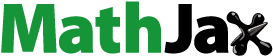
Abstract
In today’s world, with the advancement of technology, several emerging technologies are coming. Faced with massive emerging technologies which are the component of the technology pool, how to identify the commercial potential of emerging technologies in theory and practice is an important problem. The scientific approach to the selection of these emerging technologies is one of the main objectives of the research. In this paper, we extend Muirhead mean (MM) operator and dual MM (DMM) operator to process the Pythagorean fuzzy numbers (PFNs) and then to solve the multiple attribute decision making (MADM) problems. Firstly, we develop some Pythagorean fuzzy Muirhead mean operators by extending MM and DMM operators to Pythagorean fuzzy information. Then, we prove some properties and discuss some special cases with respect to the parameter vector. Moreover, we present some new methods to deal with MADM problems with the PFNs based on the proposed MM and DMM operators. Finally, we verify the validity and reliability of our methods by using an application example for potential evaluation of emerging technology commercialization, and analyze the advantages of our methods by comparing with other existing methods.
1. Introduction
Atanassov (1986, 2000) introduced the concept of intuitionistic fuzzy set (IFS), which is a generalization of the concept of fuzzy set (Zadeh, Citation1965). Each element in the IFS is expressed by an ordered pair, and each ordered pair is characterized by a membership degree and a non-membership degree. The sum of the membership degree and the non-membership degree of each ordered pair is less than or equal to 1. More recently, a Pythagorean fuzzy set (PFS) (Yager, Citation2013, Citation2014) has emerged as an effective tool for depicting uncertainty of the MADM problems. The PFS is also characterized by the membership degree and the non-membership degree, whose sum of squares is less than or equal to 1; the PFS is more general than the IFS. In some cases, the PFS can solve the problems that the IFS cannot; for example, if a DM gives the membership degree and the non-membership degree as 0.8 and 0.6, respectively, then it is only valid for the PFS. In other words, all the intuitionistic fuzzy degrees are a part of the Pythagorean fuzzy degrees, which indicates that the PFS is more powerful to handle the uncertain problems. Zhang and Xu (Citation2014) provided the detailed mathematical expression for PFS and introduced the concept of Pythagorean fuzzy number (PFN). Meanwhile, they also developed a Pythagorean fuzzy TOPSIS (Technique for Order Preference by Similarity to Ideal Solution) for handling the MCDM problem within PFNs. Peng and Yang (Citation2015) proposed the division and subtraction operations for PFNs, and also developed a Pythagorean fuzzy superiority and inferiority ranking method to solve multicriteria group decision making problem with PFNs. Afterwards, Beliakov and James (Citation2014) focused on how the notion of ‘averaging’ should be treated in the case of PFNs and how to ensure that the averaging aggregation functions produce outputs consistent with the case of ordinary fuzzy numbers. Reformat and Yager (Citation2014) applied the PFNs in handling the collaborative-based recommender system. Gou, Xu, and Ren (Citation2016) investigated the Properties of Continuous PFN. Ren, Xu, and Gou (Citation2016) proposed the Pythagorean fuzzy TODIM approach to multi-criteria decision making. Garg (Citation2016a) proposed the new generalized Pythagorean fuzzy information aggregation by using Einstein Operations. Zeng, Chen, and Li (Citation2016) developed a hybrid method for Pythagorean fuzzy multiple-criteria decision making. Garg (Citation2016b) studied a novel accuracy function under interval-valued PFSs for solving multicriteria decision making problems. Wei (Citation2017a) utilized arithmetic and geometric operations (Wang, Wei, & Lu, Citation2018a; Wei, Gao, & Wei, Citation2018a; Wei, Lu, Tang, & Wei, Citation2018b; Wu, Wang, Wei, & Wei, Citation2018) to develop some Pythagorean fuzzy interaction aggregation operators: Pythagorean fuzzy interaction weighted average (PFIWA) operator, Pythagorean fuzzy interaction weighted geometric (PFIWG) operator, Pythagorean fuzzy interaction ordered weighted average (PFIOWA) operator, Pythagorean fuzzy interaction ordered weighted geometric (PFIOWG) operator, Pythagorean fuzzy interaction hybrid average (PFIHA) operator and Pythagorean fuzzy interaction hybrid geometric (PFIHG) operator. Wei and Lu (Citation2018a) extended Maclaurin symmetric mean (Maclaurin, Citation1729) to Pythagorean fuzzy environment to propose the Pythagorean fuzzy Maclaurin symmetric mean (PFMSM) operator and Pythagorean fuzzy weighted Maclaurin symmetric mean (PFWMSM) operator. Wei and Lu (Citation2018b) utilized power aggregation operators (Yager, Citation2001) to develop some Pythagorean fuzzy power aggregation operators: Pythagorean fuzzy power average (PFPA) operator, Pythagorean fuzzy power geometric (PFPG) operator, Pythagorean fuzzy power weighted average (PFPWA) operator, Pythagorean fuzzy power weighted geometric (PFPWG) operator, Pythagorean fuzzy power ordered weighted average (PFPOWA) operator, Pythagorean fuzzy power ordered weighted geometric (PFPOWG) operator, Pythagorean fuzzy power hybrid average (PFPHA) operator and Pythagorean fuzzy power hybrid geometric (PFPHG) operator. Lu, Wei, Alsaadi, Hayat, and Alsaedi (Citation2017) proposed some hesitant pythagorean fuzzy hamacher aggregation operators and their application to multiple attribute decision making. Wei and Lu (Citation2017a) defined some dual hesitant Pythagorean fuzzy Hamacher aggregation operators in multiple attribute decision making. Wei and Lu (Citation2017b) developed some Pythagorean hesitant fuzzy Hamacher aggregation operators in multiple attribute decision making. Wu and Wei (Citation2017) gave some Pythagorean fuzzy Hamacher aggregation operators and their application to multiple attribute decision making. Wei, Lu, Alsaadi, Hayat, and Alsaedi (Citation2017a) proposed some Pythagorean 2-tuple linguistic aggregation operators: Pythagorean 2-tuple linguistic weighted average (P2TLWA) operator, Pythagorean 2-tuple linguistic weighted geometric (P2TLWG) operator, Pythagorean 2-tuple linguistic ordered weighted average (P2TLOWA) operator, Pythagorean 2-tuple linguistic ordered weighted geometric (P2TLOWG) operator, Pythagorean 2-tuple linguistic hybrid average (P2TLHA) operator and Pythagorean 2-tuple linguistic hybrid geometric (P2TLHG) operator. Lu and Wei (Citation2017) proposed some Pythagorean uncertain linguistic aggregation operators for multiple attribute decision making.
In some real decision making, there exist the interrelationships among the attributes in MADM problems. Bonferroni mean (BM) operators (Bonferroni, Citation1950; Deng, Wei, Gao, & Wang, Citation2018; Liu, Chen, & Liu, Citation2017; Wang, Wei, & Wei, Citation2018b; Wei, Citation2017b; Zhu, Xu, & Xia, Citation2012) and the Heronian mean (HM) (Beliakov, Pradera, & Calvo, Citation2007; Chu & Liu, Citation2015; Liu, Liu, & Zhang, Citation2014; Liu, Zhu, Liu, & Hao, Citation2013; Yu, Citation2013; Yu, Zhou, Chen, & Wang, Citation2015) operators provided a tool to consider the interrelationships of aggregated arguments; however, they can only consider the interrelationships between two attributes and cannot process the interrelationships among three or more than three attributes. Muirhead mean (MM) (Muirhead, Citation1902) is a well-known aggregation operator which can consider interrelationships among any number of arguments assigned by a variable vector, and some existing operators, such as arithmetic and geometric operators (not considering the interrelationships), both BM operator and Maclaurin symmetric mean (Maclaurin, Citation1729) are the special cases of MM operator. Therefore, the MM can offer a flexible and robust mechanism to process the information fusion problem and make it more adequate to solve MADM problems. However, the original MM can only deal with the numeric arguments, in order to make the MM operator to process the linguistic information, Qin and Liu (Citation2016) extended the MM operator to process the 2-tuple linguistic information, and proposed some 2-tuple linguistic MM operators and applied the proposed operators to solve the MADM problems.
Because PFNs can easily describe the fuzzy information, and the MM operator and dual MM (DMM) operator can capture interrelationships among any number of arguments assigned by a variable vector, it is necessary to extend the MM and DMM operator to deal with the PFNs. The purpose of this paper is to propose some Pythagorean fuzzy MM operators by extending MM and DMM operators to Pythagorean fuzzy information, then to study some properties of these operators, and applied them to solve the MADM problems in which the attributes take the form of PFNs.
In order to achieve this purpose, the rest of this paper is set out as follows. Section 2 reviews some basic concepts and theory of PFSs. In Section 3, we propose the some Pythagorean fuzzy MM operators, and study some properties of these operators. In Section 4, we develop two MADM methods for PFNs based on the PFWMM operator and PFWDMM operator. In Section 5, an illustrative example for potential evaluation of emerging technology commercialization is given to verify the validity of the proposed methods and to show their advantages. In Section 6, we give some conclusions of this study.
2. Preliminaries
In this section, we review some fundamental concept of Pythagorean fuzzy set and MM, which will be used in the next section.
2.1. Pythagorean fuzzy set
The basic concepts of PFSs (Yager, Citation2013, Citation2014) are briefly reviewed in this section. Afterwards, novel score and accuracy functions for PFNs are proposed. Furthermore, a new comparison method for PFNs is developed.
Definition 1
(Yager, Citation2013, Citation2014). Let be a fix set. A PFS is an object having the form
(1)
(1)
where the function
defines the degree of membership and the function
defin1es the degree of non-membership of the element
to
respectively, and, for every
it holds that
(2)
(2)
Definition 2
(Wei, Citation2017a). Let be a Pythagorean fuzzy number, a score function
of a Pythagorean fuzzy number can be represented as follows:
(3)
(3)
Definition 3
(Ren et al., Citation2016). Let be a Pythagorean fuzzy number, an accuracy function
of a Pythagorean fuzzy number can be represented as follows:
(4)
(4)
to evaluate the degree of accuracy of the Pythagorean fuzzy number
where
The larger the value of
is, the more the degree of accuracy of the Pythagorean fuzzy number
Based on the score function S and the accuracy function in the following, we shall give an order relation between two Pythagorean fuzzy numbers, which is defined as follows:
Definition 4
(Wei, Citation2017a). Let and
be two Pythagorean fuzzy numbers,
and
be the scores of
and
respectively, and let
and
be the accuracy degrees of
and
respectively, then if
then
is smaller than
denoted by
if
then
if
then
and
represent the same information, denoted by
(2) if
is smaller than
denoted by
Definition 5
(Reformat & Yager, Citation2014). Let
and
be three Pythagorean fuzzy numbers, and some basic operations on them are defined as follows:
2.2. Muirhead mean (MM)
The MM was first introduced by Muirhead (Citation1902), the advantage of the MM operator is that it can capture the overall interrelationships among the multiple input arguments and it is a generalization of some existing aggregation operators. It was defined as follows:
Definition 6
(Muirhead, Citation1902). Let be a set of crisp numbers and
then the Muirhead mean (MM) operator is defined as
(5)
(5)
Where
is any permutation of
and
is the set of all permutation of
By assigning some special vectors to we can obtain some special cases of the MM operator:
If
the MM is reduced to
(6)
(6)
Which is the arithmetic averaging operator.
If
the MM is reduced to
(7)
(7)
Which is the BM operator (Bonferroni, Citation1950).
If
the MM is reduced to
(8)
(8)
which is the Maclaurin symmetric mean (MSM) operator (Maclaurin, Citation1729).
If
the MM is reduced to
(9)
(9)
which is the arithmetic averaging operator.
3. Pythagorean fuzzy Muirhead mean operators
In this section, we shall develop some Pythagorean fuzzy Muirhead mean operators based on the operations of PFNs.
3.1. PFMM operator
The MM operator has usually been applied to a situation where the aggregation assessments exhibit interaction relationship. Next, we extend MM operator to PFS. From definition 5 and 6, we can obtain:
Definition 7.
Let be a set of PFN and
be a vector of parameters, then the Pythagorean Fuzzy Muirhead mean (PFMM) operator is defined as
(10)
(10)
Where
is any permutation of
and
is the collection of all permutations of
Based on the operations of the PFN described, we can drive the Theorem 1.
Theorem 1.
Let be a collection of PFNs, then their aggregated value by using the PFMM operator is also a PFN, and
(11)
(11)
Proof
:
(12)
(12)
(13)
(13)
Thereafter,
(14)
(14)
(15)
(15)
Therefore,
(16)
(16)
And then, we can know:
(17)
(17)
(18)
(18)
We can obtain from the definition of PFS, so
(19)
(19)
We complete the proof.
Example 1.
Let be three PFNs, and
then we have
In the following, we give some properties of PFMM operator.
Property 1. (Idempotency) let then
(20)
(20)
Proof
:
(21)
(21)
Property 2. (Monotonicity) let and
be two sets of PFNs, If
and
then
(22)
(22)
Proof
:
(23)
(23)
(24)
(24)
Therefore,
(25)
(25)
Similarly, we also can obtain
(26)
(26)
then, the proof is completed. Then
If and
then
If and
then
If and
then
If and
then
Property 3. (Boundedness) Let be a set of PFNs. If
and
According the process of property of Monotonicity and Idempotency, it is easy to got that
(27)
(27)
3.2. PFWMM operator
In Section 3.1, it can be seen that the PFMM operator doesn't consider the importance of the aggregated arguments. However, in many real practical situations, especially in multiple attribute decision making, the weights of attributes plays an important role in the process of aggregation. To overcome the limitation of PFMM, we shall propose the Pythagorean fuzzy weighted MM (PFWMM) operator as follows.
Definition 8.
Let be a set of PFNs with weights vector being
and
then the Pythagorean fuzzy weighted Muirhead mean (PFWMM) operator is defined as
(28)
(28)
Based on the operations of the PFN described, we can drive the Theorem 2.
Theorem 2.
Let be a collection of PFNs, then their aggregated value by using the PFWMM operator is also a PFN, and
(29)
(29)
proof
:
(30)
(30)
(31)
(31)
Thereafter,
(32)
(32)
(33)
(33)
Thus,
(34)
(34)
Therefore,
(35)
(35)
and we can get followed easily,
(36)
(36)
(37)
(37)
Therefore,
(38)
(38)
(39)
(39)
We complete the proof.
Example 2.
Let be three PFNs, and
then we have
The PFWMM operator has the property of boundedness and monotonicity, but it does not satisfy the property of idempotency. In the following, we omitted the process of proof, because it is similar with the PFMM monotonicity property.
Property 4. (Monotonicity) let and
be two sets of PFNs, If
and
then
(40)
(40)
Property 5. (Boundedness) Let be a set of PFNs with weights vector being
If
and
because of property 4, then
(41)
(41)
3.3. PFDMM operator
Qin and Liu (Citation2016) proposed the dual Muirhead mean (DMM) based on MM operator.
Definition 9.
Let be a set of non-negative real numbers, and
be a vector of parameters. If
(42)
(42)
Then we called the dual Muirhead mean (DMM) operator, where
is any a permutation of
and
is the set of all permutation of
In the following, we proposed the Pythagorean fuzzy dual MM (PFDMM) operator for PFNs.
Definition 10.
Let be a collection of PFNs and there exists parameter vector
then
(43)
(43)
Based on the operations of the PFN described, we can drive the Theorem 3.
Theorem 3.
Let be a collection of PFNs, then their aggregated value by using the PFDMM operator is also a PFN, and
(44)
(44)
Proof
:
(45)
(45)
(46)
(46)
Therefore,
(47)
(47)
(48)
(48)
Then, we can get
(49)
(49)
From the aggregation result above, we prove the result of PFDMM aggregation is also a PFN in the following, then
(50)
(50)
(51)
(51)
And, we can prove
(52)
(52)
So, we proved that the aggregation result of PFDMM is also a PFN.
Example 3.
Let be three PFNs, and
then we have
Property 6. (Idempotency) if all are equal, i.e.,
then
(53)
(53)
Property 7. (Monotonicity) let and
be two sets of PFNs, If
and
then
(54)
(54)
Property 8. (Boundedness). Let be a set of PFNs. If
and
because of property 7 and property 8, then
(55)
(55)
3.4. PFWDMM operator
In Section 3.3, it can be seen that the PFDMM operator doesn't consider the importance of the aggregated arguments. However, in many real practical situations, especially in multiple attribute decision making, the weights of attributes plays an important role in the process of aggregation. To overcome the limitation of PFDMM operator, we shall propose the Pythagorean fuzzy weighted DMM (PFWDMM) operator as follows.
Definition 11.
Let be a collection of PFNs with weights vector being
and there exists parameter vector
then
(56)
(56)
Based on the operations of the PFN described, we can drive the Theorem 4.
Theorem 4.
Let be a collection of PFNs, then their aggregated value by using the PFWDMM operator is also a PFN, and
(57)
(57)
Proof
:
(58)
(58)
(59)
(59)
Thereafter
(60)
(60)
(61)
(61)
Therefore,
(62)
(62)
Then, we can get
(63)
(63)
(64)
(64)
Because therefore
(65)
(65)
So, the aggregation result of PFWDMM is also PFN.
Example 4.
Let be three PFNs, and
then we have
PFWDMM is also satisfying property boundedness and monotonicity, but it is not satisfying the property of idempotency.
Property 10. (Monotonicity) let and
be two sets of PFNs with weights vector being
If
and
then
(66)
(66)
Property 11. (Boundedness) Let be a set of PFNs with weights vector being
If
and
because of property 10, then
(67)
(67)
5. Models for MADM with PFNs
Based the PFWMM and PFWDMM operators, in this section, we shall propose the model for MADM with PFNs. Let be a discrete set of alternatives, and
be the set of attributes,
is the weighting vector of the attribute
where
Suppose that
is the Pythagorean fuzzy decision matrix, where
indicates the degree that the alternative
satisfies the attribute
given by the decision maker,
indicates the degree that the alternative
doesn’t satisfy the attribute
given by the decision maker,
In the following, we apply the PFWMM (PFWDMM) operator to the MADM problems with PFNs.
Step 1. We utilize the PFNs given in matrix
and the PFWMM operator
Or
(69)
(69)
to derive the
of the alternative
Step 2. Calculate the scores
of the overall PFNs
to rank all the alternatives
and then to select the best one(s). If there is no difference between two scores
and
then we need to calculate the accuracy degrees
and
of the overall PFNs
and
respectively, and then rank the alternatives
and
in accordance with the accuracy degrees
and
Step 3. Rank all the alternatives
and select the best one(s) in accordance with
Step 4. End.
6. Numerical example and comparative analysis
6.1. Numerical example
For the time being, emerging technologies have mushroomed up gradually with the rapid development of science and technology, and emerging technologies have brought obvious impacts on states, industries and societies. There are no countries which can oversee the development of emerging technologies, it can be said that the competition between countries and states is the one of emerging technologies, especially the quality, quantity and speed of commercialization and industrialization of emerging technologies. It is not only a difficult task for countries and enterprises to identify, further evaluate and select emerging technologies, finally commercialize and industrialize emerging technologies; but also a weak issue for researchers to study theoretically. Thus, in this section we shall present a numerical example to show potential evaluation of emerging technology commercialization with Pythagorean fuzzy information in order to illustrate the method proposed in this paper. There is a panel with five possible emerging technology enterprises to select. The experts selects four attributes to evaluate the five possible emerging technology enterprises: ①G1 is the technical advancement; ②G2 is the potential market and market risk; ③G3 is the industrialization infrastructure, human resources and financial conditions; ④G4 is the employment creation and the development of science and technology. The five possible emerging technology enterprises
are to be evaluated using the PFNs according to four attributes (whose weighting vector
as shown in .
Table 1. PFN decision matrix.
In the following, in order to show potential evaluation of emerging technology commercialization of five possible emerging technology enterprises, we utilize the PFMM, PFWMM, PFDMM and PFWDMM operators to solve MADM problem with PFNs, which concludes the following calculating steps:
Step 1. According to , aggregate all PFNs
by using the PFMM, PFWMM, PFDMM and PFWDMM operators to derive the overall PFNs
of the emerging technology enterprises
The aggregating results are shown in .
Table 2. The aggregating Result of PFMM, PFWMM, PFDMM and PFWDMM operators.
Step 2. According to the aggregating results shown in and the score functions of the emerging technology enterprises are shown in .
Table 3. The rank and score of emerging technology enterprises by using PFMM, PFWMM, PFDMM and PFWDMM operators.
According the result of emerging technology enterprises order, we can know that the best choice is emerging technology enterprise O4, we get same result by different aggregation, that proved the effectiveness of the result.
6.2. Influence of the parameter on the final result
The aggregation method of extend PFS with MM has two advantages, one is that it can reduce the bad effects of the unduly high and low assessments on the final result, the other is that it can capture the interrelationship between PFNs. These aggregation operators have a parameter vector, which make extended operator more flexible, so the different vector leads to different aggregation results, different scores and ranking results. In order to illustrate the influence of the parameter vector R on the ranking result, we discuss the influence with several parameter vectors, the result you can find in and .
Table 4. Ranking results by utilizing different parameter vector R in the PFWMM operator.
Table 5. Ranking results by utilizing different parameter vector R in the PFWDMM operator.
We can see that the different parameters lead to a different result and different ranking order. More attributes we consider, the bigger the scores, the bigger the attribute value and the more lower the scores. Therefore, the parameter vector can be considered as decision maker’s risk preference.
6.3. Comparative analysis
The prominent characteristic of the PFMM, PFWMM, PFDMM and PFWDMM operators is that they can consider the interrelationship among the PFNs. We investigate some comparative analyses to demonstrate the advantages of the proposed operators. presents further details.
Table 6. The Comparison of the different aggregation operators under PFNs.
Table 7. Ordering of the emerging technology enterprises.
shows that the aggregation operators introduced in (Yager, Citation2014; Garg, Citation2016a; Zeng et al., Citation2016; Wei & Lu, Citation2017a; Wu & Wei, Citation2017; Wei, Citation2017c) cannot consider the interrelationship between the PFNs. Although PFCIA, PFCIG, PFPWA and PFPWG can capture the interrelationship between the PFNs, they only change the weight vector of the aggregation operators. In addition, the correlations of the aggregated arguments are measured subjectively by the decision makers. PFMSM, PFWMSM, PFIWA, PFIWG, PFIOWA, PFIOWG, PFIHA, PFIHG, GPFWBM, GPFWBGM, GPFWHM, PFGWHM, PFMM, PFDMM, PFWMM, PFWDMM operators focus on the aggregated PFNs. In addition, the GPFWBM, GPFWBGM, PFMM, PFDMM, PFWMM, PFWDMM operators have a parameter vector, thereby enabling the aggregation process to be substantially flexible.
At the same time, we compare our proposed method with other existing methods including the Pythagorean fuzzy weighted averaging (PFWA) operator (Yager, Citation2014), Pythagorean fuzzy weighted geometric (PFWG) operator (Yager, Citation2014), symmetric Pythagorean fuzzy weighted averaging (SPFWA) operator (Ma & Xu, Citation2016) and symmetric Pythagorean fuzzy weighted geometric (SPFWG) operator (Ma & Xu, Citation2016).
From the , we can get the same optimal emerging technology enterprises and four methods’ ranking results are slightly different from the proposed approaches. However, the existing aggregation operators, such as PFWA operator, PFWG operator, SPFWA operator and SPFWG operator, do not consider the information about the relationship between arguments being aggregated, and thus cannot eliminate the influence of unfair arguments on decision results. Our proposed operators, such as PFMM operator, PFDMM operator, PFWMM operator and PFWDMM operator consider the information about the relationship among arguments being aggregated.
7. Conclusion
Aggregation operators have become a hot issue and an important tool in the decision making fields in recent years. However, they still have some limitations in practical applications. For example, some aggregation operators suppose the attributes are independent of each other. However, the MM operator and dual MM operator have a prominent characteristic that it can consider the interaction relationships among any number of attributes by a parameter vector Motivated by the studies about MM operator and dual MM operator, in this paper, we proposed some new MM and DMM aggregation operators to deal with MADM problems under a Pythagorean fuzzy environment, included the Pythagorean fuzzy MM (PFMM) operator, Pythagorean fuzzy weighted MM (PFWMM) operator, Pythagorean fuzzy dual MM (PFWMM) operator and the Pythagorean fuzzy weighted dual MM (PFWDMM) operator. Then, the desirable properties were proved. Moreover, these proposed operators are utilized to solve the MADM problems with PFNs. Finally, we used an illustrative example for potential evaluation of emerging technology commercialization to show the feasibility and validity of the proposed operators by comparing with the other existing methods. In the future, we shall extend the proposed operators to dynamic and complex decision making (Chen, Citation2015; De & Sana, Citation2014; Gao, Citation2018; Gao, Wei, & Huang, Citation2018; Huang & Wei, Citation2018; Tang & Wei, Citation2018; Wang, Wei, & Lu, Citation2018c; Wei, Citation2017c, Citation2018a, Citation2018b; Wei, Gao, Wang, & Huang, Citation2018c; Yue & Jia, Citation2013), risk analysis (Wei, Liu, Lai, & Hu, Citation2017b; Wei, Yu, Liu, & Cao, Citation2018d) and many other fields under uncertain environment (Chen, Citation2017; Mardani et al., Citation2015, Citation2018; Peng & Selvachandran, Citation2017; Rostamzadeh, Esmaeili, Nia, Saparauskas, & Ghorabaee, Citation2017; Wang, Wei, & Gao, Citation2018d; Wei, Citation2018c; Wei & Wei, Citation2018; Zeng, Mu, & Balezentis, Citation2018).
Disclosure statement
No potential conflict of interest was reported by the authors.
Additional information
Funding
References
- Atanassov, K. (1986). Intuitionistic fuzzy sets. Fuzzy Sets and Systems, 20(1), 87–96. doi:10.1016/S0165-0114(86)80034-3
- Atanassov, K. (2000). Two theorems for intuitionistic fuzzy sets. Fuzzy Sets and Systems, 110(2), 267–269. doi:10.1016/S0165-0114(99)00112-8
- Beliakov, G., & James, S. (2014). Averaging aggregation functions for preferences expressed as Pythagorean membership grades and fuzzy orthopairs. FUZZ-IEEE, pp. 298–305.
- Beliakov, G., Pradera, A., & Calvo, T. (2007). Aggregation functions: A guide for practitioners. Berlin. Springer.
- Bonferroni, C. (1950). Sulle medie multiple di potenze. Bolletino Matematica Italiana, 5, 267–270.
- Chen, T. Y. (2017). Multiple criteria decision analysis using prioritised interval type-2 fuzzy aggregation operators and its application to site selection. Technological and Economic Development of Economy, 23(1), 1–21. doi:10.3846/20294913.2016.1209249
- Chen, T.-Y. (2015). The inclusion-based TOPSIS method with interval-valued intuitionistic fuzzy sets for multiple criteria group decision making. Applied Soft Computing, 26, 57–73. doi:10.1016/j.asoc.2014.09.015
- Chu, Y. C., & Liu, P. D. (2015). Some two-dimensional uncertain linguistic Heronian mean operators and their application in multiple-attribute decision making. Neural Computing and Applications, 26(6), 1461–1480. doi:10.1007/s00521-014-1813-8
- De, S. K., & Sana, S. S. (2014). A multi-periods production-inventory model with capacity constraints for multi-manufacturers-A global optimality in intuitionistic fuzzy environment. Applied Mathematics and Computation, 242, 825–841. doi:10.1016/j.amc.2014.06.075
- Deng, X. M., Wei, G. W., Gao, H., & Wang, J. (2018). Models for safety assessment of construction project with some 2-tuple linguistic Pythagorean fuzzy Bonferroni mean operators. IEEE Access, 6, 52105–52137. doi:10.1109/ACCESS.2018.2869414
- Gao, H. (2018). Pythagorean fuzzy Hamacher prioritized aggregation operators in multiple attribute decision making. Journal of Intelligent & Fuzzy Systems, 35(2), 2229–2245. doi:10.3233/JIFS-172262
- Gao, H., Wei, G. W., & Huang, Y. H. (2018). Dual hesitant bipolar fuzzy Hamacher prioritized aggregation operators in multiple attribute decision making. IEEE Access, 6(1), 11508–11522. doi:10.1109/ACCESS.2017.2784963
- Garg, H. (2016a). A new generalized Pythagorean fuzzy information aggregation using Einstein operations and its application to decision making. International Journal of Intelligent Systems, 31(9), 886–920. doi:10.1002/int.21809
- Garg, H. (2016b). A novel accuracy function under interval-valued Pythagorean fuzzy environment for solving multicriteria decision making problem. Journal of Intelligent & Fuzzy Systems, 31(1), 529–540. doi:10.3233/IFS-162165
- Gou, X., Xu, Z., & Ren, P. (2016). The properties of continuous Pythagorean fuzzy information. International Journal of Intelligent Systems, 31(5), 401–424. doi:10.1002/int.21788
- Huang, Y. H., & Wei, G. W. (2018). TODIM method for Pythagorean 2-tuple linguistic multiple attribute decision making. Journal of Intelligent & Fuzzy Systems, 35(1), 901–915. doi:10.3233/JIFS-171636
- Liu, P. D., Liu, Z. M., & Zhang, X. (2014). Some intuitionistic uncertain linguistic Heronian mean operators and their application to group decision making. Applied Mathematics and Computation, 230, 570–586. doi:10.1016/j.amc.2013.12.133
- Liu, P.-D., Chen, S.-M., & Liu, J. (2017). Multiple attribute group decision making based on intuitionistic fuzzy interaction partitioned Bonferroni mean operators. Information Sciences., 411, 98–121. doi:10.1016/j.ins.2017.05.016
- Liu, X. D., Zhu, J. J., Liu, G. D., & Hao, J. J. (2013). A multiple attribute decision making method based on uncertain linguistic Heronian mean. Mathematical Problems in Engineering, 2013, 1–11. doi:10.1155/2013/597671
- Lu, M., & Wei, G. W. (2017). Pythagorean uncertain linguistic aggregation operators for multiple attribute decision making. International Journal of Knowledge-Based and Intelligent Engineering Systems, 21(3), 165–179. doi:10.3233/KES-170361
- Lu, M., Wei, G. W., Alsaadi, F. E., Hayat, T., & Alsaedi, A. (2017). Hesitant Pythagorean fuzzy Hamacher aggregation operators and their application to multiple attribute decision making. Journal of Intelligent & Fuzzy Systems, 33(2), 1105–1117. doi:10.3233/JIFS-16554
- Ma, Z., & Xu, Z. (2016). Symmetric Pythagorean fuzzy weighted geometric/averaging operators and their application in multicriteria decision-making problems. International Journal of Intelligent Systems, 31(12), 1198–1219. no. doi:10.1002/int.21823
- Maclaurin, C. (1729). A second letter to Martin Folkes, Esq.; concerning the roots of equations, with demonstration of other rules of algebra. Philosophical Transactions of the Royal Society of London Series A, 36, 59–96.
- Mardani, A., Jusoh, A., Nor, K. M., Khalifah, Z., Zakwan, N., & Valipour, A. (2015). Multiple criteria decision-making techniques and their applications-a review of the literature from 2000 to 2014. Economic Research-Ekonomska Istraživanja, 28(1), 516–571. doi:10.1080/1331677X.2015.1075139
- Mardani, A., Nilashi, M., Zavadskas, E. K., Awang, S. R., Zare, H., & Jamal, N. M. (2018). Decision-making methods based on fuzzy aggregation operators: Three decades review from 1986 to 2017. International Journal of Information Technology & Decision Making, 17, 391–466. doi:10.1142/S021962201830001X
- Muirhead, R. F. (1902). Some methods applicable to identities and inequalities of symmetric algebraic functions of n letters. Proceedings of the Edinburgh Mathematical Society, 21(3), 144–162. doi:10.1017/S001309150003460X
- Peng, X. D., & Yang, Y. (2016). Pythagorean fuzzy choquet integral based MABAC method for multiple attribute group decision making. International Journal of Intelligent Systems, 31(10), 989–1020. doi:10.1002/int.21814
- Peng, X., & Selvachandran, G. (2017). Pythagorean fuzzy set: State of the art and future directions. Artificial Intelligence Review, 1, 1–55.
- Peng, X., & Yang, Y. (2015). Some results for Pythagorean fuzzy sets. International Journal of Intelligent Systems, 30(11), 1133–1160. doi:10.1002/int.21738
- Qin, J. Q., & Liu, X. W. (2016). 2-tuple linguistic Muirhead mean operators for multiple attribute group decision making and its application to supplier selection. Kybernetes, 45(1), 2–29. doi:10.1108/K-11-2014-0271
- Reformat, M., & Yager, R. R. (2014). Suggesting recommendations using Pythagorean fuzzy sets illustrated using Netflix movie data. IPMU, 1, 546–556.
- Ren, P., Xu, Z., & Gou, X. (2016). Pythagorean fuzzy TODIM approach to multi-criteria decision making. Applied Soft Computing, 42, 246–259. doi:10.1016/j.asoc.2015.12.020
- Rostamzadeh, R., Esmaeili, A., Nia, A. S., Saparauskas, J., & Ghorabaee, M. K. (2017). A fuzzy Aras method for supply chain management performance measurement in SMEs under uncertainty. Transformations in Business & Economics, 16(2A), 319–348.
- Tang, X. Y., & Wei, G. W. (2018). Models for green supplier selection in green supply chain management with Pythagorean 2-tuple linguistic information. IEEE Access, 6, 18042–18060. doi:10.1109/ACCESS.2018.2817551
- Wang, J., Wei, G. W., & Wei, Y. (2018b). Models for green supplier selection with some 2-tuple linguistic neutrosophic number Bonferroni mean operators. Symmetry, 10(5), 131. doi:10.3390/sym10050131
- Wang, J., Wei, G., & Gao, H. (2018d). Approaches to multiple attribute decision making with interval-valued 2-tuple linguistic pythagorean fuzzy information. Mathematics, 6(10), 201. doi:10.3390/math6100201
- Wang, J., Wei, G., & Lu, M. (2018a). TODIM method for multiple attribute group decision making under 2-tuple linguistic neutrosophic environment. Symmetry, 10(10), 486. doi:10.3390/sym10100486
- Wang, J., Wei, G., & Lu, M. (2018c). An extended VIKOR method for multiple criteria group decision making with triangular fuzzy neutrosophic numbers. Symmetry, 10(10), 497. doi:10.3390/sym10100497
- Wei, G. W. (2017a). Pythagorean fuzzy interaction aggregation operators and their application to multiple attribute decision making. Journal of Intelligent & Fuzzy Systems, 33(4), 2119–2132. doi:10.3233/JIFS-162030
- Wei, G. W. (2017b). Picture uncertain linguistic Bonferroni mean operators and their application to multiple attribute decision making. Kybernetes, 46(10), 1777–1800. doi:10.1108/K-01-2017-0025
- Wei, G. W. (2017c). Some cosine similarity measures for picture fuzzy sets and their applications to strategic decision making. Informatica, 28(3), 547–564. doi:10.15388/Informatica.2017.144
- Wei, G. W. (2018a). Some similarity measures for picture fuzzy sets and their applications. Iranian Journal of Fuzzy Systems, 15(1), 77–89.
- Wei, G. W. (2018b). Picture fuzzy Hamacher aggregation operators and their application to multiple attribute decision making. Fundamenta Informaticae, 157(3), 271–320. doi:10.3233/FI-2018-1628
- Wei, G. W. (2018c). TODIM method for picture fuzzy multiple attribute decision making. Informatica, 29(3), 555–566. doi:10.15388/Informatica.2018.181
- Wei, G. W., & Lu, M. (2017a). Dual hesitant Pythagorean fuzzy Hamacher aggregation operators in multiple attribute decision making. Archives of Control Sciences, 27(3), 365–395. doi:10.1515/acsc-2017-0024
- Wei, G. W., & Lu, M. (2017b). Pythagorean hesitant fuzzy Hamacher aggregation operators in multiple attribute decision making. Journal of Intelligent Systems. DOI: doi:10.1515/jisys-2017-0106
- Wei, G. W., & Lu, M. (2018a). Pythagorean fuzzy Maclaurin symmetric mean operators in multiple attribute decision making. International Journal of Intelligent Systems, 33(5), 1043–1070. doi:10.1002/int.21911
- Wei, G. W., & Lu, M. (2018b). Pythagorean fuzzy power aggregation operators in multiple attribute decision making. International Journal of Intelligent Systems, 33(1), 169–186. doi:10.1002/int.21946
- Wei, G. W., Gao, H., & Wei, Y. (2018a). Some q-rung orthopair fuzzy Heronian mean operators in multiple attribute decision making. International Journal of Intelligent Systems, 33(7), 1426–1458. doi:10.1002/int.21985
- Wei, G. W., Gao, H., Wang, J., & Huang, Y. H. (2018c). Research on risk evaluation of enterprise human capital investment with interval-valued bipolar 2-tuple linguistic information. IEEE Access, 6, 35697–35712. doi:10.1109/ACCESS.2018.2836943
- Wei, G. W., Lu, M., Alsaadi, F. E., Hayat, T., & Alsaedi, A. (2017a). Pythagorean 2-tuple linguistic aggregation operators in multiple attribute decision making. Journal of Intelligent & Fuzzy Systems, 33(2), 1129–1142. doi:10.3233/JIFS-16715
- Wei, G. W., Lu, M., Tang, X. Y., & Wei, Y. (2018b). Pythagorean hesitant fuzzy Hamacher aggregation operators and their application to multiple attribute decision making. International Journal of Intelligent Systems, 33(6), 1197–1233. doi:10.1002/int.21978
- Wei, G., & Wei, Y. (2018). Some single-valued neutrosophic dombi prioritized weighted aggregation operators in multiple attribute decision making. Journal of Intelligent & Fuzzy Systems, 35(2), 2001–2013. doi:10.3233/JIFS-171741
- Wei, Y., Liu, J., Lai, X., & Hu, Y. (2017b). Which determinant is the most informative in forecasting crude oil market volatility: Fundamental, speculation, or uncertainty? Energy Economics, 68, 141–150. doi:10.1016/j.eneco.2017.09.016
- Wei, Y., Yu, Q., Liu, J., & Cao, Y. (2018d). Hot money and China’s stock market volatility: Further evidence using the GARCH-MIDAS model. Physica A: Statistical Mechanics and Its Applications, 492, 923–930. doi:10.1016/j.physa.2017.11.022
- Wu, S. J., & Wei, G. W. (2017). Pythagorean fuzzy Hamacher aggregation operators and their application to multiple attribute decision making. International Journal of Knowledge-Based and Intelligent Engineering Systems, 21(3), 189–201. doi:10.3233/KES-170363
- Wu, S., Wang, J., Wei, G., & Wei, Y. (2018). Research on construction engineering project risk assessment with some 2-tuple linguistic neutrosophic Hamy mean operators. Sustainability, 10(5), 1536. doi:10.3390/su10051536
- Yager, R. R. (2001). The power average operator. IEEE Transactions on Systems, Man, and Cybernetics - Part A: Systems and Humans, 31(6), 724–731. doi:10.1109/3468.983429
- Yager, R. R. (2013). Pythagorean fuzzy subsets. Proceeding of The Joint IFSA Wprld Congress and NAFIPS Annual Meeting, Edmonton, Canada, pp. 57–61.
- Yager, R. R. (2014). Pythagorean membership grades in multicriteria decision making. IEEE Transactions on Fuzzy Systems, 22(4), 958–965. doi:10.1109/TFUZZ.2013.2278989
- Yu, D. J. (2013). Intuitionistic fuzzy geometric Heronian mean aggregation operators. Applied Soft Computing, 13(2), 1235–1246. doi:10.1016/j.asoc.2012.09.021
- Yu, S. M., Zhou, H., Chen, X. H., & Wang, J. Q. (2015). A multi-criteria decision-making method based on Heronian mean operators under a linguistic hesitant fuzzy environment. Asia-Pacific Journal of Operational Research, 32(05), 1550035. doi:10.1142/S0217595915500359
- Yue, Z., & Jia, Y. (2013). A method to aggregate crisp values into interval-valued intuitionistic fuzzy information for group decision making. Applied Soft Computing, 13(5), 2304–2317. doi:10.1016/j.asoc.2012.12.032
- Zadeh, L. A. (1965). Fuzzy sets. Information and Control, 8(3), 338–356. doi:10.1016/S0019-9958(65)90241-X
- Zeng, S. Z., Mu, Z. M., & Balezentis, T. (2018). A novel aggregation method for Pythagorean fuzzy multiple attribute group decision making. International Journal of Intelligent Systems, 33(3), 573–585. doi:10.1002/int.21953
- Zeng, S., Chen, J., & Li, X. (2016). A hybrid method for Pythagorean fuzzy multiple-criteria decision making. International Journal of Information Technology & Decision Making, 15(02), 403–422. doi:10.1142/S0219622016500012
- Zhang, R. T., Wang, J., Zhu, X. M., Xia, M. M., & Yu, M. (2017). Some generalized Pythagorean fuzzy Bonferroni mean aggregation operators with their application to multiattribute group decision-making. Complexity, 2017, 1–16. Volume Article ID 5937376, pages. doi:10.1155/2017/5937376
- Zhang, X. L., & Xu, Z. S. (2014). Extension of TOPSIS to multiple criteria decision making with Pythagorean fuzzy sets. International Journal of Intelligent Systems, 29(12), 1061–1078. doi:10.1002/int.21676
- Zhu, B., Xu, Z. S., & Xia, M. M. (2012). Hesitant fuzzy geometric Bonferroni means. Information Sciences, 205, 72–85. doi:10.1016/j.ins.2012.01.048