Abstract
Social media are increasingly used as platforms to not only socialise but also to shop for products and services. Social commerce is the new trend in e-commerce that leverages the enhanced consumer-to-consumer interactions to support shopping processes. Based on the concept of social commerce constructs suggested by Nick Hajli, this article investigates the role of social commerce constructs, emotional and informative support, and trust as drivers of social commerce intention. We apply multi-group analysis to validate the drivers of social commerce for two of the most relevant social media platforms: Facebook and Instagram. Based on a sample of 800 social commerce users, our findings reveal that social commerce constructs are strong drivers of social commerce intention for both social media platforms; however, there are differences between the two as Facebook seems to generate higher emotional support and trust than Instagram.
1. Introduction
The transition to Web version 2.0 has allowed consumers to play a more active role as they can use social networks to create and disseminate content, sharing experiences and knowledge about products and services (Berthon et al., Citation2012). As a result, social commerce (s-commerce) has appeared as a new stream in e-commerce defined by the integration of social media in e-commerce platforms (Hajli & Sims, Citation2015). With the popularity of social media, the role of the Internet has changed, since the interaction between consumers allows them to obtain social support from the online community, thus supporting online purchasing decision-making (Hajli, Citation2014a; Liang et al., Citation2011). Consumers can access information on the previous experiences of other consumers published on digital platforms, these contents being a relevant source of information for purchase decision-making (Park et al., Citation2007). Organisations are heavily investing in different social media applications to capture the value of users’ social interaction in those online platforms (Trkman & Trkman, Citation2018). According to Euromonitor International (Citation2019), 24% of consumers worldwide bought online via social media in 2019, and data suggest there is much potential for social media platforms as a lucrative sales channel.
The literature on s-commerce is still limited, and additional efforts are needed to clarify the concept and differentiate it from e-commerce. The work of Hajli et al. helped to advance our knowledge of s-commerce in two directions. First, by investigating the role of social media in building trust and driving buying intentions (Hajli, Citation2014b; Hajli et al., Citation2017). Second, by contributing to enrich the concept of s-commerce by developing the so-called social commerce constructs (SCCs) (Hajli, Citation2015; Hajli & Sims, Citation2015). By providing constructs that are unique to s-commerce, the differential features of s-commerce that require separate investigation are highlighted. SCCs have emerged with the development of Web version 2.0 along with the popularity of social media to allow interaction between consumers and between consumers and firms in social platforms. SCCs have a triple dimension: 1) forums and communities; 2) comments and reviews; and 3) recommendations and referrals (Hajli, Citation2015).
Different researchers have used the SCC concept developed by Hajli (Citation2015) to investigate its relationships with other variables that influence consumers’ behaviour to perform s-commerce activities. Thus, consideration has been given to the relationship of SCCs with variables such as: social support (Shanmugam et al., Citation2016), trust (Hajli, Citation2015), purchase intention (Hajli, Citation2015), perceived risk (Soleimani et al., Citation2017) and loyalty (Zamrudi et al., Citation2016). Despite the growing body of literature, there is still the need to build theoretical frameworks to include social interactions of consumers in an integrative model that helps to understand the behaviour of users of s-commerce, and that refers to specific social networks other than Facebook (Hajli et al., Citation2017). Most studies do not refer to specific social networks (Shanmugam et al., Citation2016), and when they do, they refer to Facebook (Hajli & Sims, Citation2015).
To bridge this research gap, this study aims to analyse the role of social commerce constructs, informational social support, emotional social support, and trust as drivers of s-commerce for two different social media platforms, namely Facebook and Instagram. Facebook and Instagram are the most relevant social network sites at a global level. Facebook was the pioneering social media platform, and with 2.27 billion users is the leading network. Instagram was born as a photo-sharing platform, being the first of its kind; today, it has 500 million users (Euromonitor International, Citation2019). However, these networks are different in terms of the type of content they host, as well as the profiles of their users (Waterloo et al., Citation2018). S-commerce models have not been tested among users of different social media platforms. By performing MGA using two independent samples of Facebook and Instagram users, we aim to test the robustness of the model within social network users.
The following sections of this paper review literature on s-commerce, social support, and trust in social networks. Then, the research model and hypotheses are presented. After describing the methodology, the findings are presented and discussed, followed by the conclusions and proposed future lines of research.
2. Literature review
2.1. S-commerce and social commerce constructs
S-commerce emerged from the evolution of e-commerce and its interaction with social networks, innovatively bringing benefits based on interactive communication between consumers (Wang et al., Citation2019). Liang and Turban (Citation2011) defined s-commerce as the use of Web version 2.0 and social technologies that facilitate consumers’ interactions to support the purchase of products or services on the Internet. According to Lin et al. (Citation2017), s-commerce encompasses a variety of commercial activities that support consumers in the evaluation or pre-purchase stage, in purchasing decisions and in the post-purchase phase. Indeed, s-commerce does not only encompass purchasing through social networks, but it also includes interactions on social networks during any phase of the purchasing process: prior information, recommendation and subsequent evaluation (Kim & Park, Citation2013; Lin et al., Citation2017).
With the emergence of social media, different spaces and platforms were created that enable interaction between users through social networking sites, allowing potential buyers to socialise with each other (Hajli & Sims, Citation2015). Through social networks, considered s-commerce platforms, consumers can access information published by other members and learn about previous experiences to support decision-making during the purchasing process (Hajli, Citation2015; Hajli et al., Citation2014; Liang & Turban, Citation2011; Lin et al., Citation2017; Sarulatha & Sasirekha, Citation2018).
According to Hajli (Citation2015), the platforms enabled by social technologies are social commerce constructs or SCCs. Thus, SCCs are defined as ‘social platforms which have emerged from Web 2.0 and empowered consumers to generate content and share their experiences’ (Hajli, Citation2015, p. 184). Three SCCs are proposed, which despite sharing the basic function of facilitating the exchange of information, show different technical capacities. These constructs are:
Ratings and reviews. Through ratings and reviews, consumers provide comprehensive information about products and services for the benefit of other potential customers. This information even reduces the seller’s need for commercial information.
Recommendations and referrals. This dimension plays an important role in s-commerce, since sensorial absence makes people trust other members’ recommendations and referrals before purchasing a product.
Forums and communities. These social spaces play an important role because of the social interaction they facilitate. In online forums and communities, their members support one another by sharing experiences and information. This is a powerful new means of generating eWOM.
2.2. Social support on social media
Social support refers to the relationships between people who form a community, where they share experiences, knowledge and feelings. Studies in social psychology have demonstrated the benefits social support has for the quality of relationships and reducing people’s stress. According to social support theory (Cobb, Citation1976), the objective of the individual is to satisfy social needs such as affection, esteem, approval, sense of belonging, identity and security through interaction with others.
Social support theory has proved useful for studying individuals’ relationships on virtual social networks. According to researchers, the primary motivation for people to participate in social communities, sharing information and previous experiences, is for social support (Hajli & Sims, Citation2015; Shanmugam et al., Citation2016). In a digital environment, the information shared through social networks is perceived as social support. Therefore, individuals who interact via digital media will be willing to receive and share valuable information from previous experiences of buying products and services with other users (Bai et al., Citation2015; Hajli, Citation2014a). Online social support is defined as ‘online actions that individuals carry out by collaborating with peers through social media’ (Hajli & Sims, Citation2015).
Social support in digital media has two dimensions: emotional support and informational support (Liang et al., Citation2011). Emotional support refers to empathy, concern, care, love, understanding, and encouragement among members of online communities, making the receiver of such support feel that they are valued and taken into account. Informational support focuses on the generation of information for other members of their social network, offering advice, opinions or suggestions that can provide relevant information to solve problems, generate new ideas or make better purchasing decisions (Hajli, Citation2015; Liang et al., Citation2011).
2.3. Trust in social networks
Trust has been widely studied in the field of business studies. Trust is recognised as a key element of relationships between individuals, between organisations, and between an individual and an organisation (Morgan & Hunt, Citation1994). Trust is the belief by an individual that an exchange will occur in a manner consistent with expectations of confidence (Ba & Pavlou, Citation2002). Trust acquires greater relevance when transactions take place in an online context, where there is a high level of uncertainty (Bai et al., Citation2015; Pavlou, Citation2003). Thus, building trust is a key to overcome online consumers’ perceptions of risk and insecurity (Peštek et al., Citation2011). The absence of physical interaction between buyers and sellers in digital purchasing processes makes trust a critical factor for s-commerce, as it helps to mitigate the risks perceived by consumers (Hajli, Citation2014a; Sarulatha & Sasirekha, Citation2018).
According to trust transfer theory, trust can be transferred by different sources such as individuals or contexts (Shi et al., Citation2013). Various studies have shown that trust transfer can occur in both physical and digital environments, i.e., between members of a virtual community (Shi et al., Citation2013). Trust transfer can be generated in s-commerce with the support of a trusted third party, because according to trust transfer theory, trust can be transmitted from one user to another, or from the seller to the buyer (Bai et al., Citation2015).
3. Research model and hypotheses
Our review of the literature revealed that SCCs can be powerful generators of social support and trust (Hajli, Citation2015). Based on social support theory we include in the model informational social support and emotional social support. Although additional dimensions of social support could be relevant in an offline context, Liang et al. (Citation2011) argued that, in an online context, informational and emotional support capture the motivations for people to participate in virtual social networks. Trust in social networks has been found to be a key factor in predicting users’ purchase intentions (Hajli et al., Citation2017), and is further increased by social support (Shanmugam et al., Citation2016). Thus, our model () suggests relationships between five relevant variables in s-commerce that had not been previously investigated in the same model.
3.1. Social commerce constructs and social support
In online channels, the information that consumers have about purchasing processes carried out by third parties helps them make decisions more quickly and securely (Chen & Xie, Citation2005). Social platforms allow consumers to create and share content in a collaborative environment that empowers them to generate information that can influence other consumers’ brand choices (Hajli, Citation2014b; Hajli, Citation2015).
According to Cobb’s social support theory (1976), the benefits of social support stem from the information exchanged between people, and the perception of being loved, esteemed and valued as a member of a social group. The information shared through SCCs can provide social support to consumers, helping them throughout the decision-making process in s-commerce activities (Hajli et al., Citation2014; Hajli & Sims, Citation2015).
Thus, it has been suggested that people generate interactions through SCCs that, in turn, help to provide social support, both emotional, fuelling security due to the sense of community, and informational, by allowing others to obtain information on products and on other users’ shopping and consumption experiences (Hajli & Sims, Citation2015). According to the information presented, the following causal relationships are proposed:
H1a: SCCs have a positive effect on the emotional support perceived by users on social networks.
H1b: SCCs have a positive effect on the informational support perceived by users on social networks.
3.2. Social commerce constructs and social media trust
Trust transfer theory helps to understand the relationship between SCCs and trust, since it explains that a known entity can transfer the perception of trust to another relatively unknown entity, thus helping to build trust (Shi et al., Citation2013). In an online context, consumers do not have sensory cues like those they have in a physical environment such as a traditional purchasing channel (Farivar et al., Citation2017); hence, in online channels trust must be generated through other means.
Interactions between members of a social network influence other users’ level of trust, which impacts their intention to buy online (Gibreel et al., Citation2018). SCCs allow social interaction and information exchange, thus generating trust among users (Shanmugam et al., Citation2016). On the one hand, when people in forums and communities read comments and ratings about a product or service, their level of familiarity with a website or social networking site is likely to increase, thus enhancing trust towards that site (Hajli et al., Citation2014). On the other hand, it was found that a consumer’s ratings and comments about a product influence other users’ satisfaction with and trust in making purchases via virtual media (Hajli, Citation2015). Thus, our second research hypothesis posits:
H2: SCCs have a positive influence on users’ trust in social networks.
3.3. Social commerce constructs and s-commerce intention
SCCs enable users to generate and transmit content that helps consumers obtain answers during the purchase process, positively influencing users’ intentions to engage in s-commerce activities (Hajli, Citation2015). Indeed, the generation of content through the dimensions of SCCs generates value for other potential consumers (Huang & Benyoucef, Citation2013).
The information created and transmitted through SCCs is based on consumers’ prior shopping experiences, and it has been proved that such content can have a positive effect on other users’ intention to carry out s-commerce activities (Hajli, Citation2015). Similarly, SCCs allow consumer interaction on social media, generating valuable information that can influence other consumers in s-commerce activities (Hajli & Sims, Citation2015). According to the information presented, the following causal relationship is proposed:
H3: SCCs have a positive influence on s-commerce intention.
3.4. Influence of social support on trust in social networks
In a s-commerce context, consumers have the opportunity to share prior experiences with products or services, by reviewing the opinions of others, participating in forums, narrating their experiences and recommending products and services (Hajli, Citation2015). It has been suggested that consumers who have already carried out commercial activities through social networks often share their experiences with other customers, supporting them both informationally and emotionally in the s-commerce process (Hajli et al., Citation2014).
The theories of social support and trust transfer help us understand the influence of content and experiences shared on social networks in the transmission of trust between users (Shi et al., Citation2013). Thus, trust would stem from social support, either because the information transmitted between members of an online community leads to the forging of an emotional bond (emotional support) that facilitates the building of trust in a relationship, or because the content shared contributes to problem solving (informational support) (Hajli, Citation2014b; Shanmugam et al., Citation2016). Thus, an increase in social support among members of an online community makes people feel more connected with one another, and more trustful (Hajli & Sims, Citation2015). According to the information presented, the following causal relationships are proposed:
H4a: Emotional support has a positive effect on user trust in social media.
H4b: Informational support has a positive effect on user trust in social media.
3.5. Social media trust and s-commerce intention
Trust is a key variable that influences business relationships (Pavlou, Citation2003), acquiring greater relevance in virtual environments due to the uncertainty that users may experience when using digital media to perform transactions (Mutz, Citation2005).
Trust transfer theory offers a framework that allows us to understand the way in which a user transfers trust to another user and how this influences s-commerce intention (Shi et al., Citation2013). The virtual environment of online transactions prompts customers to search for trusted third parties to help them reduce the risk of purchasing on social networks (Bai et al., Citation2015). It has been found that interaction between users generates trust and this, in turn, influences s-commerce intention (Hajli, Citation2014a). Researchers have pointed out that greater trust generated by the interaction between users and brands through SSCs favours the conditions for the generation of transactions (Hajli, Citation2015).
Thus, previous research argued that trust in social network sites would determine credibility in the generation of content and activities carried out by virtual sellers (Hajli et al., Citation2017). Likewise, the content generated by members of an online community positively influences the trust of other users and the purchase decisions of other users in s-commerce activities (Hajli, Citation2015; Wang et al., Citation2019). Based on the information presented, the following hypothesis is proposed:
H5: User trust in social networks has a positive influence on s-commerce intention.
4. Research methodology
4.1. Measurement and data collection
The constructs of the research model were measured using scales previously used in literature, with slight modifications where necessary (see Appendix). Responses were collected using seven-point Likert scales, from 1 (strongly disagree) to 7 (strongly agree). The SCCs variable is a second-order, reflective-type construct including three dimensions: forums and communities, ratings and reviews and recommendations and referrals (Hajli, Citation2015). The remaining variables constructs are first-order reflective constructs.
Data were collected by means of an online survey conducted in Mexico. A pre-test of the questionnaire was carried out with 20 students to check the correct interpretation of the questions, resulting in the recommendation of minor modifications in the wording of certain items. The criteria for selecting respondents were that they had to use either Facebook or Instagram and had used them in purchase processes, either to search for information or to buy. The questionnaire was distributed electronically via Facebook, Instagram and email to maximise the number of participants. In total, 1796 links were sent; 400 valid responses were obtained for Facebook and 400 for Instagram. The final sample comprised 64% women and 36% men, of whom 63% were aged 18–23, 20% 24–29, 5% 30–34 and 12% over 35.
4.2. Analytical technique
The research model was estimated through structural equations, more specifically applying partial least squares (PLS-SEM). Unlike covariance-based SEM, PLS is appropriate for testing models with latent constructs of formative nature. This technique offers additional advantages (Hair et al., Citation2016): it simultaneously evaluates the validity and reliability of the constructs, it is effective with small samples, and it is useful for comparatively analysing two identical models using the multi-group analysis (MGA) technique.
5. Results
In order to assess the model for Facebook and Instagram users using PLS-SEM and to compare the results of the estimated path coefficients, this study employed a three-stage analysis approach: assessment of measurement models, assessment of structural models and MGA.
5.1. Measurement model
and show the values for simple and composite reliability for the Facebook and Instagram models, respectively. Simple reliability was evaluated using Cronbach’s alpha, considering values equal to or greater than 0.70 as good (Hair et al., Citation2016). Composite reliability considers load values above 0.70 to be satisfactory (Hair et al., Citation2016). This criterion was met, being very close to the cut-off point for two indicators, SCI5 for Facebook and SCI6 for Instagram. We decided to keep both indicators due to the MGA requirement to maintain equality between the groups to be analysed. Likewise, the convergent validity of the measurement models for Facebook () and Instagram () was validated, since the AVE values reached the cut-off value of 0.50 (Fornell & Larcker, Citation1981).
Table 1. Reliability and validity – Facebook model.
Table 2. Reliability and validity – Instagram model.
To evaluate discriminant validity, the Fornell and Larcker (Citation1981) method was applied. This method proposes the existence of discriminant validity between two latent variables if the shared variance (R2) between two constructs is less than the square root of the AVE of each construct. The results for both models, Facebook and Instagram, indicated that there was discriminant validity. Additionally, discriminant validity and convergent validity were evaluated by means of cross-loadings analysis ( and ), which requires an indicator to show a greater load in its own construct than in any other construct of the structural model (Hair et al., Citation2016).
Table 3. Indicator loadings and cross loadings – Facebook model.
Table 4. Indicator loadings and cross loadings – Instagram model.
5.2. Structural model
and present the results of the estimation of the model for Facebook and Instagram, respectively, showing the values of the R2 statistic for the dependent variables and the values of the structural paths and their significance for the relationships between the constructs. The path coefficients were evaluated using the bootstrapping procedure with 4999 samples, based on the original sample (Henseler et al., Citation2016). The models show an adequate fit to the data as the values of standardised root mean square residual (SRMR) are 0.09 for Facebook and 0.086 for Instagram. According to Ringle et al. (Citation2015), values for SRMR less than 0.10 or of 0.08 (in a more conservative version) are considered a good fit.
Figure 2. MGA structural model Facebook. Notes. *p <.05; ***p <.001. Broken lines indicate non-significant relationships. Source: Authors’ own work.
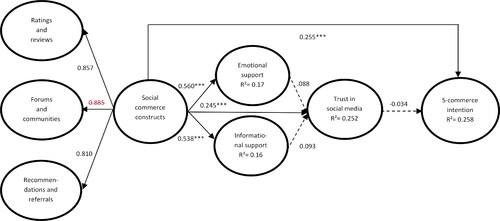
Figure 3. MGA structural model Instagram. Notes. ***p <.001. Broken lines indicate non-significant relationships. Source: Authors’ own work.
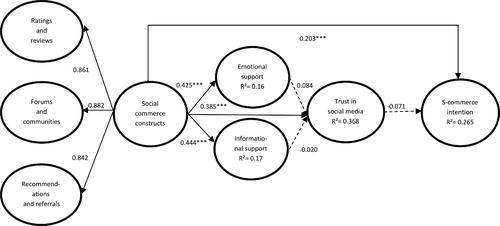
For the Facebook model (), H1a and H1b were supported since SCCs had a positive impact on emotional (0.560) and informational (0.538) social support. The relationship established between SCCs and confidence was positive and significant (0.245), thus confirming H2. SCCs showed to have a positive impact on s-commerce intention (0.255), thus supporting H3. As regards the effects of social support, no significant effect of emotional social support (0.088) or informational social support (0.093) on trust was observed; therefore, H4a and H4b were rejected. Similarly, trust did not have a significant impact on s-commerce intention (0.034), so H5 was rejected for the Facebook model.
For the Instagram model (), SCCs were observed to have a positive impact on emotional (0.425) and informational (0.444) social support, so H1a and H1b were accepted. Likewise, SCCs showed a positive and significant impact on trust (0.385), hence H2 was accepted. H3, which established that SCCs had a positive relationship on s-commerce intention, was also corroborated (0.203). Regarding social support, the effect of emotional support (0.084) or informational support (−0.020) on trust was not significant, hence H4a and H4b were rejected. Finally, H5 was not fulfilled, since trust did not show a significant impact on s-commerce intention (−0.071).
5.3. Multi-group analysis (MGA)
In order to examine the robustness of our research model within users of different social media networks, we applied MGA using two independent samples of Facebook users and Instagram users. Before conducting MGA, measurement invariance must be assessed using the measurement invariance of composites (MICOM) approach (Henseler et al., Citation2016). The MICOM process consists of three steps: (a) evaluation of configuration invariance; (b) evaluation of compositional invariance; and (c) evaluation of equal means and variances. Following the MICOM procedure, total measurement invariance was established as a prerequisite for comparing and interpreting the specific differences between coefficients of the MGA groups (Henseler et al., Citation2016).
Two non-parametric methods were used: Henseler’s MGA method (Henseler et al., Citation2009) and the permutation test (Chin & Dibbern, Citation2010). These techniques are useful for assessing the differences in path coefficients between two groups (Sarstedt et al., Citation2011). shows the results of the MGA. Henseler’s MGA directly compares group-specific bootstrap estimates from each bootstrap sample. According to this method, a p value of the differences between the path coefficients of less than .05 or greater than .95 indicates significant differences (p<.05) between the path coefficient across two groups (Henseler et al., Citation2009). The permutation test also returns a p value, with significant differences at 5% if the p value is less than .05. The results revealed significant differences between the Facebook model and the Instagram model in three of the relationships proposed: the relationship between SCCs and emotional support (H1a), the relationship between SCCs and trust (H2), and the relationship between emotional support and trust (H4a). The remaining relationships (i.e., hypotheses H1b, H3, H4b and H5) did not present significant differences between the Facebook and Instagram models, since the differences between the path coefficients yielded values above 0.05 and below 0.95.
Table 5. Multi-group analysis.
6. Discussion and conclusion
This article employed the social support and trust transfer theories to propose a model to explain consumer intention to carry out s-commerce activities within two different social networks, Facebook and Instagram. The model was tested applying MGA on SEM-PLS with data from a survey answered by 800 social network users. Based on the central variable of SCCs, our research confirmed that individuals’ interaction through social networks has a direct influence on s-commerce intention.
Our findings show that the interaction and communication between users through the dimensions of SCCs – forums and communities, ratings and reviews, and recommendations and referrals – generates a perception of support among members of the social network, be it emotional or informational. These results confirm the results presented in previous studies reporting this relationship (Hajli & Sims, Citation2015; Shanmugam et al., Citation2016). Moreover, the results presented here confirm a positive relationship between SCCs and users’ trust in social networks. These findings are consistent with those reported in previous studies that prove that the information generated and shared by users through social media influences other consumers’ trust in performing s-commerce activities (Hajli, Citation2015; Hajli et al., Citation2014).
The results do not show that emotional social support and informational social support influence users’ trust. The rejection of these relationships suggests that Facebook and Instagram users do not need to obtain emotional and informative social support through social networks to generate trust, but rather that trust appears thanks to the contents generated by other users who share their experiences in s-commerce activities. Likewise, the relationship between trust and s-commerce intention was not confirmed for either the Facebook model or the Instagram model. However, previous research reported that content generated by users on social networks helps build trust in an online community (Hajli, Citation2015; Shanmugam et al., Citation2016). One possible explanation is that many young people today are digital natives, and they use social networks, such as Facebook and Instagram not only for personal interactions but also for commercial and marketing purposes. These consumers (who made up a significant part of our sample) do not require social support to have trust in social networks, nor do they require high levels of trust to carry out s-commerce activities.
Perhaps the most interesting conclusion of our research is that the social interaction of users through SCCs directly and positively influences their decision to use social networks in the purchasing process, corroborating the findings of Hajli and Sims (Citation2015). Although the interaction of individuals on s-commerce platforms influences the generation of social support and trust in social networks, our research found that s-commerce intention is not dependent on social support or trust. Thus, SCCs emerge as the sole generators of s-commerce intention.
A relevant contribution made by this paper to the s-commerce literature is the execution of a MGA to evaluate the robustness of the model and the generalisability of the results for two social networks: Facebook and Instagram. The results of the MGA revealed significant differences between the social networks in terms of the effects of SCCs and emotional support (H1a), the relationship between SCCs and trust (H2) and the relationship between emotional support and trust (H4a). The differences indicate that Facebook users have a stronger perception than Instagram users that the social network is a means for generating emotional support or trust. Although Instagram has been reported to be a more engaging platform than Facebook (Rival, Citation2020), our results point out that Facebook is more effective in generating social support and trust. Engagement rates are high if users spend time on the social media sites and like the posts; however, social support and trust build not from the attractiveness of the posts but from the strength of the bonds between the social media users. Facebook is based on reciprocal following, while Instagram relies on non-reciprocal following. Non-reciprocal following is associated with weaker social ties and the inclusion of strangers in one’s network (Waterloo et al., Citation2018). Facebook is a more private network than Instagram; Facebook users typically form networks with existing offline friends and family, whereas Instagram users usually follow, in addition to their friends, other users such as celebrities or politicians. Phua et al. (Citation2017) found that bonding social capital was higher among Facebook than Instagram users; higher bonding capital means that Facebook users, to a greater extent than Instagram users, trust each other, and get emotional support and advice for solving their problems.
Moreover, the credibility and robustness of our model were strengthened by the results of MGA (Henseler et al., Citation2016). Although the size of three path coefficients differed between the two groups, the basic structure of the model was confirmed for the two groups, i.e., the same hypotheses are accepted/rejected. Therefore, the findings confirmed the robustness of our model as the results can be extended to users of Facebook and Instagram, which are the two most popular social networks worldwide. Previous studies testing some of the hypotheses of our model, such as SSC on trust (Hajli, Citation2015) have been tested on samples of Facebook users or have not assessed the stability of the results among users of different social media (e.g., Shanmugam et al., Citation2016). It is worthwhile highlighting that the MGA analysis confirmed that SCCs are the sole drivers of s-commerce intention for both social networks.
This study contributes to the development of the theoretical body of research in the field of social media marketing by integrating the social support and trust transfer theories to understand users’ interaction in s-commerce. The theoretical model used in this study is a comprehensive model integrating SCCs with other relevant constructs in the field of social media and e-commerce, such as social support and trust, to explain s-commerce intention. Furthermore, a key contribution of our research is the analysis of the differences existing in the model for two of the most popular social network sites, Facebook and Instagram. Thus, we have provided a theoretical base for future research on s-commerce.
Our results also have interesting implications for marketing managers. Knowledge of the relationships of the proposed model offers a broad vision to understand consumer behaviour in commercial transactions involving social networks. S-commerce, unlike e-commerce, is based on user-interactions, which take place in different ways and spaces as represented by the three SCCs: forums and communities; comments and reviews; and recommendations and referrals. Our findings show that user interactions through SCCs are key drivers of the intention to carry out commercial activities through both social networks.
Firms can use social media to strengthen commercial relationships with customers and increase social media profitability by generating sales from users’ interactions in social media. If firms want to be more effective in directing traffic from social media to their commercial websites, they should target key users with large numbers of followers; hence their influence would impact potential buyers through communities, recommendations and reviews. Additionally, firms would benefit if more consumers rate and review their products and services, as they would impact on other potential buyers. Hence, they should devise effective incentives for customers not only to rate products but also to write reviews and recommend the product or service to other users.
Furthermore, the correct implementation of SCCs will allow having access to information generated through the social interaction of consumers, developing a possible feedback mechanism that can be useful for generating new on-going improvement strategies and processes. Although our study confirmed that s-commerce intention is built from SCCs for both social networks, specific differences exist that require each social network to be addressed differently. Our study has revealed that SCCs generate higher social support and trust for Facebook users. Thus, community managers should leverage this advantage by selecting to posts contents on Facebook when they want to build trust between their customers, such as the introduction of new products or services or even more in the event of a crisis. On the other side, Instagram would be more effective for generating awareness and attachment towards the brands and products.
Future research opportunities on social commerce are abundant. This study has contributed to the literature as it compared how social commerce develops for users of the two social networks with the most penetration worldwide, Facebook and Instagram. We found that both networks support social commerce; however, there are small differences related to the capability of the social networks to generate emotional support and trust, which is higher for Facebook. Future research could look into these differences, analysing the possible reasons, as well as the implications for firms. In investigating this topic, researchers could also evaluate additional social networks, which could shed some light on the question of the different power of social network sites to generate consumer trust in the purchase process using social media. There are several variables that we did not contemplate in our study that may affect consumer behaviour in s-commerce; for instance, the perceived risk and privacy issues of using social network sites for shopping tasks, as well as the perceived usefulness and ease of use of doing that. Moreover,8 future research could look into brand-specific variables. Social media marketing is a great ally to build powerful brands that engage customers and build loyalty. Looking into the influence of SSCs on brand-related constructs would be an interesting contribution to the field of s-commerce. Some limitations of our research methodology also offer opportunities for researchers. This study was conducted in Mexico; future research can include a cross-cultural analysis to augment the generalisability of the results. The culture variable could be employed in a MGA to understand its influence in s-commerce. This would help global brands to decide the extent of adaptation of the social media marketing strategy.
Disclosure statement
No potential conflict of interest was reported by the authors.
Additional information
Funding
References
- Ba, S., & Pavlou, P. A. (2002). Evidence of the effect of trust building technology in electronic markets: Price premiums and buyer behavior. MIS Quarterly, 26(3), 243–268. https://doi.org/https://doi.org/10.2307/4132332
- Bai, Y., Yao, Z., & Dou, Y. F. (2015). Effect of social commerce factors on user purchase behavior: An empirical investigation from renren.com. International Journal of Information Management, 35(5), 538–550. https://doi.org/https://doi.org/10.1016/j.ijinfomgt.2015.04.011
- Berthon, P. R., Pitt, L. F., Plangger, K., & Shapiro, D. (2012). Marketing meets Web 2.0, social media, and creative consumers: Implications for international marketing strategy. Business Horizons, 55(3), 261–271. https://doi.org/https://doi.org/10.1016/j.bushor.2012.01.007
- Chen, Y., & Xie, J. (2005). Third-Party product review and firm marketing strategy. Marketing Science, 24(2), 218–240. https://doi.org/https://doi.org/10.1287/mksc.1040.0089
- Chin, W. W., & Dibbern, J. (2010). An introduction to a permutation-based procedure for multi-group PLS analysis: Results of tests of differences on simulated data and a cross cultural analysis of the sourcing of information system services between Germany and the USA. In V. Esposito Vinzi, W. W. Chin, J. Henseler, & H. Wang (Eds.), Handbook of partial least squares (pp. 171–193). Springer.
- Cobb, S. (1976). Presidential address-1976. Social support as a moderator of life stress. Psychosomatic Medicine, 38(5), 300–314. https://doi.org/https://doi.org/10.1097/00006842-197609000-00003
- Euromonitor International. (2019). Digital disruptors: The global competitive landscape of social media. Euromonitor International.
- Farivar, S., Turel, O., & Yuan, Y. (2017). A trust-risk perspective on social commerce use: An examination of the biasing role of habit. Internet Research, 27(3), 586–607. https://doi.org/https://doi.org/10.1108/IntR-06-2016-0175
- Fornell, C., & Larcker, D. F. (1981). Evaluating structural equation models with unobservable variables and measurement error. Journal of Marketing Research, 18(1), 39–50. https://doi.org/https://doi.org/10.2307/3151312
- Gibreel, O., AlOtaibi, D. A., & Altmann, J. (2018). Social commerce development in emerging markets. Electronic Commerce Research and Applications, 27(1), 152–162. https://doi.org/https://doi.org/10.1016/j.elerap.2017.12.008
- Hair, J. F., Hult, G. T. M., Ringle, M., & Sarstedt, M. (2016). A Primer on partial least squares structural equation modeling. Sage Publications.
- Hajli, M. N. (2014b). A study of the impact of social media on consumers. International Journal of Market Research, 56(3), 387–404. https://doi.org/https://doi.org/10.2501/IJMR-2014-025
- Hajli, N. (2014a). The role of social support on relationship quality and social commerce. Technological Forecasting and Social Change, 87(7), 17–27. https://doi.org/https://doi.org/10.1016/j.techfore.2014.05.012
- Hajli, N. (2015). Social commerce constructs and consumer’s intention to buy. International Journal of Information Management, 35(2), 183–191. https://doi.org/https://doi.org/10.1016/j.ijinfomgt.2014.12.005
- Hajli, N., & Sims, J. (2015). Social commerce: The transfer of power from sellers to buyers. Technological Forecasting and Social Change, 94(5), 350–358. https://doi.org/https://doi.org/10.1016/j.techfore.2015.01.012
- Hajli, N., Lin, X., Featherman, M., & Wang, Y. (2014). Social word of mouth: How trust develops in the market. International Journal of Market Research, 56(5), 673–689. https://doi.org/https://doi.org/10.2501/IJMR-2014-045
- Hajli, N., Sims, J., Zadeh, A. H., & Richard, M. O. (2017). A social commerce investigation of the role of trust in a social networking site on purchase intentions. Journal of Business Research, 71(2), 133–141. https://doi.org/https://doi.org/10.1016/j.jbusres.2016.10.004
- Henseler, J., Ringle, C. M., & Sarstedt, M. (2016). Testing measurement invariance of composites using partial least squares. International Marketing Review, 33(3), 405–431. https://doi.org/https://doi.org/10.1108/IMR-09-2014-0304
- Henseler, J., Ringle, C. M., & Sinkovics, R. R. (2009). The use of partial least squares path modeling in international marketing. Advances in International Marketing, 20, 277–320. https://doi.org/https://doi.org/10.1108/S1474-7979(2009)0000020014
- Huang, Z., & Benyoucef, M. (2013). From e-commerce to social commerce: A close look at design features. Electronic Commerce Research and Applications, 12(4), 246–259. https://doi.org/https://doi.org/10.1016/j.elerap.2012.12.003
- Kim, S., & Park, H. (2013). Effects of various characteristics of social commerce (s-commerce) on consumers’ trust and trust performance. International Journal of Information Management, 33(2), 318–332. https://doi.org/https://doi.org/10.1016/j.ijinfomgt.2012.11.006
- Liang, T. P., Ho, Y. T., Li, Y. W., & Turban, E. (2011). What drives social commerce: The role of social support and relationship quality. International Journal of Electronic Commerce, 16(2), 69–90. https://doi.org/https://doi.org/10.2753/JEC1086-4415160204
- Liang, T. P., & Turban, E. (2011). Introduction to the special issue social commerce: A research framework for social commerce. International Journal of Electronic Commerce, 16(2), 5–14. https://doi.org/https://doi.org/10.2753/JEC1086-4415160201
- Lin, X., Li, Y., & Wang, X. (2017). Social commerce research: Definition, research themes and the trends. International Journal of Information Management, 37(3), 190–201. https://doi.org/https://doi.org/10.1016/j.ijinfomgt.2016.06.006
- Morgan, R. M., & Hunt, S. D. (1994). The commitment-trust theory of relationship marketing. Journal of Marketing, 58(3), 20–38. https://doi.org/https://doi.org/10.2307/1252308
- Mutz, D. C. (2005). Social trust and e-commerce: Experimental evidence for the effects of social trust on individuals’ economic behavior. Public Opinion Quarterly, 69(3), 393–416. https://doi.org/https://doi.org/10.1093/poq/nfi029
- Park, D. H., Lee, J., & Han, I. (2007). The effect of on-line consumer reviews on consumer purchasing intention: The moderating role of involvement. International Journal of Electronic Commerce, 11(4), 125–148. https://doi.org/https://doi.org/10.2753/JEC1086-4415110405
- Pavlou, P. A. (2003). Consumer acceptance of electronic commerce: Integrating trust and risk with the technology acceptance model. International Journal of Electronic Commerce, 7(3), 101–134. https://doi.org/https://doi.org/10.1080/10864415.2003.11044275
- Peštek, A., Resić, E., & Nožica, M. (2011). Model of trust in e-transactions. Economic Research-Ekonomska Istraživanja, 24(3), 131–146. https://doi.org/https://doi.org/10.1080/1331677X.2011.11517472
- Phua, J., Jin, S. V., & Kim, J. J. (2017). Uses and gratifications of social networking sites for bridging and bonding social capital: A comparison of Facebook, Twitter, Instagram, and Snapchat. Computers in Human Behavior, 72, 115–122. https://doi.org/https://doi.org/10.1016/j.chb.2017.02.041
- Ringle, C. M., Wende, S., & Becker, J. M. (2015). SmartPLS 3. SmartPLS.
- Rival, I. Q. (2020). 2020 social media industry benchmark report. https://www.rivaliq.com/blog/social-media-industry-benchmark-report/
- Sarstedt, M., Henseler, J., & Ringle, C. (2011). Multi-Group analysis in partial least squares (PLS) path modeling: Alternative methods and empirical results. Advances in International Marketing, 22(1), 195–218. https://doi.org/https://doi.org/10.1108/S1474-7979(2011)0000022012
- Sarulatha, N., & Sasirekha, V. (2018). A study on the role of social commerce constructs for shopping fashion products. International Journal of Civil Engineering and Technology, 9(1), 508–518.
- Shanmugam, M., Sun, S., Amidi, A., Khani, F., & Khani, F. (2016). The applications of social commerce constructs. International Journal of Information Management, 36(3), 425–432. https://doi.org/https://doi.org/10.1016/j.ijinfomgt.2016.01.007
- Shi, Y., Sia, C. L., & Chen, H. (2013). Leveraging social grouping for trust building in foreign electronic commerce firms: An exploratory study. International Journal of Information Management, 33(3), 419–428. https://doi.org/https://doi.org/10.1016/j.ijinfomgt.2013.02.001
- Soleimani, M., Danaei, H., Jowkar, A., & Parhizgar, M. M. (2017). Factors affecting social commerce and exploring the mediating role of perceived risk. Iranian Journal of Management Studies, 10(1), 63–90. https://doi.org/https://dx.doi.org/10.22059/ijms.2017.212223.672191
- Trkman, M., & Trkman, P. (2018). A framework for increasing business value from social media. Economic Research-Ekonomska Istraživanja, 31(1), 1091–1110. https://doi.org/https://doi.org/10.1080/1331677X.2018.1456355
- Wang, X., Lin, X., & Spencer, M. K. (2019). Exploring the effects of extrinsic motivation on consumer behaviors in social commerce: Revealing consumers’ perceptions of social commerce benefits. International Journal of Information Management, 45(2), 163–175. https://doi.org/https://doi.org/10.1016/j.ijinfomgt.2018.11.010
- Waterloo, S. F., Baumgartner, S. E., Peter, J., & Valkenburg, P. M. (2018). Norms of online expressions of emotion: Comparing Facebook, Twitter, Instagram, and WhatsApp. New Media & Society, 20(5), 1813–1831. https://doi.org/https://doi.org/10.1177/1461444817707349
- Zamrudi, Z., Suyadi, I., & Abdillah, Y, Universitas Brawijaya (2016). The effect of social commerce construct and brand image on consumer trust and purchase intention. Profit, 10(01), 1–13. https://doi.org/https://doi.org/10.21776/ub.profit.2016.010.01.1
Appendix:
Measurement scales
Social commerce constructs (Hajli, Citation2015)
Recommendations and referrals
I feel my friends’ recommendations in Facebook/Instagram are generally frank.
I feel my friends’ recommendations in Facebook/Instagram are generally reliable.
Overall, my friends’ recommendations in Facebook/Instagram are trustworthy.
I trust my friends in Facebook/Instagram and share my status and pictures with them.
Forums and communities
I feel my friends on forums and communities in Facebook/Instagram are generally frank.
I feel my friends on forums and communities in Facebook/Instagram reliable.
Overall, my friends on forums and communities in Facebook/Instagram are trustworthy.
I trust my friends on forums and communities and share my status and pictures with them in Facebook/Instagram.
Rating and reviews
I feel my friends’ rating and reviews are generally frank in Facebook/Instagram.
I feel my friends’ rating and reviews reliable in Facebook/Instagram.
Overall, my friends’ rating and reviews are trustworthy in Facebook/Instagram.
I trust my friends’ ratings and reviews and share my status and pictures with them in Facebook/Instagram.
Social support (Liang et al., Citation2011)
Emotional support
When faced with difficulties, some people on Facebook/Instagram are on my side.
When faced with difficulties, some people on Facebook/Instagram comforted and encouraged me.
When faced with difficulties, some people on Facebook/Instagram listened to me talk about my private feelings.
When faced with difficulties, some people on Facebook/Instagram expressed interest and concern in my well-being.
Informational support
On Facebook/Instagram, some people would offer suggestions when I needed help.
When I encountered a problem, some people on Facebook/Instagram would give me information to help me overcome the problem.
When faced with difficulties, some people on Facebook/Instagram would help me discover the cause and provide me with suggestions.
Trust (Hajli, Citation2014a)
The performance of Facebook/Instagram always meets my expectations.
Facebook/Instagram can be counted on as a good social networking site.
Facebook/Instagram is a reliable social networking site.
Social commerce intention (Liang et al., Citation2011)
I am willing to provide my experiences and suggestions when my friends on Facebook/Instagram want my advice on buying something.
I am willing to share my own shopping experience with my friends on Facebook/Instagram.
I am willing to recommend a product that is worth buying to my friends on Facebook/Instagram.
I will consider the shopping experiences of my friends on Facebook/Instagram when I want to shop.
I will ask my friends on Facebook/Instagram to provide me with their suggestions before I go shopping.
I am willing to buy the products recommended by my friends on Facebook/Instagram.