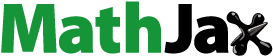
Abstract
Supply chain management (SCM) has a dynamic structure involving the constant flow of information, product, and funds among different participants. SCM is a complex process and most often characterized by uncertainty. Many values are stochastic and cannot be precisely determined and described by classical mathematical methods. Therefore, in solving real and complex problems individual methods of artificial intelligence are increasingly used, or their combination in the form of hybrid methods. This paper has proposed the decision support system for determining economic order quantity and order implementation based on Adaptive neuro-fuzzy inference systems - ANFIS. A combination of two concepts of artificial intelligence in the form of hybrid neuro-fuzzy method has been applied into the decision support system in order to exploit the individual advantages of both methods. This method can deal with complexity and uncertainty in SCM better than classical methods because they it stems from experts’ opinions. The proposed decision support system showed good results for determining the amount of economic order and it is presented as a successful tool for planning in SCM. Sensitivity analysis has been applied, which indicates that the decision support system gives valid results. The proposed system is flexible and can be applied to various types of goods in SCM.
1. Introduction
Scientific and technological progress, in coordination with economic development, affects all areas of the economy and its capabilities are used in the search for solutions for better organisation and efficiency of the supply chain. According to Stadtler, Kilger, and Meyr (Citation2015), supply chain management (SCM) is a set of rules and procedures by which the transport chain provides raw materials, semi-finished or finished products necessary for the implementation of the production process, and the needs of users or consumers.
In recent years, SCM disruptions have impacted the performance of companies (Ho, Zheng, Yildiz, & Talluri, Citation2015). Previously, the most productive and commercial organisation could make profit despite the inefficient SCM (Tempelmeier, Citation2011). Today that is not the case, because most companies operate at small profit levels (Bokor, Citation2012). Cost reduction involves rationalisation in all areas of the company (Stevic, Pamučar, Vasiljevic, Stojic, & Korica, Citation2017), and research in the field of SCM has shown that it is possible to achieve savings of up to 30% (Chen, Chen, Fan, & Huang, Citation2013).
One of the main objectives of SCM is to organise and coordinate the flow of producing products from manufacturer to retailers that must meet the needs of the end users (for this purpose end customers’ value expectations). The paper discusses data issues and decision support system designing in SCM for the economic order quantity (EOQ) of the final product by a retailer.
Taking into account the current knowledge in the field of SCM (Azadeh, Zarrin, Saberi, & Ebrahimi, Citation2015; Bhatnagar & Chee-Chong, Citation2009; Fallahpour, Wong, Olugu, & Musa, Citation2017), researches in the paper focus on whether the dynamic model on the principle of the hybrid method of artificial intelligence (Abdollahzade, Miranian, Hassani, & Iranmanesh, Citation2015; Fallahpour, Olugu, Musa, Khezrimotlagh, & Wong, Citation2016; Ghorabaee, Amiri, Zavadskas, & Antucheviciene, Citation2017; Tamosaitiene, Zavadskas, Sileikaite, & Turskis, Citation2017; Tavana, Fallahpour, Di Caprio, & Santos-Arteaga, Citation2016) can be used for planning, organising and implementation of order quantity. During the decision support system design (Zavadskas, Antucheviciene, Vilutiene, & Adeli, Citation2017), all the peculiarities of SCM processes were taken into account, as well as the nature and degree of the influence of key factors in determining EOQ for the observed company and the type of goods.
The basic hypothesis of this paper is that it is possible to design a decision support system on a hybrid neuro-fuzzy approach of artificial intelligence to determine EOQ and its realisation by a retailer. Such a system can effectively be used in the observed company in a highly dynamic and changing business environment. One of the objectives is that the proposed system shall be flexible and applicable in other companies for other types of goods in SCM. The basic motive for the design of such a decision support system is the development of the tool for EOQ that will be able to fully imitate, control and replace the work of logistics experts, or perform complex and real processes of SCM using a hybrid artificial intelligence technique.
Combining the neural network and fuzzy system concepts in order to combine the advantages of both the techniques, addresses their respective limitations in the real business world. The main idea of adaptive neuro-fuzzy techniques is based on fuzzy modelling methods and learning based on a given set of data. Thanks to these advantages, in this paper, the authors have chosen to develop a model for determining economic order quantity and order implementation.
The basic contribution of this paper is that the proposed model is a real application and it is used to solve the specific problem of determining the quantity of order of the finished product taking into account all the specifics for the order of the given type of goods. Compared to existing models, the benefits are complex and integrate considerations of many parameters that influence the decision on the determination of EOQ and the realisation of procurement. In this sense, when determining the parameters of the defined criteria, the learning effect model with imprecise parameters has been applied.
Another advantage is that the model has been developed by countering the assumption of full transfer of learning, i.e., the model that completely mimics the work of an experienced expert. The learning ability of the connectionist neural networks is incorporated into the human as reasoning ability of the fuzzy systems.
The third advantage of the model is that it is flexible and with small modifications and entering specificities of input parameters, it can be upgraded and used to determine the EOQ of other types of goods, semi-products and raw materials. The proposed model addresses various difficult research problems in a business system that absorbs new knowledge (being able to combine different styles and showing creativity).
The rest of this paper is organised as follows. The relevant literature review is classified and reviewed in Section 2. Section 3 describes ANFIS (Adaptive Neuro-Fuzzy Inference Systems) techniques used in the proposed methodology. Section 4 presents decision support system design, proposed models, a sensible analysis for different membership functions was made and discussion. Conclusion remarks are drawn in Section 5.
2. Literature review
Many phenomena in nature, society and the economy cannot be described and it is not possible to predict their behaviour by traditional mathematical methods. Due to the lack of flexibility of this approach, the human factor compensates for the uncertainty of mathematical modelling using knowledge based on experience and the ability to make decisions based on data that are difficult to enter into a mathematical model (Efendigil, Citation2014; Jovanoski, Minovski, Lichtenegger, & Voessner, Citation2013).
A century ago, Harris (Citation1913) introduced the EOQ inventory model. Most companies apply the EOQ model to determine the maximum level of inventory or ordering lot size (Ali, Paul, Ahsan, & Azeem, Citation2011). The application of classical methods for EOQ is based on limited assumptions that cannot cover the nature of modern complex logistics processes such as - demand is constant in unit time, lead time is deterministic and stationary, constant price etc (Maddah & Noueihed, Citation2017). But, making decisions in SCM takes place in an environment where objectives and constraints are not and cannot often be precisely defined (Latif, Paul, & Azeem, Citation2014; Seker, Erol, & Botsali, Citation2013; Taleizadeh, Khanbaglo, & Cárdenas-Barrón, Citation2016). Therefore a certain approximation is required in order to obtain a high quality model of a real system where the application of artificial intelligence has an important role (Sremac, Gladovic, Đelosevic, Matic, & Matic et al., Citation2013). Consequently, individual methods of artificial intelligence (Keshavarz Ghorabaee, Zavadskas, Amiri, & Antucheviciene, Citation2016) or their combination in the form of a hybrid method are increasingly used in solving real and complex problems (Liou, Tamošaitienė, Zavadskas, & Tzeng, Citation2016; Radeerom & Kulthon, Citation2013; Teksan & Geunes, Citation2016; Zavadskas, Govindan, Antucheviciene, & Turskis, Citation2016).
Some researchers (Davis-Sramek & Fugate, Citation2007) interviewed a few visionaries in the field of SCM and recognised the irresistible call of these individuals for modelling and simulation to be involved in the research (Wallin, Rungtusanatham, & Rabinovich, Citation2006). Modelling of the SCM seeks for the best possible system configurations to minimise costs and increase operational efficiency in order to meet customer expectations (Bowersox, Closs, & Cooper, Citation2010). Modelling of SCM and logistics problem solving, traditionally relies on three basic methods: optimisation, simulation and heuristics (Griffis, Bell, & Closs, Citation2012). An important issue in SCM is the need to make the right decision, despite the occurrence of significant ambiguity (Giannoccaro, Pontrandolfo, & Scozzi, Citation2003). In addition to fluctuations in demand and delivery times, vagueness is associated with the lack of information from the production and distribution processes in SCM (Chatfield, Hayya, & Cook, Citation2013; Wu, Kazaz, Webster, & Yang, Citation2012). Some authors expressed uncertainty of market demand and inventory costs (Azizi, Yazid B. Ali, & Wei Ping, Citation2015; Nakandala, Lau, & Zhang, Citation2014) in the model theory of fuzzy sets (Gumus & Guneri, Citation2009).
There is a review of some works from the field of SCM based on the neuro-fuzzy approach. Jang (Citation1993) first introduced the ANFIS method by embedding the Fuzzy Inference System into the framework of adaptive networks. Demand uncertainty is considered in the optimisation model of Gupta and Maranas (Citation2003) in which by a two-stage stochastic programming model they consider all production decisions in the first stage and all the supply chain decisions in the second. Didehkhani, Jassbi, and Pilevari (Citation2009) used ANFIS for evaluating flexibility in a supply chain. Atsalakis and Valavanis (Citation2009) applied ANFIS to create a forecasting system that predicts the next day’s trend for a stock. Yazdani-Chamzini, Yakhchali, Volungevičienė, and Zavadskas (Citation2012) used ANFIS and an artificial neural network (ANN) model for modelling the gold price.
Later on Holimchayachotikul, Leksakul, Montella, and Savino (Citation2010) proposed a predictive system based on the neuro-fuzzy concept, multi-criteria decision attributes and B2B supply chain performance evaluation system for the supply chain. Guneri, Ertay, and Yucel (Citation2011) developed a new method using ANFIS for the supplier selection problem. Vahdani, Iranmanesh, Meysam Mousavi, and Abdollahzade (Citation2012) presented numerous quantitative methods for supplier selection and evaluation in the literature, where the most current technique is Hybrid approaches. Later Ozkan and Inal (Citation2014) employed ANFIS in supplier selection and evaluation process. Aksoy, Öztürk, and Sucky (Citation2014) applied ANFIS for demand prediction in an apparel industry.
Several methods for EOQ in SCM have appeared in the literature, including approaches based on a neuro-fuzzy (Yazdani, Zarate, Coulibaly, & Zavadskas, Citation2017). Efendigil, Onut, and Kahraman (Citation2009) developed a comparative forecasting mechanism based on ANN and ANFIS techniques to manage the demand forecasting issue under fuzziness. Paul, Azeem, and Ghosh (Citation2015) presents the application of ANFIS and ANN in an inventory management problem to determine optimum inventory level. Abdel-Aleem, El-Sharief, Hassan, and El-Sebaie (Citation2017) study and analyse the optimal lot size in a real production system to obtain the optimal production quantity.
ANFIS has a wide application in the fields of finance, marketing, distribution, business planning, information systems, production, logistics etc (Ambukege, Justo, & Mushi, Citation2017; Mardani et al., Citation2017; Pamučar & Ćirović, Citation2018; Rajab & Sharma, Citation2017; Stojčić, Citation2018; Svalina, Galzina, Lujic, & Simunovic, Citation2013; Xiao et al., Citation2014; Yazdani-Chamzini, Zavadskas, Antucheviciene, & Bausys, Citation2017). Efficient SCM, including EOQ, is crucial for survival and success in a turbulent world. SCM is one of the most researched topics in recent years and most of the papers are focussed on artificial intelligence methods for supply chain optimisation (Groznik & Trkman, Citation2015).
In comparative analysis of classical methodologies, individual artificial intelligence methodologies and ANFIS methodology applied in the paper for the determination of EOQ have been carried out. The basic criteria have been defined as the characteristics for each of the three groups of the mentioned methodologies and they have been mutually compared. The classic method is one of the oldest EOQ models in the fields of operations and inventory management (Axsäter, Citation1996; Harris, Citation1913), however, it has limited assumptions and cannot be adapted and cannot efficiently function in today’s environment. The application of individual methods of artificial intelligence (Çelebi, Citation2015; Kazemi, Olugu, Abdul-Rashid, & Ghazilla, Citation2016; Mondal & Maiti, Citation2003; Roy & Maiti, Citation1997) developed more flexible models compared to the classic ones characterised by simplicity and robustness. Individual artificial intelligence methods have many advantages as listed in , but they are not able to cover all the specificities of the order process in SCM, and thus developed models lack sensitivity and flexibility above all.
Table 1. Differences between studies for the determination of EOQ applying the classical, individual and hybrid methodology used in the paper
Under the complex conditions of functioning of the real business world, the best results are achieved by applying some of the hybrid methods of artificial intelligence, as confirmed by the papers: Zavadskas et al., Citation2016; Yazdani et al., Citation2017; Teksan & Geunes, Citation2016; Liou et al., Citation2016 etc. This, in solving the complex problem of determining EOQ for a particular type of goods, was confirmed during the research in this paper, where the application of ANFIS gave good results.
3. Adaptive neuro-fuzzy inference systems
ANFIS are the modern class of hybrid systems of artificial intelligence. They are described as artificial neural networks characterised by fuzzy parameters. By combining two different concepts of artificial intelligence we can try to exploit the individual strengths of fuzzy logistics and artificial neural networks in hybrid systems of homogeneous structure. Such engineered systems are increasingly used to solve everyday complex problems, and with the assistance of logistics experts and historical data, this approach can be designed on the basis of computer aided systems.
The decision support system for EOQ is based on the integration of the concepts of fuzzy logic and artificial neural networks. Fuzzy logic, based on fuzzy sets, provides the mathematical potential for describing ambiguity related to cognitive processes in humans, such as thinking and reasoning (Ghazinoory, Esmail Zadeh, & Kheirkhah, Citation2010). Using fuzzy logic it is possible to make conclusions on the basis of incomplete and insufficiently precise information, so-called approximate reasoning.
Fuzzy logic was created as a result of attempts to model human thinking, experience and intuition in the decision-making process based on inaccurate data. It is suitable for expressing vagueness and uncertainty. To explain the meaning of the term fuzzy logic, one can use the explanation of Zadeh who is its ideological creator (Teodorovic & Vukadinovic, Citation1998). A large number of sets we face in reality has no precisely defined boundaries that separate elements in a set of elements outside the set.
A fuzzy set A of set X can be defined as a set of ordered pairs given in Equation(1)(1)
(1) .
(1)
(1)
Where X is a set of considerations in which a fuzzy set A is defined and μA (x) is a membership function of the element x of the set A. The fuzzy logic introduces the notion of a membership function which is interpreted as a degree of truthfulness of the claim, and thus it is‘closer’ related to problems and events from everyday life. Each fuzzy set is completely determined by its membership function which represents the degree of belonging of the elements x to the fuzzy set A, which is represented in Equation(2)(2)
(2) .
(2)
(2)
Fuzzy numbers are defined as convex normalised fuzzy sets. A fuzzy set is normalised if at least one element belongs to this set with degree of belonging 1.
According to the fuzzy theory, the choice of the membership function, i.e., the form of the function and the size of the confidence interval, is most often performed on the basis of subjective assessment or experience. The most common forms of the membership function are: triangular, trapezoidal, Gaussian, and bell-shaped. The Gaussian fuzzy number is applied in the paper, which is defined with two parameters A = [σ; c], The membership function can be defined as shown in Equation(3)(3)
(3) .
(3)
(3)
On the other hand, artificial neural networks, with their different architectures built on the concept of artificial neurons, were developed to imitate biological nervous systems in performing functions such as learning and pattern recognition. While fuzzy logic provides a mechanism for reasoning with incomplete and insufficiently precise information, artificial neural networks provide opportunities for learning, adaptation and generalisation (Ćirović & Pamučar, Citation2013). Neural networks can learn from an example – automatically, but it is difficult to describe knowledge gained in this way. On the other hand, fuzzy logic makes approximate reasoning possible, but it does not have the characteristic of adaptability.
Ability to display the model in the form of fuzzy neural networks is commonly used in automatic procedures for determining parameters of fuzzy model based on the available input-output data (Negnevitsky, Citation2011).
The structure of the neuro-fuzzy intelligent systems applied in the paper is similar to the neural network. Membership functions of the input data are mapped to the input of neural network and the output data via neural networks to define the input-output legality ().
Parameters typical for the corresponding membership functions are changed through the process of network learning. The calculation of these parameters is usually done on the basis of the vector gradient, which represents a measurement accuracy of the mapping of fuzzy system reasoning input to the output data set for a given set of the checked parameters (Cetisli, Citation2010).
ANFIS have the described structure of the neuro-fuzzy intelligent systems. The basic idea of ANFIS is based on the methods of fuzzy modelling and learning according to the given data set. The introduction of fuzzy systems in neural networks uses a learning ability of neural networks to improve the performance of the model which gave much better results during the survey than using only one concept of artificial intelligence. Corresponding fuzzy inference system is created and parameters of membership functions are calculated according to the input-output data set. Parameters of functions that are members of a fuzzy system can be adjusted using the backpropagation algorithm or a combination of the aforementioned algorithm and least square method. This setting allows fuzzy system learning based on input-output data set. This method of learning is similar to the method of neural networks. represents ANFIS with five layers (Azarbad, Azami, Sanei, & Ebrahimzadeh, Citation2014). The output of each layer is represented by where i is the i-th node of the first layer.
The adaptive neuro-fuzzy inference system operates in the following manner (Efendigil et al., Citation2009):
The nodes in the first layer represent linguistic variables of the input variables quantified with fuzzy sets. In the first layer the degree of membership is calculated:
(4)
(4)
(5)
(5)
Where:
and
are the corresponding membership functions for fuzzy sets Ai and Bi.
All elements in this layer are fixed differently from the first layer with the adaptive elements. The output of each node of the second layer represents the intensity of activation of the corresponding rule. Relation AND is used, i.e., operator product:
(6)
(6)
In the i-node the ratio of the intensity of appropriate activation and the sum of the intensity of activation of the corresponding rules is counted:
(7)
(7)
The output values are calculated based on conclusion rules in the fourth layer. For an i-th node output value rule is calculated:
(8)
(8)
Where:
is normalised rule activation intensity and
represent a modified parameters set.
In the output node all input intensities are summarised:
(9)
(9)
ANFIS calculate error function as follows:
(10)
(10) Where:
is a desired output and i
is an output from the model.
ANFIS only support fuzzy systems of Sugeno type (Tahmasebi & Hezarkhani, Citation2010). An initial Sugeno fuzzy inference system was formed after defining the parameters of the membership functions of the input-output variables. Benefits of Sugeno type are that it is computationally more efficient, suitable for mathematical analysis, works well with linear, optimisation and adaptive techniques. The formation of the proposed neuro-fuzzy model consists of the following steps:
Determination of input-output data set in the form customised for training of the neuro-fuzzy inference system.
The model structure with parameters is assumed, which by the rules reflects the input membership functions into output functions.
The model is trained on the training data. In doing so, the parameters of the membership functions are modified according to the selected error criterion in order to get the valid model results.
This way of modelling is appropriate if the training data are fully representative for all the properties that the neuro-fuzzy model should have. In some cases, the data used to train the network contain measurement errors so they are not fully representative for all features that should be included in the model. Therefore, the model should be checked using the testing data. There are two ways of testing the model. The first way is to check the model when input data are those that are not used for training. This procedure shows how accurately the model predicts the output value set and it is implemented in the paper. Another way to test the model is a mathematical procedure when the data that were used for training are now used as a data set for testing and it is necessary to obtain the output with a minimal error.
4. Decision support system design
In this paper, the decision support system for the economic order quantity in SCM has been developed. Decision support system is used to analyse and estimate EOQ (Lee, Lin, & Pasari, Citation2014), and it decides whether to realise an order. Due to the complexity of the issue, decision support system is divided into two interdependent models:
The economic order quantity model (EOQ Model) and
Order implementation model (OI Model).
shows the structure of the proposed decision support system. The input variables in EOQ Model are: the size of demand, inventory levels and prices. The output result of that model is EOQ that, with the data on the availability of vehicles and emergency of order, represents the input variables in the OI model. The output variable, the order implementation, has two options: the order is carried out or not. If the order is realised, the final result of the decision support system is the economic order quantity by EOQ Model. If the order is not realised, the process of determining the quantities of supplies starts again. The criterion of optimal order is the size of the errors in the testing and training network.
Decision support system was formed on the basis of a company that successfully operates in SCM. Input values were determined by interviewing experienced logistics experts in the company which incorporated all parameters relevant for EOQ and its implementation into proposed input values. Decision support system was tested in a specialised trading company of electrical products on the example of the electrical cables that are widely used. The main packages of the electrical cables are coils of cable with the length of 100 m, which are supplied packaged in heat ‘stretch’ foils and cardboard boxes.
4.1. Proposed models
The main reason for presenting the fuzzy system with neural networks is the use of learning ability of neural networks to improve the performance of the model. The aim of the neuro-fuzzy modelling is to reduce the role of the expert in the development of the model and the use of specific data of a business enterprise for the formation of the model. Decision support system for the EOQ was developed in the MATLAB environment.
Proposed models in decision support system have the form of a multilayer neural network with feed forward. The first layer is the input variable, the hidden (middle) layer represents fuzzy rules and the third layer is the output variable. Fuzzy sets are defined as connection weights between nodes. In the adaptive nodes the adjustment is implemented in order to reduce error that is obtained at the output of the model. Error represents the difference between the known output values and the values that are obtained at the output of the neuro-fuzzy networks (Karaboga & Kaya, Citation2018). Reports are spread forward and the errors are spread backward. Thus, output numerical value approaches the optimum, i.e., requested value.
Data set for training the neural network was obtained on the basis of concrete data on the operations of the observed companies. We used a hybrid optimisation method which consists of:
backpropagation method (back propagation algorithm), which determines the error variables recursively from the output layer to the input layer and
the least squares method to determine the optimal set of effect parameters.
The structure of fuzzy models mapped in a neural network for individual models is shown in . At EOQ Model, size of the input demand variable has five values, while the number of membership functions of input variables (inventory level and prices) is three. The input variable size of demand has the values in the interval [0, 250], inventory level is in the interval [0, 200], while the price is presented in linguistic values in the interval [1, 3]. The interval of the output variable economic order quantity is [0, 250]. Regarding the OI model, the input variable economic order quantity has values in the interval [0, 250], availability of the vehicle in the interval [0, 10], since the company owns 10 vehicles and the emergency of order is expressed by linguistic values (small, medium and large) in the interval [0, 1]. The output variable preference order is expressed by linguistic values (very small, small, medium, large and very large) in the interval [0, 1]. In case the supply preference is within the limits of 0 ÷ 0.4, the procurement is not realised, and if the preference of procurement is within the limits of 0.4 ÷ 1, the procurement is realised. If the preference value is 0.4 the priority is given to the realisation of the procurement. In the case of the OI model, the number of membership functions of the input variable, quantity order and availability of the vehicle is five, while the urgency of the order has three values. Membership functions have the shape of a Gaussian curve, because in similar studies (Atsalakis & Valavanis, Citation2009; Guneri et al., Citation2011) this form of curve gave the best results.
represents parameters used in both models according to which the decision support system was designed.
Table 2. Parameters of neuro-fuzzy decision support system
We applied the technique of network sharing (grid partition) to generate single output models and hybrid optimisation method. It is assumed that the output membership functions are of the constant type. Number of training cycles (epochs) is 500 (), although in the OI model it has been shown that the minimum error is achieved after only 15 epochs ().
In EOQ Model 50 input-output data sets were used for the network training. Interval of the output variable quantity procurement is 50 to 250 units of supply. In EOQ Model there is an error at the exit of the neural network that amounts to 2.15 ().
In OI Model there is an error at the exit of the neural network that amounts to 0.122 (). One hundred input-output data sets for decision on implementation of the procurement were used for the network training. The output variable of OI Model has two values: 1 - supply is not realised and 2 - supply is implemented.
After the training phase, the neural network has been tested on the basis of input-output data sets that were not used during the training model. Testing enables verification of the functioning of the neuro-fuzzy model. Output data, which were generated by the network, are compared with the available data. Deviations in the functioning of the model must be within the boundaries of the tolerance provided.
The average error in testing the neural network of EOQ Model was 4.03. shows that there is a high agreement of the known and results obtained on a basis of testing of 10 cases of EOQ that were not used in training of the neural network.
The average error in testing the neural network of OI model was 0.24. shows that there is a high agreement between the decisions made by the company expert and the decisions made by the model. Testing of 20 cases of determining the OI has shown that the model works with deviations within the boundaries of provided tolerance and that the proposed model gives valid results.
The constant question during the neuro-fuzzy modelling is how many data should be used for training, and how many for testing? In the literature various relationships could be found and they are distinctive for each problem (Cetisli, Citation2010). In the case, we used 83.32% of the data for training and 16.67% for the testing model.
Testing the model allowed testing the functioning of the proposed EOQ model. The output data generated by the network were compared with known data of the company. shows that there is a high agreement between the actual and obtained results. The average relative error of the EOQ Model is 3.28% and on the basis of this analysis, it can be said that the EOQ model gives valid results.
Table 3. EOQ model testing
OI model was tested on the basis of input-output data sets that were not used in the training phase of the model. We compared the results given by the model and the actual results of the company for the same input parameters. Testing of OI model () showed a high level of agreement between decisions made by experts in the company and the model decisions (95%), thus proving that the OI model gives valid results.
Table 4. OI model testing (1 - order is not realised and 2 - order is implemented)
By testing the EOQ and OI models acceptable values were obtained and the proposed decision support system was verified.
4.2. Sensitivity analysis
One of the basic requirements of the modelling is to achieve a sufficient sensitivity model. This means that when certain small changes happen in input variables, the output of the model must also have a small change in the value. Sensitivity analysis of the decision support system was performed by changing the shape of the membership functions of input variables and the number of values of input variables for each individual EOQ and OI model (). Instead of Gaussian curves, tested membership functions are triangular, trapezoidal, and bell-shaped.
Table 5. Sensitivity analysis of the decision support system
For defined cases of testing the sensitivity of EOQ and OI models, the average errors obtained by training and testing of the network reveal negligible differences, indicating that the decision support system gives valid results.
4.3. Discussion
The basic purpose of this chapter is, using the comparative analysis, to compare the results of the classical fuzzy and hybrid neuro-fuzzy model, to note the advantages and disadvantages of the applied artificial intelligence methods and, based on the analysis, to apply one of the methods mentioned above.
In the first phase of the research, the decision support system for EOQ was formed on the principle of fuzzy logic. Below are the basic results of the models that are used for comparative analysis. The basic elements of the fuzzy decision support system (EOQ model, OI model, structure, input and output variables, number of membership functions, curve form) are the same as with the already presented ANFIS decision support system. The appearance of the membership functions of the EOQ model is presented in , while the parameters of the membership functions are listed in .
Figure 8. Fuzzy set membership functions in fuzzy EOQ model a) size of demand, b) inventory level, c) prices and d) order quantity.
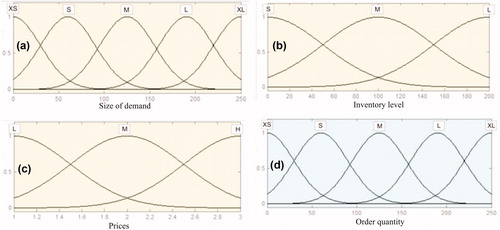
The second part of the decision support system related to the realisation of the procurement is presented in .
Figure 9. Fuzzy set membership functions in fuzzy OI model a) order quantity, b) availability of vehicles, c) emergency of order and d) order implementation.
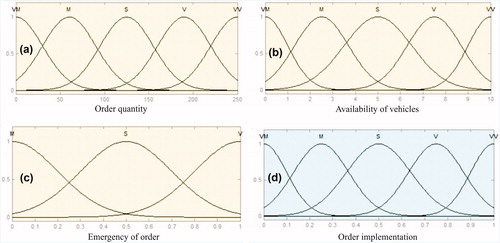
The summarised graphical presentation of the output variable of the decision support system for both fuzzy models depending on the input variables is presented in .
The basic characteristics of the decision support system formed on the basis of the fuzzy and ANFIS methods are shown in .
Table 6. Comparative analysis of fuzzy and ANFIS decision support system
The results of the proposed models are compared using comparative analysis. The comparison criterion is the average relative error of the model's results. The errors of the proposed models are within the tolerance limits. By testing the EOQ model, the average relative error of the ANFIS model (3.28%) is significantly lower than the fuzzy model (5.6%). The error of the fuzzy OI model (7.3%) is higher than the ANFIS model OI (5%). During the research, the aim was to improve the performance of the fuzzy model using the ANFIS method by forming a decision support system. The comparative analysis showed that relative errors are less in the ANFIS model, i.e., the application of the neuro-fuzzy method gives more precise results. Training of the neural network has given improved membership functions with new parameters (). Based on the conducted research and the results obtained, the advantage can be given to the application of hybrid neuro-fuzzy systems of artificial intelligence in regard to the fuzzy systems.
During the research, it was noted that in addition to the advantages mentioned in the paper, each of the applied concepts of artificial intelligence has certain disadvantages, and that none of the tools is universally applicable. The observed disadvantages of the fuzzy logic are that the process of developing the fuzzy model is complex, and that the choice and adjustment of the membership functions of the variables are a very sensitive field that has a significant impact on the results of the model.
Using a neuro-fuzzy method, training time of the neuronal network usually requires a large amount of data and can be very long, and therefore the need for frequent repetitions of the training can make the model unusable. A small number of input parameters gives rough and imprecise results, so the end results of the model have a greater deviation from the real results.
5. Conclusion
In a highly dynamic and changing business environment, firms should build effective retailers by applying adequate scientific techniques in SCM. In this paper, a technique of artificial intelligence is presented, based on the hybrid ANFIS method, for determining EOQ and its realisation by retailer.
Here, the basic hypothesis in this paper, that the decision support system on the neuro-fuzzy approach can effectively manage one part of SCM, has been confirmed in a systematic way. The proposed decision support system showed good results and it is presented as a very successful tool for planning, organising and implementation of the EOQ model. The model is flexible, encompasses a time-dependent request, and can be applied to various kinds of goods flow in SCM. It contributes to the efficient SCM, and therefore to the greater satisfaction of socio-economic needs of citizens and businesses.
The advantages of such a designed decision support system have been used for modelling of complex and real processes of SCM in which it can be difficult to determine the interdependence of the variables presented by the application of other methods. The application of this method has enabled the integration of large amounts of data in the model and allowed that the proposed model can fully imitate the work of logistics experts in the observed company.
The proposed neuro-fuzzy model has several main advantages over other methods. First, in relation to the classic models, it has the ability of adaptability, which is reflected in the possibility of setting the base of the fuzzy rules. Fuzzy rules are very important for managing such systems, especially for a descriptive approach that prefers intuitive, heuristic search of solutions in the process of economic order quantity. This flexibility allows overcoming the limitations of conventional models for the economic order quantity that perform a prediction of the flow through the observation of parameters without the intervention of intuitiveness. Secondly, the shown neuro-fuzzy model is effective under the conditions of uncertainty and can provide support to decision makers when there is uncertainty in logistic processes and activities. Thirdly, it can be implemented as a computer system that supports a dynamic decision-making process for economic order quantity.
Decision support system, besides being scientific, has a practical feature, as it has been tested in commercial practice in a particular company, which is the current intention of the research in a large portion of the scientific community. The proposed model is adjusted to the needs of the business of the observed company and with minor modifications it can be applied to any company that deals with the realisation of the flow of goods.
The disadvantage of the neuro-fuzzy modelling is that time for the training of the neuro-fuzzy network requires a large number of data and it can be long as well, so the need for frequent repetitions of training can make the application unusable.
In future researches, in the case of business and the needs of other companies and new market trends, the proposed model can be extended by introducing a larger number of input variables, conducting a detailed economic quantification of costs (ordering costs, inventory holding costs) and covering other logistics processes in SCM (Abdulshahed & Badi, Citation2018; Yazdani et al., Citation2017). The proposed model can be used for determination of the amount of order of other types of goods, raw materials or semi-finished products, with adaptation of the input model parameters to specificity for the given order. In order to control and mitigate the negative effects caused by SCM disruptions (Sreedevi & Saranga, Citation2017), a significant amount of work in the area of supply chain risk management is undertaken in both academia and practitioner circles.
One of the aims of the research was to improve the computer aided system in order to optimise logistics processes in SCM, which was achieved by reducing the role of a logistics expert. Also, the distributed system is computationally more efficient and can be implemented in the information system. This effect can be increased by harmonising the existing decision support system with MRP. It is a complex procedure that requires thorough research and a detailed explanation and as such it will be the subject of further researches.
Acknowledgements
The paper is a part of the research done within the project TR 36030. The authors would like to thank to the Ministry of Education, Science and Technological Development of the Republic of Serbia.
Disclosure statement
No potential conflict of interest was reported by the authors.
Additional information
Funding
References
- Abdel-Aleem, A., El-Sharief, A. M., Hassan, A. M., & El-Sebaie, G. M. (2017). Implementation of fuzzy and adaptive neuro-fuzzy inference systems in optimization of production inventory problem. Applied Mathematics & Information Sciences, 11(1), 289–298. doi:10.18576/amis/110135
- Abdollahzade, M., Miranian, A., Hassani, H., & Iranmanesh, H. (2015). A new hybrid enhanced local linear neuro-fuzzy model based on the optimized singular spectrum analysis and its application for nonlinear and chaotic time series forecasting. Information Sciences, 295, 107–125. doi:10.1016/j.ins.2014.09.002
- Abdulshahed, A., & Badi, I. (2018). Prediction and control of the surface roughness for the end milling process using ANFIS. Operational Research in Engineering Sciences: Theory and Applications, 1(1), 1–12. doi:10.31181/oresta1901201011a
- Aksoy, A., Öztürk, N., & Sucky, E. (2014). Demand forecasting for apparel manufacturers by using neuro-fuzzy techniques. Journal of Modelling in Management, 9(1), 18–35. doi:10.1108/JM2-10-2011-0045
- Ali, S. M., Paul, S. K., Ahsan, K., & Azeem, A. (2011). Forecasting of optimum raw material inventory level using artificial neural network. International Journal of Operations and Quantitative Management, 17(4), 333–348.
- Ambukege, G., Justo, G., & Mushi, J. (2017). Neuro fuzzy modelling for prediction of consumer price index. International Journal of Artificial Intelligence & Applications, 8(5), 33–44. doi:10.5121/ijaia.2017.8503
- Atsalakis, G. S., & Valavanis, K. P. (2009). Forecasting stock market short-term trends using a neuro-fuzzy based methodology. Expert Systems with Applications, 36(7), 10696–10707. doi:10.1016/j.eswa.2009.02.043
- Axsäter, S. (1996). Using the deterministic EOQ formula in stochastic inventory control. Management Science, 42(6), 830–834. doi:10.1287/mnsc.42.6.830
- Azadeh, A., Zarrin, M., Saberi, M., & Ebrahimi, Z. (2015). Improved trust prediction in business environments by adaptive neuro fuzzy inference systems. International Journal of Emerging Technology and Advanced Engineering, 5(6), 19–26.
- Azarbad, M., Azami, H., Sanei, S., & Ebrahimzadeh, A. (2014). New neural network-based approaches for GPS GDOP classification based on neuro-fuzzy inference system, radial basis function, and improved bee algorithm. Applied Soft Computing, 25, 285–292. doi:10.1016/j.asoc.2014.09.022
- Azizi, A., Yazid B. Ali, A., & Wei Ping, L. (2015). Modelling production uncertainties using the adaptive neuro-fuzzy inference system. The South African Journal of Industrial Engineering, 26(1), 224–234. doi:10.7166/26-1-560
- Bhatnagar, R., & Chee-Chong, T. (2009). Role of logistics in enhancing competitive advantage: A value chain framework for global supply chains. International Journal of Physical Distribution & Logistics Management, 39(3), 202–226. doi:10.1108/09600030910951700
- Bokor, Z. (2012). Cost calculation model for logistics service providers. Promet – Traffic & Transportation, 24(6), 515–524.
- Bowersox, D. J., Closs, D. J., & Cooper, M. B. (2010). Supply chain logistics management. New York, NY: McGraw-Hill.
- Çelebi, D. (2015). Inventory control in a centralized distribution network using genetic algorithms: A case study. Computers & Industrial Engineering, 87, 532–539. doi:10.1016/j.cie.2015.05.035
- Cetisli, B. (2010). Development of an adaptive neuro-fuzzy classifier using linguistic hedges: Part 1. Expert Systems with Applications, 37(8), 6093–6101. doi:10.1016/j.eswa.2010.02.108
- Chatfield, D. C., Hayya, J. C., & Cook, D. P. (2013). Stockout propagation and amplification in supply chain inventory systems. International Journal of Production Research, 51(5), 1491–1507. doi:10.1080/00207543.2012.697205
- Chen, M. Y., Chen, D. R., Fan, M. H., & Huang, T. Y. (2013). International transmission of stock market movements: An adaptive neuro-fuzzy inference system for analysis of TAIEX forecasting. Neural Computing and Applications, 23(S1), 369–378. doi:10.1007/s00521-013-1461-4
- Ćirović, G., & Pamučar, D. (2013). Decision support model for prioritizing railway level crossings for safety improvements: Application of the adaptive neuro- fuzzy system. Expert Systems with Applications, 40 (6), 2208–2223. doi:10.1016/j.eswa.2012.10.041
- Davis-Sramek, B., & Fugate, B. (2007). State of logistics: A visionary perspective. Journal of Business Logistics, 28(2), 1–34. doi:10.1002/j.2158-1592.2007.tb00056.x
- Didehkhani, H., Jassbi, J., & Pilevari, N. (2009). Assessing flexibility in supply chain using adaptive neuro fuzzy inference system. International Conference on Industrial Engineering and Engineering Management, Hong Kong, China.
- Efendigil, T. (2014). Modelling product returns in a closed-loop supply chain under uncertainties: A neuro fuzzy approach. Journal of Multiple-Valued Logic and Soft Computing, 23(3-4), 407–426.
- Efendigil, T., Onut, S., & Kahraman, C. (2009). A decision support system for demand forecasting with artificial neural networks and neuro-fuzzy models: A comparative analysis. Expert Systems with Applications, 36(3), 6697–6707. doi:10.1016/j.eswa.2008.08.058
- Fallahpour, A., Olugu, E. U., Musa, S. N., Khezrimotlagh, D., & Wong, K. Y. (2016). An integrated model for green supplier selection under fuzzy environment: Application of data envelopment analysis and genetic programming approach. Neural Computing and Applications, 27(3), 707–725. doi:10.1007/s00521-015-1890-3
- Fallahpour, A., Wong, K. Y., Olugu, E. U., & Musa, S. N. (2017). A predictive integrated genetic-based model for supplier evaluation and selection. International Journal of Fuzzy Systems, 19(4), 1041–1057. doi:10.1007/s40815-017-0324-z
- Ghazinoory, S., Esmail Zadeh, A., & Kheirkhah, A. S. (2010). Application of fuzzy calculations for improving portfolio matrices. Information Sciences, 180(9), 1582–1590. doi:10.1016/j.ins.2010.01.012
- Ghorabaee, M. K., Amiri, M., Zavadskas, E. K., & Antucheviciene, J. (2017). Supplier evaluation and selection in fuzzy environments: A review of MADM approaches. Economic Research-Ekonomska Istraživanja, 30(1), 1073–1118. doi:10.1080/1331677X.2017.1314828
- Giannoccaro, I., Pontrandolfo, P., & Scozzi, B. (2003). A fuzzy echelon approach for inventory management in supply chains. European Journal of Operational Research, 149(1), 185–196. doi:10.1016/S0377-2217(02)00441-1
- Griffis, S. E., Bell, J. E., & Closs, D. J. (2012). Metaheuristics in logistics and supply chain management. Journal of Business Logistics, 33(2), 90–106. doi:10.1111/j.0000-0000.2012.01042.x
- Groznik, A., & Trkman, P. (2015). Current issues and challenges of supply chain management. Economic Research-Ekonomska, 25(4), 1101–1112. Istrazivanja, doi:10.1080/1331677X.2012.11517551
- Gumus, A. T., & Guneri, A. F. (2009). A multi-echelon inventory management framework for stochastic and fuzzy supply chains. Expert Systems with Applications, 36(3), 5565–5575. doi:10.1016/j.eswa.2008.06.082
- Guneri, A. F., Ertay, T., & Yucel, A. (2011). An approach based on ANFIS input selection and modeling for supplier selection problem. Expert Systems with Applications, 38(12), 14907–14917. doi:10.1016/j.eswa.2011.05.056
- Gupta, A., & Maranas, C. D. (2003). Managing demand uncertainty in supply chain planning. Computers & Chemical Engineering, 27, 1219–1227. doi:10.1016/S0098-1354(03)00048-6
- Harris, F. W. (1913). How many parts to make at once. Factory, the Magazine of Management, 10(2), 135–136.
- Ho, W., Zheng, T., Yildiz, H., & Talluri, S. (2015). Supply chain risk management: A literature review. International Journal of Production Research, 53(16), 5031–5069. doi:10.1080/00207543.2015.1030467
- Holimchayachotikul, P., Leksakul, K., Montella, D. R., & Savino, M. (2010). Predictive collaborative performance system in B2B supply chain using neuro-fuzzy. In Selected topics in system science and simulation in engineering (pp. 348–353). Japan: World Scientific and Engineering Academy and Society (WSEAS).
- Jang, J. S. R. (1993). ANFIS: Adaptive-network-based fuzzy inference system. IEEE Transactions on Systems, Man, and Cybernetics, 23(3), 665–685. doi:10.1109/21.256541
- Jovanoski, B., Minovski, R. N., Lichtenegger, G., & Voessner, S. (2013). Managing strategy and production through hybrid simulation. Industrial Management & Data Systems, 113(8), 1110–1132. doi:10.1108/IMDS-09-2012-0342
- Karaboga, D., & Kaya, E. (2018). Adaptive network based fuzzy inference system (ANFIS) training approaches: A comprehensive survey. Artificial Intelligence Review, 1–31.
- Kazemi, N., Olugu, E. U., Abdul-Rashid, S. H., & Ghazilla, R. A. R. (2016). A fuzzy EOQ model with backorders and forgetting effect on fuzzy parameters: An empirical study. Computers & Industrial Engineering, 96, 140–148. doi:10.1016/j.cie.2016.03.004
- Keshavarz Ghorabaee, M., Zavadskas, E. K., Amiri, M., & Antucheviciene, J. (2016). A new method of assessment based on fuzzy ranking and aggregated weights (AFRAW) for MCDM problems under type-2 fuzzy environment. Economic Computation and Economic Cybernetics Studies and Research, 50(1), 39–68.
- Latif, H. H., Paul, S. K., & Azeem, A. (2014). Ordering policy in a supply chain with adaptive neuro-fuzzy inference system demand forecasting. International Journal of Management Science and Engineering Management, 9(2), 114–124. doi:10.1080/17509653.2013.866332
- Lee, C. K. M., Lin, D., & Pasari, R. (2014). Strategic procurement from forward contract and spot market. Industrial Management & Data Systems, 114(5), 778–796. doi:10.1108/IMDS-07-2013-0308
- Liou, J. J. H., Tamošaitienė, J., Zavadskas, E. K., & Tzeng, G.-H. (2016). New hybrid COPRAS-G MADM model for improving and selecting suppliers in green supply chain management. International Journal of Production Research, 54(1), 114–134. doi:10.1080/00207543.2015.1010747
- Maddah, B., & Noueihed, N. (2017). EOQ holds under stochastic demand, a technical note. Applied Mathematical Modelling, 45, 205–208. doi:10.1016/j.apm.2016.12.026
- Mardani, A., Zavadskas, E. K., Khalifah, Z., Zakuan, N., Jusoh, A., Nor, K. M., & Khoshnoudi, M. (2017). A review of multi-criteria decision-making applications to solve energy management problems: Two decades from 1995 to 2015. Renewable & Sustainable Energy Reviews, 71, 216–256. doi:10.1016/j.rser.2016.12.053
- Mondal, S., & Maiti, M. (2003). Multi-item fuzzy EOQ models using genetic algorithm. Computers & Industrial Engineering, 44(1), 105–117. doi:10.1016/S0360-8352(02)00187-0
- Nakandala, D., Lau, H., & Zhang, J. (2014). Optimization model for transportation planning with demand uncertainties. Industrial Management & Data Systems, 114(8), 1229–1245. doi:10.1108/IMDS-06-2014-0192
- Negnevitsky, M. (2011). Artificial intelligence: A guide to intelligent systems. Toronto, Canada: Pearson Education Canada.
- Ozkan, G., & Inal, M. (2014). Comparison of neural network application for fuzzy and ANFIS approaches for multi-criteria decision making problems. Applied Soft Computing, 24, 232–238.
- Pamučar, D., & Ćirović, G. (2018). Vehicle route selection with an adaptive neuro fuzzy inference system in uncertainty conditions. Decision Making: Applications in Management and Engineering, 1(1), 13–37.
- Paul, S. K., Azeem, A., & Ghosh, A. K. (2015). Application of adaptive neuro-fuzzy inference system and artificial neural network in inventory level forecasting. International Journal of Business Information Systems, 18(3), 268–284. doi:10.1504/IJBIS.2015.068164
- Radeerom, M., & Kulthon, M. L. (2013). A rule-based neuro-fuzzy stock trading decision support system using technical analysis. Advanced Science Letters, 19(2), 534–538. doi:10.1166/asl.2013.4785
- Rajab, S., & Sharma, V. (2017). A review on the applications of neuro-fuzzy systems in business. Artificial Intelligence Review, 49, 481–510.
- Roy, T. K., & Maiti, M. (1997). A fuzzy EOQ model with demand—dependent unit cost under limited storage capacity. European Journal of Operational Research, 99(2), 425–432. doi:10.1016/S0377-2217(96)00163-4
- Seker, A., Erol, S., & Botsali, R. (2013). A neuro-fuzzy model for a new hybrid integrated process planning and scheduling system. Expert Systems with Applications, 40(13), 5341–5351. doi:10.1016/j.eswa.2013.03.043
- Sreedevi, R., & Saranga, H. (2017). Uncertainty and supply chain risk: The moderating role of supply chain flexibility in risk mitigation. International Journal of Production Economics, 193, 332–342. doi:10.1016/j.ijpe.2017.07.024
- Sremac, S., Gladovic, P., Đelosevic, M., Matic, B., & Matic, A. (2013). Development of polymer packaging for power cable. Metalurgija, 53(4), 693–696.
- Stadtler, H., Kilger, C., & Meyr, H. (2015). Supply chain management and advanced planning: Concepts, models, software, and case studies. Germany: Springer-Verlag Berlin Heidelberg.
- Stevic, Z., Pamučar, D., Vasiljevic, M., Stojic, G., & Korica, S. (2017). Novel integrated multi-criteria model for supplier selection: Case study construction company. Symmetry, 9(11), 279–312. doi:10.3390/sym9110279
- Stojčić, M. (2018). Application of ANFIS model in road traffic and transportation: A literature review from 1993 to 2018. Operational Research in Engineering Sciences: Theory and Applications, 1(1), 40–61. doi:10.31181/oresta19012010140s
- Svalina, I., Galzina, V., Lujic, R., & Simunovic, G. (2013). An adaptive network-based fuzzy inference system (ANFIS) for the forecasting: The case of close price indices. Expert Systems with Applications, 40(15), 6055–6063. doi:10.1016/j.eswa.2013.05.029
- Tahmasebi, P., & Hezarkhani, A. (2010). Application of adaptive neuro-fuzzy inference system for grade estimation; case study, Sarcheshmeh porphyry copper deposit, Kerman, Iran. Australian Journal of Basic and Applied Sciences, 4(3), 408–420.
- Taleizadeh, A. A., Khanbaglo, M. P. S., & Cárdenas-Barrón, L. E. (2016). An EOQ inventory model with partial backordering and reparation of imperfect products. International Journal of Production Economics, 182, 418–434. doi:10.1016/j.ijpe.2016.09.013
- Tamosaitiene, J., Zavadskas, E. K., Sileikaite, I., & Turskis, Z. (2017). A novel hybrid MCDM approach for complicated supply chain management problems in construction. Procedia Engineering, 172, 1137–1145. doi:10.1016/j.proeng.2017.02.168
- Tavana, M., Fallahpour, A., Di Caprio, D., & Santos-Arteaga, F. J. (2016). A hybrid intelligent fuzzy predictive model with simulation for supplier evaluation and selection. Expert Systems with Applications, 61, 129–144. doi:10.1016/j.eswa.2016.05.027
- Teksan, Z. M., & Geunes, J. (2016). An EOQ model with price-dependent supply and demand. International Journal of Production Economics, 178, 22–33. doi:10.1016/j.ijpe.2016.04.023
- Tempelmeier, H. (2011). Inventory management in supply networks (3rd ed.). Norderstedt: University of Cologne.
- Teodorovic, D., & Vukadinovic, K. (1998). Traffic control and transport planning: A fuzzy sets and neural networks approach. Netherland: Springer. ISSN 1382-3434.
- Vahdani, B., Iranmanesh, S. H., Meysam Mousavi, S., & Abdollahzade, M. (2012). A locally linear neuro-fuzzy model for supplier selection in cosmetics industry. Applied Mathematical Modelling, 36(10), 4714–4727. doi:10.1016/j.apm.2011.12.006
- Wallin, C., Rungtusanatham, M. J., & Rabinovich, E. (2006). What is the right inventory management approach for a orderd item. International Journal of Operations & Production Management, 26(1), 50–68. doi:10.1108/01443570610637012
- Wu, Z., Kazaz, B., Webster, S., & Yang, K. K. (2012). Ordering, pricing, and lead-time quotation under lead-time and demand uncertainty. Production and Operations Management, 21(3), 576–589. doi:10.1111/j.1937-5956.2011.01289.x
- Xiao, Y., Liu, J. J., Hu, J., Wang, Y., Lai, K. K., & Wang, S. (2014). A neuro-fuzzy combination model based on singular spectrum analysis for air transport demand forecasting. Journal of Air Transport Management, 39, 1–11. doi:10.1016/j.jairtraman.2014.03.004
- Yazdani-Chamzini, A., Yakhchali, S. H., Volungevičienė, D., & Zavadskas, E. K. (2012). Forecasting gold price changes by using adaptive network fuzzy inference system. Journal of Business Economics and Management, 13(5), 994–1100. doi:10.3846/16111699.2012.683808
- Yazdani-Chamzini, A., Zavadskas, E. K., Antucheviciene, Y., & Bausys, R. (2017). A model for shovel capital cost estimation, using a hybrid model of multivariate regression and neural networks. Symmetry, 9(12), 298–311. doi:10.3390/sym9120298
- Yazdani, M., Zarate, P., Coulibaly, A., & Zavadskas, E. K. (2017). A group decision making support system in logistics and supply chain management. Expert Systems with Applications, 88, 376–392. doi:10.1016/j.eswa.2017.07.014
- Zavadskas, E. K., Antucheviciene, J., Vilutiene, T., & Adeli, H. (2017). Sustainable decision-making in civil engineering, construction and building technology. Sustainability, 10(2), 14. doi:10.3390/su10010014
- Zavadskas, E. K., Govindan, K., Antucheviciene, J., & Turskis, Z. (2016). Hybrid multiple criteria decision-making methods: A review of applications for sustainability issues. Economic Research-Ekonomska Istraživanja, 29(1), 857–887. doi:10.1080/1331677X.2016.1237302