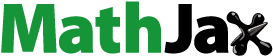
Abstract
Group emergency decision-making is an uncertain and dynamic process, in which the decision makers may be bounded rational and have a risk appetite. To depict the vague qualitative assessments, the probabilistic linguistic term sets are employed to express the perceptions of decision makers. First, considering the regret-aversion of the decision makers’ psychological characteristic, the value function and the regret-rejoice function in the regret theory are modified to adapt the probabilistic linguistic information. Second, the definition and aggregation operators of the probabilistic linguistic time variable are proposed to describe and aggregate the dynamic decision information. Third, the probabilistic linguistic models based on the dynamic reference point method and the regret theory are studied to maximise the expectation-levels of alternatives at the relative time point. The proposed method is applied to select the optimal response strategy for the outbreak of COVID-19 in China. Finally, the comparative analysis is designed to verify the applicability and reasonability of the proposed method.
JEL CLASSIFICATIONS:
1. Introduction
In recent years, various emergencies have caused huge economic losses and casualties to human society, such as September 11 terrorist attacks in 2001, severe acute respiratory syndrome (SARS) in 2003, Wenchuan earthquake in 2008, Japan's nuclear leakage event caused by earthquake in 2011. As of May 18, 2020, there have been more than 4.5 million confirmed cases of COVID-19 and 300,000 deaths worldwide (World Health Organization, Citation2020). When the emergency occurs, decision makers should make some effective emergency measures to reduce casualties and economic loss as much as possible. In the decision-making process, it is of great significance to adjust strategies as emergency situations evolve (Jiang et al., Citation2011). In the early stage of emergency, since the collected assessment information is usually incomplete and inaccurate, the decision makers need to estimate the seriousness of emergency by combining the objective information with their own subjective experience and implement response measures immediately (Ge et al., Citation2010; Mendonca et al., Citation2001). At the beginning of the emergency, it is essential to collect the relevant information and adopt response measures within a very short time to ensure the safety of life and minimise the economic loss. Due to the uncertainty in the development and evolution process, the emergency probably presents a variety of complex situations. Therefore, the strategy selection of uncertain emergency is a risky decision-making problem (Peng & Li, Citation2019). When the emergency plan is executed, the development trend of the emergency gradually becomes clear and the situation of emergency may change over time. The initial measures may be inappropriate to response the emergency of next stage and therefore policymakers need to constantly adjust previous strategy according to the updated decision information (Altay & Green, Citation2006).
In conclusion, in the emergency decision-making (EDM), the decision makers may encounter the following issues: (1) Express the uncertain and incomplete assessment information in a limited time; (2) Consider decision maker’s psychological behaviour characterised by bounded rationality; (3) Establish a dynamic decision-making model to respond to the changing circumstances of an emergency.
Considering that human thought and characteristic of evaluation index are fuzzy (Wang et al., Citation2018), the real numbers are difficult to describe the uncertain quantitative assessments. For example, the risk levels of COVID-19 for some provinces in China are usually expressed by ‘High-risk’, ‘General-risk’, ‘Low-risk’, etc. In some cases, since decision makers are more likely to evaluate some criteria by qualitative estimation rather than quantitative data, the linguistic term is in alignment with the human expressive habits (He et al., Citation2016; Wang et al., Citation2018; Zadeh, Citation1975). However, the single linguistic term has limitations in expressing the increasing uncertainties due to the complexity of uncontrolled factors, such as the limited time and knowledge in the professional field (He et al., Citation2019). To tackle these problems, the probabilistic linguistic term sets (PLTSs) (Pang et al., Citation2016) were introduced to depict the uncertain linguistic information. The PLTSs not only can express several linguistic terms, but also reflect the probability of each linguistic term, such as ‘30% sure very low-risk and 70% sure low-risk’.
Owning to the effectiveness and flexibility of describing uncertain linguistic information, the PLTSs have aroused increasing attention (Liao et al., Citation2020; Liu et al., Citation2019; Zhang et al., Citation2017) and many ranking methods based on the probabilistic linguistic information were proposed to solve the decision-making problems (Lei et al., Citation2020; Wei et al., Citation2019, Citation2020). In the process of the EDM, due to the complexity, strong destructiveness and uncertainty of the incident, the incomplete decision-making information increases the difficulty for decision makers to formulate strategy. It is impossible for all the decision makers to have comprehensive understanding of the changing emergency circumstances due to the limitations of personal cognition and the urgency of time. When decision makers are under enormous social stress, they are characterised by bounded rationality, loss sensitivity and susceptible to the emergency (Ding et al., Citation2019). Therefore, decision makers usually do not satisfy the conditions of totally rationality in the dynamic EDM.
Recently, regret theory (Bell, Citation1982; Loomes & Sugden, Citation1982) has attracted a lot attention of scholars and applied to many domains (Chorus, Citation2012; Gong et al., Citation2019; Wang et al., Citation2020). Regret theory considers the comparison between the selected strategy and other unselected strategies. If the decision makers find that the benefit of selected strategy is greater than others, they rejoice, otherwise they regret. The key to regret theory is to avoid selecting the strategy that makes the decision makers regret, that is, the decision makers are regret-aversion.
Given that the dynamic feature of emergency events, some dynamic reference point methods (Gao et al., Citation2017; Wang et al., Citation2015) are proposed to adjust the response measures of emergency. Based on the TOPSIS method and the proposed probability density function, a prospect theory-based dynamic reference point method is presented under the interval values circumstance (Wang et al., Citation2015). A dynamic decision-making method is developed to handle the hesitant probabilistic fuzzy information by the dynamic reference point method (Gao et al., Citation2017). Inspired by Gao et al. (Citation2017), we propose a dynamic reference point method with probabilistic linguistic information combining the modified regret theory.
There are three challenges when we extend the dynamic reference point method to the probabilistic linguistic environment considering the regret-aversion feature of decision makers. (1) The classical utility function of regret theory cannot be applied directly to the probabilistic linguistic environment, because the results may exceed the scale of linguistic term set and change the essential feature of regret theory. We propose the modified utility function and regret-rejoice function to bridge the gap combining with original characteristic of regret theory. (2) A concept should be proposed to express the decision matrix represented by PLTSs with time variable. In the paper, we define the probabilistic linguistic time variable (PLTV) to describe the dynamic probabilistic linguistic decision matrix. The operations of PLTVs with the weight vector of time variable are presented according to the weighted averaging and geometric operators. (3) The dynamic model should be established to maximise the expectation-levels of alternatives at the corresponding time point. First, we define the deviation degree of PLTSs and the probabilistic linguistic positive and negative ideal solutions. Then the expectation-levels of alternatives are proposed with unknown weight vector inspired by the TOPSIS method. Combining with the modified perceived utility function of regret theory, the dynamic nonlinear programming models at any time point are constructed. Finally, according to the calculated weights of decision makers by solving the models and the aggregating operator, the optimal alternatives of each stage can be obtained for the dynamic emergency. The main contributions of the paper are summarised as follows:
The paper proposes the modified perceived utility function of regret theory which includes the revised utility and regret-rejoice functions under the probabilistic linguistic circumstance. The modified regret theory inherits the essential characteristic of classic regret theory and reflects the decision make’s risk appetite effectively.
The definition and aggregation methods of PLTVs are developed to describe the dynamic probabilistic linguistic decision matrix with the time variable.
Combining with the modified regret theory, the probabilistic linguistic dynamic reference point (PLDRP) method is proposed to handle the optimal alternative selection of dynamic EDM problems at different time points. The nonlinear programming model whose objective function maximises the expectation-levels of alternatives is built to obtain decision makes’ unknown weight values.
The PLDRP method is applied to the dynamic optimal alternative selection of the outbreak of COVID-19 in China. The comparative analysis with other probabilistic linguistic decision-making methods illustrates the applicability and effectiveness of the proposed method.
The remainder of the paper is organised as follows: In Section 2, the literature review about the current EDM and probabilistic linguistic decision-making methods is presented. Section 3 introduces some related definitions about PLTSs and regret theory. In Section 4, based on the modified regret theory for PLTSs and the proposed PLTV, the PLDRP method is presented to find the optimal alternatives at different time points. In Section 5, the procedure of the PLDRP method is introduced. In Section 6, the PLDRP method is applied to a numerical example about the selection of dynamic optimal alternatives and its superiority is illustrated by the comparative analysis. Conclusions and future directions are drawn in Section 7.
2. Literature review
In the EDM, how to select the appropriate response strategy in the limited time is a crucial issue when the decision makers encounter uncertain decision-making information. The multiple criteria decision making (MCDM) methods have been applied widely to respond and resolve the emergency events (Ding & Liu, Citation2019a). A dynamic case-based reasoning group decision making approach is proposed for a gas explosion by choosing the most suitable case from a set of similar cases after case retrieval (Zheng et al., Citation2020). To describe decision makers’ psychological characteristics, a dynamic EDM method based on the prospect theory and interval-valued Pythagorean fuzzy linguistic variables is developed to determine the optimal solution dynamically under different emergency status (Ding et al., Citation2019). According to the constructed prospect decision matrix, an extended VIKOR approach is proposed to handle the EDM problems with 2-dimension uncertain linguistic information (Ding & Liu, Citation2019b). To avoid information loss and keep the form of PLTS, the probabilistic linguistic weighted averaging operator combining Dempster-Shafer evidence theory is applied to a serious mine accident (Li & Wei, Citation2019). An EDM model under the Z-uncertain probabilistic linguistic circumstance is developed using the information credibility and the maximising deviation method (Chai et al., Citation2021). To select the best alternative in the multi-stage group decision-making problems, type alpha and type gamma consensus are constructed combining the mining consensus sequences (Tang et al., Citation2020). Based on the decision makers' preference transfer and cumulative prospect theory, a large group risk dynamic EDM method is presented to select the optimal scheme in the emergency incident (Xu et al., Citation2018). Zhou et al. (Citation2018) provided an overview of the EDM for natural disasters and illustrated the construction of emergency decision support system in detail.
The aim of multiple criteria group decision making (MCGDM) is to support decision makers when they choose the best solution among alternatives considering multiple criteria (Tian et al., Citation2017, Citation2018), which can be applied to the strategy selection problems in the EDM. As for the decision matrix represented by the probabilistic linguistic information, many decision-making methods have been developed to copy with the MCDM problems (Wei et al., Citation2020). The aggregation operators (Liu & Teng, Citation2018; Pang et al., Citation2016) calculate each alternative’s overall utility value based on the specific operation laws of PLTSs, then rank the alternatives. The probabilistic linguistic MULTIMOORA method derives three subordinate utility values and adopts the improved Borda rule to obtain the ranking results (Wu et al., Citation2018). The main purpose of the reference point-based methods is to select a reference point which is closest to the positive ideal solution. The TOPSIS method (Pang et al., Citation2016) and the VIKOR method (Zhang & Xing, Citation2017) are two classical reference point-based methods which are widely applied to solve the decision-making problems. Pan et al. (Citation2018) extended the ELECTRE II method to probabilistic linguistic environment to deal with the therapeutic schedule evaluation problem. Based on the proposed differential evolution algorithm, the probabilistic linguistic PROMETHEE method is developed to evaluate the meteorological disaster risk (Yu et al., Citation2019). Feng et al. (Citation2019) defined some concordance and discordance indexes according to the proposed possibility degrees of PLTSs and then presented a probabilistic linguistic QUALIFLEX method. Most MCGDM methods are based on the expected utility theory which assumes that decision makers are totally rational. Considering the psychological factors of decision makers, the probabilistic linguistic TODIM method is proposed and applied to the evaluation of water security (Zhang et al., Citation2019).
Although various of MCGDM theories and methods have been introduced to handle the strategy selection in the uncertain EDM. However, the existing literature rarely considers the regret theory which combines decision makers’ regret-aversion characteristic under the probabilistic linguistic circumstance. Besides, the research of dynamic reference point method with probabilistic linguistic information to deal with the emergencies has not found from the existing literature. To bridge these research gaps, we propose a PLDRP method considering the regret theory and the dynamic evolutionary process of public health EDM. With the proposed dynamic EDM method, the local government can accurately and quickly select the optimal response strategy for the outbreak of COVID-19.
3. Preliminaries
In this section, we recall some basic concepts and the comparison rules about PLTSs and regret theory.
3.1. PLTSs
To express the cognitive uncertain information, Pang et al. (Citation2016) proposed the PLTSs by assigning corresponding probability to each linguistic term.
Definition 1
(Pang et al., Citation2016). Let be fixed and
be a linguistic term set, a PLTS on
is
with
(1)
(1)
where
is the
th linguistic term
with the probability
The linguistic terms
are arranged in ascending order.
is the probabilistic linguistic element and
is the number of different
in
When the judgement information is missing or incomplete, that is some methods (Pang et al., Citation2016; Wu et al., Citation2018) are proposed to distribute the missing probability. The missing probability could be distributed to the linguistic terms which appear in
Let
then
(Pang et al., Citation2016). The distribution proportion of unknown probability to each linguistic term in
is equal in this paper.
Furthermore, since the number of PLTSs may be different, it is difficult to directly operate the PLTSs. To make the PLTSs with different numbers of linguistic terms computable and comparable, an extension method (Pang et al., Citation2016) is developed as follows: for any two PLTSs and
if
then add
linguistic terms to
where the corresponding probabilities of added linguistic terms are equal to zero. The extension method is proposed without changing any previous information of the PLTSs. Then we get the normalised PLTS (NPLTS):
The comparison rules and the deviation degree of PLTSs are introduced as follows:
Definition 2
(Pang et al., Citation2016). Let be a PLTS, and
be the subscript of linguistic
The score function of
is:
(2)
(2)
where
The deviation function of is defined as:
(3)
(3)
The comparison method of any two PLTSs and
is given as follows:
if
then
if
then
if
then we need to make further comparison:
if
then
if
then
if
then
Definition 3
(Pang et al., Citation2016). Let be two PLTSs with
Then the deviation degree between
and
can be defined as:
(4)
(4)
where
and
are the subscripts of linguistic term
and
respectively.
3.2. Regret theory
Regret theory is an important behavioural decision theory proposed by Bell (Citation1982) and Loomes and Sugden (Citation1982) which depicts the regret-aversion characteristic of decision makers. In the regret theory, the policymakers not only concern the obtained results, but also focus on the results if they select other solutions in the decision-making process. The perceived utility of the regret theory consists of two parts: the current results of the utility function and regret-rejoice function compared with other results.
Definition 4
(Zhang et al., Citation2016). Let be the attribute value, then the utility function
can be defined as follows:
(5)
(5)
where the first derivative satisfies
and the second derivative satisfies
and
is the risk aversion coefficient of the decision maker.
Definition 5
(Zhang et al., Citation2016). The regret-rejoice function can be defined as follows:
(6)
(6)
where
denotes the utility difference of two alternatives, and
represents the regret-rejoice function of
Similar to
the first and second derivatives of
satisfy
and
and
is the regret aversion coefficient of the decision maker. If
is the rejoice value; otherwise,
is the regret value.
Definition 6 (Zhang et al., Citation2016).
Let and
denote the evaluation values of the alternatives
and
respectively. The perceived utility value of
is obtained by the utility function and regret-rejoice function as follows:
(7)
(7)
4. The PLDRP method
In this section, the modified regret theory under the probabilistic linguistic circumstance and the PLTV are proposed, which build a basic framework for the PLDRP method.
4.1. The modified regret theory for PLTSs
The classical utility function of regret theory cannot be applied directly to the EDM with probabilistic linguistic information, because the results may exceed the scale of linguistic term set and change the essential feature of the utility function and regret-rejoice function.
The NPLTSs are composed of two parts: the linguistic term
and its probability
In the modified regret theory for PLTSs, we only revise the value and regret-rejoice functions for the linguistic term
The probability
associated with
remains unchanged.
Definition 7.
Let denote the evaluation value of the alternative
expressed by the NPLTS. The modified value function of
under the probabilistic linguistic circumstance is as follows:
(8)
(8)
(9)
(9)
where
is the subscript of the linguistic term
The modified value function
with respect to different
is shown in .
Since the range of is
the domain of utility function
for PLTSs should be
which satisfies the range of
within the virtual linguistic term sets. First, we translate
in EquationEquation (5)
(5)
(5) into
to hold
Then, we zoom
to
Considering that individuals are much more sensitive to loss than gain, we multiply
by
to hold
then obtain
Hence, the value function of regret theory is transferred to EquationEquation (8)
(8)
(8) . When
the value function is
When
the value function is
When
the value function is
The modified
is a function obtained by translating and zooming in and out the classical value function of regret theory. When
the outcomes obtained by experiments are in accordance with the original data (Tversky & Kahneman, Citation1992).
Definition 8.
Let and
denote the evaluation value of the alternatives
and
expressed by NPLTSs, respectively. The modified regret-rejoice function of
compared with
under the probabilistic linguistic circumstance is as follows:
(10)
(10)
(11)
(11)
where
and
are the subscripts of the linguistic terms
and
respectively.
Since the range of is
the domain of regret-rejoice function
for PLTSs should be
to satisfy that the range of
within the virtual linguistic term sets. Considering that individuals are much more sensitive to loss than gain, we multiply
by
to hold
then we obtain
Hence, the regret-rejoice value of regret theory is converted to EquationEquation (10)
(10)
(10) . When
the regret-rejoice value is
When
the regret-rejoice value is
When
the regret-rejoice value is
The modified regret-rejoice function with respect to different values of
is shown in . It is a function that zooms in and out the classical regret-rejoice function of regret theory. shows that the decision maker is more sensitive to
than
which indicates that the decision maker is more regret aversive. We adopt
in this paper which is recommended in Wang et al. (Citation2020).
Definition 9.
Let and
denote the evaluation value of the alternatives
and
expressed by NPLTSs, respectively. The perceived utility value of
compared with
can be obtained by the modified utility function and regret-rejoice function as follows:
(12)
(12)
(13)
(13)
where
and
are the subscript of the linguistic term
and
and
is a parameter to adjust the importance degree of the utility function and regret-rejoice function. Referring to the classical regret theory, we assume
in the following part of the paper.
4.2. The PLTV
When the emergency occurs, decision-making environment will change over time due to human intervention and the evolution of emergency. The decision matrix should be updated to response the changing emergency in the multi-stage process. In order to describe the multi-stage probabilistic linguistic information, we propose the definition of PLTV.
Definition 10.
Let be fixed and
be a time variable, then the PLTV at time
on a linguistic term set
is
with
(14)
(14)
where
is the
th linguistic term
with the probability
at time
in
The linguistic terms
are arranged in ascending order.
is called the probabilistic linguistic time element (PLTE).
If then
indicate
PLTEs of the element
collected at
different stages. In other words, the values of a PLTV are PLTEs. The PLTV can record the changes of decision makers’ evaluation information over time. The operations of PLTEs with the time weight vector
are as follows:
Definition 11.
Let
be
PLTEs, where
and
are the
th linguistic term and corresponding probability in
The probabilistic linguistic time weighted averaging (PLTWA) operator can be defined as:
where
represents the sum symbol of PLTEs,
is the time weight vector of
and
If then the PLTWA operator is reduced to the probabilistic linguistic time averaging operator.
Definition 12.
Let
be
PLTEs, where
and
are the
th linguistic term and corresponding probability in
The probabilistic linguistic time weighted geometric (PLTWG) operator can be defined as:
where
represents the sum symbol of PLTEs,
is the time weight vector of
and
If then the PLTWG operator is reduced to the probabilistic linguistic time geometric operator.
4.3. The PLDRP model
The emergency event is a dynamic development and evolution process considering that most decision information is uncertain and incomplete in the initial period. With more decision information being collected, the emergency response should be adjusted timely as the reference point changes. In this part, based on the proposed positive ideal solution and negative ideal solution for PLTSs, the expectation-levels of alternatives are proposed. Then the nonlinear programming models are established to maximise the reference point of emergency response. Combining with the modified regret theory with the probabilistic linguistic information, the PLDRP model is presented to obtain optimal alternative for the EDM.
Definition 13.
Let be a probabilistic linguistic decision matrix with
Then
is called the probabilistic linguistic positive ideal solution (PLPIS), where
is the subscript of the linguistic term
Definition 14.
Let be a probabilistic linguistic decision matrix with
Then
is called the probabilistic linguistic negative ideal solution (PLNIS), where
be the subscript of the linguistic term
We first calculate the deviation degree between for the alternative
of the decision maker
and the PLPIS.
Then we compute the deviation degree between for the alternative
of the decision maker
and the PLNIS.
Motivated by the TOPSIS method, the expectation-levels of alternatives are as follows:
Definition 15.
The expectation-levels of the alternatives
with the weight vector
where
and
at the time point
are defined as:
(15)
(15)
where the parameter
denotes the risk appetite of the decision maker:
means the decision maker is a general risk seeker;
means the opposite.
The aim of the PLDRP model is to maximise the reference point of emergency response at the time point
based on the proposed expectation-levels of alternatives. To obtain the reference point and form the weight vector
at the time point
the nonlinear model is built as follows:
Model 1
Combining EquationEquation (15)(15)
(15) , Model 1 is translated to Model 2 as follows:
Model 2
Solving Model 2, the optimal weight vector and the optimal reference point
of the alternatives
can be calculated at the time point
We obtain the initial reference point
by solving Model 2, then the optimal alternative can be selected by the probabilistic linguistic weighted averaging (PLWA) operator or the probabilistic linguistic weighted geometric (PLWG) operator (Zhang et al., Citation2017). In the next stage, that is, at the time point
the programming model is developed as follows:
Model 3
Combining EquationEquation (15)(15)
(15) , Model 3 can be converted to Model 4:
Model 4
Solving Model 4, if there is no optimal solution, then the reference point isn’t consistent with the time point
Hence, we need to reset the optimal reference point by Model 2 and rank the alternatives by the recomputed
and
In the process of the EDM, due to the urgency and complexity of the incident, it is difficult for decision makers to collect the uncertain and incomplete decision-making information by crisp values. When the decision makers are under enormous social stress, they are characterised by bounded rationality and regret-aversion in the process of risk decision. Therefore, in order to meet the actual requirement of the EDM, regret theory is introduced to the dynamic reference point method under the probabilistic linguistic circumstance. The models of the PLDRP method with regret theory are as follows:
Model 5
where
and
are the perceived utility value of
and
respectively.
where
By solving Model 5 we obtain the optimal weight vector and the optimal reference point
of the alternatives
The PLWA or PLWG operator (Zhang et al., Citation2017) is utilised to aggregate the decision information of the alternatives
according to
and
then choose the best alternative.
For we solve the optimal programming of Model 6 based on the reference point
and
If Model 6 does not exist an optimal solution, then return to solve Model 5 to obtain a new optimal reference point and adjust the emergency response strategy according to
and
When
we select the optimal alternatives of each stage
that form the optimal alternative chain of the EDM.
Model 6
where
and
are the perceived utility value of
and
respectively.
where
5. The procedure of the PLDRP method
The following steps can be used to build the PLDRP method for emergency response under the probabilistic linguistic environment:
Step 1. Identify the alternatives
and the decision makers
and then construct the PLTV decision matrices
about the emergency at different time points
Step 2. Calculate the modified perceived utility values for all probabilistic linguistic elements in
according to Equation (12), denoted by
Step 3. Compute the deviation degrees
and
and then obtain the expectation-levels of the alternative
with the weight vector
at different time points
Step 4. Solve Model 5 to obtain the optimal weight vector
and the optimal reference point
of the alternatives
Step 5. Utilise the PLWA or PLWG operator (Zhang et al., Citation2017) to aggregate the decision information of the alternatives
according to
and
and then choose the best alternative.
Step 6. For
solve the optimal programming of Model 6 based on the reference point
and
If Model 6 does not exist an optimal solution, then return to Step 4 to obtain a new optimal reference point and adjust the emergency response strategy according to
and
Step 7. When
end the algorithm.
Step 8. Select the optimal alternatives of each stage
that form the optimal alternative chain of the EDM.
The procedure of the PLDPR method is manifested by .
6. A numerical example: strategy selection in the EDM
6.1. Case description: the application in the public health EDM
In December 2019, some cases of pneumonia with unknown cause suffered from the symptoms, such as fever, cough and fatigue were reported in Wuhan, China. The epidemic disease is proven to be COVID-19, which have not been found before (The 2019-nCoV Outbreak Joint Field Epidemiology Investigation Team & Li, Citation2020). The current research indicates that the novel coronavirus has strong affinity to a human respiratory receptor and can be spread by droplets and close contact (Li et al., Citation2020). Afterwards, the existence of human-to-human transmission is confirmed, then the lockdown of 11 million people in Wuhan from January 23, 2020 is implemented, which is rare in human public health history. The highway traffic is strictly controlled and the public-transport system is temporarily suspended over the whole country. The holidays of Chinese New Year are extended and people are forbidden to go out except some special situations. The cross-provincial traffic is limited and some retail stores and restaurants are closed to reduce gathering and cross-infection as low as possible. A total of 74,185 confirmed cases and 2004 cases of death have been reported on February 18, 2020 (Health.people.com.cn, Citation2020). As the number of confirmed cases continues to increase in China, on January 30, 2020 World Health Organization (WHO) declared the outbreak of COVID-19 as a Public Health Emergency of International Concern (World Health Organization, Citation2020).
Since the outbreak of COVID-19, China has been recognised and praised by WHO for its rapid and efficient prevention and control measures. On March 13, 2020, the number of new confirmed cases dropped to 11 cases in China mainland and the number of new confirmed cases dropped for 15 consecutive days outside Hubei province. The strict control measures have proven to be effective in the early days of outbreak, such as banning all public gatherings, closing entertainment venues and suspending public transport. More than 38,000 medical staffs, among which the intensive care medical professionals have nearly 10% of the nation, were sent to Hubei province. Adequate medical treatment and epidemic prevention materials have made a significant contribution to reducing mortality of COVID-19 and containing outbreaks.
The sudden outbreak of COVID-19 has unprecedented negative influences on China's economic and social development. According to the report of National Bureau of Statistics of China, the national economy shrinks 6.8% in the first quarter of 2020 (National Bureau of Statistics of China, 2020). The growth rate over the same period last year of Wholesale and Retail Trades, Accommodation and Restaurants, Manufacturing and Construction are −17.8%, −35.3%, −10.2% and −17.5%, respectively. Chinese people have made great sacrifices to contain the outbreak of novel coronavirus. Along with the normalisation of COVID-19, it is of great significance to arouse public consumption in combination with efforts to improve the public service system. At present, China's epidemic prevention and control situation is further consolidated, but the international epidemic continues to spread. When and how to resume work and reboot economy in an orderly manner are severe questions and challenges for the government. The measures of epidemic response should keep up with the changing epidemic situation and flexibly adjust.
The PLDRP method can be applied to deal with the optimal alternative selection for the response of COVID-19. Through interviews and survey, four alternatives are selected as follows: Adopt public epidemic prevention measures,
Reopen school,
Lockdown and
Resumption of work. The time points are determined as:
January 23, 2020,
February 18, 2020 and
March 13, 2020. Five experts
are invited to make evaluation towards the alternative
at different time points
is a member of National Health Commission who organises the specific work of emergency response to COVID-19 and medical relief.
is member of National Health Commission who is responsible for guiding and supervising the implementation of epidemic prevention.
and
are members of the government's leadership group on the epidemic prevention and control.
is an expert of clinical medical research on the respiratory diseases. Suppose that
is a linguistic term set, whose linguistic terms are
extremely poor,
poor,
slightly poor,
fair,
slightly good,
good and
extremely good.
6.2. Case solution
The specific steps of selecting the optimal alternatives of each stage
are as follows:
Step 1. The PLTV decision matrices about the emergency at different time points
are collected as .
Table 1. Evaluation information of
Table 2. Evaluation information of
Table 3. Evaluation information of
Step 2. Calculate the modified perceived utility values for all probabilistic linguistic elements in
according to EquationEquation (12)
(12)
(12) and then compute the deviation degrees
and
as .
Table 4. The deviation degrees
Table 5. The deviation degrees
Table 6. The deviation degrees
Table 7. The deviation degrees
Table 8. The deviation degrees
Table 9. The deviation degrees
Step 3. Set and solve Model 5 based on the deviation degrees in and as follows:
We obtain the initial weight vector and the initial optimal reference point
Step 4. According to and
we utilise the PLWA operator (Zhang et al., Citation2017) to aggregate the decision information of the alternatives and obtain corresponding score functions:
Then we choose the best alternative and the ranking order of alternatives is
Step 5. For we solve the nonlinear programming of Model 6 based on the reference point
obtained in Model 5. Model 6 converts to the following nonlinear programming problem:
By solving the model, we obtain the weight vector and the optimal reference point
According to and
we aggregate the decision information of the alternatives and obtain corresponding score functions:
Then we choose the best alternative and the ranking of alternatives is
Step 6. For we solve nonlinear programming of Model 6 based on the obtained reference point
Model 6 is converted to the nonlinear programming problem as follows:
By solving the model, we obtain the weight vector and the optimal reference point
According to and
we aggregate the decision information of the alternatives and obtain corresponding score functions:
Then we choose the best alternative and the ranking of alternatives is
Based on the above results obtained by the proposed PLDRP method at the time points we get the optimal decision chain:
The results indicate that: at the time point
the government should enforce a strict lockdown policy, that is the alternative
since
is the early days of the outbreak. Besides, it is quite necessary to implement some public epidemic prevention measures to curb the spread of the epidemic, that is the alternative
At the time point
the optimal alternative is still
Although the spread of COVID-19 is still serious, the production of society can be properly resumed, that is the alternative
which is followed the optimal alternative
At the time point
the optimal alternative is
Resumption of work and economic development are urgent, while the government should adopt some public epidemic prevention measures to contain the spread of COVID-19.
6.3. Comparative analysis
In this part, first, we compare the PLDRP method with the PLDRP method without regret theory to discuss the influence of regret theory on the PLDRP method. Next, the PLWA operator (Zhang et al., Citation2017) is employed to compare the decision-making results by the example in Section 6.2 with the PLDRP method. Note that the decision makers are endowed with same weight in the aggregating process of the PLWA operator. The concrete results of comparison are shown in .
Table 10. The results of comparison.
Although the ranking results of the PLDRP method and the PLDRP method without regret theory are roughly consistent, there are still some small difference between them. For example, at the time point
the result of PLDRP method indicates that the alternative
works better than the alternative
but the result of the PLDRP method without regret theory is the opposite. The reason for this is because the participation of regret theory considers the bounded rationality and regret-aversion feature of decision makers. The comparison results of the two methods show the importance of regret theory which can directly affect the ranking of alternatives, especially in the decision-making problems which exist little difference among various alternatives.
The optimal alternatives at the time points
are the same as those obtained by the PLDRP method and the PLWA operator, but the concrete ranking orders vary. For example, at the time point
the ranking of alternatives is
by the PLDRP method and the ranking is
by the PLWA operator. The reason lies in that the PLDRP method is dynamic process that the last stage will affect the next stage. In other words, the result of the time point
will influence the decision-making process of the time point
in the PLDRP method.
The rankings of the PLDRP method, which consider both the vague decision information and the decision maker’s bounded rationality, are different from the rankings obtained by the PLDRP method without regret theory and the PLWA operator. In most EDM situations, the decision-making problems are uncertain, risk and dynamic process. It is unreasonable to assume that the decision makers are perfectly rational and different decision stages are unconnected. The proposed PLDRP method which considers the decision maker’s characteristic of bounded rationality and the interconnected relationship of different stages in the EDM process is much more effective and reliable to resolve the EDM problems.
7. Conclusions
In the paper, we propose a PLDRP method to select the optimal alternative considering the decision maker’s psychological behaviour. The proposed method not only fills the theoretical gap of the reference point method with the probabilistic linguistic information, but also has significant practical value from the prospective of the dynamic evolutionary process of the EDM. The utility and regret-rejoice functions of regret theory are modified to adapt the probabilistic linguistic information. Then the concept and related operations of PLTV are presented to lay a foundation for the PLDRP model. Based on the expectation-levels of alternatives and dynamic reference points, the PLDRP model is established to maximise the reference point of emergency response. We apply the proposed method to select the optimal response strategy for the outbreak of COVID-19 in China. Furthermore, the comparative analysis is conducted to compare the ranking results obtained by the PLDRP method and other two probabilistic linguistic decision-making methods, which indicates the effectiveness of the proposed method.
The limitations of the proposed method are list as follows: (1) The PLDRP method should be generalised to the large-scale group decision-making combining different decision makers’ characteristics; (2) The heterogeneous information need to be considered in the decision matrix, since different decision makers may differ in their preferences and habits when they express their assessments; (3) The time variable and PLTE are discrete in the proposed method, the continuous PLDRP method should be extended in the further research. In other words, it is necessary to extend the multistage decision-making process into continuous process. In the future, the time series analysis with probabilistic linguistic information can be further explored in the EDM process. The PLDRP model can be applied into the prevention phase of diseases.
Disclosure statement
No potential conflict of interest was reported by the authors.
Additional information
Funding
References
- Altay, N., & Green, W. G. (2006). OR/MS research in disaster operations management. European Journal of Operational Research, 175(1), 475–493. https://doi.org/https://doi.org/10.1016/j.ejor.2005.05.016
- Bell, D. E. (1982). Regret in decision-making under uncertainty. Operations Research, 30(5), 961–981. https://doi.org/https://doi.org/10.1287/opre.30.5.961
- Chai, J. H., Xian, S. D., & Lu, S. C. (2021). Z-uncertain probabilistic linguistic variables and its application in emergency decision making for treatment of COVID-19 patients. International Journal of Intelligent Systems, 36(1), 362–402. https://doi.org/https://doi.org/10.1002/int.22303
- Chorus, C. G. (2012). Regret theory-based route choices and traffic equilibria. Transportmetrica, 8(4), 291–305. https://doi.org/https://doi.org/10.1080/18128602.2010.498391
- Ding, X. F., & Liu, H. C. (2019a). A new approach for emergency decision‐making based on zero‐sum game with Pythagorean fuzzy uncertain linguistic variables. International Journal of Intelligent Systems, 34(7), 1667–1684. https://doi.org/https://doi.org/10.1002/int.22113
- Ding, X. F., & Liu, H. C. (2019b). An extended prospect theory-VIKOR approach for emergency decision making with 2-dimension uncertain linguistic information. Soft Computing, 23(22), 12139–12150. https://doi.org/https://doi.org/10.1007/s00500-019-04092-2
- Ding, X. F., Liu, H. C., & Shi, H. (2019). A dynamic approach for emergency decision making based on prospect theory with interval-valued Pythagorean fuzzy linguistic variables. Computers & Industrial Engineering, 131, 57–65. https://doi.org/https://doi.org/10.1016/j.cie.2019.03.037
- Feng, X. Q., Liu, Q., & Wei, C. P. (2019). Probabilistic linguistic QUALIFLEX approach with possibility degree comparison. Journal of Intelligent & Fuzzy Systems, 36(1), 719–730. https://doi.org/https://doi.org/10.3233/JIFS-172112
- Gao, J., Xu, Z. S., & Liao, H. C. (2017). A dynamic reference point method for emergency response under hesitant probabilistic fuzzy environment. International Journal of Fuzzy Systems, 19(5), 1261–1278. https://doi.org/https://doi.org/10.1007/s40815-017-0311-4
- Ge, L., Mourits, M., Kristensen, A. R., & Huirne, R. (2010). A modelling approach to support dynamic decision-making in the control of FMD epidemics. Preventive Veterinary Medicine, 95(3–4), 167–174. https://doi.org/https://doi.org/10.1016/j.prevetmed.2010.04.003
- Gong, X. M., Yu, C. R., & Wu, Z. H. (2019). An extension of regret theory based on probabilistic linguistic cloud sets considering dual expectations: An application for the stock market. IEEE Access, 7, 171046–171060. https://doi.org/https://doi.org/10.1109/ACCESS.2019.2956065
- He, Y., Lei, F., Wei, G. W., Wang, R., Wu, J., & Wei, C. (2019). EDAS method for multiple attribute group decision making with probabilistic uncertain linguistic information and its application to green supplier selection. International Journal of Computational Intelligence Systems, 12(2), 1361–1370.
- He, Y. H., Guo, H. W., Jin, M. Z., & Ren, P. Y. (2016). A linguistic entropy weight method and its application in linguistic multi-attribute group decision making. Nonlinear Dynamics, 84(1), 399–404. https://doi.org/https://doi.org/10.1007/s11071-015-2595-y
- Health.people.com.cn. (2020). National health commission (NHC): 1749 newly confirmed cases from 0:00 to 24:00 on February 18. http://health.people.com.cn/n1/2020/0219/c14739-31593756.html
- Jiang, Y. P., Fan, Z. P., & Su, M. M. (2011). Study on the dynamic adjusting method for emergency decision. Chinese Journal of Management Science, 19(5), 104–108.
- Lei, F., Wei, G. W., Gao, H., Wu, J., & Wei, C. (2020). TOPSIS method for developing supplier selection with probabilistic linguistic information. International Journal of Fuzzy Systems, 22(3), 749–759. https://doi.org/https://doi.org/10.1007/s40815-019-00797-6
- Li, P., & Wei, C. P. (2019). An emergency decision‐making method based on D‐S evidence theory for probabilistic linguistic term sets. International Journal of Disaster Risk Reduction, 37, 101178. https://doi.org/https://doi.org/10.1016/j.ijdrr.2019
- Li, Q., Guan, X., Wu, P., Wang, X., Zhou, L., Tong, Y., Ren, R., Leung, K. S. M., Lau, E. H. Y., Wong, J. Y., Xing, X., Xiang, N., Wu, Y., Li, C., Chen, Q., Li, D., Liu, T., Zhao, J., Liu, M., … Feng, Z. (2020). Early transmission dynamics in Wuhan, China, of novel coronavirus-infected pneumonia. New England Journal of Medicine, 382(13), 1199–1207. https://doi.org/https://doi.org/10.1056/NEJMoa2001316
- Liao, H. C., Mi, X. M., & Xu, Z. S. (2020). A survey of decision-making methods with probabilistic linguistic information: bibliometrics, preliminaries, methodologies, applications and future directions. Fuzzy Optimization and Decision Making, 19(1), 81–134. https://doi.org/https://doi.org/10.1007/s10700-019-09309-5
- Liu, A. J., Qiu, H. W., Lu, H., & Guo, X. R. (2019). A consensus model of probabilistic linguistic preference relations in group decision making based on feedback mechanism. IEEE Access, 7, 148231–148244. https://doi.org/https://doi.org/10.1109/ACCESS.2019.2944333
- Liu, P. D., & Teng, F. (2018). Some Muirhead mean operators for probabilistic linguistic term sets and their applications to multiple attribute decision-making. Applied Soft Computing, 68, 396–431. https://doi.org/https://doi.org/10.1016/j.asoc.2018.03.027
- Loomes, G., & Sugden, R. (1982). Regret theory: An alternative theory of rational choice under uncertainty. The Economic Journal, 92(368), 805–824. https://doi.org/https://doi.org/10.2307/2232669
- Mendonca, D., Beroggi, G., & Wallace, W. A. (2001). Decision support for improvisation during emergency response operations. International Journal of Emergency Management, 1(1), 30–38. https://doi.org/https://doi.org/10.1504/IJEM.2001.000507
- National Bureau of Statistics of China. (2020). Preliminary accounting results of GDP for the first quarter of 2020. http://www.stats.gov.cn/english/PressRelease/202004/t20200420_1739811.html
- Pan, L., Ren, P. J., & Xu, Z. S. (2018). Therapeutic schedule evaluation for brain-metastasized non-small cell lung cancer with a probabilistic linguistic ELECTRE II method. International Journal of Environmental Research and Public Health, 15(9), 1799. https://doi.org/https://doi.org/10.3390/ijerph15091799
- Pang, Q., Wang, H., & Xu, Z. S. (2016). Probabilistic linguistic term sets in multi-attribute group decision making. Information Sciences, 369, 128–143. https://doi.org/https://doi.org/10.1016/j.ins.2016.06.021
- Peng, X. D., & Li, W. Q. (2019). Algorithms for interval-valued Pythagorean fuzzy sets in emergency decision making based on multiparametric similarity measures and WDBA. IEEE Access, 7, 7419–7441. https://doi.org/https://doi.org/10.1109/ACCESS.2018.2890097
- Tang, M., Liao, H. C., & Kou, G. (2020). Type alpha and type gamma consensus for multi-stage emergency group decision making based on mining consensus sequences. Journal of the Operational Research Society. https://doi.org/https://doi.org/10.1080/01605682.2020.1830724
- The 2019-nCoV Outbreak Joint Field Epidemiology Investigation Team & Li, Q. (2020). Notes from the field: An outbreak of NCIP (2019-nCoV) infection in China – Wuhan, Hubei Province, 2019-2020. China CDC Weekly, 2(5), 79–80. https://doi.org/https://doi.org/10.46234/ccdcw2020.022
- Tian, G., Zhang, H., Feng, Y., Jia, H., Zhang, C., Jiang, Z., Li, Z., & Li, P. (2017). Operation patterns analysis of automotive components remanufacturing industry development in China. Journal of Cleaner Production, 164, 1363–1375. https://doi.org/https://doi.org/10.1016/j.jclepro.2017.07.028
- Tian, G., Zhang, H., Feng, Y., Wang, D., Peng, Y., & Jia, H. (2018). Green decoration materials selection under interior environment characteristics: A grey-correlation based hybrid MCDM method. Renewable and Sustainable Energy Reviews, 81(1), 682–692. https://doi.org/https://doi.org/10.1016/j.rser.2017.08.050
- Tversky, A., & Kahneman, D. (1992). Advances in prospect theory: Cumulative representation of uncertainty. Journal of Risk and Uncertainty, 5(4), 297–323. https://doi.org/https://doi.org/10.1007/BF00122574
- Wang, H., Xu, Z. S., & Zeng, X. J. (2018). Modeling complex linguistic expressions in qualitative decision making: An overview. Knowledge-Based Systems, 144, 174–187. https://doi.org/https://doi.org/10.1016/j.knosys.2017.12.030
- Wang, H. D., Pan, X. H., Yan, J., Yao, J. L., & He, S. F. (2020). A projection-based regret theory method for multi-attribute decision making under interval type-2 fuzzy sets environment. Information Sciences, 512, 108–122. https://doi.org/https://doi.org/10.1016/j.ins.2019.09.041
- Wang, L., Zhang, Z. X., & Wang, Y. M. (2015). A prospect theory-based interval dynamic reference point method for emergency decision making. Expert Systems with Applications, 42(23), 9379–9388. https://doi.org/https://doi.org/10.1016/j.eswa.2015.07.056
- Wei, G. W., Lei, F., Lin, R., Wang, R., Wei, Y., Wu, J., & Wei, C. (2020). Algorithms for probabilistic uncertain linguistic multiple attribute group decision making based on the GRA and CRITIC method: Application to location planning of electric vehicle charging stations. Economic Research-Ekonomska Istraživanja, 33(1), 828–846. https://doi.org/https://doi.org/10.1080/1331677X.2020.1734851
- Wei, G. W., Lu, J. P., Wei, C., & Wu, J. (2020). Probabilistic linguistic GRA method for multiple attribute group decision making. Journal of Intelligent & Fuzzy Systems, 38(4), 4721–4732. https://doi.org/https://doi.org/10.3233/JIFS-191416
- Wei, G. W., Wei, C., Wu, J., & Wang, H. J. (2019). Supplier selection of medical consumption products with a probabilistic linguistic MABAC method. International Journal of Environmental Research and Public Health, 16(24), 5082. https://doi.org/https://doi.org/10.3390/ijerph1624
- World Health Organization. (2020). WHO coronavirus disease (COVID-19) dashboard. https://covid19.who.int/
- World Health Organization. (2020). WHO timeline - COVID-19. https://www.who.int/news-room/detail/27-04-2020-who-timeline–-covid-19
- Wu, X. L., Liao, H. C., Xu, Z. S., Hafezalkotob, A., & Herrera, F. (2018). Probabilistic linguistic MULTIMOORA: A multicriteria decision making method based on the probabilistic linguistic expectation function and the improved Borda rule. IEEE Transactions on Fuzzy Systems, 26(6), 3688–3702. https://doi.org/https://doi.org/10.1109/TFUZZ.2018.2843330
- Xu, X. H., Pan, B., & Yang, Y. S. (2018). Large-group risk dynamic emergency decision method based on the dual influence of preference transfer and risk preference. Soft Computing, 22(22), 7479–7490. https://doi.org/https://doi.org/10.1007/s00500-018-3387-3
- Yu, X. B., Chen, H., & Ji, Z. H. (2019). Combination of probabilistic linguistic term sets and PROMETHEE to evaluate meteorological disaster risk: Case study of southeastern China. Sustainability, 11(5), 1405. https://doi.org/https://doi.org/10.3390/su11051405
- Zadeh, L. A. (1975). The concept of a linguistic variable and its application to approximate reasoning-I. Information Sciences, 8(3), 199–249. https://doi.org/https://doi.org/10.1016/0020-0255(75)90036-5
- Zhang, S. T., Zhu, J. J., Liu, X. D., & Chen, Y. (2016). Regret theory method-based group decision-making with multidimensional preference and incomplete weight information. Information Fusion, 31, 1–13. https://doi.org/https://doi.org/10.1016/j.inffus.2015.12.001
- Zhang, X. L., & Xing, X. M. (2017). Probabilistic linguistic VIKOR method to evaluate green supply chain initiatives. Sustainability, 9(7), 1231. https://doi.org/https://doi.org/10.3390/su9071231
- Zhang, Y. X., Xu, Z. S., & Liao, H. C. (2017). A consensus process for group decision making with probabilistic linguistic preference relations. Information Sciences, 414, 260–275. https://doi.org/https://doi.org/10.1016/j.ins.2017.06.006
- Zhang, Y. X., Xu, Z. S., & Liao, H. C. (2019). Water security evaluation based on the TODIM method with probabilistic linguistic term sets. Soft Computing, 23(15), 6215–6230. https://doi.org/https://doi.org/10.1007/s00500-018-3276-9
- Zheng, J., Wang, Y. M., Zhang, K., & Liang, J. (2020). A dynamic emergency decision-making method based on group decision making with uncertainty information. International Journal of Disaster Risk Science, 11(5), 667–679. https://doi.org/https://doi.org/10.1007/s13753-020-00308-4
- Zhou, L., Wu, X. H., Xu, Z. S., & Fujita, H. (2018). Emergency decision making for natural disasters: An overview. International Journal of Disaster Risk Reduction, 27, 567–576. https://doi.org/https://doi.org/10.1016/j.ijdrr.2017.09.037