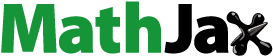
Abstract
The purpose of the paper is to outline the empirical framework of the model of the impact misallocation of human capital on productivity (TFP).Using provincial panel data from 2001 to 2015, this paper studies the effect of the misallocation of human capital on productivity in China. We find that misallocation of human capital reduces China’s productivity significantly. Most importantly, we argue that the important channels through which misallocation of human capital affects productivity are industrial structure upgrading, technological innovation and labour productivity. Furthermore, counterfactual experiments show that eliminating the labour mismatch between industries completely could be associated with an increase in productivity of around 41% for the whole sample in China. The results suggest correcting the current imperfections of incentives in non-productive sectors, where encouraging more human capital to work in high-tech enterprises may be a vital measure to stimulate the development of emerging economies.
1. Introduction
One of the most obvious changes to human capital allocation in China in recent years has been the unbalanced human capital allocation between various industries. It has emerged that, within China, a considerable number of workers choose to work in government bureaucracy and in high-income monopoly industries, especially in the financial industry. In comparison, the labour force in the computer and high-tech industries is in seriously short supply.
Murphy, Shleifer, and Vishny (Citation1991) argue that allocation of human capital–time, labour and effort–is extremely sensitive to the quality of institutions. Of special importance is the distribution of talent and entrepreneurship, as they are key drivers of economic growth. However, in China, in most cases, the occupational choice of workers is not entrepreneurs but entering the government bureaucracy, monopoly industries and finance.
In this context, one natural question arises: does the misallocation of human capital in China have an impact on productivity? To answer this question, the paper contains an exploratory study to outline the empirical framework of the model of the impact misallocation of human capital has on productivity. The study was based on the example of the Chinese economy. More specifically, we wish to understand and (if possible) explain, provided that there were alterations in human capital allocation's extent over time, this could have led to a substantial decrease in total factor productivity (TFP).We also wish to uncover mechanisms involved in running from misallocation of human capital to productivity.
By studying the case of China, this paper presents new evidence on the impact of misallocation of human capital on economic performance and, in particular, on productivity. A large body of literature has examined the link between allocation of resource and economic performance across countries (see, e.g., Alfaro, Charlton, & Kanczuk, Citation2008; Hsieh & Klenow, Citation2009; Midrigan & Xu, 2010; Restuccia & Rogerson, Citation2008).Specifically, using producer-level data, Midrigan and Xu (Citation2014) have measured fiscal frictions' role in effecting productivity. However, relatively little attention has been paid to how the misallocation of human capital affects productivity. Previous research on mismatch of human capital and TFP has not identified any mechanisms to account for the link between misallocation of human capital and productivity.
Our paper contributes to the existing literatures in three different ways. First, the research contributes to the literature on resource misallocation's role in accounting for aggregate productivity across countries (Hsieh & Klenow, Citation2009; Midrigan & Xu, Citation2014).Our research follows the work of Hsieh and Klenow (Citation2009), who analyse manufacturing units in China, India and the U.S. Several applications of research to other countries have reached the conclusion that misallocation can be the cause of significant aggregate TFP decline. Also, the research evidence helps to extend the existing literature by taking into account the role of human capital’s allocation among industries in different TFP, which has been neglected in the existing literature.
Secondly, our study extends the existing literature by considering mechanisms to account for the link between the misallocation of human capital and productivity. Here we have tested three mechanisms and argue that misallocation of human capital can reduce productivity via three channels. It adds to the limited empirical studies on the link of misallocation of human capital and TFP in the context of an emerging economy, providing evidence that the important channels through which misallocation of human capital affects productivity are industrial structure upgrading, technological innovation and labour productivity.
Thirdly, relative to the existing work, our contribution has been to introduce a new perspective on the role of misallocation of human capital in productivity and presents new evidence on the impact of this misallocation on economic performance by studying the case of China. Our findings help to extend the role of human capital's desirable allocation in emerging economies.
Our key contribution has been to underline the usefulness of considering policies that introduce incentives to allocate more human capital to innovative activities, especially in obtaining sustainable development in the future. Behind China’s current economic downturn, our findings provide a novel clue for solving this puzzle made up of the following elements:correcting the current imperfections of incentives in the non-productive sectors and encouraging more workers to enter high-tech enterprises that may prove crucial measures in stimulating the development of emerging economies.
The rest of the paper is structured as follows. The next section presents a brief literature review. The following sections then focus on research methodology outlines, model specification, methodology and data. The penultimate section discusses the empirical results and their interpretation, while the conclusion is reported in the final section.
2. Literature review
The impact of allocation of resource on productivity has become one of the main topics of growth and development research. Theoretically, this paper is related to two strands of literature.
First, our study is related to literature on the effects of resource allocation on productivity. Recent literature has highlighted the negative effects of the allocation of resources on productivity (see, e.g., Alfaro et al., Citation2008; Hsieh & Klenow, Citation2009; Restuccia & Rogerson, Citation2008).
Hsieh and Klenow (Citation2009), for example, take manufacturing micro data on establishments as a tool for quantifying the potential extent of misallocation in China and India in comparison to the U.S; their finding is that moving to “U.S. efficiency” will increase TFP by 30–50% in China and 40–60% in India. Moreover, if capital has accumulated in response to aggregate TFP gains, then output gains will be roughly twice as large. Another finding is that deteriorating allocation levels may have taken 2% from the Indian manufacturing sector’s TFP growth from 1987 to 1994, whereas China may have boosted its TFP by 2% per year over 1998–2005 by spreading its distortions.
Furthermore, using producer-level data, Midrigan and Xu (Citation2014) have measured fiscal frictions' role in deciding total factor productivity (TFP).They realize that fiscal frictions can diminish significantly by nearly 40% the degree of yield, consumption and TFP in our model economy. A large amount of these losses is on account of the distortions. In contrast, the TFP losses from misallocation of capital among modern-sector producers are substantially smaller (5–10%) and occupy only a fraction of the total efficiency losses that are connected with a tightening of funding restraints.
Recently, for the most part stimulated by these considerations, a huge quantity of research has attempted to identify particular mechanisms to explain why TFP differences are not eliminated by market-based resource reallocation. One well-known case is Olley and Pakes (Citation1996), in research of productivity development in the telecommunications facilities industry. They found that the reallocation of yield to more productive corporations explains a large part of aggregated productivity growth. A common element of the emerging literature outlined above is that heterogeneity in productivity performance on a firm level may suggest resources' misallocation across firms with adverse impacts at the aggregated level. Although existing literature has recognised that resource allocation has some negative effects on productivity performance, the issue of the impact of human capital allocation on productivity has not been fully explored.
Secondly, this paper is also related to literature on the effect of human capital mismatch and productivity. Although the centrality of human capital accumulation for economic development has been firmly established (Romer, Citation1989), evidence of the significance of human resources allocation to jobs is only beginning to emerge. Here, McGowan and Andrews (Citation2015) examine the relationship between misallocation and labour productivity by using cross-country industry data for many OECD nations. Applying misallocation indicators aggregated from micro-data, they indicate that greater skills and qualification mismatch are related to lower labour productivity, with under-qualification and over-skilling explaining most of this impact. However, only a few comparative, descriptive studies have been carried out on the issue of human capital mismatch and productivity. Very little is known about human capital mismatch and how it affects productivity in different industries. In addition, there is a lack of formal evidence showing the impact of the misallocation of human capital on productivity, as is shown by studying the case of China. Assuming an elimination of mismatch of human capital between industries, to what extent will productivity increase?
In the strands of the existing literature outlined above1, it is observable that although economic studies have devoted a large amount of attention to the first strand, the second one has stayed largely unexplored. We aim to fill these gaps by examining the impact of misallocation of human capital on productivity (TFP). Specifically, we examine the mechanisms involved here.
3. Model and empirical design
To examine the relationship between mismatch and productivity, we estimate a main regression of the following form:
(1)
(1)
where the dependent variable is the total factor productivity for province i in industry j each year. The explanatory variables (hmatchjit) are a set of misallocation of human capital which measure misallocation of human capital for province i in industry j each year. Controls are variables that may influence
whereas
and
are individual and year fixed effects. Finally,
is an error term. The coefficient of interest is
capturing the impact of mismatch on productivity.
As many factors affect productivity, we must control for these factors to overcome the bias of omitted variables. Following the steps outlined in previous literature (Hsieh & Klenow, Citation2009; McGowan & Andrews, Citation2015), we select Labour force proportion and Human capital level as control variables. In a similar way, we control for education, which is predicted to be positively connected with productivity. For the same reason, our controls also include the openness index measured by the proportion of total imports and exports to GDP and the fixed capital formation rate derived by Hsieh and Klenow (Citation2009). Furthermore, we control for the level of government expenditure and original technological progress derived by Midrigan and Xu (Citation2014). We also include wages as control variable, as wages are an important channel for labour allocation, especially highly skilled examples. We believe this is relevant not only for labour allocation, but also further productivity in currently less productive sectors (Garnaut & Johnston, Citation2016). Finally, the fixed effects are controlled for other unobserved factors that do not alter within years or provinces.
4. Empirical analysis
4.1. Data
Our sources of data on productivity (TFP) are from China's Yearly Survey of Industrial Firms (CASIF), which is provided through the National Bureau of Statistics (NBS) of China. Explanatory variables and various control variables come from the China Statistical Yearbook. We utilize a panel data set of 31 provinces over the years from 2001 to 2015 to estimate EquationEquation (1)(1)
(1) .
The definitions and data sources of key variables are detailed in .
Table 1. Measurement and source of variables.
shows some descriptive statistics and reports the correlation matrix among all dependent and explanatory variables. It is shown that, among the key variables, the Hmatch is significantly negatively related to TFP, Labour_proportion, Openness and Orig_tech, also the univariate correlation between TFP and Labour_proportion, Human_capital, Educ, Orig_tech is significantly positive. The correlations between the variables show the predicted signs and most of the coefficients are statistically significant, providing preliminary evidence for our research objective.
Table 2. Descriptive statistics.
Table 3. Correlation matrix.
4.2. Benchmark test
EquationEquation (1)(1)
(1) is estimated using the ordinary least squares (OLS) by pooling together the time-series and cross-section data. Panel data analysis is also conducted to account for regional heterogeneity across provinces in .
Table 4. Baseline results of the link between mismatch and productivity.
In the first specification, we estimate model (1) with TFP as a dependent variable and report these results in . In the first step, we run an OLS regression between mismatch of human capital and productivity in columns (1). Then we conduct estimations using both the random effect and fixed effect models in columns (2) and columns (3).In the second step, we add all control variables into the regression to test the effects of mismatch of human capital on productivity by the pooled data and panel data analyses (columns (4)–columns (6)).
In the first column with no control variables, the coefficient of the mismatch of human capital is, as expected, negative and highly significant. When we add our control variables (columns (4)), the negative association between productivity and mismatch of human capital remains highly significant and grows in magnitude.
Then turning to columns (5) and columns (6), after we add our control variables, we find significantly negative effects of mismatch of human capital on productivity in both the fixed effect and the random effect models, with the estimated coefficients being around −.102 and −.109, respectively. The high values of obtained in the Hausman test (65.71) suggest that the null hypothesis of random effects is rejected. Thus, the effect of mismatch of human capital on productivity in this case is −.102 as the fixed effect model shows, a little smaller than the pooled data estimation in columns (4).
As can be seen, the effects of mismatch of human capital on productivity are statistically significant and negative in all the cases in . The magnitudes of human capital misallocation effects on productivity are relatively large: a 1% increase in the mismatch is associated with a 0.102% decrease of total productivity.
4.2. Endogenous
Endogeneity problems may exist between misallocation of human capital and productivity (Acemoglu, Citation1995; Ebeke et al., Citation2015; Sanders & Weitzel, Citation2013).First, there may be a reverse causation between human capital mismatch and TFP; for example, low productivity could affect people’s occupation choice. Owing to productivity decline, people are more willing to enter a bureaucracy or a monopoly industry for stability.
Secondly, our set of controls could neglect some perhaps unobserved factors likewise correlated with productivity. For example, government regulation is not only the principal factor leading to the mismatch of human capital, but it also has an impact on productivity. Hence, endogeneity may lead to biased estimates. To deal with the possible endogeneity issue existing here, we will conduct a 2SLS test between human capital's misallocation and productivity.
Here, we select employment culture as instrument variable. Employment cultures are significantly related to misallocation of human capital. In China, for instance, it is generally believed that northerners are prouder of working in a bureaucracy, while southerners are more willing to make money by conducting business (Sørensen, Citation2007). At the same time, this culture may influence the labour market by affecting individual career choices, which in turn affects productivity.
The link is not so obvious between employment cultures and productivity. We cannot come to the conclusion that some provinces are more productive simply as these provinces exhibit a particular employment culture. As a result, we think that employment culture is a good instrument variable for the misallocation of human capital.
The ratio of college enrolments in law to total college enrolments, and the same ratio for engineering are significantly related to employment culture and is often used as a proxy variable in the allocation of human capital research literature (Murphy et al., Citation1991).The reason why we choose as a denominator total enrolments rather than population, or population of a given age, is that we are interested in the allocation of the most able people between fields. In fact, the fractions of college enrolment in law and engineering serve to measure the incentives found in these subject fields in contrast to the numbers in college more generally.
The results of the 2SLS regression are shown in . We choose Employment culture as instrument variable. Employment culture is measured by the ratios of college enrolment in law and engineering. We find that the results for hmatch are all consistent with our bench test. The sign of the coefficients of the hmatch variable is still negative, but significant. On the whole, the 2SLS test's outcomes are basically the same as the benchmark OLS test.
Table 5. Misallocation of human capital and productivity (2SLS estimation).
4.3. Robustness checks
4.3.1. The impact of different industries on productivity
We use various modifications of our empirical model to check the robustness of the above findings and particularly to address the concern that our control variables do not fully eliminate the omitted variable bias that might be expected for the extremely diverse group of industries in our sample. In addition, we consider the issue that the allocation of human capital in different industries has differing effects on productivity. To do so, we reduce the sample to various more homogeneous sub-groups and estimate model (1) for such sub-groups with in each year as a measure of misallocation of human capital and TFP as the dependent variable.
First, we restrict our estimation to the high-tech industry. Next, we perform the other exercise by retaining only the research and technology services industry of the sample. The four other estimations are confined to the categories Real estate, Finance, Government sector and Monopoly. Our industry classification is based on the results of .
The results of the robustness checks are shown in . The coefficient of hmatch is strongly negative and significant across all of the above specifications. The value of this coefficient is higher for Real estate, Finance, Government sector and Monopoly industry than for the sample of the high-tech industry, Research and technology services.
Table 6. Robustness checks.
The human capital mismatch of the Real estate industry, Finance, Government sector and Monopoly industry seriously reduces productivity and the effect grows in magnitude. For example, for Real estate, a 1% increase in the mismatch is associated with a 0.215% decrease in total productivity. For Finance, a 1% increase in the mismatch is associated with a 0.229% decrease of total productivity. For the Government sectors, a 1% increase in the mismatch is associated with a 0.249% decrease of total productivity. For Monopoly, a 1% increase in the mismatch is associated with a 0.255% decrease of total productivity.
China's finance, public institutions and monopoly industries have extremely high human capital intensity (Hsieh & Klenow, Citation2009), but the productivity of these industries has been significantly reduced. The reason is that serious human capital mismatch hinders technological innovation, inhibits the improvement of labour productivity and thus reduces productivity. Instead, human capital with innovative potential is likely to be employed in non-productive, non-innovative sectors owing to the existence of salary incentives. The employment of too many excellent human capitals in the above industries will inevitably lead to a large waste of human capital; they cannot display their strengths in the corresponding departments and so reduce a country's productivity.
4.3.2. Different periods test
We reduce the sample to three different period sub-groups and estimate model (1) for such sub-groups with the in each year as a measure of misallocation of human capital and TFP as the dependent variable. The results of the different time periods checks are shown in . We find that the coefficients of the hmatch variable is still negative, and significant. The results are basically the same as the benchmark OLS test and 2SLS estimation.
Table 7. Different periods test.
However, our empirical results show that the absolute coefficients of the hmatch variable in 2006–2010 years and 2011–2015 years are much higher than in 2001–2005.This suggests that with the deepening of China’s reform, the misallocation of human capital has not been alleviated. The effect of human capital mismatch on TFP has increased. This is mainly because with the aggravation of misallocation of human capital in China, the release of human capital dividend is hindered. Human capital mismatch makes it impossible to transform human capital into real productive forces, thus inhibiting the promotion of total factor productivity.
4.4. Counterfactual experiment
If we reduce the labour mismatch at different level, how much will productivity gain? We carried out these counterfactual experiments.
Suppose the human capital mismatch is reduced by half, that is
is the distortion of labour factors in industry j, followed by Hsieh and Klenow (Citation2009) who use the degree of dispersion to indicate the degree of mismatch of elements, and the standard deviation of the new factor mismatches becomes 1/2 of the original.
Suppose the human capital mismatch is completely eliminated, that is
Suppose the human capital mismatch is reduced to the best practice of the U.S.A., we get the data of U.S. human capital mismatch from Hsieh, Hurst, Jones, and Klenow (Citation2013).
shows a counterfactual productivity outcome based on reducing the human capital mismatch level in half between industries, eliminating the human capital mismatch completely, and also supposing the human capital mismatch is reduced to best practice of the U.S.A.It finds that even assuming a 50% reduction in human capital mismatch between industries, it has little impact on total TFP. However, after considering the elimination of mismatch between human capital in industries and setting the level of human capital mismatch as the U.S. standard, the overall TFP has increased significantly. The results suggest that the impact of human capital mismatch between industries on TFP in China is relatively large.
Then we assume that the labour mismatch between industries is completely eliminated. The results of the Counterfactual productivity gains in different regions are shown in . The figure shows the difference between the actual allocated human capital efficiency and a counterfactual productivity outcome based on eliminating the skills mismatch between industries in different regions. Speculatively, shows that there is much room for improvement in productivity in the Northeast and Western regions. The results suggest that eliminating the labour mismatch between industries completely in the Northeast and Western regions could be associated with an increase in productivity of around 52.3% and 48.9%, respectively. Since 2013, the room for productivity improvement has increased year by year. Moreover, counterfactual productivity gains in the Eastern region are also relatively high, with an increase in productivity of 31.4%. Furthermore, counterfactual productivity gains from the full sample are up to 41%.
This means that China – a country with high industry mismatch and low allocative efficiency – could boost its level of productivity greatly if it were to reduce its level of mismatch within each industry to that corresponding to best practice of the U.S.A.
4.5. Mechanisms test
The channels under consideration include those that impede the upgrading of industrial structure, hinder technological innovation and reduce labour productivity. As we cannot provide any evidence that the adopted intermediate variables can directly affect productivity, we extend the theoretical description to indicate the cause-and-effect relationship between the industrial structure upgrading, technical innovations, labour productivity and total factor of productivity.
First, Li (2017) argues that the misallocation of human capital is strongly negatively associated with industrial structure upgrading. If misallocations of human capital prevent the improvement of the human capital level, then this will result in labour market distortion. Moreover, such impediments are not conducive to the adjustment of human capital structure to the direction of industrial transformation and hence lower productivity (TFP).
Secondly, it is obvious that the misallocation of human capital will cause skilled labour to deviate from innovation activities. Owing to low returns in productive activities, it will turn the innovative workforce into a non-scientific and innovative sector, resulting in the restriction of technological innovation (Murphy et al., Citation1991) and the reduction of productivity (TFP).
Thirdly, as noted by many researchers (Acemoglu, Citation1995; Hsieh et al., Citation2013; Murphy et al., 1993), the mismatch of human capital will create opportunities for the low allocation of human capital in the productive sector. A large number of workers choose to enter the non-productive sector, which will depress the compensation, reduce work enthusiasm and, hence, reduce labour productivity (Jovanovic, Citation2014). These factors combine to give rise to lower productivity (TFP).
The results of three mediating mechanisms tests are shown in . The three dependent variables are in log. Industrial structure upgrading is measured by where
is the proportion of the output value of the m industry to the total output value m = 1, 2, 3, indicating the first, second and third industries, respectively (Zhuo & Deng, 2018).The greater the Indus value, the higher the industrial structure level in the region. Technological innovation is measured by R&D input efficiency; labour productivity is measured by the output per unit of labour.
Table 8. A test of three mediating mechanisms.
To tackle these possible endogeneity problems, we have also chosen employment cultures as an instrument variable for misallocation of human capital. We have hence conducted a 2SLS test between all possible channels and productivity.
Columns (1) estimate the effect of misallocation of human capital on industrial structure upgrading. Industrial structure upgrading is measured by which shows that the coefficient of hmatch is strongly negative and significant at the 1% level, entailing that misallocation of human capital inhibits industrial structure upgrading. On average, a 1% increase in the mismatch is associated with a 0.23% decrease of industrial structure upgrading. This demonstrates that obstructing the upgrading of industrial structure is an important channel for misallocation of human capital, affecting productivity growth.
Column (2) shows the impact of the misallocation of human capital on technological innovation. Technological innovation is measured by R&D input efficiency, demonstrating that the coefficient of hmatch is strongly negative and significant at the 5% level. On average, a 1% increase in the misallocation of human capital is associated with a 0.52% decrease of technological innovation. This means that misallocation of human capital is restricting technological innovation and suggests that this s an important channel for misallocation of human capital, thereby affect productivity growth.
Column (3) shows the impact of misallocation of human capital on labour productivity, which is measured by the output per unit of labour. It shows that the coefficient of hmatch is strongly negative and significant at the 1% level. According to this finding, a 1% increase in the misallocation of human capital is associated with a 0.19% decrease of labour productivity. This means that misallocation of human capital reduces labour productivity and demonstrates that a reduction of labour productivity is an important channel where misallocation of human capital affects productivity growth.
As discussed above, our empirical results show that the channels through which misallocation of human capital affects productivity are industrial structure upgrading, technological innovation and labour productivity.
5. Discussion and conclusion
The mismatch between human capital and different industries is a great dilemma alongside economic transition. This study takes a first step toward estimating the impact of mismatch of human capital on productivity (TFP) by using data from a large China representative panel. As predicted, there is a negative association between mismatch of human capital and productivity. We have demonstrated that the negative association between mismatch of human capital and productivity is explained by industrial structure upgrading, technological innovation and labour productivity.
We have also found that the link between misallocation of human capital and productivity was present in almost every industry, but that the negative effect grows in magnitude among real estate industry, finance, public institution and monopolised industry. It shows that the negative effect of human capital mismatch on TFP has increased with the deepening of China’s reform to a significant degree.
Moreover, we consider how misallocation of human capital may influence productivity. It has been shown that the important channels through which misallocation of human capital affects productivity is industrial structure upgrading, technological innovation and labour productivity.
Furthermore, we performed a counterfactual experiment, the results of which suggest that eliminating the labour mismatch between industries completely in the Northeast and Western regions could be associated with an increase in productivity of around 52.3% and 48.9%, respectively. Also it shows that eliminating the labour mismatch between industries completely could be associated with an increase in productivity of around 41% for the whole sample in China.
We have found that misallocation of human capital is a vital factor affecting the productivity of Chinese firms. Attracting more human capital into productive sectors is an effective measure in spurring emerging economies' growth. Our findings are in line with the existing literature which suggests that occupational choice depends on returns rewarding ability and on compensation contracts (Hsieh et al., Citation2013; Murphy et al., Citation1991). These findings indicate that improving the allocation of human capital enables an increase in economic growth.
Our results suggest that proper allocation of human capital in different industries is playing a vital role in facilitating productivity. In the context of China’s economic downturn, these findings hence emphasise the importance of encouraging more human capital to work in high-tech enterprises rather than simply relying on government investment to stimulate the economy.
Our results have significant implications. For policymakers, the existence of these mechanisms raises the possibility that high productivity countries may be those that intrinsically have proper industrial structure upgrading, significant technological innovation and high labour productivity for the choice of occupation for human capital.
For researchers who study the allocation of human capital, the message of the paper is that the misallocation of human capital maybe the underlying cause of TFP decline in China. At present, too much labour is concentrated in Finance, the Government sector and the Monopoly industry and this hinders technological innovation, inhibits the improvement of labour productivity and thus reduces productivity.
6. Implication & future directions
6.1. Implications
The results suggest a number of business practice implications for policymakers. First, the Chinese government may need to attract more human capital into productive sectors by reducing the wage premium of non-productive sectors; for example, reducing rent-seeking opportunities and limiting the income of monopoly industries. Secondly, the findings indicate that the Chinese government should introduce incentives to allocate more human capital to innovative activities and the “big cakes” of productive activities to obtain sustainable development in the future.
Finally, our results shed light on the reasons behind China’s current economic downturn. Our findings hence provide a novel clue to solve the puzzle outlined here, namely, that correcting the current imperfections of incentives in the non-productive sectors and encouraging more human capital work in high-tech enterprises may be important measures in stimulating the development of emerging economies. The natural implication to consider when facing the key issues here is that policies that are directed at reallocating human resources or reducing human capital distortions can help to accomplish substantial welfare increases.
Also, our research has some implication that would stimulate the development of science. The important message of the paper is that, to a significant degree, with the deepening of China’s reform, the effect of human capital mismatch on TFP has increased over time and the misallocation of human capital has not been alleviated. This phenomenon deserves further research to assess its importance.
Moreover, this study indicates that misallocation of human capital between industries is the main source of the current decline of China's TFP. Past research has mainly focused on the misallocation of capital on TFP (Collard-Wexler, Asker, & De Loecker, Citation2011; Hsieh & Klenow, Citation2009; Jovanovic, Citation2014). Instead, this paper has supplied a new perspective on the role of the misallocation of human capital in economic productivity, providing quantitative estimates of the impact of misallocation of human capital on productivity (TFP). Our findings implicitly suggest that alleviation of misallocation of human capital is an effective measure for improving economic efficiency in China.
6.2. Limitations and future direction
It is important to admit two limitations to our study. First, mismatch of human capital is measured by in each year; hence, it is expected that measurement error will be far from trivial. The current difficulty is limited by data sources in China. It is vital to replicate the current findings with better proxy indicators. However, this is the best proxy we can consider at present, where further study could produce insights into this vital problem. Secondly, we have only examined industrial structure upgrading, technological innovation and labour productivity as channels through which misallocation of human capital affects productivity. There might be other possible channels that were not evaluated in this research. Despite these limitations, we believe that the selected study design and methods were appropriate for achieving the study’s goals and for making some important contributions.
Looking to the future, the following lines of research may be desirable. First, our research mainly focuses on the Chinese economy, but there is lack of formal evidence on comparisons with research in other emerging countries. Numerous emerging and transitional nations like Brazil, India and Russia have gone through fast economic development and drastic economic-systemic transformation, so a possible further research direction could be exploring how the impact of human capital misallocation on productivity may vary between different emerging countries. Also, making some comparisons with these countries is worth exploring. Secondly, as some sections contain elements of the theoretical model, the description of which is missing in the Literature review, we will consider the scope of empirical research and devote more attention to the development of premises for building a theory explaining the studied relationship in the future. Thirdly, there remain some challenging related issues that need to be solved, such as how does the misallocation of human capital affect income inequality or innovation? We will develop a new analytical framework to estimate the effects of the misallocation of human capital on income inequality or innovation. These two aspects may well provide new directions for future research. Last, the paper contains an exploratory study outlining the empirical framework the model ohas on productivity. This would allow us to outline the conceptual framework of such a model (even graphically: misallocation of human capital -> industrial structure, productivity innovations, labour productivity -> productivity) in the future.
Acknowledgments
First, the authors wish to express their thanks to Professor George W. Leeson, University of Oxford, for his valuable suggestions and comments on the earlier version of this paper. Also, we are grateful to anonymous reviewers and other Professors’ assistance of this manuscript.
Additional information
Funding
Notes
1 It should be clearly noted that we do not have a description of the theoretical model in the literature review; as far as we know, nobody has developed such a model so far, so the research designed is exploratory.
2 Though the construction of variable "Human_capital" is relative similar to "hmatch".But they are different. "Human_capital" is calculated as log of the proportion of the population with a college degree or above in the total population. but we use "hmatch" to measure the extent of human capital mismatch in each industry. The calculation formula is where
is real total labour force with bachelor degree or above for province i industry j each year.
is average labour force in all industries with Bachelor degree or above.
References
- Acemoglu, D. (1995). Reward structures and the allocation of talent. European Economic Review, 39(1), 17–33. doi: 10.1016/0014-2921(94)00014-Q
- Alfaro, L., Charlton, A., & Kanczuk, F. (2008). Plant-size distribution and cross-country income differences (Working Paper No. w14060). Cambridge: National Bureau of Economic Research.
- Bernardini, M., & Peersman, G. (2018). Private debt overhang and the government spending multiplier: Evidence for the United States. Journal of Applied Econometrics, 33(4), 485–508. doi: 10.1002/jae.2618
- Blundell, R., Costa Dias, M., Meghir, C., & Shaw, J. (2016). Female labor supply, human capital and welfare reform. Econometrica, 84(5), 1705–1753. doi: 10.3982/ECTA11576
- Bowen, W. G., & Finegan, T. A. (2015). The economics of labor force participation (Vol. 2054). Princeton University Press.
- Collard-Wexler, A., Asker, J., & De Loecker, J. (2011). Productivity volatility and the misallocation of resources in developing economies (Working Paper No. w17175). Cambridge: National Bureau of Economic Research.
- Dang, J., & Motohashi, K. (2015). Patent statistics: A good indicator for innovation in China? Patent subsidy program impacts on patent quality. China Economic Review, 35, 137–155.
- Ebeke, C., Omgba, L. D., & Laajaj, R. (2015). Oil, governance and the (mis)allocation of talent in developing countries. Journal of Development Economics, 114, 126–141. doi: 10.1016/j.jdeveco.2014.12.004
- Färe, R., & Grosskopf, S. (1996). Productivity and intermediate products: A frontier approach. Economics letters, 50(1), 65–70.
- Garnaut, R., & Johnston, L. (2016). China’s New Sources of Economic Growth: Vol. 1: Reform, Resources and Climate Change. China's New Sources of Economic Growth Vol. 2: Human Capital, Innovation, and Technological Change.
- Hanushek, E. A., Schwerdt, G., Woessmann, L., & Zhang, L. (2017). General education, vocational education, and labor-market outcomes over the lifecycle. Journal of Human Resources, 52(1), 48–87. doi: 10.3368/jhr.52.1.0415-7074R
- Hsieh, C. T., Hurst, E., Jones, C. I., & Klenow, P. J. (2013). The allocation of talent and us economic growth (Working Paper No. w18693). Cambridge: National Bureau of Economic Research.
- Hsieh, C. T., & Klenow, P. J. (2009). Misallocation and manufacturing TFP in China and India. Quarterly Journal of Economics, 124(4), 1403–1448. doi: 10.1162/qjec.2009.124.4.1403
- Jing, L. (2017). Human capital mismatch: The evolution of industrial comparative advantage and its interpretation. Journal of Statistics & Information, 32(10), 95–101.
- Jovanovic, B., & Petreski, M. (2014). Monetary policy, exchange rates and labor unions in see and the cis during the financial crisis. Economic Systems, 38(3), 309–332.
- Krüger, J. J. (2003). The global trends of total factor productivity: Evidence from the nonparametric Malmquist index approach. Oxford Economic Papers, 55(2), 265–286.
- Mahy, B., Rycx, F., & Vermeylen, G. (2015). Educational mismatch and firm productivity: Do skills, technology and uncertainty matter?. De Economist, 163(2), 233–262. doi: 10.1007/s10645-015-9251-2
- McGowan, M. A., & Andrews, D. (2015). Labor market mismatch and labor productivity: Evidence from PIAAC data (Working Paper No. 1206). OECD Economics Department.
- Midrigan, V., & Xu, D. Y. (2014). Finance and misallocation: Evidence from plant-level data. American Economic Review, 104(2), 422–458. doi: 10.1257/aer.104.2.422
- Murphy, K. M., Shleifer, A., & Vishny, R. W. (1991). The allocation of talent: Implications for growth. The Quarterly Journal of Economics, 106(2), 503–530. doi: 10.2307/2937945
- Olley, G. S., & Pakes, A. (1996). The dynamics of productivity in the telecommunications equipment industry. Econometrica, 64(6), 1263. doi: 10.2307/2171831
- Restuccia, D., & Rogerson, R. (2008). Policy distortions and aggregate productivity with heterogeneous establishments. Review of Economic Dynamics, 11(4), 707–720. doi: 10.1016/j.red.2008.05.002
- Romer, P. M. (1989). What determines the rate of growth and technological change? (Vol. 279). World Bank Publications.
- Sanders, M., & Weitzel, U. (2013). Misallocation of entrepreneurial talent in post-conflict environments. Journal of Conflict Resolution, 57(1), 41–64. doi: 10.1177/0022002712464852
- Sørensen, J. B. (2007). Bureaucracy and entrepreneurship: Workplace effects on entrepreneurial entry. Administrative Science Quarterly, 52(3), 387–412. doi: 10.2189/asqu.52.3.387