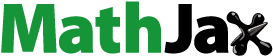
Abstract
Evaluating criteria selection has significant impacts on data envelopment analysis (DEA) efficiency estimates. Selecting the proper evaluation criteria lead to successful and meaningful results of decision-making. This study aims to use the Decision Making Trial and Evaluation Laboratory (DEMATEL) method to evaluate the most important constructs and criteria and also establish causality relationships among others for financial holding companies (FHCs) of banks’ operating ability in Taiwan. In this research 15 criteria were confirmed through reviewing various articles associated with this issue. Then, the information from the questionnaire was turned into the DEMATEL questionnaire and was distributed among nine experts and also members of the FHCs of Taiwan. The research results show that employees, total assets, total liabilities, non-interest income, income on investments, net profits before tax, net worth, and EPS are eight causal criteria. Furthermore, operating expenses, capital, interest expenses, interest income, operating income, return on assets (ROA), and return on equity (ROE) are seven effect criteria.
1. Introduction
The banking industry in Taiwan was under government protection and control before 1986. Such control was gradually released with the rise of financial liberalisation and following the changes in the business environment. The Taiwanese government also launched the first financial reform in 2004 to improve the structure and profitability of financial institutions in Taiwan and to revitalise the domestic economy. Compared with other countries, however, as financial institutions in Taiwan are smaller, more homogeneous and lack international competitiveness, the Taiwanese government launched a second financial reform (Financial Reform II), hoping to enhance the operational efficiency and competitiveness of financial institutions in Taiwan through continuous improvement of operational conditions of Taiwan’s financial industry. Sherman and Gold (Citation1985) were amongst the earliest scholars to measure the operational efficiency of financial institutions with data envelopment analysis (DEA). Previous studies measured the operational efficiency of the financial industry using the DEA and emphasised the effect of input and output on efficiency, which is identical with measuring the efficiency of the production process of general traditional industries. However, the characteristics of the financial industry are different from that of traditional industries. Therefore, the selection of proper criteria will lead to successful and meaningful results in the decision-making of financial holding companies (FHCs).
According to Seiford and Zhu (Citation1999) the financial industry has multiple inputs and multiple outputs. Wagner and Shimshak (Citation2007) pointed out that past studies often measured the operational efficiency of the financial industry with the data in the financial reports of financial institutions. Paradi and Zhu (Citation2013) found that little had been discussed in previous studies about how to reduce ‘not fully used’ inputs and how to increase expected outputs, so it is critical to select the appropriate inputs and outputs. Most studies referred to the input and output variables proposed in previous studies and analysed the ‘related criteria’ between input and output with the Pearson correlation test to explain the correlations between input and output variables. However, Paradi and Zhu (Citation2013) suggested that integrating financial theories with the DEA, which contains the opinion of more operators with practice experience, would be a better solution. Therefore, the purpose of this study intends to use the Decision Making Trial and Evaluation Laboratory (DEMATEL) method to select the most important criteria and establish causality relationships among others for FHCs operating ability in Taiwan. Apart from collating and inducing the variables proposed in previous studies, DEMATEL will examine these constructs and criteria using the questionnaire expert survey before testing them with ‘causality analysis’.
The rest of this article is organised as follows. Section 2 contains some ideas about constructs and criteria selection and DEMATEL method. Section 3 presents how this research adopted the methodology in the FHCs. Section 4 describes the results and Section 5 provide our conclusion and recommendations.
2. Literatures review
As efficiency is measured to improve organisational management, the selection of input and output items must correspond to management assessment. Also, as capital lending is the core business of banks, capital input and capital availability are the two major inputs, and profit is the major output. If the input and output items are negative values, Ali and Seiford (Citation1993) proposed the addition of a positive value to each item to ensure that the value is a positive real number. If the input or output value of an assessed unit is ‘0’, although this can still meet the linear programming requirements, this will become extremely unreasonable in actual situation. Also, as the analysis outcomes are difficult to explain, assessed units of such kind are often called the ‘outlier’, and they should be avoided. When accessing bank operational efficiency with DEA, the choice of input and output items varies due to different points of view. Believing that banks are the bridge between capital demanders and capital suppliers, Favero and Papi (Citation1995) selected the balance sheet titles as the input and output items. However, the production process of the financial industry differs from that of manufacturing industries. For example, the input and output of manufacturing industries are readily identifiable, the output is quantifiable and its value is measurable. In contrast, the financial industry absorbs capital with different tools, provides services, and provides capital demanders with different types of loans or financing. As the output services of the financial industry are difficult to quantify, scholars define the input and output of the financial industry in different ways. According to Bergendahl (Citation1998), it is important to analyse the assumptions and definitions of input and output with the DEA, and these assumptions and definitions vary based on the research aims and viewpoints of scholars. An FHC has a host of input and output items, general measuring methods include the production approach (Camanho & Dyson, Citation2008; Sturm & Williams, Citation2004) and the intermediation approach (Huang & Wang, Citation2004; Kwan, Citation2006; Ray, Citation2007; Weill, Citation2010).
Chao, Yu, and Chen (Citation2010) took an intermediation approach to measure the FHCs performance of Taiwan with a multi-activity DEA After reviewing 196 studies measuring the FHCs operational efficiency during 1998–2009, Fethi and Pasiouras (Citation2010) found that profitability and productivity were the most important outcomes, and the difference between input and output selection was significant when measuring financial institution efficiency with DEA After reviewing 80 published papers investigating the operational efficiency of financial institutions in 24 countries with DEA, Paradi and Zhu (Citation2013) suggested that it is necessary to reduce some constraints by analysing the input and output variables with DEA to ensure aggregation, as this will help to distinguish models from one another. For example, service employees, sales employees, and back-office employees can be categorised as employees in the input items. After summarising 100 past studies investigating the financial industry with DEA during 1990–2011, this article performs the variable analysis on the input and output items in these studies, including employees, operating fees, total capital asset (loan), total liability (deposits), spread income, non-spread income, profit from investment, net value, return on assets (ROA), return on equity (ROE), and earnings per share (EPS). Scholars analysed the correlations among both groups of variables with the Pearson correlation test to ensure that they met the input and output variable requirements in the DEA Elyasiani and Wang (Citation2012) used labour, fixed assets, deposits, non-interest expenses, loan, securities, non-interest income, security, and trading eight variables to examines whether banking holding company (BHC) diversification is associated with improvement or detriment its production efficiency. Hsu and Li (Citation2013) used a risk-adjusted return on capital (RAROC). framework as the basis of input and output through DEA to assessment performance of Taiwan’s 14 FHCs. Huang, Chen, and Yin (Citation2014) used fixed assets, equity, personnel expenses, deposits and short-term funding, other raised funds, gross loans, other earning assets and non-performing loans eight variables to assess the efficiency of a bank in China. Liu and Hsu (Citation2014) used ROA, ROE, and non-operating expenses ratio (NOE) to examine the determinants of profit performance of FHCs. Yang (Citation2014) used deposits, personnel expense, fixed assets, loans, portfolio investment, non-interest revenue, and non-performing loans seven variables to measure technical efficiencies of banks both standing-alone and subordinate to FHCs. Wang, Lu, and Liu (Citation2014) used total liability ratio, total equity ratio, unit employee cost, profit ratio, ROA, ROE, book-to-market equity ratio, earnings to price ratio eight variables to measure the performance of profitability and value creativity of US. BHCs. Curi, Lozano-Vuvas, and Zelenyuk (Citation2015) used labour, capital, interbank deposits, customer deposits, interbank loans, customer loans, securities, and directly charged services eight variables to measure operational efficiencies of foreign banks and explored the relations between diversification and efficiency. Wu (Citation2015) introduced loans, operating revenue, other revenue, deposits, number of employees, number of branches, and fixed capital seven variables into the DEA and the Malmquist productivity index (MPI) to examine the operational efficiency of Taiwan’s bank of FHCs. Peng et al. (Citation2017) used total deposits, number of employees, total fixed assets, total loans, other investment assets, and other non-interest income six variables to measure the banking industry of Taiwan. According to Jenkins and Anderson (Citation2003), apart from using scientific and statistical techniques, the selection of input and output variables for efficiency assessment should be practical and reasonable. Therefore, scholars began establishing causality models with Structural Equation Model (SEM) in recent years. However, after examining the flight safety efficiency of airlines with DEMATEL and SEM, Liou et al. (Citation2010) found that the former can reinforce the latter, which is often misused by users revising the model with data and even infer theories without theoretical support. Also, DEMATEL has been widely applied to test the criteria in e-learning, decision-making, knowledge management, operations research, customer behaviour, and selection system. Therefore, this study will test the correlations among the input and output variables with DEMATEL Apart from collating and inducing the variables proposed in past studies, this study will examine these constructs and criteria with questionnaire expert survey before testing them with ‘causality analysis’.
DEMATEL was developed by the Battelle Memorial Institute of Geneva during 1972–1976 in the Science and Human Affairs Program for constructing a mutually associated network-based structure to analyse the complex situations in the real world (Grbus & Fontela, Citation1972). DEMATEL effectively resolves complex and entangled social issues and explains the complex causality structure by means of hierarchical structures. It examines the pair-wise effect between constructs, criterion and calculates the causality and effect of all criteria by means of matrix (Liou et al., Citation2010). DEMATEL can be applied to analyse the correlations of issues among departments or criteria among issues under imprecise, uncertain and unpredictable environments, so as to find the causality among issues or criteria (Ho et al., Citation2011; Ilieva, Citation2017; Li & Mathiyazhagan, Citation2018; Si et al., Citation2018). DEMATEL designs the experience, knowledge, and opinion in a model structure. Compared with SEM, apart from reflecting the causality effect among variables with the impact-relation map (IRP), it provides criteria for comparison (Tzeng, Chiang, & Li, Citation2007). Therefore, DEMATEL explains the importance-impact relations within the cluster and verifies the mutual impact relations among criteria or clusters. Mirmousa and Dehnavi (Citation2016) used DEMATEL to evaluate 43 criteria for universities of Yazd selected supplier. Büyüközkan and Güleryüz (Citation2016) used DEMATEL to analyse and select the most appropriate renewable energy resources (RER) in Turkey. Lin, Hong, and Cheng (Citation2017) used DEMATEL to analyse key patent and relations of a light-emitting diode (LED) bicycle light. Song and Cao (Citation2017) used DEMATEL to evaluate relations between the criteria of a product–service system. Kumar and Dixit (Citation2018) used DEMATEL to evaluate the barriers to the management of waste of electrical and electronic equipment (WEEE). Li and Mathiyazhagan (Citation2018) used DEMATEL to analyse the influential indicators of sustainable supply chain adoption in the auto components manufacturing sector. Therefore, DEMATEL is a practical and reasonable approach to analyse relations between criteria. The detailed procedure of DEMATEL is briefly summarised as five steps:
Step 1: Defining the research problem
A literature review is required to explore and compile relevant data for designing the research problem. The views of experts are very significant to understand and achieve the desired goal.
Step 2: Establish a direct-relation matrix (A)
In step 2, each experts were asked to rate the barriers and to form a direct relationship matrix based on the scale. The scale designed has five levels: ‘0 (No influence), 1 (Very low influence), 2 (Low influence), 3 (High influence), 4 (Very high influence)’. The initial data can be obtained as the direct relationship matrix. To incorporate all the responses from Y respondent, the average direct relation matrix can be developed as EquationEquation (1)
(1)
(1) :
(1)
(1)
where, K = number of respondent with
;N = number of research criteria
Step 3: Normalising the direct-relation matrix (D):
Follow the basis of the direct-relation matrix of average matrix (M), the normalised matrix (D) can be obtained through EquationEquation (2)(2)
(2) :
(2)
(2)
Step 4: Attaining the total relation matrix (T):
The total relation matrix (T) is developed by using EquationEquation (3)(3)
(3) :
(3)
(3)
Step 5: Developing a causal diagram:
The sum of rows and sum of column
represents the vectors of the total relation matrix respectively. Subsequently, the horizontal axis vector
named as ‘Prominence’ exhibits the overall effect contributed and experienced by criteria ‘i’. Similarly, the vertical axis vector
named as ‘Relation’ may divide criteria ‘i’ into cause group and effect group. Generally, if
is positive, then criteria is grouped into cause group, while if
is negative, then the criteria is grouped into effect group (Tseng, 2009; Kumar & Dixit, Citation2018).
3. Methodology
3.1. Research constructs and criteria
As efficiency is measured to improve organisational management, the selection of input and output items must correspond to management assessment. Also, as capital lending is the core business of banks, capital input and capital availability are the two major inputs, and profit is the major output. When assessing bank operational efficiency with the DEA, the choice of input and output items varies due to different points of view. As it is difficult to investigate the management information in the production process of the financial industry with 1-DEA, The multi-DEA model is designed to examine the efficiency of individual service within different but highly homogeneous multi-subsidiary companies. The multi-DEA approach is developed to estimate relative efficiency for multi-activity decision-making units (DMUs) such as FHCs. The multi-stage DEA input–output criteria process is shown in .
Furthermore, most studies used the intermediation approach, as banks are considered as the mediator of financial services that input capital and labour to convert savings into loans and investments, with amount as the measuring unit. After reviewing the criteria-related literature, this study selected criteria based on those used in the related literature and the database of the Taiwan Economic Journal (Cheng, Liang, & Huang, Citation2014; Elyasiani & Wang, Citation2012; Huang & Chung, Citation2017; Lee & Yang, Citation2014; Liu & Hsu, Citation2014; Wu, Citation2012; Yen, Yang, Lin, & Lee, Citation2012). Also, the titles and data in the financial reports announced by the research samples during 2001–2010 are other sources of the criteria selection in this study. This study combined view of a multi-stage DEA and related literature and generalised sales ability, revenue ability, profitability and operational ability of four constructs. The descriptions of the four constructs are shown in . The list and description of the 15 criteria under the four constructs of this study selected are presented in .
Table A1. Description of four constructs.
Table A2. List and description of 15 criterions.
3.2. Data collection
So far, there are no related literatures of DEMATEL specifically demonstrating the appropriate number of experts for study, reliability, and validity (Lee, Tzeng, Yeih, Wang, & Yang, Citation2013; Si et al., Citation2018). As the operating abilities and criteria of the FHC of banks is the subject matter of this study, the assessment constructs included sales, revenues, profits and operation abilities. Because it covers a wide variety of aspects, the in-depth interview was applied to compensate for the inadequacy of the related literature and experience. Therefore, the respondent selection, those with a deep understanding and rich experience in the operating strategy of FHCs were selected. And this study collected nine respondents who were in service in an FHC Bank associate managers, officers of higher levels and senior consultants with president experience all had more than 20 years financial service length, which means respondents of this study all possessed the extensive experience to evaluate the performance of FHC The basic data and coding of respondents are shown in .
Table A3. Basic data and coding of respondents.
Moreover, in order to verify the results of DEMATEL analysis and enhance the reliability and validity of the study, the elite interview was conducted to this study to complement DEMATEL under limited sample size (Bhatia & Srivastava, Citation2018; Lee et al., Citation2013). The elite interview could provide valuable information concerning the scope, present status, difficulty and future development of the question for the researcher to better understand the present status of cases or scenarios from limited and representative respondents (Harvey, Citation2011; Mikecz, Citation2012; Morris, Citation2009; Stephens, Citation2007; Welch et al., Citation2002). In terms of this study, the elite interview not only further helped to establish causality of constructs and criteria with DEMATEL, but it also proposed suggestions after examining the relations of the constructs and criteria of the study by cross-verifying the results of DEMATEL analysis and elite interviews. This study smoothly interviewed nine respondents with open questions. The main aim of this study is to evaluate the criteria for FHC’s operating ability. The question of the elite interview was ‘Considering the financial environment today, what criteria are important to FHCs’ operating ability and performance?’
4. Result and discussion
4.1. The result of the elite interview
The result of the elite interview with nine respondents from the FHCs in banks showed in . First of all, due to highly sophisticated, competitive and uncertain business environment, the characteristics of the FHCs are different from that of traditional industries in Taiwan. Therefore, respondents of interviews suggested that FHCs should have multi-dimensional perspective in operating and evaluation to become competitive. Moreover, respondents also suggested that FHCs should be sensitive enough to adjust their investment policies based on the changes in the business environment, especially after the global financial crisis and euro zone debt crisis. According to the result of elite interviews, the criteria, ‘employee’ (H1), ‘interest income’ (I1), ‘non-interest income’ (I2), ‘income on investments’ (I3), ‘operating income’ (P1), ‘net profits before tax’ (P2) ‘ROA’ (S1), ‘ROE’ (S2), and ‘EPS’ (S3), were all the important criteria of FHCs and conducted in DEMATEL As to the result of DEMATEL, the importance of ‘income on investment’ was in third place with the weight of prominence and relation. Apart from ‘income on investment’ (I3), ‘interest income’ (I1), ‘non-interest income’ (I2), ‘ROA’ (S1), and ‘EPS’ (S3) were regarded as the major items to FHCs by respondents. In the result of DEMATEL, ‘interest income’ (I1), ‘non-interest income’ (I2), ‘ROA’ (S1), and ‘EPS’ (S3) were important criteria with influence and received influence. And the operating income has direct effects with ‘net profits before tax’ (P2), ‘income on investment’ (I3), and ‘interest income’ (I1) as the result of elite interviews. Therefore, results of DEMATEL were consistent with elite interviews and it also showed the validation of the study includes results of DEMATEL and nine rich experience respondents.
Table A4. Result of elite interviews.
4.2. Verification of importance and impact of constructs
The framework and inter-relationship of the operating efficiency of FHCs were verified with DEMATEL The mutual impact of the four constructs of operating abilities and 15 criteria were analysed to obtain the importance and impact and the net relation map as .
The total impact relations of operational ability assessment constructs are showed in . As shown in the table, revenue ability has the highest prominence (8.330), profitability the second highest (7.701), and operational ability the least (6.292). This suggests that revenue ability is the most influential and the most important construct in the entire structure; while ‘operational ability’ is the least influential.
Table A5. Total impact relationship of operating ability assessment constructs.
In terms of causality, sales ability and operational ability are the two of the four professional abilities with positive values; with an inclination toward the initial relation category, which belongs to the active influence criteria. The relationship value of operational ability is the greatest and most positive (0.421), suggesting that operational ability has a greater direct influence on other ability constructs than vice versa. As its prominence is the smallest, it has a weaker total impact relationship.
The relation value of revenue ability and profitability is negative, with an inclination toward the effect relation category, which belongs to the passive influence criteria. This suggests that other constructs influence these two criteria more than they can influence others. Profitability has the lowest relation value (-0.142), that is, it is a factor of low relationship but with a high prominence, with an inclination toward effect, representing that it is most easily influenced by other constructs. This result suggests that profitability is the most critical and most important core ability for enhancing operating efficiency, but it is most easily influenced by other active influence criteria.
4.3. Verification of importance and impact of criteria
The total impact relationship of 15 criteria is shown in . Moreover, the ‘strength of prominence and relationship of 15 criteria’ is shown in .
Table A6. Total impact relations of 15 criterions.
Table A7. Strength of prominence and relationship of 15 criterions.
As shown in the table, the top three criteria in prominence (r + d) are ‘interest expense’ (Y3), ‘non-interest income’ (I2), and ‘non-interest income’ (I3), suggesting that the total impact relationship and centrality of these three criteria are the highest among all input and output criteria. This is to say that they are the most important and most influential criteria among all the 15 input and output criteria. ‘Total asset’ (Y1) has the lowest centrality (6.292), representing that its centrality is the lowest among all input and output criteria and its total impact relationship is the weakest when compared to other criteria.
If relation (r – d) is a positive value, this means that the criterion is an active influence in the causality of all criteria and its net influence is positive. Seven of the 15 input–output criteria have an active influence, suggesting that they incline toward the initial relationship category. This is to say that these criteria have a greater direct influence on the others than vice versa. These criteria by their relationship strength in ascending order are: ‘EPS’ (S3), ‘total asset’ (Y1), ‘interest income’ (I1), ‘total liabilities’ (Y2), ‘non-interest income’ (I2), ‘net worth’ (P3) and ‘employees’ (H1).
If relation (r – d) has a negative value, this means that the criterion is a passive influence on the causality of all criteria. In this model, the 15 ability criteria with a negative value are categorised in the effect relation category, meaning that these ability criteria are influenced more easily by others than they can influence others. These criteria by relationship strength in the ascending order are: ‘ROA’ (S1), ‘capital’ (H3), ‘net profits before tax’ (P2), ‘interest expense’ (Y3), ‘interest income’ (I1), ‘operating expenses’ (H2), and ‘ROE’ (S1). ‘ROE’ (S1) has the lowest negative relationship value (–0.862), suggesting that ‘ROE’ (S1) is most easily influenced by other criteria.
Concluding the above, the criteria of operating ability of FHCs constructed by this study verified the operating ability constructs and criteria of FHCs. By further analysing the impact relationship and inter-relationship among individual operating ability constructs and criteria with DEMATEL, the constructs and criteria that are the most critical core abilities and the most important operating abilities of FHCs are located. From the causality among constructs and criteria, we can better understand the mutual influence among the ability constructs and criteria. When assessing operational efficiency, these results provide a reference for prioritising the improvement of operating abilities to enhance operating efficiency.
Expert interviews and the causality survey were implemented in accordance with the steps in DEMATEL The outcomes of the test for causality among the criteria showed a high impact relationship among the criteria. The four constructs of this study included: operational ability, sales ability, profitability and revenue ability. The criteria in each construct included: ‘employees’ (H1), ‘operating expenses’ (H2), ‘capital’ (H3), ‘total assets’ (Y1), ‘total liabilities’ (Y2) and ‘interest expenses’ (Y3) in sales ability; ‘interest income’ (I1), ‘non-interest income’ (I2) and ‘income on investments’ (I3) in revenue ability; ‘operating income’ (P1), ‘net profits before tax’ (P2), and ‘net worth’ (P3) in profitability; and ‘ROA’ (S1), ‘ROE’ (S2), and ‘EPS’ (S3) in revenue ability.
5. Conclusion
Based on the related literature and elite interviews, operating constructs, criteria and their cause–effect relations were generalised and measured with a quantitative survey. As the DEMATEL outcomes show, in the total prominence impact, ‘interest expense’ (Y3), ‘interest income’ (I1), and ‘non-interest income’ (I2) are the three most influential criteria affecting relations and the core. This implies that ‘interest expense’ (Y3) is the payment for capital gain, that is, the capital cost of deposits is the prime concern in the operating process. Next, ‘interest income’ (I1), the main income source in traditional FHC operation representing the gap between interest income from lending and interest expense for deposits, was still a major output regardless of the changes in the financial business environment in recent years. ‘Non-interest income’ (I2) is the service fee charged from the sales of wealth management products, which is another major variable increasing output. ‘EPS’ (S3) and ‘total assets’ (Y1) are the powerful criteria directly influencing other criteria amongst the cause criteria of relation. This implies that the highest ‘EPS’ (S3) is the criteria measuring the final operational efficiency of FHCs. ‘Total assets’ (Y1) are the lending business and financial product sales of FHCs, which are the largest income source with direct influence on the criteria at different operational stages. Amid the effect criteria of relation, ‘ROE’ (S2) and ‘capital’ (H3) have the highest received influence. That is, they are most easily influenced by other input and output variables. This implies that enterprises must make investments and invest various operating fees so as to increase revenues. Therefore, ‘capital’ (H3) is easily influenced by other inputs and outputs. The net value is the accumulative value of an enterprise’s overall operation. Therefore, the influence of other criteria on ‘ROE’ (S2) also increases.
Conclusively, given the characteristic of multiple inputs and multiple outputs of FHC, using statistical methods to explore and analyse performance criteria of FHCs is challenging today. Due to the limited data and construct, previous studies with statistical methods, such as SEM, still encountered obstacles on dealing with negative value and complicated constructs (Jenkins & Anderson, Citation2003; Seiford & Zhu, Citation1999). This study collected 15 criteria from previous studies and categorised as four constructs. For future studies, these constructs and criteria could not only help researchers have more awareness of FHCs operation but also help them choose the correct criteria to evaluate the performance based on their research subjects. It is also the first contribution and novelty of the study. Moreover, the net relation map of 15 criteria was analysed and presented by using DEMATEL in the study. And the net relation map not only visualised complicated relations among criteria but also extended the input and output perspective for FHCs’ managers. In the meanwhile, FHCs’ managers could develop a clear strategic map and make a more detailed and accurate strategy through the net relation map. It is the second contribution and novelty of the study. Finally, although DEMATEL could explain the importance of impact relations within the cluster and verifies the mutual impact relations among criteria or clusters, but there were no related literatures demonstrated specifically the appropriate number of experts for study, reliability, and validity today (Lee et al., Citation2013; Si et al., Citation2018). Therefore, this study linking qualitative and quantitative approaches, the elite interview and DEMATEL, to evaluate performance criteria for FHC would compensate for the inadequacy of previous studies and enhance the validity of the study. It is the third contribution and novelty of the study.
Disclosure statement
No potential conflict of interest was reported by the authors.
References
- Ali, A. I., & Seiford, L. M. (1993). Computational accuracy and infinitesimals in data envelopment analysis. INFOR: Information Systems and Operational Research, 31(4), 291–297. doi: 10.1080/03155986.1993.11732232
- Bergendahl, G. (1998). DEA and benchmarks—An application to Nordic banks. Annals of Operations Research, 82, 233–250. doi: 10.1023/A:1018910719517
- Bhatia, M. S., & Srivastava, R. K. (2018). Analysis external barriers to remanufacturing using grey-DEMATEL approach: An Indian perspective. Resources, Conservation & Recycling, 136, 79–87.
- Büyüközkan, G., & Güleryüz, S. (2016). An integrated DEMATEL-ANP approach for renewable energy resources selection in Turkey. International Journal of Production Economics, 182, 435–448. doi: 10.1016/j.ijpe.2016.09.015
- Camanho, A. S., & Dyson, R. G. (2008). A generalization of the Farrell cost efficiency measure applicable to non-fully competitive settings. Omega, 36(1), 147–162. doi: 10.1016/j.omega.2005.12.004
- Chao, C. M., Yu, M. M., & Chen, M. C. (2010). Measuring the performance of financial holding companies. The Service Industries Journal, 30(6), 811–829. doi: 10.1080/02642060701849857
- Cheng, C.-P., Liang, L.-W., & Huang, C.-T. (2014). Effect of internationalization on the cost efficiency of Taiwan’s banks. Emerging Markets Finance and Trade, 50(sup6), 204–228. doi: 10.1080/1540496X.2014.1013857
- Curi, C., Lozano-Vuvas, A., & Zelenyuk, V. (2015). Foreign bank diversification and efficiency prior to and during the financial crisis: Does one business model fit all? Journal of Banking & Finance, 61(sup1), 22–35. doi: 10.1016/j.jbankfin.2015.04.019
- Elyasiani, E., & Wang, Y. (2012). Bank holding company diversification and production efficiency. Applied Financial Economics, 22(17), 1409–1428. doi: 10.1080/09603107.2012.657351
- Favero, C. A., & Papi, L. (1995). Technical efficiency and scale efficiency in the Italian banking sector: A non-parametric approach. Applied Economics, 27(4), 385–395. doi: 10.1080/00036849500000123
- Fethi, M. D., & Pasiouras, F. (2010). Assessing bank efficiency and performance with operational research and artificial intelligence techniques: A survey. European Journal of Operational Research, 204(2), 189–191. doi: 10.1016/j.ejor.2009.08.003
- Grbus, A., & Fontela, E. (1972). World problems an invitation to further thought within the framework of DEMATEL. Geneva: Battle Geneva Research Centre.
- Harvey, W. S. (2011). Strategies for conducting elite interviews. Qualitative Research, 11(4), 431–441. doi: 10.1177/1468794111404329
- Ho, W. R. J., Tsai, C. L., Tzeng, G. H., & Fang, S. K. (2011). Combined DEMATEL technique with a novel MCDM model for exploring portfolio selection based on CAPM. Expert Systems with Applications, 38(1), 16–25. doi: 10.1016/j.eswa.2010.05.058
- Hsu, J., & Li, K. A. (2013). Performance assessments of Taiwan’s financial holding companies. Journal of Productivity Analysis, 40(1), 137–151. doi: 10.1007/s11123-012-0321-z
- Huang, J., Chen, J., & Yin, Z. (2014). A network DEA model with super efficiency and undesirable outputs: An application to bank efficiency in China. Mathematical Problems in Engineering, 2014, 1–14. doi: 10.1155/2014/793192
- Huang, T. H., & Chung, M. T. (2017). Do undesirables matter on the examination of banking efficiency using stochastic directional distance functions. The Quarterly Review of Economics and Finance, 65, 194–211. doi: 10.1016/j.qref.2016.09.007
- Huang, T. H., & Wang, M. H. (2004). Comparisons of economic inefficiency between output and input measures of technical inefficiency using the Fourier flexible cost function. Journal of Productivity Analysis, 22(1/2), 123–142. doi: 10.1023/B:PROD.0000034747.85182.78
- Ilieva, G. (2017). Group decision analysis with interval type-2 fuzzy numbers. Cybernetics and Information Technologies, 17(1), 31–44. doi: 10.1515/cait-2017-0003
- Jenkins, L., & Anderson, M. (2003). A multivariate statistical approach to reducing the number of variables in data envelopment analysis. European Journal of Operational Research, 147(1), 51–61. doi: 10.1016/S0377-2217(02)00243-6
- Kumar, A., & Dixit, G. (2018). Evaluating critical barriers to implementation of WEEE management using DEMATEL approach. Resources, Conservation & Recycling, 131, 101–121.
- Kwan, S. H. (2006). The X-efficiency of commercial banks in Hong Kong. Journal of Banking & Finance, 30(4), 1127–1147. doi: 10.1016/j.jbankfin.2005.05.016
- Lee, H. S., Tzeng, G. H., Yeih, W., Wang, Y. J., & Yang, S. C. (2013). Revised DEMATEL: Resolving the infeasibility of DEMATEL. Applied Mathematical Modelling, 37(10–11), 6746–6757. doi: 10.1016/j.apm.2013.01.016
- Lee, Y. C., & Yang, Y. H. (2014). Performance of financial holding companies in Taiwan: An application of network data envelopment analysis. Global Journal of Business Research, 8(3), 19–30.
- Li, Y., & Mathiyazhagan, K. (2018). Application of DEMATEL approach to identify the influential indicators towards sustainable supply chain adoption in the auto components manufacturing sector. Journal of Cleaner Production, 172, 2931–2941.
- Lin, Z. C., Hong, G. E., & Cheng, P. F. (2017). A study of patent analysis of LED bicycle light by using modified DEMATEL and life span. Advanced Engineering Informatics, 34, 136–151. doi: 10.1016/j.aei.2017.09.004
- Liou, J. J. H., Tzeng, G. H., Tsai, C. Y., & Hsu, C. C. (2010). A hybrid ANP model in fuzzy environments for strategic alliance partner selection in the airline industry. Applied Soft Computing, 11(4), 3515–3524. doi: 10.1016/j.asoc.2011.01.024
- Liu, W. C., & Hsu, C. M. (2014). Profit performance of financial holding companies: Evidence from Taiwan. Emerging Markets Finance and Trade, 50(sup3), 190–200.
- Mikecz, R. (2012). Interviewing elites: Addressing methodological issues. Qualitative Inquiry, 18(6), 482–493. doi: 10.1177/1077800412442818
- Mirmousa, S., & Dehnavi, H. D. (2016). Development of criterions of selecting the supplier by using the fuzzy DEMATEL method. Procedia - Social and Behavioral Sciences, 230, 281–289. doi: 10.1016/j.sbspro.2016.09.036
- Morris, Z. S. (2009). The truth about interviewing elites. Politics, 29(3), 209–217. doi: 10.1111/j.1467-9256.2009.01357.x
- Paradi, J. C., & Zhu, H. (2013). A survey on bank branch efficiency and performance research with data envelopment analysis. Omega, 41(1), 61–79. doi: 10.1016/j.omega.2011.08.010
- Peng, J. L., Jeng, V., Wang, J. L., & Chen, Y. C. (2017). The impact of bancassurance on efficiency and profitability of banks: Evidence from the banking industry in Taiwan. Journal of Banking & Finance, 80, 1–13. doi: 10.1016/j.jbankfin.2017.03.013
- Ray, S. C. (2007). Are some Indian banks too large? An examination of size efficiency in Indian banking. Journal of Productivity Analysis, 27(1), 41–56. doi: 10.1007/s11123-006-0022-6
- Seiford, L. M., & Zhu, J. (1999). Profitability and marketability of the top 55 US. commercial banks. Management Science, 45(9), 1270–1288. doi: 10.1287/mnsc.45.9.1270
- Sherman, H. D., & Gold, F. (1985). Bank branch operating efficiency: Evaluation with data envelopment analysis. Journal of Banking & Finance, 9(2), 297–315. doi: 10.1016/0378-4266(85)90025-1
- Si, S. L., You, X. Y., Liu, H. C., & Zhang, P. (2018). DEMATEL technique: A systematic review of the state-of-the-art literature on methodologies and applications. Mathematical Problems in Engineering, 2018, 1–33. doi: 10.1155/2018/3696457
- Song, W., & Cao, J. (2017). A rough DEMATEL-based approach for evaluating interaction between requirements of product-service system. Computers & Industrial Engineering, 110, 353–363. doi: 10.1016/j.cie.2017.06.020
- Stephens, N. (2007). Collecting data from elites and ultra elites: Telephone and face-to-face interviews with macroeconomists. Qualitative Research, 7(2), 203–216. doi: 10.1177/1468794107076020
- Sturm, J. E., & Williams, B. (2004). Foreign bank entry, deregulation and bank efficiency: Lessons from the Australian experience. Journal of Banking & Finance, 28(7), 1775–1799. doi: 10.1016/j.jbankfin.2003.06.005
- Tzeng, G. H., Chiang, C. H., & Li, C. W. (2007). Evaluating intertwined effects in e-learning programs: A novel hybrid MCDM model based on factor analysis and DEMATEL. Expert Systems with Applications, 32(4), 1028–1044. doi: 10.1016/j.eswa.2006.02.004
- Wagner, J. M., & Shimshak, D. G. (2007). Stepwise selection of variables in data envelopment analysis: Procedures and managerial perspectives. European Journal of Operational Research, 180(1), 57–67. doi: 10.1016/j.ejor.2006.02.048
- Wang, W. K., Lu, W. M., & Liu, P. Y. (2014). A fuzzy multi-objective two-stage DEA model for evaluating the performance of US bank holding companies. Expert Systems with Applications, 41(9), 4290–4297. doi: 10.1016/j.eswa.2014.01.004
- Weill, L. (2010). Does restructuring improve banking efficiency in a transition economy? Applied Economics Letters, 9(5), 279–281. doi: 10.1080/13504850110068125
- Welch, C., Marschan-Piekkari, R., Penttinen, H., & Tahvanainen, M. (2002). Corporate elites as information in qualitative international business research. International Business Review, 11(5), 611–628. doi: 10.1016/S0969-5931(02)00039-2
- Wu, H. Y. (2012). Constructing a strategy map for banking institutions with key performance indicators of the balanced scorecard. Evaluation and Program Planning, 35(3), 303–320. doi: 10.1016/j.evalprogplan.2011.11.009
- Wu, M. T. (2015). The impact of transformation on economic efficiency—A case study of financial holding companies in Taiwan. Journal of the Asia Pacific Economy, 20(3), 465–488. doi: 10.1080/13547860.2015.1054170
- Yang, C. C. (2014). An enhanced DEA model for decomposition of technical efficiency in banking. Annals of Operations Research, 214(1), 167–185. doi: 10.1007/s10479-011-0926-z
- Yen, G. F., Yang, Y. H., Lin, Y. H., & Lee, A. K. (2012). Operating performance analysis of Taiwan’s financial holding companies: Using super SBM efficiency model and Co-plot analysis. International Journal of Organizational Innovation, 5(2), 141–163.
Appendix
Appendix A: Tables
Appendix B: Figures