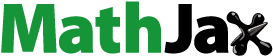
Abstract
In this study, we examine the determinants of social media adoption by local banks and its consequences. We use a unique dataset covering almost 600 local banks in Poland during the 2010–2016 period. This dataset contains information on the activity of local banks in social media, their financial performance and branch locations as well as the information on bank customers obtained from sociological surveys. We find the likelihood of social media adoption positively related to bank size and the technological and financial sophistication of clients. We show also that the impact of factors related to local competition is conditional on the performance of neighboring banks already present in social media. Finally, we establish that social media adoption helps local banks to defend their interest margins in the environment of low interest rates and intensifying competition. Therefore, our study suggests that social media can be used as a tool to develop a business model based on frequent interactions with clients and close relationships.
1. Introduction
Social media was one of the main driving forces of sociological as well as business changes during the last decade for two reasons. First, the number of social media users increased from 0.97 billion in 2010 to 2.46 billion in 2017 worldwide. This number is estimated to rise further to around 3.02 billion users by 2021 (eMarketer, Citation2017; We Are Social, 2018). Second, social media offered a wide range of previously unprecedented business opportunities. It provided companies with new means for strengthening long-term relationships (Durkin et al., Citation2014; Kaplan & Haenlein, Citation2010; Laroche et al., Citation2012; Lipsman et al., Citation2012; Mangold & Faulds, Citation2009), improving the efficiency of customer-oriented or relationship marketing (Cawsey & Rowley, Citation2016; Edelman, Citation2010; Gallaugher & Ransbotham, Citation2010; Pozza, Citation2014), increasing consumer engagement (Bolton, Citation2011; Tsimonis & Dimitriadis, Citation2014), stimulating brand awareness, loyalty, and trust (Farshid et al., Citation2011; Laroche et al., Citation2013; Larsson & Viitaoja, Citation2017; Silver & Vegholm, Citation2009), spreading positive word-of-mouth information (Gruen et al., Citation2006), facilitating knowledge sharing (Behringer & Sassenberg, Citation2015; Gandomi & Haider, Citation2015), and meeting heightened customer expectation of personalisation (BCG, Citation2018).
Despite the extraordinary growth in popularity of social media among potential customers and the tremendous business opportunities created therewith, not all industries and firm types showed equal vigor in social media-related activities. Local banks operating in Poland constitute an interesting example of an important business sector clearly lagging behind other financial intermediaries with respect to social media usage. For this reason, we intend to examine the difficult love story between local banks and social media. Polish local banks as a sector adopted social media only recently. In 2012, only 7.7% of local banks were using social media to communicate with clients, whereas all commercial banks with nationwide branch networks were active on social media. Moreover, local banks favored the use of Facebook and adopted other social media types sporadically. By the end of 2017, only 0.5% and 6.1% of local banks employed Twitter and YouTube, respectively. The slow pace of social media adoption by local banks seems surprising, because theoretically local banks applying a business model based on relationships, direct contact with clients, and soft information gathering should benefit significantly from social media use.
Our investigation has two goals. On the one hand, we analyse the factors determining local banks’ decisions to adopt social media. On the other hand, we investigate the impact of these decisions on the banks’ financial performance. To achieve our research goals, we amalgamate several data sources: a unique and dynamic dataset of local banks’ activity in social media, financial statements of local banks, the geo-location of bank branches, sociological surveys, official demographic and economic statistics, and information on local banks’ public issuance of debt instruments. To infer the determinants of social media adoption, we estimate panel logit models. To assess the impact of social media adoption on bank performance, we employ dynamic panel models and the GMM estimator. All models control for bank-specific factors, local economic and demographic situations, local banking competition, and macroeconomic tendencies.
As expected, logit panel model estimation reveals the likelihood of social media adoption increasing in accordance with the banks’ size of operations as well as clients’ financial and technical sophistication. For example, this probability is higher when more people in a local banking market served by a given bank use computers and Internet banking services. In contrast, the results pertaining to the role of competitive factors and forced imitation are partially surprising. The sheer presence of peers communicating with clients through social media in the vicinity of a given bank has a negative effect on the dependent variable in logit models. However, when we control for the profitability of social media-active local competitors, we find that only the presence of relatively poorly performing competitors discourages other local banks from engaging in social media. The presence of relatively well-performing social media-active peers influences the likelihood of social media adoption in the opposite direction. We explain these empirical patterns by the fact that a low relative profitability may be interpreted as a sign of poor-quality management. Consequently, if presumably poorly managed banks decide to adopt social media, this decision does not seem to be worth imitating; in contrast, when well-performing banks—which we assume are also well-managed—embrace social media, their example should more easily find followers.
The adoption of social media is found beneficial for local banks in our sample. In general, it helps local banks to defend their interest margins in an environment characterised by diminishing interest rates and rising competition. Dynamic panel model estimations show that social media-active local banks report higher interest income, net interest margins, and operating profitability. They also attract more deposits. These empirical patterns may be explained by the fact that social media enables local banks to strengthen their business models based on relationships and frequent contacts with clients. The observed regularities, particularly increasing interest margins, are also concordant with the view that local banks more successfully lock-in their customers in the existing relationships through social media use. Moreover, we obtain weak evidence that the positive effects of social media engagement take time to appear. Finally, we show that Facebook comments count more than ‘likes’ as predictors of local bank performance, and that having many comments will additionally improve local banks’ interest income and net interest margins.
These research outcomes are stable and withstand several robustness checks, and they are unlikely to be distorted by endogeneity and any reverse-causation phenomena. The results are not only insensitive to changes in econometric procedures and the application of alternative definitions of local banking markets, but also account for banks’ more aggressive, multi-channel social media strategies. Moreover, the relationship between Facebook’s presence and local banks’ performance only in the case of Facebook comments exhibits some weak signs of non-linearity.
The originality of our empirical analysis stems above all from the fact that, to the best of our knowledge, it constitutes the first attempt in the literature to investigate social media adoption by local banks. We are not aware of any works directly comparable to our study. Additionally our investigation contributes to the literature in three ways. First, unlike the vast majority of existing studies using static dataset, we use a dynamic dataset of banks’ social media activities. Thus, we can address crucial causality issues by applying appropriate lag structures. Moreover, our inferences use local banks’ objective, historical, and measurable information on social media adoption; therefore, we are not obliged to rely on survey data. Second, we concentrate on the financial drivers of social media adoption and its financial consequences. Consequently, this investigation complements the scarce evidence on financial aspects of social media adoption by banks. Extant studies mainly concentrate on the role of social media in bank relationship marketing (e.g. see Gallaugher & Ransbotham, Citation2010; Hinson et al., Citation2012; Mitic & Kapoulas, Citation2012; Wongsansukcharoen et al., Citation2015). Academicians adopting financial perspectives usually focus on specific issues such as influence of social media on the observability of depositor action (Kiss et al., Citation2014) and the impact of rating and verbalised emotions on bank performance (Tang et al., Citation2016). When we consider the scope of investigation, a recent work by Giaretta and Chesini (Citation2019) is the closest to our study. However, this work considers a different type of banks and analyses their experiences over a shorter time period. Third, our paper broadens our knowledge of small, local bank functioning. Despite their relatively modest scale of operations, these banks play an important economic role in numerous countries by alleviating the financial constraints of small and medium sized enterprises (SMEs; Berger et al., Citation2017; Berger & Udell, Citation2002; Hasan et al., Citation2017; Höwer, Citation2016; Petersen & Rajan, Citation1994).
The remainder of this paper is structured in five parts. Section 2 presents the institutional background of our investigation. Section 3 develops hypotheses on the local banks’ decision to adopt social media and the consequences thereof for bank performance. Section 4 describes our data sources and empirical strategy. Section 5 discusses the empirical results. Section 6 contains robustness checks while Section 7 concludes the paper and presents the managerial implications of our findings.
2. Institutional background—local banks and their social media activity in Poland
The Polish financial system is dominated by banks. Data from the National Bank of Poland show that bank assets accounted for approximately 73% of all financial institution assets in recent years (NBP, Citation2017). In 2017, the banking system comprised 616 institutions: 35 commercial banks, 553 cooperative banks, and 28 credit institution branches (PFSC, Citation2018). Cooperative banks are small, with very limited geographical reach, usually encompassing one or two counties. For this reason, we call these banks local banks in this paper. Local banks mainly perform basic financial intermediation functions, specialise in relationship lending and gathering of soft information thanks to their proximity with customers. Consequently, very few cooperative banks issue debt instruments. For example, during the period 2010–2017, the maximum number of local banks listed on the Catalyst market (the Polish bond market) was 22. Despite their large number, local banks hold only 7% of the banking sector assets. However, because of the specificity of their business model, local banks account for as much as 30% of all the bank branches and 20% of the banking staff (PFSC, Citation2018). They also play a disproportionally important role in alleviating the SME’s financial constraints (Hasan et al., Citation2017). Within local banking markets, local banks compete with around 20 large commercial banks having extensive nationwide branch networks.
The local banks’ interest income, contributing to 77% of the operating income in 2017, remains the most important driver of their profitability. However, the interest margins of local banks diminished substantially during the last few years (from 4.1% in 2010 to 2.9% in 2017), leading to deterioration of their ROA (from 1.1% in 2010 to only 0.5% in 2017) (PFSC, Citation2018). Although profitability is not the main goal of cooperative banks, profit is the main source of their capital increases necessary to expand activities and meet the rising regulatory requirements. Two factors contributed to the reduction of local banks’ interest margins: increasing competition in the local banking market, and declining interest rates in Poland, which currently stand at the historically lowest level (since 2010, the main interest rates of the central bank dropped by 50%).
Local banks serve customers mainly through branches, lagging significantly behind commercial banks in Internet and mobile banking implementation (Filip et al., Citation2017) as well as social media adoption. In 2010, 40% of commercial banks were active in social media, and 7 years later this share reached 90%. In contrast, local banks were almost totally lacking in social media in 2010. Even today, for every four local banks, only one is present in any social media.
Despite the enormous variety of social media, Facebook and YouTube remain the most popular platforms in Poland’s banking sector, as in other countries (EFMA, Citation2013; eMarketer, Citation2017; We Are Social, 2018). As regards Polish local banks, not only a small part of them use social media, but also they use a lesser number social media types. In 2010, all of the local banks active in social media were active only in one type of social media. Even 7 years later, in 2017, about 85% of them were still using only one social media type. Currently, some local banks are using two channels, but only two banks use three platforms. According to our data, Facebook remains the most popular platform for local banks. Almost 25% of them have tried to use Facebook from 2010 to 2017 (in 12 cases, the local bank’s presence was incidental and discontinued). The interest in Facebook has been growing steadily over the last 7 years. Local banks reveal very limited or marginal use of other types of social media. Given the local banks’ limited scope and frequency of using different types of social media and the requirements of statistical inference, we focus exclusively on the banks’ Facebook activities in this study.
3. Literature review and hypotheses development
We base our hypotheses on two strands of the literature. The first strand elucidates the reasons for local banks’ adoption of social media. The second strand considers the impact of social media on local bank performance.
3.1. Local bank adoption of social media
The financial literature on the determinants of banks’ social media adoption is modest. Therefore, to substantiate our hypotheses, we additionally employ earlier studies on the Internet and mobile banking adoption as well as social media adoption by non-financial entities. Three factors seem to play a primordial role in shaping the decision of banks on engaging in social media-related activities. These factors are competitive pressure, size of operations, and customer characteristics.
With regard to competitive considerations, Hernández-Murillo et al. (Citation2010) find that the adoption of online banking by US banks during the 2003–2006 period was faster in markets where their rivals were already active in the new distribution channels. Similarly, with regard to mobile banking adoption, Mullan et al. (Citation2017) underline the significant role of new technologies in maintaining market share and customer relationships. Moreover, external pressures are frequently highlighted as an important driver of non-financial firms’ electronic commerce or social media adoption (e.g. see Cao et al., Citation2018; Grandon & Pearson, Citation2004). As indicated in the previous section, Polish local banks compete with their peers as well as the large commercial banks having nationwide presence. The latter, as a sector, adopted social media earlier than the local banks. Therefore, considering the existing literature and the specificity of the Polish banking sector, we expect the local banks’ social media adoption to be positively related to the presence of commercial bank branches and branches of local banks already active in social media in the vicinity of local banks. We thus present our predictions in the following hypothesis:
H1: The local banks’ adoption of social media is positively related to local competition stemming from large commercial banks and other local banks already active in social media.
H1 assumes that local banks are forced to adopt new technologies and social media as a strategic response to competitive threats. However, as Paniagua and Sapena (Citation2014) underline, the utility of social media networks is fully exploited only when a critical mass of agents is reached. Considering the local banks’ limited size and scope of operations in Poland, they probably cannot capture the positive effects of social media engagements, particularly in the early implementation stages. The lack of quick and demonstrable successes of social media pioneers among local banks may deter neighboring banks from investing resources in creation of social media networks. Therefore, the negative varication of H1 is also possible.
Size of operation constitutes the second factor which should positively influence the decision to adopt social media. Empirical evidence substantiating this claim comes from both the early literature on online banking adoption as well as the literature on social media adoption by non-financial entities. For a sample of 1618 US commercial banks, Courchane et al. (Citation2002) find that the probability of investing in Internet banking was positively influenced by the banks’ market share, while Hernández-Murillo et al. (Citation2010) show that (in the sample described above) both the size and the fact of belonging to a holding company favored the decision to adopt online banking services. With regard to non-financial entities, Thong and Yap (Citation1995), Wamba and Carter (Citation2014), and Cao et al. (Citation2018) report that the size of operations is positively related to the probability of adopting information technologies or social media. Additionally, several studies indicate that the lack of knowledge (Bogea et al., Citation2018), shortage of qualified personnel (Michaelidou et al., Citation2011), insufficient internal financial resources (Dahnil et al., Citation2014) and difficulties in obtaining resources necessary to finance investments and costs related to social media adoption (Giaretta & Chesini, Citation2019) are important barriers to implementing social media. All these barriers should diminish in size. Consequently, we conjecture that relatively large local banks adopt social media faster. Thus, we expect a positive relationship between a local bank size and the probability of social media adoption and formulate the following hypothesis:
H2: The probability of social media adoption by local banks is positively related to the size of bank operations.
While we predict the positive verification of H2, the literature on non-financial firms suggests the possibility of a negative verification. For example, considering 45 wineries, Galati et al. (Citation2017) demonstrate that small firms are more involved in social media, as shown by high values of intensity, richness and responsiveness compared to other firms.
The demand for engagement in social media should also play a non-trivial role in banks’ decisions on their communication and distribution channels. The existing literature suggests that this demand is mainly shaped by customer or market characteristics. Courchane et al. (Citation2002) find that banks are more likely to offer online services in regions with a high percentage of college enrollees or elevated income per person. Szopiński (Citation2016) reports that the adoption of online Internet banking services in Poland is influenced by the use of Internet and other banking products, as well as trust in commercial banks. Durkin et al. (Citation2015) underline the relevance of customer age as a determinant of Facebook appropriateness for achieving banks’ goals. For non-financial firms, several authors claim that customers’ mass adoption of social media is an important factor for companies’ adoption-related decisions (e.g. see Bogea et al., Citation2018; Sinclaire & Vogus, Citation2011). Consequently, we expect a positive relationship between financial sophistication and social media adoption. To be more specific, we conjecture that the local banks operating in regions populated by clients who are relatively well familiarised with new technologies and online banking to be more eager to adopt social media. We thus state this prediction in the following hypothesis:
H3: Local banks serving customers familiarised with new technologies and online banking are more willing to adopt social media.
Hypotheses H1 to H3 are based on the existing literature. In contrast, our final hypothesis, which is related to the determinants of local banks’ social media adoption, originates from a specific trait of the Polish cooperative banking sector. As mentioned in Section 2, some cooperative banks issued publicly debt instruments during the sample period on the Catalyst market. As a result, a new group of stakeholders—the bondholders—became important for these banks, who for the first time were obliged to engage in investor relationship activities. We conjecture that banks with debt instruments listed on the Catalyst market have stronger incentives to adopt social media than other local banks. Our line of reasoning is supported by the recent work of Chauhan and Kumar (Citation2018), who find positive firm evaluation effects associated with non-financial disclosure in an emerging economy. Thus, we expect a positive relationship between bond listings and social media adoption and present the following hypothesis:
H4: Local banks with listed bonds have stronger incentives to adopt social media than their peers.
3.2. Impact of social media on local banks’ performance
Studies examining the impact of social media on banks’ financial performance are rare. Therefore, as in the previous subsection, we employ the Internet banking literature as well as the literature on the performance of non-financial companies to justify our hypotheses.
Previous studies generally agreed that banks benefit from the addition of online banking to the traditional bank distribution channels. DeYoung et al. (Citation2007) find that the described change led to nontrivial increases in profitability for US community banks. Furthermore, the Internet delivery channel had little effect on the composition of bank loan portfolios, but modified the structure of deposit base. Similarly, Ciciretti et al. (Citation2009) provide evidence of a positive relation between Internet adoption and bank profitability for Italian banks. They also establish that banks adopting Internet services diversify more their assets as they reach new industries and geographical markets.
Abundant literature on the influence of social media on non-financial firms’ performance underlines the prevalence of positive adoption effects. For example, Hong (Citation2012) shows that the adoption of social media by newspapers is positively associated with increasing readership. Kim, Koh, et al. (Citation2015) demonstrate that the social media activities of restaurants have a positive impact on firm value. Lam et al. (Citation2016) establish that social media initiatives improved the operational efficiency and innovativeness of firms in different industries. Drummond et al. (Citation2018) find that social media enabled entrepreneurial firms in the craft brewing and artisan food sectors to co-operate with network actors on new products and services and facilitated supply chain and logistical operations.
Since social media can help local banks to defend their market shares, reach new customers, and develop business models based on relationship lending and contacts with clients, and considering the reviewed empirical evidence, we expect social media implementation to have a positive impact on the performance of local banks.
H5: The adoption of social media contributes to the improvement of local banks’ performance.
The expected positive influence of social media on local banks’ performance may, however, be modified by two factors: time and customer engagement. We discuss the relevance of these two factors below. With regard to the time factor, the literature suggests that the effects of social media adoption might be delayed till the number of social media network participants is sufficiently high. Hernando and Nieto (Citation2007) establish that the impact of Internet banking adoption took time to appear in a sample of Spanish banks. This impact was significant for profitability three years after adoption. Moreover, as mentioned earlier, Paniagua and Sapena (Citation2014) show that the impact of social media on the stock performance of Spanish firms was positive only after the social media presence exceeded a certain threshold (180 to 240 thousand ‘likes’ for Facebook and 4.1 to 4.3 thousand followers on Twitter). Finally, the results reported by Malaquias et al. (Citation2018) suggest that in the case of new technologies adoption and its consequences important effects of time exist. For those reasons, we expect a positive link between a bank’s experience in social media and its financial performance. Consequently, we conjecture that the positive impact of social media adoption should be more pronounced for the so-called early adopters. Hypothesis H6 reflects this prediction.
H6: The positive relationship between social media adoption and local banks’ performance strengthens in time.
Finally, the social media literature indicates that customer engagement and sentiment count for firm performance. Tang et al. (Citation2016) analyse the relationship between electronic word-of-mouth and firm profitability for a sample of 68 US banks. They find that both star ratings and verbalised emotions (especially negative ones) are good predictors of bank performance. Similar phenomena were observed for other industries. Kim, Lim, et al. (Citation2015) provide evidence that overall rating is the most salient predictor of hotel performance. Hsu and Lawrence (Citation2016) demonstrate that the volume, valence, and growth of online word-of-mouth exacerbated the negative effects of product recall announcements on firm values. Oh et al. (Citation2017) show that consumer engagement behavior correlated positively with first weekend movie box-office gross revenue. Thus, we expect a positive relationship between, on the one hand, customer satisfaction and engagement and, on the other hand, the impact of social media adoption on local bank performance. We express this prediction in Hypothesis H7.
H7: Customer satisfaction and engagement stimulate the positive relationship between social media adoption and local bank performance.
4. Data and methodology
4.1. Data
In our study we employed six data sources. First, we hand-collected detailed information about the Facebook activity of 558 local banks in Poland during the 2010–2016Footnote1 period. More specifically, we gathered data on the number of posts published yearly by each bank, and the number of Facebook ‘likes’ and comments. This dataset enabled us to construct five variables linked to the social media activity of banks. A binary variable FB.ACTIVE encodes the years in which a bank was active in Facebook, that is, publishing Facebook posts. FB.POSTS represents the number of a bank’s Facebook posts published in a given year. FB.YEARS is calculated as a year number since the first published post on Facebook, and is a proxy for the bank’s Facebook experience. Finally, FB.LIKES and FB.COMMENTS denote the average number of ‘likes’ and comments, respectively, recorded in a given year on a post of the bank.
Second, we gathered financial statements of the analysed local banks for the period 2009–2015. We then used this dataset to construct numerous bank-level variables reflecting the banks’ specificity, and then employ them in regression analysis to explain the determinants of Facebook adoption or the effects of using Facebook. We also construct variables describing a bank’s size (measured using a natural logarithm of the bank’s assets, its second power, or the natural logarithm of the number of the bank’s branches, that is, LN.A, SQR.LN.A, and LN.BRANCHES, respectively), loans-to-assets ratio (LOANS), equity-to-assets ratio (EQUITY), income structure (NII.SHARE), operating profitability (PROFIT), cost control (OVERHEADS), net interest margin (NIM), interest income on loans (INT.INC), interest cost on deposits (INT.COST), and growth rate of loans or deposits (LOAN.GR and DEPO.GR, respectively).
Third, we enriched our bank-level variables dataset with information from the Catalyst market on the publicly listed bonds of local banks. Thus, we construct a BOND.ISSUANCE binary variable that takes the value of 1 when the bank’s bonds are publicly listed in a given year, and 0 otherwise. This variable allows us to identify the banks engaged in investor relationship management activities and thus more likely to be active in Facebook.
The fourth data source of this study comprises the addresses of all bank branches in PolandFootnote2 (local banks as well as their commercial competitors having branches nationwide) between 2009 and 2015. We geo-located all the branches and utilised this information to reflect the Facebook activity of a bank’s neighboring competitors. As almost all commercial banks were active in Facebook during the analysed period, the presence of commercial bank branches in a given local bank’s neighborhood could represent a direct incentive to adopt social media. Consequently, for each local bank branch, we calculated the share of the commercial bank branches out of all bank branches operating within a radius of 5 km.Footnote3 By averaging this indicator over all the branches of a given local bank, we obtained the variable COMM.BANKS.AROUND, which directly describes the presence of commercial banks around a given local bank. In this manner, we constructed two variables reflecting the presence of Facebook-active local peer banks and their branches around a given local bank’s branches (FB.AROUND.BANKS and FB.AROUND.BRANCHES). Finally, our variables FB.AROUND.PROFIT.BANKS and FB.AROUND.PROFIT.BRANCHES describe the presence of highly profitable Facebook-active peer local banks and their branches within a radius of 5 km from a given local bank.Footnote4
Our fifth dataset describes the familiarity of people with new technologies and online banking in the local banks’ neighborhood. We employed data from the Social Diagnosis, that is, a periodic survey of approximately 35 thousand individuals from approximately 12 thousand Polish households. As the survey is carried out every two years, we used the data from the 2009, 2011, 2013, and 2015 survey rounds, assuming that each survey round’s results are valid also for the preceding year. Using the Social Diagnosis data, we can calculate the share of the population (aged 18 or more) using computers, the Internet, banking services, and e-banking services at the county level. By combining this county-level information with the data of each local bank’s branch locations, we can describe the bank’s potential clients by averaging these indicators over the counties covered by a given bank, whereas the number of the bank’s branches in individual counties is used as a weight. Thus, we obtain the variables COMPUTER.USE, INTERNET.USE, BANK.USE, and INTERNET.BANK.USE.
Finally, we employ the county-level information provided by the Polish Central Statistical Office (the sixth data source) to describe the situation in all the 380 Polish counties. We measure the unemployment rate, salary level, and population density at the county level, and then translate these county-level indicators into our bank-level variables UNEMPL, SALARIES, and POPUL by calculating for each bank the average value weighted with the number of a bank’s branches in individual counties. The set of measures describing a bank’s local environment is then finally augmented with the BANKS variable illustrating the number of competitors in a local banking market. Although this variable is transformed from the county level to the bank level aggregation in the same manner as UNEMPL, SALARIES and POPUL, it is based not on the data provided by the Polish Central Statistical Office, but on our already-mentioned fourth data source comprising the addresses of all bank branches in Poland.
details all the employed research variables, while provides descriptive statistics based on the number of observations utilised in the study’s first estimated specification. reveals that approximately 10% of our observations concern Facebook-active banks with a maximum seven years’ social media experience. While the average number of posts a bank publishes in a year seems relatively limited (5.605), it should be noted that this average is approximately 10 times greater for only Facebook-active banks. Finally, Facebook users seem to primarily respond to banks’ Facebook activity by granting ‘likes’, as the average number of ‘likes’ per each post a bank publishes is approximately 15 times greater than the number of comments received.
Table 1. Variable definitions.
Table 2. Descriptive statistics.
4.2. Methodology
To test hypotheses H1 to H4, we apply panel logit models with robust standard errors to our bank-year data. The models explains probability that a given local bank starts Facebook activity in a given year. EquationEquation (1)(1)
(1) illustrates the general construction of our logit panel models:
(1)
(1)
In all specifications, we control for the bank specificity (LN.A, EQUITY, LOANS, NII.SHARE, PROFIT, and LOANS.GR), local environment (UNEMPL, SALARIES, and POPUL) and macroeconomic conditions (year dummies). In addition, by employing different sets of supplementary variables, we test our hypotheses individually. First, to verify H1, we use regressors describing the Facebook activity of neighboring banksFootnote5 (COMM.BANKS.AROUND, FB.AROUND.BANKS, FB.AROUND.PROFIT.BANKS, FB.AROUND.BRANCHES, and FB.AROUND.PROFIT.BRANCHES). Second, in order to test the veracity of H2, we focus on the coefficient of LN.A, use both LN.A and its square (SQR.LN.A) to investigate the potential nonlinearities, or replace LN.A with an alternative measure of the bank’s size, that is, LN.BRANCHES. Third, with regard to H3, we introduce regressors reflecting the familiarity of people with new technologies (COMPUTER.USE, INTERNET.USE, BANK.USE, and INTERNET.BANK.USE). Fourth, to verify H4, we apply the BOND.ISSUANCE variable. As the variables used to verify each hypothesis are characterised by different sets of observations with missing values, we estimate separate models for each hypothesis, that is, with all control variables and an additional set of variables related specifically to the currently tested hypothesis. This procedure reduces the drop in the sample size due to missing observations. Nevertheless, as a kind of a robustness check, we finally estimate horse race type models in which we simultaneously employ regressors from all the variable groups in EquationEquation (1)(1)
(1) . In this manner, we check whether all the identified factors independently influence the Facebook activity of local banks.
To verify hypotheses H5 to H7, we apply a different technique, that is, dynamic panel regression models which are estimated using the GMM-SYS procedure proposed by Blundell and Bond (Citation1998). This procedure allows for including lagged dependent variables into the set of regressors. By applying this method and treating the lagged dependent variables as just sequentially exogenous explanatory variables, we control for the potential persistence in bank performance. EquationEquation (2)(2)
(2) gives the general construction of our dynamic panel models used to explain the bank performance indicators:
(2)
(2)
As dependent variable, we choose six performance indicators related to the core activities of local banks. These variables reflect a bank’s loans and deposit growth rates (LOANS.GR and DEPO.GR, respectively), net interest margin (NIM), interest income on loans (INT.INC), interest expenses on deposits (INT.DEPO), and operational return on assets (PROFIT). In all specifications, we include the lagged values of the dependent variables and control for bank specificity (LOANS, EQUITY, OVERHEADS, LN.A, and NII.SHARE in regressions for LOANS.GR, DEPO.GR and PROFIT), local environment (UNEMPL, SALARIES, POPUL and BANKS), and macroeconomic situation (year dummies). Then, to test the veracity of hypotheses H5 to H7, we use different variables explaining a bank’s Facebook activity and its customer’s Facebook response. First, to verify H5, we apply FB.ACTIVE or FB.POSTS as additional regressors. In case of H6, we use FB.YEARS apart from FB.ACTIVE. Finally, with regard to H7, the variables FB.LIKES or FB.COMMENTS supplement the baseline FB.ACTIVE variable. We expect a bank’s Facebook activity to have a relatively quick impact on its loan or deposit growth rates. Therefore, in specifications explaining these two performance indicators, we introduce unlagged regressors describing a bank’s Facebook activity. In contrast, the impact on margins and profits takes time to occur. Consequently, the regressors of Facebook activity are lagged by one period for the remaining specifications.
5. Results
In this section, we present the empirical results related to our hypotheses. To preserve presentational simplicity, we address the hypotheses on adoption of social media (H1–H4) in the following subsection (5.1), and the hypotheses on the impact of social media adoption on local bank performance (H5–H7) in subsection 5.2.
5.1. Adoption of social media
tests hypothesis H1 and investigates the determinants of local bank Facebook activities in the context of the Facebook activity of the neighboring banks. In separate specifications of Panel A, we investigate the impact of different types of banks. First, Specification (1) observes that the vicinity of commercial banks (COMM.BANKS.AROUND), which are generally active in social media, does not influence a local bank’s Facebook activity in a statistically significant manner. This result is not surprising when we consider the differences in business models, served clients, and areas of operation between these types of banks. Consequently, local banks exhibit limited willingness to follow the paths of their commercial social media-active counterparts operating in the same neighborhood. Second, Specifications (2) and (4) suggest that the vicinity of Facebook-active local banks (FB.AROUND.BANKS and FB.AROUND.BRANCHES) surprisingly discourages a cooperative bank to a certain extent from adopting Facebook as a channel for communication with its clients. However, as Specifications (3) and (5) indicate, the research outcomes related to H1 change after controlling for the vicinity of Facebook-active peer banks with relatively high as well as low profitability. That is, when we introduce variables reflecting the presence of good Facebook-active local banks in the neighborhood (FB.AROUND.PROFIT.BANKS and FB.AROUND.PROFIT.BRANCHES) and augment the estimation results from Panel A with tests for the coefficients’ sums from Panel B, we are able to refine our interpretations. On the one hand, when a local bank has only Facebook-active peers reporting relatively low profitability in its vicinity, the bank treats social media activity as a kind of unjustified eccentricity and refrains from adopting it (hence, the negative and statistically significant coefficients for FB.AROUND.BANKS and FB.AROUND.BRANCHES). On the other hand, when the bank is surrounded by relatively profitable Facebook-active peer local banks, the peers’ success positively influences the probability of the bank’s Facebook adoption (hence, the positive and statistically significant coefficients for FB.AROUND.PROFIT.BANKS and FB.AROUND.PROFIT.BRANCHES). However, the test results for the coefficients’ sums show that the positive impact of highly profitable Facebook-active neighboring peers is not enough to overcome the local banks’ general mistrust of social media. Thus, the impact of local competition-related factors is conditional on the performance of the neighboring banks already present in social media. Having Facebook-active peers with low profitability in the vicinity reduces the probability of a bank’s Facebook engagement. However, having highly profitable Facebook-active local banks in the neighborhood acts in the opposite direction, but it is still insufficient to overcome the general suspicion of social media as a tool to communicate with clients. Therefore, we fail to find evidence supporting H1.
Table 3. Determinants of local banks’ Facebook presence: Impact of neighboring banks’ Facebook presence.
The remaining regressors in Specifications (1) to (5) impact the dependent variable in the expected directions. As regards the bank-level control variables, four regressors influence Facebook adoption in a stable and statistically significant manner, with p-values below 1%. We establish that greater and more profitable banks (LN.AFootnote6 and PROFIT) with faster-growing loan portfolios (LOANS.GR) but riskier funding structure (EQUITY) are more eager to adopt Facebook as a communication channel in the following periods. In other words, more dynamic, aggressive, and competitive banks are more inclined to look for new communication technologies. Further, two out of three variables describing a bank’s local environment influence the probability of a bank’s Facebook activity at a significance level of at least 5%. First, an increase in unemployment rate in the county of a bank (UNEMPL) unsurprisingly reduces the bank’s willingness to use Facebook, as higher unemployment usually corresponds to greater poverty and customers’ worse access to new communication channels. Second, banks mostly adopt Facebook to operate in less densely populated areas (POPUL). This outcome implies that local cooperative banks are more eager to invest in social media in their strongholds, that is, in relatively smaller communities. As the results generally hold for the control variables in all specifications explaining the determinants of Facebook adoption, we do not present them in and . The full research outcomes are available upon request from the authors.
Table 4. Determinants of local banks’ Facebook presence: impact of other factors.
Table 5. Determinants of local banks’ Facebook presence: horse race models.
verifies the remaining hypotheses related to the determinants of social media adoption, that is, H2 to H4. First, Specifications (1) and (2) complement our results in , which shows that larger banks are more willing to initiate Facebook activity. While Specification (2) indicates that the outcomes hold for an alternative measure of a bank’s size (LN.BRANCHES), Specification (1) additionally suggests that the relation does not take a non-linear form and is not extraordinarily strong for the biggest local banks. We explain those finding by the fact that insufficient resources and limited abilities to mobilise them constitute important barriers to implementing information technologies or social media. Importantly, those barriers diminish in size of banks’ operations (Cao et al., Citation2018; Courchane et al., Citation2002; Giaretta & Chesini, Citation2019; Hernández-Murillo et al., Citation2010; Thong & Yap, Citation1995; Wamba & Carter, Citation2014). Second, Specifications (3) to (6) consistently show that the local banks’ decisions to engage in social media are shaped by customer characteristics. Local banks are more likely to initiate Facebook activity when they operate in areas populated by customers frequently using computers (COMPUTER.USE), the Internet (INTERNET.USE), banking services (BANK.USE) and, particularly, e-banking services (INTERNET.BANK.USE). The respective coefficients are statistically significant at least at the 10% level. Therefore, we positively verify H3. Third, in line with H4, Specification (7) show that Facebook activity is positively related to the issuance of bonds (BOND.ISSUANCE) which forces a local bank to increase its investor relationship management activities. The relevant coefficient is statistically significant at the 1% level.
Finally, we present our estimation results of the horse race-type models in , introducing simultaneously the regressors previously used individually to test hypotheses H1 to H4. The results of this exercise demonstrate that all the regressors influence probability of local banks’ social media engagement independently, since the respective coefficients are still statistically significant at least at the 5% level. Thus, the probability of a local bank’s Facebook activity is driven by the local competitors’ social media use and their profitability (FB.AROUND.BANKS and FB.AROUND.PROFIT.BANKS), bank size (LN.A), customers’ familiarity with new technologies (INTERNET.BANK.USE), and the bank’s investor relationship management needs linked to public bond offerings (BOND.ISSUANCE).
5.2. Social media impact on performance
In , we verify H5 and check how Facebook activity (FB.ACTIVE) impacts a local bank’s performance reflected in different financial indicators linked to their core activities. As we assume that social media activity has a direct and quick impact on loans and deposits and relatively delayed effect on margins, interest income, interest costs, and profitability, we introduce the FB.ACTIVE variable without lags in Specifications (1) and (2), and with a one-year lag in Specifications (3) to (6). Our research outcomes suggest that Facebook-active banks can attract more deposits at the same price, since the coefficient for the variable FB.ACTIVE is positive and statistically significant at the 5% level in Specification (2), whereas the relevant coefficient is statistically insignificant in the specification explaining the level of interest costs. Moreover, although Facebook activity does not directly contribute to increased lending (Specification 1), it significantly improves the interest income on loans (Specification 4) and finally boosts the net interest margin (Specification 3) and a bank’s operational profitability (Specification 6). In sum, we find rather strong evidence in favor of H5, since social media activity contributes to improved local bank performance. The results are statistically significant as well as economically relevant. For example, Facebook activity (FB.ACTIVE) translates into a 1.68 percentage point higher deposits growth (DEPO.GR), 0.156 percentage point increase in interest income on loans (INT.INC), and 0.072 percentage point increase in operating return on assets (PROFIT), which are equivalent to 16.0%, 5.7% and 4.5% of the sample interquartile ranges of the respective financial indicators.
Table 6. Facebook presence vs. local banks’ performance.
The positive influence of social media on the performance of local banks can be explained in two ways. First, it is possible that social media enable local banks to develop, strengthen and refine their business model based on relationship lending and frequent contacts with clients. Second, social media may help local banks to more efficiently lock-in customers in the existing relationships. It may facilitate the acquisition of soft information and increase the advantage of local banks over other potential lenders, creating a form of information monopoly (Ongena & Smith, Citation2001; Prilmeier, Citation2017; Rajan, Citation1992). Unfortunately, from our data, we cannot differentiate between these two explanations. However, the fact that the Facebook activity of local banks is positively related to the interest income, but not correlated with loans growth, indirectly supports the second explanation based on the deepening of information asymmetry.
The control variables in generally influence the dependent variables in an expected manner. First, a bank’s performance is partly a repercussion of its asset and funding structure (LOANS and EQUITY). Second, larger banks (LN.A) grow faster. Third, local banks usually perform better in their strongholds, that is, in relatively poorer areas (UNEMPL and SALARIES). Fourth, banks with increased overheads (OVERHEADS) report lower interest costs on deposits, higher net interest margin, and higher operating profitability, which may be due to either their bigger and more efficient marketing expenditures or the effects of employing staff with higher qualification. The results for the control variables do not change in further specifications related to the impact of social media activity on bank performance. Therefore, for the sake of brevity, we present only the research outcomes directly related to hypotheses testing in . The full results, however, are available upon request from the authors.
Table 7. Number of published Facebook posts vs. local banks’ performance.
Table 8. Experience in Facebook activity vs. local banks’ performance.
Table 9. Received Facebook ‘likes’ and comments vs. local banks’ performance.
To check the robustness of our key results concerning the positive impact social media on local bank performance, we use the number of published Facebook posts (FB.POSTS), instead of the binary variable encoding the local banks active in Facebook (FB.ACTIVE), in . Research outcomes almost ideally match our findings shown in and further support H5. Local banks with more published posts attract more deposits, report higher interest income, and as a result generate higher net interest margin and operating profits. In addition, Specification (1) provides evidence that posts contribute to the increase in loan growth, but the relevant coefficient is statistically significant at the 10% level only.
Social media adoption effects might appear only when the number of social media network participants becomes sufficiently high. Therefore, checks whether the positive relationship between social media adoption and local bank performance strengthens in time. The evidence in favor of H6 is relatively weak. That is, we establish that banks with longer experience in Facebook activity (FB.YEARS) generate higher net interest margin and operating profits (the respective coefficients are statistically significant at the 1% level), but do not differ from other banks in terms of loan and deposit growth rates, interest income on loans, and interest cost on deposits. Nevertheless, both statistically significant outcomes in are relevant in economic terms. To be more precise, one additional year of Facebook activity increases the net interest margin by 0.033 percentage points and operating return on assets by 0.046 percentage points, or 2.8% and 2.9% of the sample interquartile ranges of the respective financial ratios, respectively.
Finally, the positive impact of social media adoption on local bank performance may be stimulated by customer satisfaction and engagement, as H7 posits. We address this phenomenon in . Two panels regress the bank performance measures against mechanical manifestation of customers’ positive sentiments (‘likes’ per post, a FB. LIKES variable in Panel A) or a proxy for client engagement (comments per post, a FB.COMMENTS in Panel B). The research outcomes from both panels complement each other. Since it is relatively easy to gather customer ‘likes’, as they are just simple mouse clicks and do not ideally reflect the customers’ interest in the bank’s Facebook profile, we fail to find the number of received ‘likes’ stimulating a local bank’s performance. In contrast, if a bank can engage customers in discussions and receive their comments, customer engagement would improve the local banks’ performance. That is, Specifications (9) and (10) indicate that the number of Facebook comments per published post stimulates a bank’s interest income on loans and its net interest margin. Our research outcomes are economically relevant. One received comment per published post is expected to increase interest income on loans by 0.269 percentage points and the net interest margin by 0.184 percentage points, or 15.7% and 9.8% of the sample interquartile ranges of the respective dependent variables, respectively. Thus, the empirical findings in partially support H7.
6. Robustness checks
This section presents the design and results of our robustness checks. We first address fundamental questions involving potential endogeneity and reverse causation issues. Next, we focus on alternative definitions of local banking markets and the simultaneous use of different types of social media. Finally, we verify whether Facebook ‘likes’ and comments have non-linear impacts on banks’ performance. None of those robustness checks undermine previous findings and interpretations. For brevity, the robustness results are not tabulated here, but are available from the authors upon request.
In the previous section, we concluded that social media activities improve local banks’ performance; however, endogeneity and reverse causation scenarios exist. In our case, it is theoretically conceivable that well-performing banks care more about customer satisfaction and value, and consequently, are more likely to use social media. We performed two additional robustness checks to determine whether our conclusions are unaffected by endogeneity and reverse causation. First, we employed the difference-in-differences (DID) method. Specifically, we analysed the average financial ratios for two years before (the pre-treatment period) and after (the post-treatment period) social media implementation for treatment and control groups of local banks. The control group was randomly drawn from a sub-sample of banks that never opted for social media use. For each bank belonging to the treatment group, we separately assigned a control bank to assure their financial outcomes’ comparability. Generally, applying the DID method supports our previous findings, in that local banks’ social media activities positively influence their performance. Moreover, and as noted in Section 5, local banks’ benefits appear above all in the sphere of traditional financial intermediation. Facebook-active local banks enjoy higher net interest margins, lower interest costs, and consequently, higher returns on assets. Second, our regression models—which tested for social media adoption’s impact on local banks’ performance—treated the FB.ACTIVE variable as only sequentially exogenous. This methodological alteration did not change our conclusions regarding social media adoption’s influence on local banks’ performance. The coefficients obtained for the FB.ACTIVE variable are still positive and statistically significant in specifications explaining deposit growth, net interest income, interest income, and operational returns on assets. The only difference is that the relevant coefficients always differ from zero at the 1% level in this robustness check, while in they are significant at slightly lesser levels. Consequently, both additional robustness checks do not support the conjecture that the positive relationship between social media adoption and local banks’ performance found in our study is driven by the fact that well-performing banks are also the first to embrace social media; in contrast, they suggest that social media adoption improves banks’ performance in selected fields within their operations.
Our baseline regressions applied the 5 km radius to delimit a local banking market. We determined whether our results are sensitive to changes in the method applied to define a local banking market by re-estimating our regressions using counties’ administrative borders. While the sheer presence of Facebook-active peers still discourages from embracing social media, we obtain stronger evidence than in previous works that the closeness of well-performing banks already present in social media affects social media adoption-related decisions in the opposite direction.
When we determined social media adoption’s influence on local banks’ performance, we focused on Facebook activities, as only a few banks had a presence in other types of social media. However, it is possible from a theoretical perspective that more aggressive strategies involving local banks’ presence in two or more types of social media can affect these banks performance differently than in . Therefore, we re-estimated the equations from with two new binary variables. The first variable identifies the local banks active in any kind of social media, while the second encodes local banks present in more than one type of social media. As anticipated, and because our sample considers Facebook’s dominance, the estimation results indicate that the consequences of Facebook adoption are almost identical to those relating to the adoption of any kind of social media. In both cases, local banks active in social media report quicker deposit growth rates and higher profitability ratios. A more aggressive strategy to embrace social media creates some additional benefits to local banks, but only in terms of their levels of interest income.
Section 5 revealed only limited support for H7, stipulating that customers’ satisfaction and engagement increases banks’ benefits in adopting social media. One potential reason for our inconclusive results may be, on the one hand, the non-linear relationship between Facebook ‘likes’ and comments, and on the other hand, local banks’ performance. We check for this possibility by constructing binary variables that identify 25% and 33% of local banks with the highest numbers of ‘likes’ or comments received in a given year, respectively. We also employed binary variables to encode 25% and 33% of cooperative banks with the highest numbers of ‘likes’ or comments during the entire sample span, respectively. Regressions with variables related to Facebook ‘likes’ demonstrate that even a high number of ‘likes’ does not directly contribute to improving a bank’s performance. In contrast, the results concerning Facebook comments suggest that a high number of Facebook comments increases the bank’s interest income on loans and their net interest margin.
7. Conclusions and implications
In this study, we examined the decisions of local banks to enter the world of social media and the consequences of those decisions. To test our hypotheses, we combined six information sources: dynamic panel data on local banks’ social media activity, financial statements of local banks, bank branch locations data, local bank bond issuance data, official economic statistics, and sociological survey results. Thus, we could test the significance of a wide range of social media activity determinants, control for other factors potentially affecting the studied phenomena, and address the issue of causality between social media involvement and performance. The estimation of panel logit and dynamic panel GMM-style models revealed several interesting empirical patterns.
With regard to social media adoption decisions, we verified hypotheses H2 to H4 positively. We establish that the probability of social media engagement increases in accordance with the size of operations as well as Internet and financial sophistication of local customers. This likelihood is also higher for local banks issuing bonds, and therefore obliged to develop investor relationship activities. The results concerning the competition-related drivers of social media adoption contradicted H1. In contrast to previous works (e.g. Cao et al., Citation2018; Hernández-Murillo et al., Citation2010), we fail to find any evidence of simple mimetism or forced imitation. It turns out that the role of neighboring local banks’ example of engagement in social media is conditional on the financial performance of these banks. More precisely, the presence of relatively badly performing social media active local banks within a local banking market deters the local banks from adopting social media, while prosperous local banks’ proximity affects their decisions to adopt social media in the opposite direction. Interestingly, the competitive pressure from large commercial banks having nationwide branch networks (as a sector, these were the early adopters of social media) seems to be irrelevant to the decisions of local banks to engage in social media. The last empirical pattern is most probably due to the difference between local banks and the large commercial banks in business models and the customer segments or markets they focus on.
Once adopted, as predicted by H5, social media help local banks to successfully operate in difficult economic environments with increasing competition, low interest rates, and diminishing interest margins. Banks active in social media report higher deposit growth ratios, interest income on loans, interest margins, and operational profitability. The positive impact of social media on local bank performance is statistically significant as well as economically relevant. As discussed in Section 5, two mechanisms may explain the improved performance of local banks active in social media: the development of a business model based on close relationships, and the strengthening of lock-in phenomenon due to increased information asymmetry between relationship lenders and other potential capital providers (Prilmeier, Citation2017; Rajan, Citation1992). The lack of a stable and positive impact of social media activity on loan dynamics, we believe, slightly privileges the second explanation. In line with Hernando and Nieto (Citation2007), we find that the effects of new technologies and communication channels take some time to appear. However, our evidence in favor of H6 is weak because it regards only profitability measures. Finally, we establish that mechanically awarded Facebook ‘likes’ count much less as predictors of bank performance than customer and general public comments. It is noteworthy that the relationship between Facebook comments and local banks’ performance exhibit some signs of non-linearity. Consequently, our study only partially supports the predictions in H7, and supplements previous findings by Tang et al. (Citation2016) regarding the impacts of ratings and verbalised emotions on bank outcomes.
This study is based on the Polish experience, but our findings have a broader appeal because local banks play an important economic role in numerous European and non-European countries. First, from a managerial perspective, this investigation suggests that social media can be used as a tool to develop a business model based on frequent interactions with clients and close relationships. This business model, in conjunction with flat organisational structures, helps local banks to excel in soft information processing and gathering (Boot, Citation2000; Stein, Citation2002) as well as in SME financing (Berger et al., Citation2017; Berger & Udell, Citation1995; Hasan et al. Citation2017; Höwer, Citation2016). Therefore, local banks should not mechanically follow patterns established by large banks using social media. Instead, social media adoption strategies of local banks should be oriented toward strengthening their natural competitive advantages. Second, social media can also help local banks to deepen the information asymmetry between themselves and non-relationship lenders, and, as a consequence, exploit the benefits of the lock-in phenomenon (Prilmeier, Citation2017). The local banks in Poland that adopted social media were able to defend more successfully their interest margins and operational profitability than their non-adopting peers in a difficult environment of low interest rates and increasing competition. Therefore, for the sample banks, as underlined by Drummond et al. (Citation2018), social media has become more than a tool, turning almost into a company resource.
Disclosure statement
No potential conflict of interest was reported by the authors.
Additional information
Funding
Notes
1 Our sample covers 97% of the market, with 576 cooperative banks operating in at least one year from 2010 to 2016.
2 Data provided by an independent consulting company Inteliace Research.
3 The results remain unchanged when we alternatively use 2.5 km or 7.5 km radius to circumvent local banking markets. It is noteworthy that other published banking studies have also used a geo-location-based approach to determine local banking markets (e.g. Beck et al., Citation2018).
4 We define a local bank with high profit as a bank with PROFIT above the country-year median for all local banks.
5 Our baseline regressions do not weigh observations for neighboring peer banks. However, the research outcomes remain unchanged when we weigh observations using local peer banks’ assets. The relevant estimation results are available from the authors upon request.
6 While verifying H1 in Table 3, we treat LN.A as a control variable. Nevertheless, from the perspective of H2, the LN.A variable is used for hypothesis testing. Thus, we refer to the positive coefficient for LN.A once again when discussing the results presented in Table 4.
References
- Arellano, M., & Bond, S. (1991). Some Tests of Specification for Panel Data: Monte Carlo Evidence and an Application to Employment Equations. The Review of Economic Studies, 58(2), 277–297. https://doi.org/10.2307/2297968
- BCG. (2018). The global retail banking digital marketing: The power of personalization. http://image-src.bcg.com/Images/BCG-The-Power-of-Personalization-May-2018_tcm20-193016.pdf
- Beck, T., Degryse, H., De Haas, R., & Van Horen, N. (2018). When arm’s length is too far: Relationship banking over the business cycle. Journal of Financial Economics, 127(1), 174–196. https://doi.org/10.1016/j.jfineco.2017.11.007
- Behringer, N., & Sassenberg, K. (2015). Introducing social media for knowledge management: Determinants of employees’ intentions to adopt new tools. Computers in Human Behavior, 48, 290–296. https://doi.org/10.1016/j.chb.2015.01.069
- Berger, A. N., Bouwman, C. H. S., & Kim, D. (2017). Small bank comparative advantages in alleviating financial constraints and providing liquidity insurance over time. The Review of Financial Studies, 30(10), 3416–3454. https://doi.org/10.1093/rfs/hhx038
- Berger, A. N., & Udell, G. F. (1995). Relationship lending and lines of credit in small firm finance. Journal of Business, 68, 35–381.
- Berger, A. N., & Udell, G. F. (2002). Small business credit availability and relationship lending: The importance of bank organisational structure. The Economic Journal, 112(477), F32–F53. https://doi.org/10.1111/1468-0297.00682
- Blundell, R. W., & Bond, S. R. (1998). Initial conditions and moment restrictions in dynamic panel data models. Journal of Econometrics, 87(1), 115–143. https://doi.org/10.1016/S0304-4076(98)00009-8
- Bogea, F., Brito, Z., & Pereira, E. (2018). Determinants of social media adoption by large companies. Journal of Technology Management & Innovation, 13(1), 11–18. https://doi.org/10.4067/S0718-27242018000100011
- Bolton, R. N. (2011). Customer engagement: Opportunities and challenges for organizations. Journal of Service Research, 14(3), 272–274. https://doi.org/10.1177/1094670511414582
- Boot, A. W. A. (2000). Relationship banking: What do we know? Journal of Financial Intermediation, 9(1), 7–25. https://doi.org/10.1006/jfin.2000.0282
- Cao, Y., Ajjan, H., Hong, P., & Le, T. (2018). Using social media for competitive business outcomes: An empirical study of companies in China. Journal of Advances in Management Research, 15(2), 211–235. https://doi.org/10.1108/JAMR-05-2017-0060
- Cawsey, T., & Rowley, J. (2016). Social media brand building strategies in B2B companies. Marketing Intelligence & Planning, 34(6), 754–776. https://doi.org/10.1108/MIP-04-2015-0079
- Chauhan, Y., & Kumar, B. S. (2018). Do investors value the nonfinancial disclosure in emerging markets? Emerging Markets Review, 37, 32–46. https://doi.org/10.1016/j.ememar.2018.05.001
- Ciciretti, R., Hasan, I., & Zazzara, C. (2009). Do Internet activities add values? Evidence from the traditional banks. Journal of Financial Services Research, 35(1), 81–98. https://doi.org/10.1007/s10693-008-0039-2
- Courchane, M., Nickerson, D., & Sullivan, R. (2002). Investment in internet banking as a real option: Theory and tests. Journal of Multinational Financial Management, 12(4–5), 347–363. https://doi.org/10.1016/S1042-444X(02)00015-4
- Dahnil, M. I., Marzuki, K. M., Langgat, J., & Fabeil, N. F. (2014). Factors influencing SMEs adoption of social media marketing. Procedia - Social and Behavioral Sciences, 148, 119–126. https://doi.org/10.1016/j.sbspro.2014.07.025
- DeYoung, R., Lang, W. W., & Nolle, D. (2007). How the Internet affects output and performance at community banks. Journal of Banking & Finance, 31(4), 1033–1060. https://doi.org/10.1016/j.jbankfin.2006.10.003
- Drummond, C., McGrath, H., & O'Toole, T. (2018). The impact of social media on resource mobilisation in entrepreneurial firms. Industrial Marketing Management, 70, 68–89. https://doi.org/10.1016/j.indmarman.2017.05.009
- Durkin, M., McGowan, P., & Murray, L. (2014). Perspectives on the potential for social media to improve communication in small business-bank relationships. The International Journal of Entrepreneurship and Innovation, 15(4), 251–264. https://doi.org/10.5367/ijei.2014.0163
- Durkin, M., Mulholland, G., & McCartan, A. (2015). A socio-technical perspective on social media adoption: A case from retail banking. International Journal of Bank Marketing, 33(7), 944–962. https://doi.org/10.1108/IJBM-01-2015-0014
- Edelman, D. (2010). Branding in the digital age: You’re spending your money in all the wrong places. Harvard Business Review, 88, 62–69.
- EFMA. (2013). The global retail banking digital marketing report 2013. http://www.wipro.com/documents/the-global-retail-banking-digital-marketing-report-2013.pdf
- eMarketer. (2017, June). Worldwide social network users: eMarketer’s estimates and forecast for 2016–2021. https://www.emarketer.com/Article/eMarketer-Updates-Worldwide-Social-Network-User-Figures/1016178
- Farshid, M., Plangger, K., & Nel, D. (2011). The social media faces of major global financial service brands. Journal of Financial Services Marketing, 16(3–4), 220–229. https://doi.org/10.1057/fsm.2011.19
- Filip, D., Jackowicz, K., & Kozłowski, Ł. (2017). Influence of internet and social media presence on small, local banks’ market power. Baltic Journal of Economics, 17(2), 190–214. https://doi.org/10.1080/1406099X.2017.1376856
- Galati, A., Crescimanno, M., Tinervia, S., & Fagnani, F. (2017). Social media as a strategic marketing tool in the Sicilian wine industry: Evidence from Facebook. Wine Economics and Policy, 6(1), 40–47. https://doi.org/10.1016/j.wep.2017.03.003
- Gallaugher, J., & Ransbotham, S. (2010). Social media and customer dialog management at starbucks. MIS Quarterly Executive, 9, 197–212.
- Gandomi, A., & Haider, M. (2015). Beyond the hype: Big data concepts, methods, and analytics. International Journal of Information Management, 35(2), 137–144. https://doi.org/10.1016/j.ijinfomgt.2014.10.007
- Giaretta, E., & Chesini, G. (2019). Share this pic!: A picture of the adoption of online social media by Italian banks. In E. Gualandri, V. Venturelli, & A. Sclip, Frontier topics in banking: Investigating new trends and recent developments (pp. 165–187). Palgrave Macmillan.
- Grandon, E., & Pearson, J. M. (2004). Electronic commerce adoption: An empirical study of small and medium US businesses. Information & Management, 42(1), 197–216. https://doi.org/10.1016/j.im.2003.12.010
- Gruen, T. W., Osmonbekov, T., & Czaplewski, A. J. (2006). eWOM: The impact of customer-to-customer online know-how exchange on customer value and loyalty. Journal of Business Research, 59(4), 449–456. https://doi.org/10.1016/j.jbusres.2005.10.004
- Hasan, I., Jackowicz, K., Kowalewski, O., & Kozłowski, Ł. (2017). Do local banking market structures matter for SME financing and performance? New evidence from an emerging economy. Journal of Banking & Finance, 79, 142–158. https://doi.org/10.1016/j.jbankfin.2017.03.009
- Hernández-Murillo, R., Llobet, G., & Fuentes, R. (2010). Strategic online banking adoption. Journal of Banking & Finance, 34(7), 1650–1663. https://doi.org/10.1016/j.jbankfin.2010.03.011
- Hernando, I., & Nieto, M. (2007). Is the internet delivery channel changing banks’ performance? The case of Spanish banks. Journal of Banking & Finance, 31(4), 1083–1099. https://doi.org/10.1016/j.jbankfin.2006.10.011
- Hinson, R. E., Madichie, N. O., & Ibrahim, M. (2012). A dialogic communications interrogation of the online brand dispositions of banks operating in Ghana. International Journal of Bank Marketing, 30(7), 508–526. https://doi.org/10.1108/02652321211274264
- Hong, S. (2012). Online news on Twitter: Newspapers’ social media adoption and their online readership. Information Economics and Policy, 24(1), 69–74. https://doi.org/10.1016/j.infoecopol.2012.01.004
- Höwer, D. (2016). The role of bank relationships when firms are financially distressed. Journal of Banking & Finance, 65(C), 59–75. https://doi.org/10.1016/j.jbankfin.2016.01.002
- Hsu, L., & Lawrence, B. (2016). The role of social media and brand equity during a product recall crisis: A shareholder value perspective. International Journal of Research in Marketing, 33(1), 59–77. https://doi.org/10.1016/j.ijresmar.2015.04.004
- Kaplan, A. M., & Haenlein, M. (2010). Users of the world unite! The challenges and opportunities of social media. Business Horizons, 53(1), 59–68. https://doi.org/10.1016/j.bushor.2009.09.003
- Kim, G., Lim, H., & Brymer, R. A. (2015). The effectiveness of managing social media on hotel performance. International Journal of Hospitality Management, 44, 165–171. https://doi.org/10.1016/j.ijhm.2014.10.014
- Kim, S., Koh, Y., Cha, J., & Lee, S. (2015). Effects of social media on firm value for U.S. restaurant companies. International Journal of Hospitality Management, 49, 40–46. https://doi.org/10.1016/j.ijhm.2015.05.006
- Kiss, H. J., Rodriguez-Lara, I., & Rosa-García, A. (2014). Do social networks prevent or promote bank runs? Journal of Economic Behavior & Organization, 101, 87–99. https://doi.org/10.1016/j.jebo.2014.01.019
- Lam, H. K. S., Yeung, A. C. L., & Cheng, E. T. C. (2016). The impact of firms’ social media initiatives on operational efficiency and innovativeness. Journal of Operations Management, 47–48(1), 28–43. https://doi.org/10.1016/j.jom.2016.06.001
- Laroche, M., Habibi, M. R., & Richard, M. O. (2013). To be or not to be in social media: How brand loyalty is affected by social media? International Journal of Information Management, 33(1), 76–82. https://doi.org/10.1016/j.ijinfomgt.2012.07.003
- Laroche, M., Habibi, M. R., Richard, M. O., & Sankaranarayanan, R. (2012). The effects of social media based brand communities on brand community markers, value creation practices, brand trust and brand loyalty. Computers in Human Behavior, 28(5), 1755–1767. https://doi.org/10.1016/j.chb.2012.04.016
- Larsson, A., & Viitaoja, Y. (2017). Building customer loyalty in digital banking: A study of bank staff’s perspectives on the challenges of digital CRM and loyalty. International Journal of Bank Marketing, 35(6), 858–877. https://doi.org/10.1108/IJBM-08-2016-0112
- Lipsman, A., Mudd, G., Rich, M., & Bruich, S. (2012). The power of ‘like’: How brands reach (and influence) fans through social–media marketing. Journal of Advertising Research, 52(1), 40–52. https://doi.org/10.2501/JAR-52-1-040-052
- Malaquias, F., Malaquias, R., & Hwang, Y. (2018). Understanding the determinants of mobile banking adoption: A longitudinal study in Brazil. Electronic Commerce Research and Applications, 30, 1–7. https://doi.org/10.1016/j.elerap.2018.05.002
- Mangold, W. G., & Faulds, D. J. (2009). Social media: The new hybrid element of the promotion mix. Business Horizons, 52(4), 357–365. https://doi.org/10.1016/j.bushor.2009.03.002
- Michaelidou, N., Siamagka, N. T., & Christodoulides, G. (2011). Usage, barriers and measurement of social media marketing: An exploratory investigation of small and medium B2B brands. Industrial Marketing Management, 40(7), 1153–1159. https://doi.org/10.1016/j.indmarman.2011.09.009
- Mitic, M., & Kapoulas, A. (2012). Understanding the role of social media in bank marketing. Marketing Intelligence & Planning, 30(7), 668–686. https://doi.org/10.1108/02634501211273797
- Mullan, J., Bradley, L., & Loane, S. (2017). Bank adoption of mobile banking: Stakeholder perspective. International Journal of Bank Marketing, 35(7), 1154–1174. https://doi.org/10.1108/IJBM-09-2015-0145
- NBP. (2017). Financial system in Poland 2016. http://www.nbp.pl/en/systemfinansowy/fsd_2016.pdf
- Oh, C., Roumani, Y., Nwankpa, J. K., & Hu, H.-F. (2017). Beyond likes and tweets: Consumer engagement behavior and movie box office in social media. Information & Management, 54(1), 25–37. https://doi.org/10.1016/j.im.2016.03.004
- Ongena, S., & Smith, D. (2001). The duration of bank relationships. Journal of Financial Economics, 61(3), 449–475. https://doi.org/10.1016/S0304-405X(01)00069-1
- Paniagua, J., & Sapena, J. (2014). Business performance and social media: Love or hate? Business Horizons, 57(6), 719–728. https://doi.org/10.1016/j.bushor.2014.07.005
- Petersen, A. M., & Rajan, G. R. (1994). The benefits of lending relationship: Evidence from small business data. The Journal of Finance, 49(1), 3–38. https://doi.org/10.1111/j.1540-6261.1994.tb04418.x
- PFSC. 2018. Monthly data on the banking sector. Polish Financial Supervision Authority. https://www.knf.gov.pl/en/REPORTS_AND_ANALYSIS/Banking/Monthly_data
- Pozza, I. D. (2014). Multichannel management gets “social”. European Journal of Marketing, 48, 1274–1295.
- Prilmeier, R. (2017). Why do loans contain covenants? Evidence from lending relationships. Journal of Financial Economics, 123(3), 558–579. https://doi.org/10.1016/j.jfineco.2016.12.007
- Rajan, R. G. (1992). Insiders and outsiders: The choice between informed and arm’s-length debt. The Journal of Finance, 47(4), 1367–1440. https://doi.org/10.1111/j.1540-6261.1992.tb04662.x
- Silver, L., & Vegholm, F. (2009). The dyadic bank–SME relationship. Journal of Small Business and Enterprise Development, 16(4), 615–627. https://doi.org/10.1108/14626000911000956
- Sinclaire, J. K., & Vogus, C. E. (2011). Adoption of social networking sites: An exploratory adaptive structuration perspective for global organizations. Information Technology and Management, 12(4), 293–314. https://doi.org/10.1007/s10799-011-0086-5
- Stein, J. C. (2002). Information production and capital allocation: Decentralized versus hierarchical firms. The Journal of Finance, 57(5), 1891–1921. https://doi.org/10.1111/0022-1082.00483
- Szopiński, T. S. (2016). Factors affecting the adoption of online banking in Poland. Journal of Business Research, 69(11), 4763–4768. https://doi.org/10.1016/j.jbusres.2016.04.027
- Tang, C., Mehl, M. R., Eastlick, M. A., He, W., & Card, N. A. (2016). A longitudinal exploration of the relations between electronic word-of-mouth indicators and firms’ profitability: Findings from the banking industry. International Journal of Information Management, 36(6), 1124–1132. https://doi.org/10.1016/j.ijinfomgt.2016.03.015
- Thong, J. Y. L., & Yap, C. S. (1995). CEO characteristics, organizational characteristics and information technology adoption in small businesses. Omega, 23(4), 429–442. https://doi.org/10.1016/0305-0483(95)00017-I
- Tsimonis, G., & Dimitriadis, S. (2014). Brand strategies in social media. Marketing Intelligence & Planning, 32(3), 328–344. https://doi.org/10.1108/MIP-04-2013-0056
- Wamba, S. F., & Carter, L. (2014). Social media tools adoption and use by SMEs: An empirical study. Journal of Organizational and End User Computing, 26(2), 1–16. https://doi.org/10.4018/joeuc.2014040101
- We are Social. 2018. Global digital report 2018. https://wearesocial.com/blog/2018/01/global-digital-report-2018
- Wongsansukcharoen, J., Trimetsoontorn, J., & Fongsuwan, W. (2015). Social CRM, RMO and business strategies affecting banking performance effectiveness in B2B context. Journal of Business & Industrial Marketing, 30(6), 742–760. https://doi.org/10.1108/JBIM-02-2013-0039