). The results of the study also demonstrate that there is a direct relation between stock returns and volatilities of the emerging and developed stock markets. These results are also consistent with previous research studies (Balsara et al., Citation2007; Kim et al., Citation2001; Lal, Mubeen, Hussain, & Zubair, Citation2016; Ribeiro et al., Citation2017; Slim et al., Citation2017; Trypsteen, Citation2017). Since the results indicate the mean reversion process in all the 12 emerging and developed markets, the returns of these markets revert back to their original past mean values after the certain time periods. The speed of mean reversion is fast in emerging markets as compared to the developed markets, which have a slow mean reversion process. Therefore, the mean reversion phenomenon provides an opportunity to investors to forecast the future values of the equity returns on the basis of past values. These findings are also consistent with previous literature (Al-Hajieh, Citation2017; Chaudhuri & Wu, Citation2003; Chaves & Viswanathan, Citation2016; Chen et al., Citation2012; Mohammadi, Citation2017; Riaz, Citation2014).
The results of the present study further determined the speed of mean reversion of emerging and developed stock markets by using the half-life method. The half-life method provides insights and wisdom to investors and financial experts to identify the rate at which the prices revert back to their mean value. This will not only help them in the selection of strategies but also plan their entry and exit in the trade. The K.S.E. 100 index shows that the returns reverted back to half of their mean value within 17 days, and this suggested that investors of the K.S.E. 100 index must open a position at 0 days and must close after the 34th day. The K.O.S.P.I. has the slowest mean reversion process, and it takes 307 days to revert back to half of its mean value. Therefore, the investors of the K.O.S.P.I. should open at 0 days and must close after 614th day. Thus, the K.O.S.P.I. has provided the maximum leverage to the investors to operate as compared to the K.S.E. 100, which provides the least time period to operate freely. Therefore, the K.O.S.P.I. has rank 1 and K.S.E. 100 rank 12 among emerging and developed equity markets. Hence, for short-term investment the K.S.E. 100 index could be the choice of market among other emerging and developed markets. But, in contrast, for the long-term investment, it is better to invest in the K.O.S.P.I., which has the slowest mean reversion and the greater half-life.
The research undertaken is a significant contribution to existing literature pertaining to the mean reversion phenomenon and stocks volatility because we examine the behaviour of the equity returns before and after the financial crunch of 2007–2008. Moreover, the current study compared the speed and pace of mean reversion between the important emerging and developed equity markets of the world. The undertaken research also measured the comparative and relative volatilities and relative markets efficiencies. This research is substantial because we have taken major emerging and developed equity markets, which set the trends for other markets, and enable investors to identify the rate at which the prices revert back to their mean value. This will not only help them in the selection of strategies but also plan their entry and exit in the trade. The results of the study further provided the basis for the secure and risk-free investment in emerging and developed financial markets of different regions of the world. Similarly, this research provides a stepping-stone for conducting advance research studies on the subject matter.
This research provides the basic foil for researchers in their advance studies pertaining to the mean reversion, volatility and half-life concept. The potential areas of future research are to incorporate the more robust techniques including asymmetric volatility models such as threshold-A.R.C.H. (T.A.R.C.H.) that also incorporates the signs of the innovation of volatilities, which can influence the volatility of returns. Secondly, future research could be carried out by employing a G.A.R.C.H. (1,1)-X model that also incorporates the exogenous variables of the environment while calculating the mean reversion and volatilities of the equity returns. Future studies may also take important exogenous variables that can influence the stock markets efficiency, and then examine the impact of mean reversion, volatility and half-life on the stock returns. Future study may include the G.A.R.C.H.-M model that establishes the relationship between expected returns and expected volatility.
Disclosure statement
No potential conflict of interest was reported by the authors.
References
- Ahmad, N., Ahmed, R. R., Vveinhardt, J., & Streimikiene, D. (2016). Empirical analysis of stock returns and volatility: Evidence from Asian stock markets. Technological and Economic Development of Economy, 22(6), 808–829.10.3846/20294913.2016.1213204
- Al-Hajieh, H. (2017). Evaluated the success of fractionally integrated-G.A.R.C.H. models on prediction stock market return volatility in gulf arab stock markets. International Journal of Economics and Finance, 9(7), 200–213.10.5539/ijef.v9n7p200
- Annaert, J., & Hyfte, W. V. (2005). Long run mean reversion for the brussels stock exchange: Evidence for the 19th century. EFA 2005 Moscow Meetings Paper, SSRN Electronic Journal. Retrieved from https://doi.org/10.2139/ssrn.676006
- Arefin, M. K., & Ahkam, S. N. (2017). Return and volatility spillover between financial market participants of Dhaka stock exchange using asymmetric G.A.R.C.H. methods. International Journal of Trade, Economics and Finance, 8(3), 133–140.10.18178/IJTEF
- Balsara, N. J., Chen, G., & Zheng, L. (2007). The Chinese stock market: An examination of the random walk model and technical trading rules. Quarterly Journal of Business and Economics, 46, 43–63.
- Balvers, R., Wu, Y., & Gilliland, E. (2000). Mean reversion across national stock markets and parametric contrarian investment strategies. The Journal of Finance, 55(2), 745–772.10.1111/0022-1082.00225
- Banerjee, A., & Sarkar, S. (2006). Modeling daily volatility of the Indian stock market using intraday data. Working Paper No. 588, IIM, Calcutta. Retrieved March 1, 2017, from http://www.iimcal.ac.in/res/upd%5CWPS%20588.pdf
- Bollerslev, T. (1986). Generalized autoregressive conditional heteroskedasticity. Journal of Econometrics, 31(3), 307–327. doi:10.1016/0304-4076(86)90063-1
- Chaudhuri, K., & Wu, Y. (2003). Mean reversion in stock prices: Evidence from emerging markets. Managerial Finance, 29(10), 22–37.10.1108/03074350310768490
- Chaves, D. B., & Viswanathan, V. (2016). Momentum and mean-reversion in commodity spot and futures markets. Journal of Commodity Markets, 3(1), 39–53.10.1016/j.jcomm.2016.08.001
- Chen, Q., Jiang, Y., & Li, Y. (2012). The state of the market and the contrarian strategy: Evidence from China's stock market. Journal of Chinese Economic and Business Studies, 10(1), 89–108.10.1080/14765284.2012.638473
- Chi, Z., Dong, F., & Wong, H. Y. (2016). Option pricing with threshold mean reversion. Journal of Futures Markets, 37(2), 107–131. doi:10.1002/fut.21795
- Covitz, D., Liang, N., & Suarez, G. A. (2013). The evolution of a financial crisis: Collapse of the asset-backed commercial paper market. The Journal of Finance, 68(3), 815–848.10.1111/jofi.12023
- De Bondt, W. F. M., & Thaler, R. (1985). Does the stock market overreact. Journal of Finance, 40(3), 793–805.10.1111/j.1540-6261.1985.tb05004.x
- Dickey, D. A., & Fuller, W. A. (1981). Likelihood ratio statistics for autoregressive time series with a unit root. Econometrica, 49(4), 1057–1072.10.2307/1912517
- Dupernex, S. (2007). Why might share prices follow a random walk? Student Economic Review, 21, 167–179.
- İzgi, B., & Duran, A. (2016). 3D extreme value analysis for stock return, interest rate and speed of mean reversion. Journal of Computational and Applied Mathematics, 297, 51–64.
- Engle, R. F. (1982). Autoregressive conditional heteroskedasticity with estimates of the variance of UK inflation. Econometrica, 50(4), 987–1007.10.2307/1912773
- Engle, R. (2001). G.A.R.C.H. 101: The use of A.R.C.H./G.A.R.C.H. Models in applied econometrics. Journal of Econometric Perspectives, 15(4), 157–168.10.1257/jep.15.4.157
- Fama, E. F., & French, K. R. (1988). Permanent and temporary components of stock prices. Journal of Political Economy, 96(2), 246–273.10.1086/261535
- Gimpel, H. (2007). Preferences in negotiations: The attachment effect. New York, NY: Springer Science & Business Media.
- Goudarzi, H. (2013). Volatility mean reversion and stock market efficiency. Asian Economic and Financial Review, 3, 1681–1692.
- Gulay, E., & Emec, H. (2017). Comparison of forecasting performances: Does normalization and variance stabilization method beat G.A.R.C.H.(1,1)-type models? Empirical evidence from the stock markets. Journal of Forecasting. Retrieved from https://doi.org/10.1002/for.2478
- Hakim, S., & Neaime, S. (2003). Mean-reversion across M.E.N.A. stock markets: Implications for portfolio allocations. International Journal of Business, 8(3), 347–358 doi:10.2139/ssrn.420247
- Hart, C. E., Lence, S. H., Hayes, D. J., & Jin, N. (2015). Price mean reversion, seasonality, and options markets. American Journal of Agricultural Economics, 98, 707–725.
- Hillebrand, E. (2003). Mean reversion model of financial markets. Bremen: University of Bremen.
- Huang, X. (2017). Value-at-risk under Lévy G.A.R.C.H. models: Evidence from global stock markets. CFA Digest, 47(6), 30–53.doi:10.2469/dig.v47.n6.6
- Huggins, D., & Schaller, C. (2013). Fixed income relative value analysis: A practitioners guide to theory. Tools and Trades: John Wiley & Sons Inc.
- Kim, C. J., Morley, J. C., & Nelson, C. R. (2001). Does an intertemporal tradeoff between risk and return explain mean reversion in stock prices? Journal of Empirical Finance, 8(4), 403–426.10.1016/S0927-5398(01)00034-2
- Kuttu, S. (2017). Asymmetric mean reversion and volatility in African real exchange rates. Journal of Economics and Finance. doi:10.1007/s12197-017-9412-z
- Lal, I., Mubeen, M., Hussain, A., & Zubair, M. (2016). An empirical analysis of higher moment capital asset pricing model for Karachi stock exchange (KSE). Open Journal of Social Sciences, 4(6), 53–60.10.4236/jss.2016.46006
- Lubnau, T., & Todorova, N. (2015). Trading on mean-reversion in energy futures markets. Energy Economics, 51, 312–319.10.1016/j.eneco.2015.06.018
- Malliaropulos, D., & Priestley, R. (1996). Mean reversion in Southeast Asian stock markets. Journal of Empirical Finance, 6, 355–384. doi:10.1016/S0927-5398(99)00010-9
- Mishra, S. (2017). Volatility and calendar anomaly through G.A.R.C.H. model: Evidence from the selected G20 stock exchanges. International Journal of Business and Globalisation, 19(1), 126. doi:10.1504/ijbg.2017.10005578
- Mohammadi, M. (2017). Prediction of α -stable G.A.R.C.H. and ARMA-G.A.R.C.H.-M models. Journal of Forecasting, 36(7), 859–866.10.1002/for.v36.7
- Mtunya, A. P., Ngare, P., & Nkansah-Gyekye, Y. (2016). On steady dividend payment under functional mean reversion speed. Journal of Mathematical Finance, 6(3), 368–377.10.4236/jmf.2016.63030
- Mustafa, K., & Ahmed, R. (2013). The random walk model in the Karachi stock market: An empirical investigation. Journal of Economics and Sustainable Development, 4, 262–278.
- Neaime, S. (2015). Are emerging M.E.N.A. stock markets mean reverting? A Monte Carlo simulation. Finance Research Letters, 13, 74–80.10.1016/j.frl.2015.03.001
- Oikarinen, E., & Schindler, F. (2015). Momentum and mean reversion in regional housing markets: Evidence from variance ratio tests. International Journal of Strategic Property Management, 19(3), 220–234.10.3846/1648715X.2015.1031854
- Poshakwale, S. (2003). The random walk hypothesis in the emerging Indian stock market. Journal of Business Finance & Accounting, 29, 1275–1299.
- Riaz, T. (2014). Mean reversion in stock prices: Evidence from Karachi stock exchange. World Academy of Science, Engineering and Technology, International Science Index, Economics and Financial Engineering, 2, 657.
- Ribeiro, P. P., Cermeño, R., & Curto, J. D. (2017). Sovereign bond markets and financial volatility dynamics: Panel-G.A.R.C.H. evidence for six euro area countries. Finance Research Letters, 21, 107–114.10.1016/j.frl.2016.11.011
- Slim, S., Koubaa, Y., & BenSaïda, A. (2017). Value-at-Risk under Lévy G.A.R.C.H. models: Evidence from global stock markets. Journal of International Financial Markets, Institutions and Money, 46, 30–53.10.1016/j.intfin.2016.08.008
- Tie, J., & Zhang, Q. (2016). An optimal mean-reversion trading rule under a Markov chain model. Mathematical Control and Related Fields, 6(3), 467–488.10.3934/mcrf
- Tripathy, N. (2017). Do BRIC countries stock market volatility move together? An empirical analysis of using multivariate G.A.R.C.H. models. International Journal of Business and Emerging Markets, 9(2), 104.10.1504/IJBEM.2017.083353
- Trypsteen, S. (2017). The growth-volatility nexus: New evidence from an augmented G.A.R.C.H.-M model. Economic Modelling, 63, 15–25.10.1016/j.econmod.2017.01.012
- Tsekrekos, A. E., & Yannacopoulos, A. N. (2016). Optimal switching decisions under stochastic volatility with fast mean reversion. European Journal of Operational Research, 251(1), 148–157.10.1016/j.ejor.2015.12.011
- Wang, J., Zhang, D., & Zhang, J. (2015). Mean reversion in stock prices of seven Asian stock markets: Unit root test and stationary test with Fourier functions. International Review of Economics & Finance, 37, 157–164.10.1016/j.iref.2014.11.020
- Zakamulin, V. (2016). Secular mean reversion and long-run predictability of the stock market. Bulletin of Economic Research, 69(4), E66–E93. doi:10.1111/boer.12105