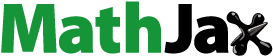
Abstract
Previous research presented in numbers of studies strongly suggests that locating in a cluster generates valuable benefits to clustered firms. These include better access to suppliers and other scarce inputs, superior knowledge and innovation, a better position from which to build a social network as well as proximate to successful competitors. Yet when the positive impact of agglomeration and clustering has been questioned on the strictly financial performance of clustered firms, the results of these studies are not so convincing and question the enthusiasm for cluster theory shown by scholars, practitioners, and policymakers. The aim of our research is to enrich existing knowledge concerning the benefits of clustering, as well as to test if localisation in a natural cluster, and membership in a cluster organisation has a positive impact on financial performance. We propose to measure that by selected financial indicators such as ROA, ROS, labour productivity and Economic Value Added, focussing our research on traditional industrial sectors – plastics and textiles seated in the Czech Republic. The results of analysing firm-level data in the period of 2009–2016 fail to confirm any significant influence of firm localisation in natural cluster or membership in the cluster organisation on financial performance for firms in studied sectors. We achieved the same results by investigation of potential differences for young firms, SMEs or underperforming firms.
1. Introduction
Global innovation and technological development, over the last few decades, have influenced the strategy of businesses. Collaboration within networks and clusters, considered by Ketels (Citation2012, p. 4) as ‘catalysts for accelerating industrial transformation and for developing new regional competitive advantages, speeding up firm and job creation and thereby contributing to growth and prosperity’ have been strongly supported to strengthen this development. It is the opinion of agglomeration scholars that the benefits of clustering lead to increased company performance derived from improved production or heightened demand (e.g., Krugman, Citation1991).
Cluster studies similarly focus on the benefits in the concept as agglomeration views. Scholars foster the assumption of positive returns to scale at the regional level, benefits from sources such as infrastructure, input access, and markets (e.g., Li & Geng, Citation2012; Nohria & Eccles, Citation1992). Co-location in an agglomeration has a positive influence on a firm’s innovative performance (Hervas-Oliver et al., Citation2018).
According to a number of economists (Enright, Citation1996; Fløysand & Jakobsen, Citation2001; Molina-Morales & Expósito-Langa, Citation2012; Porter, Citation1990; and others), participation in cluster activities may offer benefits in the form of economies of scale, reduced costs, transfer of technologies and R&D results, as well as increase of innovative potential, communicative power mainly of small and medium firms to push governments to care about specialised infrastructures, or generation of external scale effects.
Clusters have differing characteristics, such as their scope, history, participants, relationships, etc. The definition of ‘cluster’ varies in different studies. They are understood as ‘natural clusters’- shaped according to the definition of Porter (Citation1990, Citation1998, Citation2000), or they are described as ‘cluster initiatives’ or ‘organised clusters’ in the form of cluster organisations (e.g., Furman et al., Citation2002; Morgulis-Yakushev & Sölvell, Citation2017).
Using Porter‘s definition (2000, p. 16) ‘a cluster is a geographically proximate group of interconnected companies and associated institutions in a particular field, linked by commonalities and complementarities’, the benefits from agglomeration could be pointed out. A cluster organisation then means that firms, educational and research institutions, public bodies, based on the initiative of particular institutions (e.g., regional development agencies, universities, etc.) form an organised and managed legal entity. The cluster organisation (CO) plays a key role in strengthening and deepening relations between these actors through various types of ‘bridge-building’ activities which helps to meet pre-defined objectives (Lechner & Leyronas, Citation2012; Morgulis-Yakushev & Sölvell, Citation2017). Duranton (Citation2011, p. 33) states that ‘all the studies examined so far assess the effects of clustering when it occurs ‘naturally’. They do not constitute a direct assessment of cluster initiatives’.
There can be found results of empirical research questioning the ability of agglomeration economies to stimulate financial performance in the firms and the statements of the positive association between firm clustering and its performance in previous studies (e.g., Benneworth et al., Citation2003; Branco & Lopes, Citation2018; Kukalis, Citation2010; Martin & Sunley, Citation2003; Stavropoulos & Skuras, Citation2016). Kukalis (Citation2010) demonstrates no significant differences between clustered and non-clustered firms in the early stages of the industry life cycle. Further, he shows that non-clustered laggards outperformed clustered laggards in the late stages of the industry life cycle. Branco and Lopes (Citation2018) examined the firms’ economic performance in the cork industry depending on the stage of the cluster life cycle. They found that the cluster had the strongest impact on performance during its development. Conversely, at the initial and maturity stage of the cluster, the economic performance of cluster firms was not better than the national average of the industry. Stuart and Sorenson (Citation2003) investigate the effects of geographic proximity in the biotechnology industry. Their findings show that proximity effects tend to dissipate as the industry evolves. Pouder and St. John (Citation1996) point out possible limitations on innovative capabilities as well as the performance of cluster participants due to erosion of agglomeration benefits over time. These researchers suggested that the forces that draw firms together within clusters dissipate in due course just as the growth of manufacturing facilities may lead to dis-economies of scale.
According Johansson and Quigley (Citation2005), good company performance does not always result from clustering, as networks of actors can disperse over space and in this way to substitute geographical agglomeration of actors. Uzzi (Citation1997) explains why ‘over-embeddedness’ of clustered firms may result in a high risk of failure. Duranton (Citation2011) investigates small benefits from clustering on local earnings and productivity and negative role of clustering on innovation.
Kukalis (Citation2010, p. 3) mentions ‘that the theoretical views held by cluster scholars may rest more on case studies than on an examination of accurate performance measures at the firm level within the same industry’ and calls for additional empirical studies regarding the effect of clusters on financial performance.
The inconsistent results of previous research call for more empirical investigation in order to provide further evidence-based results. The aim of our research is to enrich existing knowledge of the benefits of clustering and test if firm location in a natural cluster, and membership in a cluster organisation have positive impacts on financial performance. We measure financial performance using selected financial indicators such as ROA, ROS, labour productivity, and value-based indicator Economic Value Added (EVA).
Contrary to previous research studies concentrated mostly on knowledge-based and publicly traded firms in highly innovative sectors (e.g., Audretsch et al., Citation2019; Audretsch & Keilbach, Citation2007; Kukalis, Citation2010; Stuart & Sorenson, Citation2003), we focus our research on traditional industry sectors with a high share of SMEs. We study three samples to be able to distinguish firms with different levels of hypothesised effects:
clustered firms in terms of the Porter's natural cluster,
firms-members of the cluster organisation (CO), and
non-clustered firms – isolated, but localised within the same country as natural cluster and CO.
The firm-level data of two sectors of traditional industries are examined in this study, in order to study more stable conditions within the industry life cycle. The sectors of plastics and textiles were selected for their long history and strong concentration in particular regions in the Czech Republic, with the more than 10 years existence of managed cluster organisations in the defined sector within this region, along with the presence of a significant number of non-clustered firms in the same sector. This allows the opportunity for sampling in this investigation, and to comprehensively study the influence of clustering on financial performance.
The remainder of the paper is organised as follows: after a comprehensive theoretical review in Chapter 2, the framework for data collection and research methods are described in Chapter 3. In Chapter 4, the results of analysis and hypotheses testing are introduced, followed by a discussion of the findings and conclusions in chapters 5 and 6.
2. Theoretical background
Over the last 30 years, clusters have been investigated in a number of studies. In the 1990s, a number of examples of industries in Italy, Switzerland, the USA, Germany and other countries were reported (Porter, Citation1998).
The problem of cluster is with its definition. Numerous researchers have tried to define the term ‘cluster’ using their own perspective (e.g., Cooke, Citation2002; Enright, Citation2003; OECD, Citation1999; Porter, Citation1990; Sölvell et al., Citation2003). There is no standard definition which has been agreed upon. The most of researches and also practitioners emphasise the geographical proximity of the firms and their different interactions on the horizontal and vertical level. The clusters link all important factors – available resources, individual goals to achieve competitive success, and share the idea of proximity, networking and specialisation. They stimulate cooperation in the business environment and competitive pressure, even between indirect competitors and non-competitive participants.
However, some authors dealt with the issue of spatial concentration of firms much earlier. Marshall (Citation1890) studied clustering of related industrial activities and emphasised natural conditions as the main reasons. Concentration of firms attracts educated labour, it is reflected in an increased level of invention and improvement of machinery, workflows and organisation, and resulted in savings from localisation and increased competitiveness of the industry in the area. The Marshall’s industrial district can be considered as a cluster precursor.
The cluster can arise in principle in two ways – as a result of natural market development or on the basis of the initiative of certain (usually) governmental institutions. The geographically concentrated enterprises, research, professional and educational institutions that cooperate, based on the Porter’s definition (Porter, Citation1990, Citation1998, Citation2000) in a particular region, without creating a new legal entity are perceived as a ‘natural cluster’. If a cluster is triggered by an institution, cluster initiative is talked about. Sölvell et al. (Citation2003, p. 9) defines cluster initiative as ‘organised efforts to enhance the competitiveness of a cluster, involving private industry, public authorities, and/or academic institutions’.
Ketels (Citation2007) declares the emerging of cluster initiatives as e.g., results from the projects with the possibility of conversion into formal structures. The successful cluster initiative can result in the foundation of a ‘cluster organisation ‘which could act as an entity with relations among firms, and also with participation of government, educational and research institutions or capital providers (e.g., Morgulis-Yakushev & Sölvell, Citation2017).
Clusters and cluster organisations can vary in size, breadth and state of development (Porter, Citation2000). The variances in their nature reflect the differences in the structures of industries. Some of them consist primarily of small and medium-sized enterprises, others involve both large and small firms and some have very closed relationships with research institutions and/or universities, while others have no such kind of connection. Clusters can exist with numerous common activities, with strong concentration of firms and related industries, or just a few activities in a given industry. It is possible to identify the science-driven innovative clusters and those in traditional sectors with a lower innovation capacity and ability (e.g., Enright, Citation1996, Citation2003; Ketels, Citation2007).
The impact of cluster existence (either natural or in the form of a cluster organisation) can be understood as a double. On the one hand, the cluster may impact the region, especially in its innovative performance (e.g., Delgado et al., Citation2014), while on the other hand, influence the individual entities that co-create the cluster or cluster organisation. Ketels (Citation2003) emphasises the cluster's benefits in three areas, (i) firms in the clusters are more efficient because they use specialised inputs and their suppliers are working with shorter reaction times than isolated businesses, (ii) firms and research institutions can achieve higher levels of innovation due to knowledge sharing and close interaction, (iii) thirdly, there is a tendency to cluster enlargement when setting up new businesses becomes easier because vendors and other partners are part of the cluster. Rosenfeld (Citation2002) divides benefits of clustering into hard and soft. The hard benefits result from more efficient business transactions, expert investment, cost reduction, and profits. The soft benefits lie in education, benchmarking, knowledge sharing, leading to innovation, imitation and improvement. Nie and Sun (Citation2015) consider cost reduction (e.g., for finding resources or customers) to be the strongest motive. Ridley (Citation2008) emphasises the easier or free access to information of market demand. Nie et al. (Citation2020) used a game theory to show that the cooperation of companies in the industry cluster improves their profits. Spencer et al. (Citation2010) show in their study that industries located in areas with acritical mass of related industries tend to higher incomes and rates of growth. Delgado et al. (Citation2014) assess the impact of clusters on employment growth and innovation in the region’s industries. The firms located in clusters have been found to show higher employment growth – this conclusion applies in different regions, industries and clusters. According Isaksen and Trippl (Citation2014), regional innovation system includes clusters for cross-sectoral knowledge sharing to foster renewal and regeneration.
The complementarity between employment and innovation performance in clusters and the positive role of strong clusters in the emergence of new industries in the region were also found. Delgado et al. (Citation2015) depict the role of clusters in the recession as also important, and strong clusters have been found to be resistant to the recession in 2007–2009.
Many clusters in Europe have been created with financial support from the Structural Funds and proving the effectiveness of such support started to be manifested as a hot topic. Barkley and Henry (Citation2001) report that clusters increase the efficiency of using public funds by concentrating them on supporting a limited number of business activities. At the same time, due to the close firm clustering, sector-specific support programs have a higher multiplier effect on the economy of the region. At the same time, they express a certain degree of scepticism as to whether national or local authorities are able to identify the competitive edge of the region at all, to select ‘right’ sectors to support or develop support programs for certain sectors.
Explanations of spatial clustering and positive association between firm clustering and its performance are being questioned by recent empirical evidence showing that firms in clusters fail to outperform firms outside clusters. Kukalis (Citation2010) demonstrates no significant differences between clustered and non-clustered firms in the early stages of the industry life cycle using thirty-one years of performance data for 194 publicly traded firms in the semiconductor and pharmaceutical industries. Further, he shows that non-clustered laggards outperformed clustered laggards in the late stages of the industry life cycle. Branco and Lopes (Citation2018) found that the impact of a cluster on economic performance depends on the stage of the cluster life cycle. They showed the strongest influence in the cluster development stage, when the economic performance of the cluster firms exceeded the national average in the industry (cork industry). On the contrary, in the initial stage of cluster formation, the economic performance of clustered companies was below average. In the stage of cluster maturity, the positive effect of the cluster has already been exhausted. It should be noted that their study covered a very long period of 60 years. The positive impact of the cluster on performance was identified after more than 20 years of cluster existence. Mo, He and Yang (Citation2020) found that innovation success depends on the size and type of cluster. Large traded clusters have a stronger positive impact on regional innovation through the sharing of services, labour, tacit knowledge, technology etc. than local clusters. The degree of cluster specialisation also has a positive effect on innovation. Stavropoulos and Skuras (Citation2016) studied a large sample of 410,000 manufacturing firms located across 191 NUTS 2 regions of 15 EU member states. On the basis of calculation of two profitability indicators and a labour efficiency indicator they find very small contribution to the part of the variance of these indicators attributed to the regions. According Pouder and St. John (Citation1996), concentrations of same-industry businesses may initially lead to high innovation and growth, but later these clusters could decline and influence the clustered firms’ performance. Murray and Dowell (Citation1999) draw attention to the dangers of cluster orientation for a specific product mix. Dependence on the production of specific products may lead to a significant decline in the economic level of the region in the event of crisis of the sector. A second danger lies in perceiving the unfair approach of the state to selected firms only. Cluster support logically leads to a selective advantage for cluster firms, while firms outside the cluster do not qualify for subsidies. Clustering can lead to congestion and competition effects in input markets, raising rents, materials and specialised labour (Baptista & Swann, Citation1998; Kukalis, Citation2010), that are mainly associated with mature and large clusters (Delgado et al., Citation2010). Vaan et al. (Citation2013), studying project-based industries on the example of the global video game industry, demonstrates a positive net effect of clustering after a cluster reaches a critical size. Kukalis (Citation2010) discusses that clustering can increase market competition if products are more homogeneous and/or locally consumed.
In contrast, Stojčić et al. (Citation2019) demonstrate the positive effect of cluster membership on corporate performance, labour efficiency, export performance and firm growth on the example of wood-processing and furniture clusters in Slovenia and Croatia. However, the authors come up with an interesting idea to try to find out to what extent companies actually use their membership in the cluster to increase their performance, or whether they are just formal members paying membership fees. Aranguren et al. (Citation2014) tested the effect of cluster membership on labour productivity in the Basque cluster environment in Spain in two periods (2002 and 2008). In their study, they first compared productivity in general in relation to membership. Here they concluded that there was a significant difference between the productivity levels of member companies and other companies in both periods. Cluster member companies had higher productivity. However, when they compared companies with similar characteristics (size, age, R&D, quality standards, industry, location), the weaker impact of cluster membership on productivity was demonstrated, and only in the second period.
Ben Abdesslem and Chiappini (Citation2019) examined labour productivity of French companies in the optic/photonic industry from 1996 to 2008 in relation to cluster membership. Cluster policy was implemented in France in 2005. The results of the research showed that after the introduction of cluster policy, the productivity of companies increased after only three years. At the same time, it was found that the beneficiaries of the support were rather larger companies with higher productivity. Cluster policy, on the other hand, has not led to an increase in cluster size. The companies of certified clusters were significantly more productive.
On the basis of theoretical background of scholars’ studies with inconsistent results, we aim to test theoretical propositions of expectation of the highest financial performance of firms localised in managed CO and the lowest of non-clustered firms. We define the following hypotheses:
H1: The financial performance of geographically localised firms in a natural cluster is higher than that of non-clustered firms.
H2: The financial performance of firms-members of the CO is higher than localised firms in natural cluster (non-members of CO).
Ruland (Citation2013) examined the effect of the company's headquarters position on profitability measured by the ROA indicator on US firms' 2004–2009 data. The results are not unambiguous and depend on the size of the company. For small businesses, a significant improvement in profitability was demonstrated for cluster-based firms. By contrast, for large firms, profitability was higher for firms outside the cluster. Li and Geng (Citation2012) study the sample of 294 Chinese SMEs. They give evidence of significantly higher perceptions of shared resources with cluster firms in comparison with non-clustered, and also demonstrate that shared resources exclusively available to cluster firms link to better cluster firm performance.
On the basis of the proposition that SMEs can benefit more from clustering, we define the following hypothesis:
H3: Clustered SMEs outperform non-clustered SMEs.
Sternberg and Litzenberg (Citation2004) discuss why young firms have tendency to cluster spatially more than older ones – as clusters can reduce barriers to entry industry due to availability of knowledge, skilled labour, social networks, etc. In opposition, Stuart and Sorenson (Citation2003) give evidence of worse performance of start-up firms in the biotechnology industry with proximity to dense biotechnology clusters than those in less-concentrated areas.
To study if young firms can benefit more from clustering, we define the following hypothesis:
H4: Young clustered firms outperform young non-clustered firms.
Shaver and Flyer (Citation2000) believe that firms with the best human capital, technologies, training programs, suppliers, etc. will gain less from the knowledge transfer, and rather lose their advantages in transferring knowledge to competitors. Kukalis (Citation2010) also states that superior firms do not benefit when their knowledge spills to weaker firms. Firms with worse technologies, human resources, etc., have nothing to lose by participating in a cluster, they can only gain. Thus, the best, most effective, firms will gain little in the cluster. To the contrary, the benefit for their weaker competitors can be considerable.
On the basis of these findings we define following hypothesis:
H5: Underperforming clustered firms outperform underperforming non-clustered firms.
3. Data and methods
3.1. Data and sampling
The aim of the research is to determine if location in a natural cluster, and the membership of a firm in a cluster organisation have positive impacts on financial performance. To examine the defined hypotheses and be able to identify differences in firm performance, three different samples are defined:
Members of a CO (CLU) are firms officially involved in the formal CO.
Geographically localised firms in natural clusters (REG) is a group of firms which are not members of CO but operate in the geographical location of the formal CO.
Finally, non-clustered firms (CZE) are firms which do not belong to either of previous groups.
Two different industries studied in the Czech Republic are examined for verification of the results: plastics and textiles. One country is chosen because of the same conditions in terms of macroeconomic factors and cluster policy. The industries are selected due to:
their strong concentration in particular regions in the Czech Republic – (A) plastics processing industry in Zlín region and South-Moravian region (NUTS3) and (B) textile industry in Bohemian northeastern region (Liberec + Hradec Králové+Pardubice region) (NUTS2) and northwestern Karlovarský region (NUTS3), and
within these regions, existence of more than 10 years’ old COs is possible to investigate (both of these clusters were established in 2006): PLASTR (plastics processing), CLUTEX (textile).
The textile industry has been historically one of the traditional sectors in the Czech Republic. Since 1990s until roughly late 2000s, this industry went through a phase of decline in employment and performance indicators due to the inability to compete with traditional production of cheap Asian competition. However, the situation in the monitored period 2009–2016 can be considered stable. The industry has been restructured, and existing manufacturers are mainly focussing on higher value-added production such as technical textiles for automotive and apparel design. The main centre of the textile industry is a natural cluster in the northern part of the Czech Republic with several research and educational institutions. This explains why companies in the natural textile cluster performed better than companies in other regions. Only a small number of firms have joined the cluster organisation, often with weaker performance, as an opportunity to survive in the market.
Plastics production has a significant share of the country GDP and value added and the development of this sector in the monitored period 2009–2016 can be described as growing. The plastics industry generally has better financial performance results than the textile industry. There natural cluster in the southeastern part of the Czech Republic represents, on average, firms with the highest performance within this sector, supported by universities and research centres in this region. Firms with poorer performance became members of the cluster organisation, similarly to the textile industry.
3.2. Measures
3.2.1. Dependent variables
The measure of firm performance is treated as a dependent variable. There is discussion about appropriate performance indicators. In the case of agglomeration studies, choice depends on assumptions and limitations relevant to research.
Accounting-based ratios represent the most simple approach as ratios related to profitability, e.g., Return-on-Assets (ROA) or Return-on Sales (ROS) are used in the study of agglomeration economies, since they measure the earning capability of firms and provides supplementary and less biased indicator of profitability (Diez-Vial, Citation2011; Ferreira et al., Citation2010; Jennen & Verwijmeren, Citation2010; Kukalis, Citation2010). These financial ratios are not without limitations as they are calculated using numbers from financial statements influencing by company-specific accounting policies.
In our research we used hard firm-level financial data and calculated the accounting-based financial indicators: Return on assets (ROA), Return on sales (ROS) and labour productivity (LP) for their simplicity, frequent usage and justification in previous studies. One value-based indicator as a complex measure, Economic Value Added (EVA) which includes firm’s cost of capital and enables to include undertaken risk into evaluation of firm performance, is also employed. ROA evaluates firm’s effectiveness in utilising the firm’s assets and is measured as net income/total assets. ROS measures profit margin and is calculated as net income/sales. LP is calculated as sales/number of employees. EVA is calculated as a difference of the net operating profit after taxes and cost of capital, measures the extent to which the firm contributed by its activities in the given period to the increase or decrease of value for the owners:
We applied this measure in simplified form of residual income using accounting-based data:
(1)
(1)
where: ROE – return-on-equity, E – equity, re – cost of equity
To be able to compare firms‘performance, we calculated EVA/Sales. Capital Assets Pricing Model (CAPM) was applied for calculation of the cost of equity:
(2)
(2)
where: rf – risk-free rate, β – measure of systematic risk of a security, (rm – rf) – market risk premium
10-year Treasury bond yields, published by Czech Ministry of Industry and Trade, were considered as risk-free rates (rf). CAPM modified version was applied due to the absence of capital market data, and the values of industry unlevered betas were converted to levered beta for each firm based on its debt/equity ratio. Unlevered betas and country risk premiums (rm – rf) were taken over from Aswath Damodaran’s database. The risk-free rates, tax rates and risk premiums for the Czech Republic in the period of 2009–2016 are shown in .
Table 1. Risk-free rates, tax rates and risk premiums in the Czech Republic (2009–2016).
Financial data and number of employees in the period of 2009–2016 for calculation of all variables were drawn from The MagnusWeb database (Bisnode, Citation2017). The reporting period began in 2009 as the third year of COs‘existence and finished in 2016 as the last year with complete firms‘financial data.
3.2.2. Independent variables
Industry cluster and number of firms present in each cluster (cluster size) were designated as the independent variables. To identify a scope of natural clusters in the regions, this study used the results of a mapping study from 2004 to 2005 (Berman Group, Citation2005). The mapping study applied the following methodology:
the sectors in which the region has a key competence and are important for the region’s economy were identified,
expert assessment (leaders of industry, development agencies, universities) of the importance of the industry to the region and the potential to form a cluster organisation in the industry was provided,
a qualitative analysis of services that in themselves represent potential for cluster development in the region was processed, and
evaluation of Porter's diamond elements in individual branches (conditions of input factors, demand, firm strategy, structure and competition, related and supporting industries) was elaborated.
The quantitative part relied on approaches that use location quotients (LQs) (Isserman, Citation1977) to identify the concentration of the industry in the region as the basic assumption of cluster formation. The location quotient measures industry concentrations in a region working with employment data. It refers to the ratio of an industry’s share of employment in a particular region to its national share of employment.
LQ values in reporting period of 2009–2016 are presented in . The two investigated regions show a significant concentration of plastics and textile industries.
Table 2. Location quotients of plastics and textile industries in the Czech Republic.
The particular samples investigated are based on a list of firms in plastic and textile sectors. To properly identify the samples, the business focus of firms operating in selected sectors were studied in detail. Only the firms with predominant business activities in plastic/textile sectors were included.
The plastics industry’s samples consist of firms in NACE 222:
CLU includes members of the cluster organisation PLASTR established in 2006. It has members with various subjects of activity. Its members are, in addition to plastics processing firms, also trading firms, universities, research institutes and professional associations. Based on the analysis of the core business of cluster members, the core firms of the cluster were identified.
REG includes plastic processing firms seated in the boundaries of Zlín region and South-Moravian region (NUTS3), except the members of PLASTR.
CZE contains firms that operate within the Czech Republic in plastic processing industry out of sample REG.
The firm-level data had to be filtered. The firms with no sales data available in any year, and the micro-enterprises with sales below EUR 700,000 in all years were excluded from the sample. Then firms with missing data on revenues in some years in the time series, if at the same time in the remaining years the value of sales was below EUR 700,000, were also removed. ROE and EVA make sense to calculate only for the firms with positive equity. The firms with negative equity and EBITDA < 0 were excluded in all samples investigated. If evidence of the number of employees were missing and only the interval category was listed, the centre of the interval was applied. The samples of plastics processing firms (before and after filtering) in the period of 2009–2016 are presented in .
Table 3. Numbers of plastics processing firms in the period of 2009–2016.
The textile industry’s samples consist of firms in NACE 132, 139 and 141:
CLU includes members of the cluster organisation CLUTEX established in 2006. It has members with various subjects of activity. Its members are, in addition to textile firms, also trading firms, universities, research institutes and professional associations. Based on the analysis, the core firms of the cluster were identified.
REG includes firms operating in the region of Liberec, Hradec Králové and Pardubice (NUTS2) and Karlovarský region, except the members of CLUTEX.
CZE contains firms that operate within the Czech Republic in textile industry out of sample REG.
The samples of textile firms (before and after filtering) in the period of 2009–2016 are presented in .
Table 4. Numbers of textile firms (before and after filtering) in the period of 2009–2016.
To test hypotheses H3 (concerning SMEs), H4 (young firms) and H5 (underperformed firms) we brought together firms in the samples of CLU and REG, e.g., ‘clustered firms’ because of the small number of firms in both samples.
3.2.3. Hypotheses testing
The paper aims to investigate the magnitude and strength of the relationship between a dependent variable and a set of covariates. Therefore, research hypotheses were operationalised and consequently tested by employing statistical tests of corresponding regression parameters. Linear mixed effect regression (LMER) models with time-dependent variables were chosen for two reasons. Firstly, LMER distinguishes between general effects (fixed effects) applicable to all firms (units of analysis) and individual effects observed for each firm (random effects). Secondly, LMER allowed us to control for time dynamics. Models with both random intercepts and slopes capture different levels at the beginning of the analysed period and a pace of change of the dependent variable for each firm.
Formally, models can be written in the following form:
(3)
(3)
is a value of performance indicators of the
firm observed at the time
Parameter γ is an intercept value and corresponds to the expected value of the dependent variable
at the beginning of the analysis (
) for the reference category. This category differs across hypothesis and is described in the section Results. Vector of regression coefficients α contains parameters which correspond to the data matrix X. This matrix specifies levels to which a firm belongs to (CLU, REG, CZE) using binary dummy variables. This matrix is designed according to the purpose of each hypothesis. If the hypothesis is concerned only about the difference between CLU and common group REG + CZE, as it is in the RH3, only α1 will be estimated. Parameter α1 is interpreted as an effect of not being in the cluster organisation. Parameters α1 and α2 and are estimated if all three levels of cluster memberships are being compared. Parameter β describes the effect of being the textile firm. Parameters b define the random-effect part of the model. The b0i is a value of the random intercept (estimated value of the dependent variable at the beginning of the analysed period) of the firm i. Part b1i t allows setting a growth rate over time t for an individual firm i. Error value term uit follows a multivariate normal distribution (for each firm at a time) and contain effects of unobserved variables and other factors not captured by the model. Existence of the hypothesised effect was evaluated by the formal inferential t-test and profiled 95% confidence intervals (CI95). Confidence intervals allowed us to depict the uncertainty of the estimate.
Some models were not identified during the first step due to the high variance of the data. Therefore, we have treated data as a collection of cross-sectional data and have discarded the time-dimension of the analysis in the second step.
The non-parametric Mann-Whitney test was used to determine if any difference in the medium values of the dependent variable between two groups of firms exists. If more groups were analysed, the non-parametric Kruskal-Wallis analysis of variance (KW test) for one factor was performed. This test was accompanied by post-hoc pairwise tests to identify treatment groups with different median values of the analysed performance indicator. Dunn’s all-pair test allows controlling alpha error multiplication errors by Bonferroni's p-adjustment. All computations were done in the statistical software R 3.5.1 (R Core Team, Citation2018). Data manipulation and visualisation was done with library tidyverse Wickham et al. (Citation2019). Regression models were estimated using the library lme4 (Bates et al., Citation2015).
4. Results
In order to reveal the specific impact of cluster organisations on the performance of their members, it is necessary to analyse the initial situation in the year of COs establishment. Firm performance in the particular samples is evaluated using a median value of indicators – ROA, ROS and EVA/Sales.
shows that firms entering CLUTEX (in 2006) in average underperform firms localised in a natural cluster as well as non-clustered firms operating in the same industry in all reported financial indicators. The firms entering PLASTR show higher performance measured by financial indicators than firms operating outside the region, but the firms in natural cluster that were not members of the CO outperformed all firms in this industry.
Table 5. Firm performance in particular samples in 2006 (the year of COs establishment).
demonstrates initial different conditions in the two industries concerning relationships between the level of financial performance of COs‘members – firms localised in natural clusters – firms outside of the region at the time when the COs were established.
4.1. Results of hypotheses testing
The first two research questions were empiricaly verified by a regression model detailed in the EquationEquation (4)(4)
(4) .
H1: The financial performance of geographically localised firms in a natural cluster is higher than that of non-clustered firms.
H2: The financial performance of firms-members of CO is higher than localised firms in a natural cluster (non-members of CO).
A set of regression models with different dependent variables was estimated. However, due to the estimation problems (estimator has not converged to a precise solution), only regression models with dependent variable labour productivity (LP) and Return on Assets (ROA) were identified. Estimated parameters accompanied by inferential statistics and 95% confidence intervals can be found in and .
(4)
(4)
Table 6. Regression model of the LP dependent variable.
Table 7. Regression model of the ROA dependent variable.
The reference group for both the H1 and H2 is the ‘geographically localised firms in natural clusters’ (REG) operating in the Plastics group. This model does not assume that benefit of being in CLU or CZE compared to REG differs in the plastics and textile industry (there is no interaction between the geographical and sectoral attributes. Being a CLU compared to other categories gives the same benefit for plastics and textile group). Setting a reference group to REG allows to contrast effects of being a firm in the region (REG) to an effect of being a member of a narrower set of clustered firms (CLU) and wider set of companies in the Czech Republic (CZE). Research hypothesis 1 expects that the effect of not being either a member of the cluster organisation or natural cluster is negative compared to the REG category.
Therefore, parameter CZE is expected to be negative. In both models ( and ) we failed to find any evidence supporting this claim. In the Labour productivity model, the 95% confidence interval CI95 (–56.33, 917.38) spans over null effect and the t-test is statistically non-significant with
In the ROA model, the confidence interval CI95 (–0.02, 0.03) is inconclusive as it contains both positive and negative values of possible effect. The t-test of the regression parameter CZE yielded to
The second research hypothesis predicts that the effect of being organised in the cluster organisation (CLU) compared to the reference REG category is positive. Therefore, parameter CLU is expected to be positive. As with the H1, we failed to find any supportive empirical evidence. In the Labour productivity model (), the 95% confidence interval CI95 (–710.58, 1045.52) contains possible zero effect. This is confirmed by the t-test; t(414.35) = 0.38, p = 0.71. In the ROA model the confidence interval CI95 (–0.07, 0.01) of the regression parameter contains null effect and the t-test results in t(354.29) = −1.67, p = 0.1.
A series of KW tests (for both PL and ROA variables) were performed (see annexe) to see whether there was any difference in any year of the analysed periods. KW tests have not revealed any differences in LP. There were 4 years when the KW test identified differences in median values of ROA. In 2010 Reg > CLU (contradicts H1); in 2011 Reg > CLU (contradicts H1), 2015 CZE > REG and CZE > CLU (contradicts H1 and H2). These results do not resemble any clear pattern and in some cases even contradict theoretical expectations. This is consistent with an interpretation of the LMER models which have not found any systematic evidence for supporting hypotheses H1 and H2.
H3: Clustered SMEs outperform non-clustered SMEs.
The third hypothesis classifies companies into two groups according to their participation in the cluster activities. The CZE group is used as a reference category. Being a member of the combined category of REG + CLU is expected to have a positive effect on the FP when contrasted to the CZE firms. The CZE group is used as a reference category as the evaluation of the effect of the clustered SMEs (CLU + REG) is an objective of the hypothesis.
Only one full LMER model with dependent variable ROS (see ) was estimated. Another LMER model with ROA as a dependent variable was identified when grouping variable (effect of the sector) was dropped. Estimates of the simplified model can be found in .
Table 8. Regression model of the ROS dependent variable.
Table 9. Regression model of the ROA dependent variable.
The ROS model provided a negative estimate of the parameter of the joint group (CLU + REG). This estimate contradicts the expectation in the H3. Confidence interval CI95 (–0.03, 0.01) suggests that the effect of being a member of the joint group can be either negative and negative. This is supported by the t-test of the regression parameter t(df = 414.88) = −1.22, p = 0.22. There is no empirical evidence that firms in the joint group (CLU + REG) outperform other companies.
The simplified model points in the same direction. Effect of firms in CLU + REG is no different from as the confidence interval CI95 (–0.03, 0.01) suggests. Therefore our findings do not support H3 when ROA performance indicator is concerned. Formal t-test of the regression coefficient failed to reject the null hypothesis about the null effect as well: t(df = 377.25) = −1.12, p = 0.26.
Series of the Mann-Whitney tests was computed to verify results from regression models on annual data cross-sectional data. Results confirm the outcomes of regression models as no differences in medium values were identified.
H4: Young clustered firms outperform young non-clustered firms.
The fourth research hypothesis is evaluated on the sub-sample of young companies (established in or after the year 2011). CZE firms from the subsample are set as a reference category. Three models (LP, ROA and ROS) were identified to verify the H3. The first model with Labour Productivity as a dependent variable (see ) does not provide any empirical evidence which would support H3. Confidence interval CI95 (–1993.41, 693.31) contains the null effect. T-test of the regression parameter also fails to reject its null hypothesis with t(df = 112.17) = −0.95, p = 0.35. Surprisingly, the effect of textile sectoral is positive and reaches a high value 1887.26. On the other hand, standard error of the estimate is large which projects into a wide width of the confidence interval.
Table 10. Regression model of the LP dependent variable on the young firm subsample.
The second LMER model (see ) with financial performance indicator ROA supports findings from the Labour productivity model. The confidence interval of the regression parameter CI95 (–0.09. 0.01) contains the null effect value. The t-test t(df = 101.23) = −1.59, p = 0.11 also brings no evidence for conjecture that younger clustered firms outperforming other young firms.
Table 11. Regression model of the ROA dependent variable.
The LMER model with the ROS dependent variable (see ) was identified without the group-sector variable. The model finds that the membership in the CO or being localised in the natural cluster bring the negative effect on the ROS. This can be read either from the confidence interval CI95 (–0.08, −0.01) which contains only negative values, or from the t-test of regression parameter t(df = 103.64) = −2.58, p = 0.01. These findings contradict the H4. Although Mann-Whitney tests were computed for all dependent variables for which we have a regression model identified, none of the tests identified a statistically significant result.
Table 12. Regression model of the ROS dependent variable.
H5: Underperforming clustered firms outperform underperforming non-clustered firms.
Only one model with dependent variable ROA was identified. The reference group was set to CZE firms as the research hypothesis aim to identify the effect of firms participating in cluster activities. We split the sample into under- and out-performing firms according to the mean value threshold.
Only a model with ROA dependent variable was identified successfully (see ). The results suggest that the effect of being in a group CLU + REG can be both positive and negative with narrow confidence interval CI95 (–0.01, 0.01) and the corresponding t-test with t(df = 293.23) = −0.05, p = 0.96.
Table 13. Regression model of the ROA dependent variable.
5. Discussion
Many scholars describe the positive effects of clustering on company development. Most of them focus on inputs or market access, cost reduction, or innovation improvements, using more case studies than hard data. Just several studies try to question impacts of clustering on company financial performance. To study these impacts, scholars test the samples of clustered and non-clustered firms (e.g., Ben Abdesslem & Chiappini, Citation2019; Branco & Lopes, Citation2018; Kukalis, Citation2010; Li & Geng, Citation2012; Stojčić et al., Citation2019). The results of these studies are ambiguous. Some confirm the clearly positive impact of clustering on performance (Ben Abdesslem & Chiappini, Citation2019; Li & Geng, Citation2012; Stojčić et al., Citation2019). Others, on the other hand, report that clustering contributes to performance only at certain stages of the life cycle (Aranguren et al., Citation2014; Branco & Lopes, Citation2018) or not at all (Kukalis, Citation2010). Under Czech conditions, such a study was carried out for the first time.
Our research attempted to study company performance more deeply – to distinguish firms (i) organised in the CO, (ii) localised in so-called natural cluster, and (iii) non-clustered firms. We expected that firm-members of the CO with managed activities would outperform firms in natural clusters, and firms in natural clusters outperform non-clustered firms. To be able to smooth the influence of industry life cycles, we concentrated on firms operating in traditional industries, as well as with a high share of SMEs to fulfil the research gap, as most studies focus their research on publicly traded large companies in innovative sectors (e.g., Kukalis, Citation2010; Lechner & Leyronas, Citation2012; Stuart & Sorenson, Citation2003).
We expected that this scope of research could serve the propositions to meet expectations. Based on the research conducted, no positive impact of clustering on financial performance of the firms in plastic and textile industries in the Czech Republic was confirmed. The differences between the performance of all three samples were not statistically significant at the 5% level. These results did not confirm the positive association between firm clustering and its performance, in contrast with the findings of the studies by Aranguren et al. (Citation2014) or Stojčić et al. (Citation2019).
We observed differences in firms‘performance level between textile and plastics industries when COs were established – textile firms entering the CO in 2006, on average, underperformed firms localised in natural clusters as well as non-clustered firms operating in the same industry. In all reported financial indicators, plastic firms entering the CO in the same year showed higher performance than firms operating outside the region, and the firms in natural clusters that were not members of the CO outperformed all firms in this industry. The results of our research failed to show changes in financial performance by clustering.
Even though we particularly tested SMEs, young or underperformed firms in the samples, no differences between clustered and non-clustered firms’ financial performance were displayed, however some studies (e.g., Shaver and Flyer, Citation2000; Sternberg and Litzenberg, Citation2004; Kukalis, Citation2010) suppose that weaker firms, due to age, size or underperforming, could benefit from clustering more than strong ones.
In both cases, we examined clusters established in 2006 and assumed that the positive effect of the existence of a cluster organisation on performance should be demonstrated within a time horizon of 10 years. However, some research studies (e.g., Branco & Lopes, Citation2018) show that the positive effect of clustering on economic performance can manifest itself with a significantly longer time lag (in this case, after 20 years of the cluster's existence). Thus, it cannot be confirmed that support for the emergence of clusters in the Czech Republic in these sectors was meaningless or would not be effective in the longer term. Cluster membership is also just one factor that can contribute to firms’ performance growth and can be closely linked to other factors (e.g., R&D policy), as shown by the study of Aranguren et al. (Citation2014).
The problem of the Czech cluster organisations, in addition to a shorter period of existence, is also their small size. The results of no evidence of clustering effect on firm performance in this study can be aligned with results of Delgado et al. (Citation2010) showing clustering effects associated more with mature or large clusters, and also with Mo, He and Yang (Citation2020) confirming intensification of clustering effects with increasing size.
In terms of implications for economic policy, evaluation the efficiency of the cluster policy should take place in the long term. However, it is possible to continuously examine the development of cluster activities from the perspective of management, services provided, internationalisation, shared infrastructure or collaborative research (see Pavelková et al., Citation2019) and create a system of accredited clusters for which financial support appears to be potentially promising. In France, for example, it was proved that certified clusters have a greater impact on labour productivity growth (Ben Abdesslem & Chiappini, Citation2019) than others. Training of cluster management and cluster stakeholders in the regions through accredited certification centres also seems to be a suitable tool for supporting clusters. To reduce the risk of failure, the cluster policy could follow examples of good practice from abroad in the next period (e.g., U.S. Cluster Mapping, or Global Cluster Initiative Survey supported by the Swedish Agency for Innovation Systems).
6. Limitation of research and conclusion
We are aware of the limits of our research, especially the length of the 2009 to 2016 time series. Both of the investigated COs were founded in 2006. When examining the impact of the COs on the performance of member entities, a certain time lag must be considered, and we began the collection of data in 2009. The end of the time series is affected by the availability of data acquired from financial statements. In the case of both of the investigated cluster organisations, the results may have been influenced by the relatively short period of their operation.
Another limitation is the limited amount of data in the samples, mainly in the sample of the firm-members of the COs as we only used firms’ data (no other institutions-members of CO) and COs are relatively small. As Vaan et al. (Citation2013) declares, the effects of clustering could become positive after a cluster reaches a critical size. Moreover, we had to exclude some firms from investigation due to the non-availability of data, or negative value of their equity capital.
Targeting research into two industries also has certain limits, as it is not possible to generalise the results across all sectors. The scope of the study focussed on traditional industries raises questions of future study for possible conflicting results of firms’ performance for more innovative industries, based on the conceptual framework as our research.
To overcome these limitations, future research with longer time series data, studying COs with higher number of firms-members, and targeting more industries is recommended. The comparison of results for industries with high and moderate innovative capacity within the same country’s conditions with stronger and more influential cluster policy can be interesting for obtaining comprehensive knowledge of clustering benefits.
The explanations of spatial clustering have been questioned by recent empirical evidence showing that firms in clusters fail to outperform firms outside clusters. Several studies using firm-level data in different industries have been provided, and as e.g., Kukalis (Citation2010), Vaan et al. (Citation2013) and others, we propose that our findings may be driven by the particularities of the industrial settings chosen in our research.
The results of our study contributes to questioning the positive association between firm clustering and performance (e.g., Kukalis, Citation2010; Martin & Sunley, Citation2003; Stavropoulos & Skuras, Citation2016). The results of this study inspire the raising of more questions and research connected with studying effects of agglomeration, as well as also a focus on issues of clustering support by government and regional policies and its impact on firms’ performance as studied e.g., by Ben Abdesslem and Chiappini (Citation2019) in the French optic/photonic industry.
In further research, it is necessary to focus on monitoring these two industries in the coming years, as experience of e.g., Branco and Lopes (Citation2018) shows that the positive effect of clustering can occur with long time interval. It will also be desirable to include other industries in the research, as some researches (e.g., Žižka, Citation2019) suggest that the effect of clustering is differently strong in individual industries.
Disclosure statement
No potential conflict of interest was reported by the authors.
Additional information
Funding
References
- Aranguren, M. J., de la Maza, X., Parrilli, M. D., Vendrell-Herrero, F., & Wilson, J. R. (2014). Nested methodological approaches for cluster policy evaluation: An application to the Basque Country. Regional Studies, 48(9), 1547–1562. 10/ggrd95 https://doi.org/https://doi.org/10.1080/00343404.2012.750423
- Audretsch, D. B., & Keilbach, M. (2007). The theory of knowledge spillover entrepreneurship. Journal of Management Studies, 44(7), 1242–1254. https://doi.org/https://doi.org/10.1111/joms.2007.44.issue-7
- Audretsch, D. B., Lehmann, E. E., Menter, M., & Seitz, N. (2019). Public cluster policy and firm performance: Evaluating spillover effects across industries. Entrepreneurship & Regional Development, 31(1–2), 150–165. https://doi.org/https://doi.org/10.1080/08985626.2018.1537153
- Barkley, D. L., & Henry, M. S. (2001). Advantages and disadvantages of targeting industry clusters. Clemson University Public Service Activities.
- Baptista, R., & Swann, P. (1998). Do firms in clusters innovate more? Research Policy, 27(5), 525–540. https://doi.org/https://doi.org/10.1016/S0048-7333(98)00065-1
- Bates, D., Mächler, M., Bolker, B., & Walker, S. (2015). Fitting linear mixed-effects models using lme4. Journal of Statistical Software, 67(1), 1–48. https://doi.org/https://doi.org/10.18637/jss.v067.i01
- Ben Abdesslem, A., & Chiappini, R. (2019). Cluster policy and firm performance: A case study of the French optic/photonic industry. Regional Studies, 53(5), 692–705. https://doi.org/https://doi.org/10.1080/00343404.2018.1470324
- Benneworth, P. A. U. L., Danson, M. I. K. E., Raines, P. H. I. L., & Whittam, G. (2003). Confusing clusters? Making sense of the cluster approach in theory and practice. European Planning Studies, 11(5), 511–520. https://doi.org/https://doi.org/10.1080/0965431032000088470
- Berman Group. (2005). Identifikace klastrů v regionech ČR. Author.
- Bisnode. (2017). Magnusweb: Komplexní informace o firmách v ČR a SR. Online database. Bisnode CR. Retrieved January 8, 2018, from https://magnusweb.bisnode.cz
- Branco, A., & Lopes, J. C. (2018). Cluster and business performance: Historical evidence from the Portuguese cork industry. Investigaciones de Historia Económica, 14(1), 43–53. 10/ggdkzm https://doi.org/https://doi.org/10.1016/j.ihe.2016.05.002
- Cooke, P. (2002). Knowledge economies: Clusters, learning and cooperative advantage. Routledge.
- Delgado, M., Porter, M. E., & Stern, S. (2010). Clusters and Entrepreneurship. Journal of Economic Geography, 10(4), 495–518. https://doi.org/https://doi.org/10.1093/jeg/lbq010
- Delgado, M., Porter, M. E., & Stern, S. (2014). Clusters, convergence, and economic performance. Research Policy, 43(10), 1785–1799. https://doi.org/https://doi.org/10.1016/j.respol.2014.05.007
- Delgado, M., Porter, M. E., & Stern, S. (2015). The resilience of clusters: Evidence from the great recession. Kauffman Foundation Conference Center. Retrieved January 9, 2018, from https://www.industrystudiesconference.org
- Diez-Vial, I. (2011). Geographical cluster and performance: The case of Iberian ham. Food Policy, 36(4), 517–525. https://doi.org/https://doi.org/10.1016/j.foodpol.2011.04.002
- Duranton, G. (2011). California dreamin’: The feeble case for cluster policies. Review of Economic Analysis, 3(1), 3–45.
- Enright, M. (1996). Regional clusters and economic development: A research agenda. In U. Staber, N. Schaefer, & B. Sharma (Eds.), Business networks: Prospects for regional development (pp. 190–214). De Gruyter.
- Enright, M. J. (2003). Regional clusters: What we know and what we should know. In J. Bröcker, D. Dohse, & R. Soltwedel (Eds.), Innovation clusters and interregional competition (pp. 99–129). Springer-Verlag.
- Ferreira, F. C. M., Goldszmidt, R. G. B., & Csillag, J. M. (2010). The regional concentration of industries and the performance of firms: A multilevel approach. BAR – Brazilian Administration Review, 7(4), 345–361. https://doi.org/https://doi.org/10.1590/S1807-76922010000400003
- Fløysand, A., & Jakobsen, N. (2001). Clusters, social fields, and capabilities: Rules and restructuring in Norwegian fish-processing clusters. International Studies of Management & Organization, 31(4), 35–55. https://doi.org/https://doi.org/10.1080/00208825.2001.11656826
- Furman, J. L., Porter, M. E., & Stern, S. (2002). The determinants of national innovative capacity. Research Policy, 31(6), 899–933. https://doi.org/https://doi.org/10.1016/S0048-7333(01)00152-4
- Hervas-Oliver, J. L., Sempere-Ripoll, F., Rojas Alvarado, R., & Estelles-Miguel, S. (2018). Agglomerations and firm performance: Who benefits and how much? Regional Studies, 52(3), 338–349. https://doi.org/https://doi.org/10.1080/00343404.2017.1297895
- Isaksen, A., Trippl, M. (2014). Regional industrial path development in different regional innovation systems: A conceptual analysis. No 2014/17. Papers in Innovation Studies. Lund University, CIRCLE – Center for Innovation, Research and Competences in the Learning Economy. Retrieved January 24, 2019, from https://EconPapers.repec.org/RePEc:hhs:lucirc:2014_017
- Isserman, A. M. (1977). The location quotient approach to estimating regional economic impacts. Journal of the American Institute of Planners, 43(1), 33–41. https://doi.org/https://doi.org/10.1080/01944367708977758
- Jennen, M., & Verwijmeren, P. (2010). Agglomeration effects and financial performance. Urban Studies, 47(12), 2683–2703. https://doi.org/https://doi.org/10.1177/0042098010363495
- Johansson, B., & Quigley, J. M. (2005). Agglomeration and networks in spatial economies. Papers in Regional Science, 83(1), 165–176. https://doi.org/https://doi.org/10.1007/s10110-003-0181-z
- Ketels, C. (2003). The development of the cluster concept – Present experiences and further development. U.S. Source: Cluster Mapping. Retrieved March 12, 2019, from https://clustermapping.us/resource/development-cluster-concept-present-experiences-and-further-developments
- Ketels, C. (2007). Industrial policy in the United States. Journal of Industry, Competition and Trade, 7(3–4), 147–167. https://doi.org/https://doi.org/10.1007/s10842-007-0017-7
- Ketels, C. (2012). The impact of clusters and networks of firms on EU competitiveness. Final Report: Firm networks. Specific Contract No 605748. Retrived March 10, 2019, from http://www.clustercollaboration.eu/documents
- Krugman, P. (1991). Geography and trade. MIT Press.
- Kukalis, S. (2010). Agglomeration economies and firm performance: The case of industry clusters. Journal of Management, 36(2), 453–481. https://doi.org/https://doi.org/10.1177/0149206308329964
- Lechner, C., & Leyronas, C. (2012). The competitive advantage of cluster firms: The priority of regional network position over extra-regional networks – A study of a French high-tech cluster. Entrepreneurship & Regional Development, 24(5–6), 457–473. https://doi.org/https://doi.org/10.1080/08985626.2011.617785
- Li, J., & Geng, S. (2012). Industrial clusters, shared resources and firm performance. Entrepreneurship & Regional Development, 24(5–6), 357–381. https://doi.org/https://doi.org/10.1080/08985626.2011.591841
- Marshall, A. (1890). Principles of economics. MacMillan.
- Martin, R., & Sunley, P. (2003). Deconstructing clusters: Chaotic concept or policy panacea? Journal of Economic Geography, 3(1), 5–35. https://doi.org/https://doi.org/10.1093/jeg/3.1.5
- Mo, C., He, C., & Yang, L. (2020). Structural characteristics of industrial clusters and regional innovation. Economics Letters, 188, 109003. https://doi.org/https://doi.org/10.1016/j.econlet.2020.109003
- Molina-Morales, F. X., & Expósito-Langa, M. (2012). The impact of cluster connectedness on firm innovation: R&D effort and outcomes in the textile industry. Entrepreneurship & Regional Development, 24(7–8), 685–704. https://doi.org/https://doi.org/10.1080/08985626.2012.710260
- Morgulis-Yakushev, S., & Sölvell, Ö. (2017). Enhancing dynamism in clusters. Competitiveness Review: An International Business Journal, 27(2), 98–112. https://doi.org/https://doi.org/10.1108/CR-02-2016-0015
- Murray, M. N., & Dowell, P. (1999). Examining supply gaps and surpluses in the automotive cluster in Tennessee. University of Tennessee – Center for Business and Economic Research.
- Nie, P., & Sun, P. (2015). Search costs generating industrial clusters. Cities, 42, 268–273. https://doi.org/https://doi.org/10.1016/j.cities.2014.08.002
- Nie, P., Wang, C., & Lin, L. (2020). Cooperation of firms yielding industrial clusters. Area, 52(4), 731–740. https://doi.org/https://doi.org/10.1111/area.12632
- Nohria, N., & Eccles, R. G. (1992). Networks and organizations: Structure, form, and action. Harvard Business School Press.
- OECD. (1999). Boosting innovation. The cluster approach. OECD Publishing.
- Pavelková, D., Bendó, Z., Frankowska, M., Havierniková, K., Břusková, P., Bednář, P., Knápková, A., Danko, L., Sopoligová, M., Myszak, J. M., & Somkuti, M. (2019). Towards smart cluster policies in V4 countries. Tomas Bata University in Zlín.
- Porter, M. E. (1990). The competitive advantage of nations. Free Press.
- Porter, M. E. (1998). Clusters and the new economics of competition. Harvard Business Review, 76(6), 77–90.
- Porter, M. E. (2000). Location, competition, and economic development: Local clusters in a global economy. Economic Development Quarterly, 14(1), 15–34. https://doi.org/https://doi.org/10.1177/089124240001400105
- Pouder, R., & St. John, C. (1996). Hot spots and blind spots: Geographical clusters of firms and innovation. Academy of Management Review, 21(4), 1192–1225. https://doi.org/https://doi.org/10.5465/amr.1996.9704071867
- R Core Team. (2018). R: A language and environment for statistical computing. R Foundation for Statistical Computing. Retrieved January 9, 2018, from https://www.R-project.org
- Ridley, D. B. (2008). Herding versus hotelling: Market entry with costly information. Journal of Economics & Management Strategy, 17(3), 607–631. https://doi.org/https://doi.org/10.1111/j.1530-9134.2008.00188.x
- Rosenfeld, S. (2002). A governor’s guide to cluster-based economic development. National Governors Association.
- Ruland, W. (2013). Does cluster membership enhance financial performance? iBusiness, 05(01), 1–11. https://doi.org/https://doi.org/10.4236/ib.2013.51001
- Shaver, M. J., & Flyer, F. (2000). Agglomeration economies, firm heterogeneity, and foreign direct investment in the United States. Strategic Management Journal, 21(12), 1175–1193. https://doi.org/https://doi.org/10.1002/1097-0266(200012)21:12 < 1175::AID-SMJ139 > 3.0.CO;2-Q
- Sölvell, Ö., Lindqvist, G., & Ketels, C. (2003). The cluster initiative greenbook. VINNOVA.
- Spencer, G. M., Vinodrai, T., Gertler, M. S., & Wolfe, D. A. (2010). Do clusters make a difference? Defining and assessing their economic performance. Regional Studies, 44(6), 697–715. https://doi.org/https://doi.org/10.1080/00343400903107736
- Stavropoulos, S., & Skuras, D. (2016). Firm profitability and agglomeration economies: An elusive relationship. Tijdschrift Voor Economische en Sociale Geografie, 107(1), 66–80. https://doi.org/https://doi.org/10.1111/tesg.12125
- Sternberg, R., & Litzenberg, T. (2004). Regional clusters in Germany – Their geography and their relevance for entrepreneurial activities. European Planning Studies, 12(6), 767–791. https://doi.org/https://doi.org/10.1080/0965431042000251855
- Stojčić, N., Anić, I.-D., & Aralica, Z. (2019). Do firms in clusters perform better? Lessons from wood-processing industries in new EU member states. Forest Policy and Economics, 109, 102043. 10/ggrd9f https://doi.org/https://doi.org/10.1016/j.forpol.2019.102043
- Stuart, T., & Sorenson, O. (2003). The geography of opportunity: Spatial heterogeneity in founding rates and the performance of biotechnology firms. Research Policy, 32(2), 229–253. https://doi.org/https://doi.org/10.1016/S0048-7333(02)00098-7
- Uzzi, B. (1997). Social structure and competition in interfirm networks: The paradox of embeddedness. Administrative Science Quarterly, 42(1), 35–67. https://doi.org/https://doi.org/10.2307/2393808
- Vaan, M. D., Boschma, R., & Frenken, K. (2013). Clustering and firm performance in project-based industries: The case of the global video game industry, 1972–2007. Journal of Economic Geography, 13(6), 965–991. https://doi.org/https://doi.org/10.1093/jeg/lbs038
- Wickham, H., Averick, M., Bryan, J., Chang, W., McGowan, L., François, R., Grolemund, G., Hayes, A., Henry, L., Hester, J., Kuhn, M., Pedersen, T., Miller, E., Bache, S., Müller, K., Ooms, J., Robinson, D., Seidel, D., Spinu, V., … Yutani, H. (2019). Welcome to the tidyverse. Journal of Open Source Software, 4(43), 1686. https://doi.org/https://doi.org/10.21105/joss.01686
- Žižka, M. (2019). Inter-branch comparison of cluster company performance using Malmquist Index. In M. Houda & R. Remeš (Eds.), 37th International Conference on Mathematical Methods in Economics 2019. Conference Proceedings (pp. 37–42). University of South Bohemia in České Budějovice.