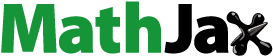
Abstract
Sci-tech and finance plays an increasingly important role and have become an important driving force in economic development. In China, the problem of insufficient financial support for sci-tech innovation is important to enterprises. According to the internal relationship between different stages of Sci-tech and the finance system, this paper is aimed at exploring the efficiency measurement method between sci-tech and finance systems. Firstly the multi-stage collaborative structure of sci-tech finance is built, where the system of sci-tech is divided into three stages including the R&D stage, transformation stage of sci-tech achievements and industrialization stage, and the financing channel is the input of the finance system into the sci-tech system at different stages. The measurement method of the multi-stage collaborative efficiency between sci-tech and finance systems is put forward by the framework of network DEA. Then, taking China as an example, we collect the information of 30 provinces and cities from 2009 to 2016 and measure the efficiency of each system and the collaborative efficiency of the both. The efficiency’s spatial correlation is tested by means of Moran index. Finally, the influencing factors of the collaborative efficiency are analyzed based on the spatial econometric regression model, which considers the financing channels and human capital. To sum up, there are significant differences in the sci-tech finance collaborative efficiency among regions in China. Among them, the collaborative efficiency of Beijing, Shanghai and Jiangsu ranks in the top three. Comparing the different stages of the sci-tech system, the commercialization stage is a weak link in many regions of China. Human capital and financing channels of sci-tech finance have different degrees of positive impact on the sci-tech finance collaborative efficiency. Among them, human capital plays a greater role in promoting the sci-tech finance collaborative development.
1. Introduction
The study on the relationship between finance and science and technology has been going on for decades of years. As long ago as 1911, Schumpeter (Citation1934) analyzed the effect of currency, credit, and interest and other various kinds of variables on business innovation and economy, and therefrom pointed out that a torrent of financial activities could pump up regional economy to a great extent by way of promoting science and technology innovation. Also, Hicks (Citation1969) surveyed the effectiveness of financial market from the perspective of science and technology innovation, which has gotten the similar result. All these time-honored studies displayed the positive effect of finance on the scientific world and constructed the research paradigm of sci-tech finance, which placed an open door for scholars in later generations.
Since then, especially when we stepped into the nineties, plenty of science and technology have been rapidly carried out, and numerous financial enterprises have simultaneously sprung up in every part of the world. This resultant force generated a series of miracles, like Silicon Valley, which has been benefited from American finance system, have astounded and inspired us again and again. As a consequence, specialists in growing numbers have conducted research to enable us to understand this kind of interaction, and to find the best way to make financial instruments to penetrate the business surface into the depth of science and technology demand.
In the study of the relation between technology development and finance, researchers paid more attention to the needs of technology development for finance, that is, the needs of technology R&D and technology output for financial tools and financial policies. Finance was regarded as one of the important influencing factors of regional, industrial and corporate innovation capabilities. Canepa and Stoneman (Citation2007), surveyed the restriction of financial factors to technological innovation in the UK, considering that the financial industry could positively affect innovative activity, especially for small- or medium-sized high-tech businesses. Using Korea as the case, Ang (Citation2010) investigated the impact of financial reforms on innovation, and the result demonstrated that financial liberalization was one of the key factors that could significantly help forward knowledge production. From the investment prospective, Arqué-Castells (Citation2012) in Spain found that business patenting production can be exceptionally increased after being funded by venture capital. Coincidentally, Popov and Roosenboom (Citation2012) gathered abundant information about venture capital and patents in Europe, referring to 21 countries and 10 industries in the period of 1991–2005. The study showed that venture capital which played an important role in stimulating innovation, largely appeared in countries with better commercial environment. Chowdhury and Maung (Citation2012) conducted a survey among 70 countries in the range of 1997–2006, in an effort to analyze whether financial market could enhance the effectiveness of research and development (R&D). Likely, the work indicated that a sound financial market and well-designed government policies would improve R&D efficiency by reducing information asymmetry. In Italy, Giannetti (Citation2012) explored the relationship between firms and banks. In short, this study suggested that banks should take different approaches to support different firms, and posited that main banks’ financial decision could positively influence the capability of business innovation. Based on India’s experience, Ang (Citation2014) and Sasidharan et al. (Citation2015) shed light on the role financial sectors played in technological innovation and the correlation between financing constraints and R&D, respectively. The general conclusion was that for promoting new knowledge production, a series of financial reforms and government interventions might help. After researching 32 developed or emerging countries, Hsu et al. (Citation2014) considered that well-developed equity markets would dramatically benefit companies which are technology-oriented and are indifferent and insensitive to external finance, yet the role credit markets played seemed to be reversed. Minetti and Herrera (Citation2015) surveyed Italian manufacturing firms in detail and found that companies with longer credit relationships were more willing to carry out innovation activities and the most noticeable effect of banks was at the stage of technology introduction, rather than technology assessment and development. Da Fonseca and Veloso (Citation2018) took the USA and Brazil as cases to discuss the influence government exerted in supporting science, technology and innovation. Comin and Nanda (Citation2019) investigated the impact of financial market development on the proliferation of 16 major technologies in 17 countries, found that the development of financial markets plays a key role in promoting the early stage of technological innovation. The greater the depth of the financial market, the faster technology spreads. Tolliver et.al (2021) studied the relationship between green innovation and green finance in Japan, South Korea and China, and discovered that finance and innovation have mutually reinforcing effects. Zhu et al. (Citation2020) research in 50 countries showed that the expansion of the financial industry may damage innovation activities and thereby damage innovation-led growth.
These researchers also concurred with those of China. As a distinctive fast-growing economy, Chinese financial and scientific activities have been positively conducted, which appeals to large numbers of researchers from all over the world. Through investigating more than 120,000 unlisted Chinese companies (including foreign-funded ones) from 2000 to 2007, Guariglia and Liu (Citation2014) looked into the matter whether financing constraints could affect innovation activities, and verified that internal finance is the main factor limiting the innovation of Chinese corporation, especially small or private firms. By virtue of game models, Li found that among various financial instruments, innovation fund, bond, and equity were the main contributors for company innovation and innovation fund was the most significant one among the three troika members. Qian and Zhang (Citation2017) collected the data of listed companies in Chinese stock markets, indicating that the development of technology finance was an important driving force for tech firms’ R&D investment. As an effort to explain China’s sustained and high-level economic growth, Söderlund and Tingvall (Citation2017) tried to reveal the relationship between finance and development at the provincial level. The result documented that free capital flow, sound finance system and regulatory system, and marketization were principally the levers for boosting economy, and in some ways this effect was even sharper in less-developed regions. Hou and Song (Citation2020) verified that finance improves the efficiency of regional R&D innovation by affecting the rate of return on capital and R&D investment.
The development of science and technology needs the financial industry to provide funds and capital input. Similarly, the development of science and technology also promotes the development of the financial industry. In recent years, “FinTech,” a contraction of “Financial technology,” has attracted increasing attention. Arner et al. (Citation2016) referred FinTech to technology enabled financial solutions. It is often seen today as the new marriage of financial services and information technology. Moreover, a group of researchers considered cooperation among finance, commercial activities, and scientific research as an ecosystem (Lee & Shin, Citation2018; Mackenzie, Citation2015), which mainly referred to the basic concepts of this kind of study and how to bring into full play the advantages of each part in practice. Palmie et al. (Citation2020) illustrated the impact the FinTech ecosystem has had on disrupting the financial services industry. Hendrikse et al. (Citation2020) believed that FinTech plays an important role in the formation of the financial ecosystem. Yang et al. (Citation2021) emphasized the impact of FinTech on the profitability, financial innovation and risk control capabilities of commercial banks.
In summary, today there are numerous papers and studies taking part in sci-tech and finance, and this kind of research is all but conducted through quantitative models to disclose the relationship, or even articulation, between financial activities and science and technology. The general conclusions of them are as follow. First, finance could exceedingly support and give impetus to the development of commercial industries and scientific research by means of participating into risk management, supervision, education, informatization, and investment, and other various kinds of processes (Sokol, Citation2017). Besides, science and technology can in turn support the financial industry to a large extent, providing theoretical or practical methodologies, and investment opportunities from which modern finance can be benefited. Second, in this process, government and regulatory institutions have often played a pivotal role that could largely facilitate and stimulate the communication and cooperation of each part.
At this point, no one would seriously question the co-existence and mutual promotion between finance and science and technology. But so far, at least to our knowledge, there is another problem truly important, yet barely noticed, i.e., what extent to which the two systems can affect each other. Without too much exaggeration, this issue might have a deep reality-oriented meaning, because it can straightforwardly instruct us how to allocate resources, how to formulate collaborative policies and whether this kind of bilateral or multilateral cooperation is necessary.
China’s national strategic plan emphasizes the integration of technology and finance, and proposes the specific implementation plans to promote innovation in financial services technology (in 2010, the Ministry of Science and Technology and other five departments jointly issued the "Promoting the Integration of Technology and Finance Pilot Implementation Plan"). Science-technology finance (Sci-tech finance), which serves technological innovation has been developed under actual demand and policy promotion. However, under the current circumstances, the development of financing channels in China's Sci-tech finance is not satisfactory: The role of venture capital investment is not fully played, the development of technology capital markets is limited, and the types of technology investment products of financial institutions are small and the service form is single. How to play the value of Sci-tech finance is an important issue? This requires several questions to be resolved: How to establish the collaborative relation between Sci-tech and finance? How to measure the collaborative efficiency of Sci-tech and finance? What are the problems with the sci-tech system and the finance system in the collaborative relationship? What are the influencing factors of the collaborative efficiency of Sci-tech and finance? Therefore, it is necessary and important to explore the collaborative structure of sci-tech finance, quantitatively disclose each system’s efficiency and the collaborative efficiency of the both, and then to analyze what are the influencing factors of the collaborative efficiency.
With this in mind, we researched massive documents about efficiency measurement, a really age-old field in operations research (Farrell, Citation1957), and finally chose data envelopment analysis (DEA) as the key technique. Briefly speaking, DEA is a well-known math model originated in the late 1970s, which could evaluate the individual efficiency of a decision making unit (DMU) within a group of interest that perform in a certain practical field (Castelli et al., Citation2004). Since the seminal work of (Charnes et al., Citation1978), DEA has evolved at a fast speed (Lampe & Hilgers, Citation2015), spawning a big, methodological system (Cook & Seiford, Citation2009; Liu et al. Citation2013; Seiford, Citation1996), and has been gradually applied to various kinds of social science areas and beyond (Liu et al. Citation2013), which can be seen as a spear head in efficiency measurement. Moreover, in the DEA family, network DEA, carried out by Färe and Grosskopf (Citation1996), is definitely a super star in recent years (Kao, Citation2014). For example, Cook et al. (Citation2010) systematically summarized the two-stage DEA model and performed efficiency decomposition to evaluate the efficiency of non-life insurance companies. Luo et al. (Citation2012) and Matthews (Citation2013) provided empirical analyses of the efficiency of Chinese commercial banks, from the perspective of index selection and risk management, respectively. Wang et al. (Citation2014) conducted the bank efficiency evaluation with the network DEA model with undesired outputs. Iftikhar et al. (Citation2016) used the network DEA to divide the economic operation system into two stages of economic output and resource allocation. Fukuyama and Matousek (Citation2017) applied the network DEA to exploring the relationship between bank income function and non-performing loans. Still, there are lots of studies dealing with efficiency evaluation, like Badiezadeh et al. (Citation2018) for supply chains, An et al. (Citation2019) for resource sharing and payoff allocation, Losa et al. (Citation2020) for the efficiency of airline groups, etc. In conclusion, it can be seen that network DEA method is widely used, which can be used to measure system efficiency with multi-stage characteristics of internal structure and internal subsystem efficiency.
As mentioned above, finance and science and technology are entangled with each other, together forming a new sophisticated social system. The process of technological innovation can be divided into three stages, namely research and development, the commercialization of sci-tech achievements and sci-tech industrialization. Finance plays an important role in this process. Financial institution technology investment, venture capital investment and sci-tech capital market investment support the realization of the three stages. We need to explore: How do the three stages of the sci-tech system relate to the finance system? What is the role of the finance system in each stage? Apparently, network DEA can be seen as an instrument to help us seeing through this “black box” and then perceiving the essence of the collaboration between the two systems. Therefore, in this work, based on Chinese experience and the fundamental knowledge of network DEA, we creatively develop a new DEA method for scaling the collaborative efficiency of sci-tech finance.
This method fully considers the operating mechanism of sci-tech finance in time sequence and divides the collaborative system into three stages, i.e., R&D, commercialization, and industrialization, so as to span all aspects of the collaboration. Through such design, we hold that this method, in some ways, could enrich the research method of sci-tech finance, and provide a model for studying the multi-system multi-stage collaborative structure, which can push forward the sci-tech finance research and DEA methodology at the same time.
Taking this brief introduction as Section 1, the work in Section 2 introduces the design proposal, process, and operational guide of the DEA method in detail. Section 3 measures the sci-tech finance collaborative efficiency and subsystem efficiency at the provincial level from 2009 to 2015 in China, and then Section 4 analyzes the influencing factors of sci-tech finance collaborative efficiency, followed by Section 5, which summarizes the entire work, and provides several pertinent suggestions for all sides of sci-tech finance activities.
2. Methodology and data
2.1. The collaborative structure of sci-tech finance
The economy system is a collection of interrelated members, which is quite similar to the biological community. In order to exist and grow up, each member of the community requires both assistance and protection from other members, which accelerates the share of information and other resources. Sci-tech system and finance system are two key subsystems in the economy system, and there is an interaction between them. If the elements within these two systems can form a mutually-promoting and mutually-affected relationship during the development process, these two systems can promote the sustainable development of the economic system. When the two systems form such a relationship, it can be called a sci-tech finance collaborative structure. This structure is a platform for interaction between the sci-tech system and the finance system, enabling various elements to play a greater role and realizing an overall function which is greater than the sum of each part function.
In the study of the collaboration between the finance system and the sci-tech system, existing literature used to integrate R&D stage and commercialization stage which is then considered as a black box. In fact, in the sci-tech system, scientific institutions have different development stages ranging from the initial technical research to industrialization in accordance with the maturity of products. Based on the experience of Chen et al. (Citation2018), this paper divides the development of the sci-tech system into three stages, i.e., R&D, commercialization, and industrialization. In this way, the cooperation of sci-tech finance systems across different stages in different regions is studied.
The first stage, R&D, mainly focuses on new technologies. After successful operation, these technologies are expected to be appeared as papers and patents which can be used by scientific institutions and enterprises to generate new products. The research institutions provide platforms and environment for sci-tech innovation. And research institutions are also willing to invest human resources to support the research and development. Therefore, this paper takes the number of sci-tech practitioners and research institutions as the measurement index of the input factor of the R&D stage. In the R&D stage, there are two types of achievements: research papers and patents, which are the output of R&D, as well as the input of commercialization stage. The number of patents includes the number of patent grants and patent applications. To fully reflect the level of innovation, we select the number of patent applications as the output factor of the R&D stage.
The second stage is the commercialization of sci-tech achievements. This stage mainly refers to that scientific institutions or enterprises turn technologies into products which are profitable. If a product could make a profit, it also completes this stage. Therefore, this paper mainly chooses the technical market turnover as the output variable for measuring this stage.
The third stage is industrialization. At this stage, new enterprises continuously come into the products’ market which then plays a pivotal role in the foundation of high-tech industries. Sci-tech innovation needs all kinds of sci-tech finance services. At the same time, enterprises may raise funds to develop the high-tech industry through capital market. The market is continuously expanded and progressively form related high-tech industries. Therefore, this paper mainly uses high-tech output value and high-tech product exports to measure the output at this stage.
2.2. Indicator selection of sci-tech system and finance system
2.2.1. Input indicators and output indicators of finance system
The input of the finance system mainly includes human resources and fixed assets of financial industry. Human resources are represented by people who work in the financial industry. Fixed assets are represented by the stock of financial fixed assets. Since the investment of the fixed assets listed in the China Statistical Yearbook is each year’s newly increased flow, the perpetual inventory method is adopted for conversion. The calculation formula is where
represents the stock of financial fixed assets in period t,
usually set as 10%, means the depreciation rate, and
is the fixed capital input flow in a period lagging behind.
The output indicators of finance system include final output indicators and intermediate output indicators of the finance system. The final output indicator for the finance system includes growth in the financial sector, which is from the China Statistical Yearbook. The intermediate output indicators of the finance system are the indicator of input to sci-tech system, which mainly includes three financing channels, include the financial institutions technological investment, the venture capital investment and the sci-tech capital market investment. The financial institutions technological investment is measured by miscellaneous investment written in the China Statistical Yearbook (Sun & Wu, Citation2017). The venture capital investment is measured by venture capital investment intensity, which is computed through dividing venture investment by GDP and regularly published by Venture Capital Development in China. The sci-tech capital market investment can be regarded as the total financing of technology companies publicly listed on the Shenzhen Stock Exchange and Shanghai Stock Exchange (Zhang & Zhao, Citation2015). For the definition of technology companies, we refer to the criteria of the Analysis Report on Technology Sectors of Listed Companies for sample selection.
2.2.2. Input indicators and output indicators of sci-tech system
The input of sci-tech system mainly includes the number of sci-tech practitioners and research institutions (Cao et al., Citation2011). Because increasing the number of sci-tech practitioners and investment in research institutions will improve the efficiency of enterprise R&D. And the quality of sci-tech practitioners has an important impact on the efficiency of enterprise innovation. Both indicators are derived from China National Bureau of Statistics.
The output of sci-tech system is comprised of the output indicators of three stages: R&D, commercialization, and industrialization.
At the R&D stage, scientific research institutions and technology companies mainly focus on basic research and common technology research, to create papers and patents which can promote the development of new products. Therefore, the output of this stage is measured by the number of patents and papers. Both indicators are derived from China Science and Technology Statistical Yearbook.
At the commercialization stage, enterprises transform patented technologies into new products to create revenue for the enterprises. Therefore, the output of this stage is measured by the new products sales revenue and the technical market turnover. Both indicators are derived from China Science and Technology Statistical Yearbook.
At the industrialization stage, new enterprises will continue to enter the market, and the market scale will gradually expand, thereby forming a science and technology industry, creating high-tech output value and exporting to the outside world. Both indicators are derived from National Bureau of Statistics of China.
The input indicators and output indicators of finance system and sci-tech system chosen in this paper are shown in .
Table 1. Input indicators and output indicators of finance system and sci-tech system.
2.3. Measurement model of collaborative efficiency of sci-tech finance
Based on the indicator selection of sci-tech system and finance system, and considering the internal operation mechanism of sci-tech system and finance system, we construct the sci-tech finance collaborative system in .
According to the collaborative structure of finance system, sci-tech system, and index system, we depict the multi-stage collaborative structure of sci-tech finance in :
Figure 2. Collaborative structure of sci-tech finance.
Source: the author based on the variable relation.
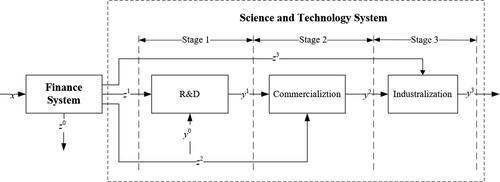
In , the input variables of the finance system (financial fixed assets and the number of financial practitioners) are set as x; the output variables of the finance system are set as z, and the final output variables of the finance system (financial industry growth) are set as z0; The intermediate output variables of the finance system (financial institution technology investment, venture capital investment, and sci-tech capital market investment) are respectively set as z1, z2, and z3.
The input and output variables of the sci-tech system are set as y. The input variables (number of sci-tech practitioners and scientific research institutions) of the R&D stage are set as y0; the output variables of the R&D stage (the number of patents and papers) are set as y1; the output variables of the commercialization stage of sci-tech achievements (new product sales revenue and technology market turnover), which also are the input variables of industrialization stage, are set as y2. The output variables of industrialization stage (hi-tech product exports and hi-tech output) are set as y3.
In order to further introduce the sci-tech finance multi-stage collaborative efficiency measurement model, the variables are set as shown in .
Table 2. Notation and definition.
shows that represents the multi-stage collaborative efficiency of sci-tech finance of measurement object k;
represents the rth input variable of measurement object k in the finance system; when p = 0,
represents the sth input variable measurement of object k in the scientific and technological development stage; when p = 1,2,3,
represents the sth output variable of the measurement object k in the p stage of the science and technology system; when q = 0,
indicates that the measurement object k is tth final outputs in the finance system; when q = 1,2,3,
represents the tth input variable of the measurement object k in the finance system's qth stage in the sci-tech system; and,
are weight coefficients of
respectively.
In the case of constant returns to scale, the DEA model of the collaborative efficiency can be described as:
(1)
(1)
Expanding output is focused when inputs are relatively determined in a multi-stage collaborative system of technology and finance. In the objective function of model (1), and
are selected respectively as the output combination of the finance system and the scientific and technological system as the objective function.
Among the constraints, the first constraint represents constraints on the input combination of finance system inputs The second constraint represents the constraints on the input combination of the technology system
and
The third constraint represents the constraints of the combination of inputs
on the outputs of finance system
The fourth constraint represents the combination of the input of the technology system
and
on the output combination
The fifth constraint means that all the weight coefficients
and
are positive.
The measures of finance system efficiency are derived from the collaborative efficiency model of sci-tech finance, which mainly extract input variables and output variables related to the finance system from Model (1). The measurement model is set as:
(2)
(2)
In Model (2), represents the finance system efficiency of measurement object k. For the output-oriented model, the output combination
is selected as the objective function. The first constraint in the constraint condition represents the constraint on the input combination
of the finance system, and the second constraint represents the relationship between on the input combination
and output combination
of the finance system.
The efficiency measure of the sci-tech system is derived from Model (1), which mainly extracts input variables and output variables related to the sci-tech system. The measurement model is set as follows:
(3)
(3)
In Model (3), represents the sci-tech system efficiency of measurement object k. For the output-oriented model, the output combination
is selected as the objective function. The first constraint in the constraint condition represents the constraint on the input combination
of the sci-tech system, and the second constraint represents the relationship between on the input combination (
and
) and the output combination
of the sci-tech system.
3. Collaborative efficiency measurement results and analysis
3.1. Sci-tech finance collaborative efficiency
In accordance with model (1), (2) and (3), by limiting the input variables and maximizing the output variables, sci-tech finance collaborative efficiency (It is referred to as sci-tech finance efficiency in the form) and its subsystem efficiency can be measured, which are shown in . The closer the value approaches 1, the higher the efficiency would be.
Table 3. 2009-2016 China's provincial sci-tech finance collaborative efficiency and subsystem efficiency.
As can be seen, 30 provinces and cities’ collaborative efficiency largely range from 0.2 to 1, which are considered very high. Beijing, Shanghai, and Jiangsu top the list, whose three kinds of efficiency are wholly effective. And the efficiency of its finance system efficiency and sci-tech system efficiency also maintain a high level. The finance system efficiency of Tianjin and Guangdong also maintain a good development trend. There is a certain gap between sci-tech finance efficiency and frontier level in central and western regions. The efficiency difference is more than 0.5, the sci-tech system efficiency still needs to be improved.
3.1.1. The spatial analysis of sci-tech finance collaborative efficiency
In , this paper further draws the spatial distribution of the collaborative efficiency of sci-tech finance in China from 2009 to 2016, in order to visually analyze the distribution of sci-tech finance efficiency in various provinces and cities:
3.1.2. The trend analysis of sci-tech finance collaborative efficiency
In , during the sample period, the three kinds of efficiency has generally soared. The efficiency of sci-tech system and finance system have increased by more than 15%. Among them, the average value of the collaborative efficiency in 2015 has reached 0.603, an increase of 10% compared with 2009, indicating that the overall collaboration of sci-tech finance is good.
Figure 4. National sci-tech finance collaborative efficiency mean line chart.
Source: the author based on the original data and empirical results.
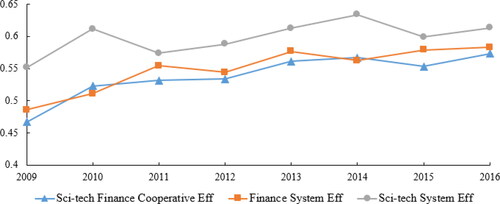
In general, all regions in China are still at the stage that research creation and value are derailed. This may be a major challenge for China during the period of economic restructuring. So, it is urgent to promote the commercialization of sci-tech achievements. The commercialization of sci-tech achievements is a systematic project, which should take not only the improvement of efficiency in each stage but also the coupling of the whole stage into consideration.
3.2. The analysis of sci-tech system and finance system
3.2.1. The comparison of the efficiency of R&D and commercialization in sci-tech system
In the period of sci-tech R&D, all regions make progress in the transformation efficiency of sci-tech achievements at present, and achieve a high efficiency value. Among them, the sci-tech R&D efficiency of 20 provinces and cities are higher than the commercialization efficiency of sci-tech achievements, indicating that China have been made some achievements in the research results. China has made some achievements in research achievements such as papers and patents, but the efficiency in the transformation stage of scientific and technological achievements is relatively low. It shows that the transformation of scientific and technological achievements is a weak link in all regions of China, and there is a certain gap between the transformation and the research and development stage of science and technology. Therefore, China needs to further strengthen the construction of institutions to assist the transformation of scientific and technological achievements, and formulate supporting strategies, so as to solve the problems of insufficient transformation of scientific and technological achievements and subsequent industrialization as soon as possible.
From the , it can be seen that finance system efficiency and sci-tech system efficiency are similar for the leading areas. And the two subsystems maintain a balanced development relationship. For the relatively backward areas in the coordinated development of science-technology and finance, sci-tech system efficiency is lower than finance system efficiency under the condition of low efficiency value of the two systems. From the output point of view, the development of science-technology system generally needs a relatively long period of time, and the centralized investment needs a certain period of time to show results. In terms of input, the construction of scientific research institutions and the talents of scientific- technological innovation also need long-term policy and financial support. Therefore, the coordinated development of science-technology and finance in backward areas needs to focus on promoting the development of science and technology system.
3.2.2. The insufficient output of financing channels in finance system
In order to further explore the impact of output variables on efficiency values and adjust the allocation of resources for the lack of output, this paper analyzes the part of the output indicators that are insufficient when generating efficiency values. The situation of insufficient DEA output in different regions from 2009 to 2016 is shown in .
Table 4. Insufficient DEA output analysis.
Since the collaborative efficiency of sci-tech finance in Beijing, Shanghai and Jiangsu has reached 1, there is no shortage of output. In the finance system, the shortage of financial institution technology investment mainly restricts the coordinated development of China's sci-tech finance, and the average output shortage rate has reached 0.209. Insufficient financial institution technology investment in Hunan, Jilin, and Inner Mongolia have reached 0.627, 0.431, and 0.428 respectively. It is necessary for these provinces to financial institution technology investment. Heilongjiang, Qinghai, and Guizhou need to strengthen venture capital investment to enhance the collaborative efficiency. In the sci-tech system, compared with the number of papers and granted patents, the technical market turnover is the main factor that restricts the coordinated development of sci-tech finance in China. The average output shortage rate has reaches 0.184, indicating that China's sci-tech system still need to be improved in the stage of commercialization.
3.3. Spatial correlation analysis of sci-tech finance collaborative efficiency
This paper uses the exploratory spatial data method, Moran index, in order to study whether the variables have spatial correlation and aggregation.
The value of the Moran index ranges from −1 to 1. The Moran index larger than 0 indicates that the coordinated development of sci-tech finance in China is spatially significant. The Moran index closer to 1 indicates that the spatial difference is small. The Moran index smaller than 0 indicates spatial negative correlation, and the region is significantly different from the surrounding area. The Moran index closer to −1 indicates that the spatial difference is big; the Moran index equal to 0 indicates that the variables are spatially irrelevant.
The represents the specific Moran coefficient value of sci-tech finance collaborative efficiency from 2009 to 2016. The value of the Moran index fluctuated within the range of 0.14 to 0.2, all of which maintained high significance. Among them, the index value in 2012 and 2015 maintained a high level, which reflects that China's multi-stage collaborative efficiency in science and technology and finance as a whole maintained a certain correlation.
Figure 6. The Moran Index value line chart of sci-tech finance collaborative efficiency.
Source: the author based on the original data and empirical results.
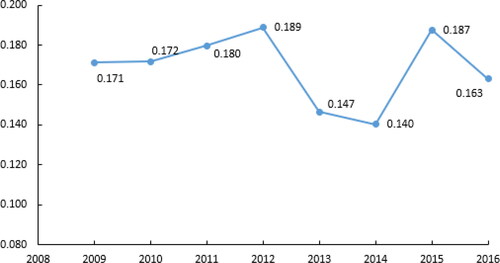
From the , the Beijing-Tianjin area and the Yangtze River Delta have a high gathering effect, while the central China and western regions of China have a low gathering effect. Therebefore, the spatial correlation of sci-tech finance in China can be taken into the consideration of promoting the collaborative development of sci-tech finance in the central and western regions.
4. Analysis of influencing factors of the collaborative efficiency
4.1. Econometric regression model
To improve the sci-tech finance multi-stage collaborative efficiency, it is necessary to analyze its influencing factors. For the development of the sci-tech finance system, human capital and financing channels of sci-tech finance are obviously direct influencing factors. This paper constructs a spatial econometric regression model to study the influencing factors of sci-tech finance collaborative efficiency. The explanatory variables of the model are selected as follows:
Related variables of human capital investment: Human capital plays an important role in the development of sci-tech system and finance system. The practitioner income has greatly affected the choice of practitioner (Zhao & Zhang, Citation2010). Besides, educational level of the whole society reflects the quality of human capital (Fan & Zhou, Citation2012), which can be measured as the number of students in high schools per 100,000 population in each region. Therefore, related variables of human capital investment are selected as: financial practitioner income, sci-tech practitioner income, and the education level.
Related variables of financing channels for sci-tech finance: In the sci-tech finance multi-stage collaborative structure, the finance system conducts in-depth collaboration with the three stages of the sci-tech system through financing channel, which reflects technology capital market investment, venture capital investment and financial institution technology investment play the important role in promoting the sci-tech finance collaborative development (Hussain et al., Citation2017). Therefore, related variables are selected as: technology capital market investment, venture capital investment and financial institution technology investment.
Control variables: Existing literature generally selects macroeconomic indicators such as degree of openness, economic development level, and economic structure as control variables when researching technological innovation and financial development. Therefore, we choose the economic development level, the openness, and the economic structure as the control variables of the influencing factor analysis model.
The influencing factors of the sci-tech finance collaborative efficiency in this paper are shown in .
Table 5. Sci-tech finance collaborative efficiency influencing factors.
The specific regression analysis formula is shown as follows:
(4)
(4)
In this equation, k represents different provinces, t represents different years, represents the sci-tech finance multi-stage collaborative efficiency in province k in year t;
represents the constant term,
represents the regression coefficient of the related variables of human capital,
represents the regression coefficient of the related variables of financing channels,
represents the regression coefficient of the control variable;
represents the financial practitioner income,
represents the technology practitioner income,
represents the education level,
represents the sci-tech capital market investment,
represents the venture capital investment,
represent the financial institution technology investment,
represents the economic development level,
represents the openness,
represents economic structure.
is a random error term.
4.2. Empirical results and analysis
Based on the data of 30 provinces and cities in China from 2009 to 2016, we carry out the descriptive statistical analysis of the sample data. The results are shown in the :
Table 6. Descriptive statistical analysis.
From , we can find that the average value of collaborative efficiency of sci-tech finance is 0.57, the maximum value is 1, the minimum value is 0.14, and the standard deviation is 0.26, indicating that the difference different kinds of efficiency exists in various regions of China.
For panel data, individual characteristics cannot be ignored. The statistic value of Hausman test is 68.44, and the P-value is 0, which means the individual characteristics need to be considered. Therefore, this paper uses a fixed effect model. For each influencing factor, the explanatory variables are the multi-stage collaborative efficiency the sci-tech finance efficiency, finance system efficiency and sci-tech system efficiency. The calculation results of the three models are obtained as shown in .
Table 7. The regression result of the influence factor of sci-tech finance collaborative efficiency.
Among the variables used in this paper, the human capital input index presents the following characteristics:
The regression coefficient of the income of sci-tech practitioners is 0.274, which is acceptable at the level of 10%, indicating that improving the income of sci-tech practitioners will promote the coordinated development of sci-tech finance from the subsystem to the overall system.
There is a significant positive correlation between the income of financial practitioners and the collaborative efficiency of sci-tech the finance system, but the correlation is not significant, which indicates that the investment of financial human capital has not effectively promoted the improvement of the industrialization efficiency of sci-tech achievements.
Education level has a positive correlation with the collaborative efficiency and subsystem efficiency of sci-tech finance. Therefore, provinces should focus on improving the quality of sci-tech human resources, and at the same time improve the management ability of human capital, so that high-skilled workers and researchers can bring their scientific research ability into full play.
Relevant indicators of financing environment show the following characteristics:
Sci-tech investment has a significant positive effect on the development of China's sci-tech finance. In terms of basic scientific research with high investment and great social influence, market players are generally unable to play a role, while government financial institutions, i.e., public players, can support the lack of investment and market failure, vigorously promote the process of scientific research and innovation, and improve the efficiency level of scientific and financial development.
Venture capital investment has a significant positive effect on the development efficiency of China's sci-tech finance. Venture capital not only provides financial support for start-ups, but also provides professional management advice for them with its unique professional advantages, which can promote the professional management and use of financial resources of sci-tech, and lay a foundation for the development of sci-tech finance.
Investment in sci-tech capital market has a significant positive effect on the development efficiency of China's sci-tech finance. The funds for the sci-tech capital market would strengthen the supervision of the sci-tech innovation activities from the outside of the main body of sci-tech innovation, improve the transparency of the use of funds, promote the spontaneous formation of a wide range of supervision within the region, and improve the efficiency of the use of sci-tech finance resources.
5. Conclusion and suggestion
Based on China's 30 provinces or cities from 2009 to 2016 as the research object, this paper sets up finance multi-stage collaborative structure of sci-tech finance and uses the network DEA to measure the input and output efficiency, and finally uses the spatial econometric regression model on the influence factors of the sci-tech finance research. The study found that there is a large space for the enhancement of the collaborative efficiency of China's sci-tech finance system, and there are significant differences among different regions. The efficiency value at the stage of the commercialization of sci-tech results in different regions is significantly lower than that at the stage of R&D, which reflects that the achievement commercialization of China's sci-tech system needs to be improved. From the perspective of space, it shows a good spatial correlation, and the correlation increases year by year. From the perspective of time, the efficiency of each system shows an increasing trend year by year. Human resources and financing environment have different degrees of positive impact on the collaborative efficiency of sci-tech finance, among which the human capital investment for scientific researchers has a greater role in promoting the collaborative development of sci-tech finance.
The contribution of the paper is to regard sci-tech finance as a system, taking into account the interdependence and mutual promotion relationship between various components in the system, and to use the network DEA method to measure the collaborative efficiency of Sci-tech finance. Although there have been studies (Ang, Citation2010, Citation2014; Comin & Nanda, Citation2019; Hsu et al., Citation2014; Tolliver et al., Citation2021), which put Sci-tech and finance together for research, they did not consider the inherent aspects of operational structure and collaborative relationship between the sci-tech system and the finance system. In addition, the regression analysis method generally used when studying the relationship between sci-tech and finance, which focuses on the idea of sci-tech’s needs for finance, and does not reflect the collaborative between sci-tech and finance. Technological innovation requires the financial system to provide venture capital and technology financing credit. At the same time, the transformation and industrialization of scientific and technological achievements will further promote the development of financing channels for sci-tech finance. The network DEA method adopted in the paper is suitable for describing the collaborative relationship between the finance system and the sci-tech system.
In the collaborative development system of sci-tech finance, the government, enterprises and intermediary agencies play different and important roles. Therefore, this paper puts forward relevant suggestions for each of them.
(1) As the guider, local governments can establish the guiding mechanism for enterprises. Local governments should increase the mentoring mechanism for science and innovation enterprises. Although all science and innovation enterprises have excellent entrepreneurial ideas and strong motivation, they lack experience in operation. Therefore, enterprise mentors with business operation experience are required to provide enterprises suitable development paths and marketing capabilities that are widely accepted in the market. Sci-tech enterprise should make full use of these experience of successful entrepreneurs and form development planning team of investors for the enterprise to provide substantial guidance services to improve the operation efficiency and optimize resources configuration.
Taking into account the expansion of innovation resources but the lack of transformation capabilities in the sci-tech systems of China's provinces and cities, the government should optimize corresponding policies and adjust resource allocation issues. Based on the sci-tech finance collaborative network, the government strengthens resource allocation and resource sharing strategies. Specific measures include: On the one hand, invalid input should be avoider. Government should increase the penetration of social networks by increasing technology and financial participants, and strengthen multi-party collaboration. Then, sci-tech finance participants could obtain information directly or indirectly related to entrepreneurship from professional service institutions, universities and research institutions, venture investors and other stakeholders. On the other hand, a good atmosphere of sci-tech finance should be established. Government should strengthen the direct contact between the surrounding network nodes and itself, and instill information about new ventures into stakeholders to promote the society’s understanding and support for technological innovation. At the same time, government also need strengthen the risk investment of financial institutions in areas with weak foundations to alleviate the regional gap in the multi-stage collaborative efficiency of China's sci-tech finance. The sci-tech finance collaborative efficiency needs to strengthen the transparency of technology capital and create an excellent regulatory environment. From the regression results, the expansion of venture capital investment scale should be encouraged and the standardized management should be implemented with strong policy means. It will finally lay the foundation for the efficient development of sci-tech finance.
(2) Sci-tech financial agency play an important role as media for the government to support the process of sci-tech development. The generation and transformation of sci-tech achievements need the long-term support of financial institutions, the capital market provides financing for scientific experiments and R&D, and the development of the insurance industry also provides a strong economic support for high-risk scientific research activities. The construction of capital market should persist in the systematic and long-term strategy, constantly improve the multi-level capital market, strengthen the risk guarantee system of sci-tech insurance, and assist the development of sci-tech industry and the transformation of sci-tech achievements.
The intermediary institutions of sci-tech finance should explore the mode of sci-tech finance and broaden the financing channels. Innovative entrepreneurship is extremely risky, so it is urgent to have technology and financial products that match it at all stages to spread risk. Financial institutions should not only effectively identify the development potential of enterprises, but also make compatible credit policy standards and risk control program. Sci-tech insurance should be constantly improved and innovated to improve the coverage of sci-tech insurance, and insurance products should be adapted to sci-tech risk factors. In addition, it is necessary to train and find inter-disciplinary employees for sci-tech finance to improve the service quality, and create a good environment for sci-tech finance.
(3) Enterprises play an important role in the commercialization of scientific research results, so they need to strengthen their own advantages and grasp the opportunity of innovation. The sci-tech enterprise itself unifies own industry superiority and uses the local resources appropriately to enhance the industrialization efficiency. For example, the heavy industry in the northeast should inject sci-tech vitality into the basic industries, adhere to the position of their own industrial areas, and promote the coverage of sci-tech innovation from point to point in order to achieve high-quality development in backward areas.
The multi-stage collaborative efficiency of sci-tech finance reflects the existence of certain spatial correlations among various regions in country. The eastern technological and financial resources should be appropriately tilted to the western part. For enterprises, appropriate expansion of the business area and drawing on technology and financial support from advanced areas is conducive to their own efficient development. At the same time, enterprises should pay attention to the construction of reasonable training and incentive mechanism of sci-tech finance talents, in order to give full play to the role of human resources.
Disclosure statement
No potential conflict of interest was reported by the authors.
Additional information
Funding
References
- An, Q., Wen, Y., Ding, T., & Li, Y. (2019). Resource sharing and payoff allocation in a three-stage system: integrating network DEA with the Shapley value method. Omega, 85, 16–25. https://doi.org/10.1016/j.omega.2018.05.008
- Ang, J. B. (2010). Research, technological change and financial liberalization in South Korea. Journal of Macroeconomics, 32(1), 457–468. https://doi.org/10.1016/j.jmacro.2009.06.002
- Ang, J. B. (2014). Innovation and financial liberalization. Journal of Banking & Finance, 47, 214–229. https://doi.org/10.1016/j.jbankfin.2014.07.007
- Arner, D., Barberis, J., & Buckley, R. (2016). The evolution of FinTech: A new post-crisis paradigm? Georgetown Journal of International Law, 47(4), 1345–1393.
- Arqué-Castells, P. (2012). How venture capitalists spur invention in Spain: Evidence from patent trajectories. Research Policy, 41(5), 897–912. https://doi.org/10.1016/j.respol.2012.01.008
- Badiezadeh, T., Saen, R. F., & Samavati, T. (2018). Assessing sustainability of supply chains by double frontier network DEA: A big data approach. Computers & Operations Research, 98, 284–290. https://doi.org/10.1016/j.cor.2017.06.003
- Canepa, A., & Stoneman, P. (2007). Financial constraints to innovation in the UK: Evidence from CIS2 and CIS3. Oxford Economic Papers, 60(4), 711–730. https://doi.org/10.1093/oep/gpm044
- Cao, H., You, J., Lu, R., & Chen, H. (2011). Empirical research on finance of science and technology development index in China. Chinese Journal of Management Science, 19(3), 134–140.
- Castelli, L., Pesenti, R., & Ukovich, W. (2004). DEA-like models for the efficiency evaluation of hierarchically structured units. European Journal of Operational Research, 154(2), 465–476. https://doi.org/10.1016/S0377-2217(03)00182-6
- Charnes, A., Cooper, W. W., & Rhodes, E. (1978). Measuring the efficiency of decision making units. European Journal of Operational Research, 2(6), 429–444. https://doi.org/10.1016/0377-2217(78)90138-8
- Chen, X., Liu, Z., & Zhu, Q. (2018). Performance evaluation of China's high-tech innovation process: Analysis based on the innovation value chain. Technovation, 74-75, 42–53. https://doi.org/10.1016/j.technovation.2018.02.009
- Chowdhury, R. H., & Maung, M. (2012). Financial market development and the effectiveness of R&D investment: Evidence from developed and emerging countries. Research in International Business and Finance, 26(2), 258–272. https://doi.org/10.1016/j.ribaf.2011.12.003
- Comin, D., & Nanda, R. (2019). Financial development and technology diffusion. IMF Economic Review, 67(2), 395–419. https://doi.org/10.1057/s41308-019-00078-0
- Cook, W. D., & Seiford, L. M. (2009). Data envelopment analysis (DEA)-thirty years on. European Journal of Operational Research, 192(1), 1–17. https://doi.org/10.1016/j.ejor.2008.01.032
- Cook, W. D., Liang, L., & Zhu, J. (2010). Measuring performance of two-stage network structures by DEA: a review and future perspective. Omega, 38(6), 423–430. https://doi.org/10.1016/j.omega.2009.12.001
- Da Fonseca, R. S., & Veloso, A. P. (2018). The practice and future of financing science, technology, and innovation. Foresight and STI Governance, 12(2), 6–22. https://doi.org/10.17323/2500-2597.2018.2.6.22
- Fan, H., & Zhou, D. (2012). Regional science and technology innovation efficiency evolution and its affect factors in Chinese provinces. Science Research Management, 33(1), 10–18.
- Färe, R., & Grosskopf, S. (1996). Intertemporal production frontiers: With dynamic DEA. Kluwer Academic.
- Farrell, M. J. (1957). The measurement of productive efficiency. Journal of the Royal Statistical Society: Series A (General), 120(3), 253–281. https://doi.org/10.2307/2343100
- Fukuyama, H., & Matousek, R. (2017). Modelling bank performance: A network DEA approach. European Journal of Operational Research, 259(2), 721–732. https://doi.org/10.1016/j.ejor.2016.10.044
- Giannetti, C. (2012). Relationship lending and firm innovativeness. Journal of Empirical Finance, 19(5), 762–781. https://doi.org/10.1016/j.jempfin.2012.08.005
- Guariglia, A., & Liu, P. (2014). To what extent do financing constraints affect Chinese firms' innovation activities? International Review of Financial Analysis, 36, 223–240. https://doi.org/10.1016/j.irfa.2014.01.005
- Hendrikse, R., Van Meeteren, M., & Bassens, D. (2020). Strategic coupling between finance, technology and the state: Cultivating a Fintech ecosystem for incumbent finance. Environment and Planning A: Economy and Space, 52(8), 1516–1538. https://doi.org/10.1177/0308518X19887967
- Hicks, J. (1969). A theory of economic history. Oxford University Press.
- Hou, S. Y., & Song, L. R. (2020). Financial technology, technology finance and regional R&D innovation. The Theory and Practice of Finance and Economics, 41(5), 11–19.
- Hsu, P. H., Tian, X., & Xu, Y. (2014). Financial development and innovation: cross-country evidence. Journal of Financial Economics, 112(1), 116–135. https://doi.org/10.1016/j.jfineco.2013.12.002
- Hussain, J., Li, J., & Scott, J. (2017). The equity gap amongst technology-based small firms: Practices and challenges for government-backed venture capital in China. China Business Model, 24(2), 99–117.
- Iftikhar, Y., He, W., & Wang, Z. (2016). Energy and CO2 emissions efficiency of major economies: a non-parametric analysis. Journal of Cleaner Production, 139, 779–787. https://doi.org/10.1016/j.jclepro.2016.08.072
- Kao, C. (2014). Network data envelopment analysis: a review. European Journal of Operational Research, 239(1), 1–16. https://doi.org/10.1016/j.ejor.2014.02.039
- Lampe, H. W., & Hilgers, D. (2015). Trajectories of efficiency measurement: A bibliometric analysis of DEA and SFA. European Journal of Operational Research, 240(1), 1–21. https://doi.org/10.1016/j.ejor.2014.04.041
- Lee, I., & Shin, Y. J. (2018). Fintech: Ecosystem, business models, investment decisions, and challenges. Business Horizons, 61(1), 35–46. https://doi.org/10.1016/j.bushor.2017.09.003
- Liu, J. S., Lu, L. Y., Lu, W. M., & Lin, B. J. (2013). A survey of DEA applications. Omega, 41(5), 893–902. https://doi.org/10.1016/j.omega.2012.11.004
- Liu, J. S., Lu, L. Y., Lu, W. M., & Lin, B. J. (2013). Data envelopment analysis 1978-2010: A citation-based literature survey. Omega, 41(1), 3–15. https://doi.org/10.1016/j.omega.2010.12.006
- Losa, E. T., Arjomandi, A., Hervé Dakpo, K., & Bloomfield, J. (2020). Efficiency comparison of airline groups in Annex 1 and non-Annex 1 countries: A dynamic network DEA approach. Transport Policy, 99, 163–174. https://doi.org/10.1016/j.tranpol.2020.08.013
- Luo, Y., Bi, G., & Liang, L. (2012). Input/output indicator selection for DEA efficiency evaluation: An empirical study of Chinese commercial banks. Expert Systems with Applications, 39(1), 1118–1123. https://doi.org/10.1016/j.eswa.2011.07.111
- Mackenzie, A. (2015). The fintech revolution. London Business School Review, 26(3), 50–53. https://doi.org/10.1111/2057-1615.12059
- Matthews, K. (2013). Risk management and managerial efficiency in Chinese banks: A network DEA framework. Omega, 41(2), 207–215. https://doi.org/10.1016/j.omega.2012.06.003
- Minetti, R., & Herrera, A. M. (2015). Informed finance and technological change: Evidence from credit relationships. Journal of Financial Economics, 83(1), 223–269.
- Palmie, M., Wincent, J., Parida, V., & Caglar, U. (2020). The evolution of the financial technology ecosystem: An introduction and agenda for future research on disruptive innovations in ecosystems. Technological Forecasting and Social Change, 151, 119779. https://doi.org/10.1016/j.techfore.2019.119779
- Popov, A., & Roosenboom, P. (2012). Venture capital and patented innovation: Evidence from. Economic Policy, 27(71), 447–482. https://doi.org/10.1111/j.1468-0327.2012.00290.x
- Qian, S., & Zhang, Y. (2017). Research on the impact of science-finance on R&D investment of firms. Studies in Science of Science, 35(9), 1320–1325.
- Sasidharan, S., Lukose, P. J., & Komera, S. (2015). Financing constraints and investments in R&D: evidence from Indian manufacturing firms. The Quarterly Review of Economics and Finance, 55, 28–39. https://doi.org/10.1016/j.qref.2014.07.002
- Schumpeter, J. A. (1934). The theory of economic development: An inquiry into profits, capital, credit, interest, and the business cycle. Harvard University Press.
- Seiford, L. M. (1996). Data envelopment analysis: The evolution of the state of the art (1978–1995. ).Journal of Productivity Analysis, 7(2-3), 99–137. https://doi.org/10.1007/BF00157037
- Söderlund, B., & Tingvall, P. G. (2017). Capital freedom, financial development and provincial economic growth in China. The World Economy, 40(4), 764–787. https://doi.org/10.1111/twec.12391
- Sokol, M. (2017). Financialisation, financial chains and uneven geographical development: towards a research agenda. Research in International Business and Finance, 39, 678–685. https://doi.org/10.1016/j.ribaf.2015.11.007
- Sun, Z., & Wu, Y. (2017). Financial investment, bank credit and scientific and technological innovation efficiency - An empirical analysis based on spatial GWR model. East China Economic Management, 31(4), 74–81.
- Tolliver, C., Fujii, H., Keeley, A. R., & Managi, S. (2021). Green innovation and finance in Asia. Asian Economic Policy Review, 16(1), 67–87. https://doi.org/10.1111/aepr.12320
- Wang, K., Huang, W., Wu, J., & Liu, Y. N. (2014). Efficiency measures of the Chinese commercial banking system using an additive two-stage DEA. Omega, 44, 5–20. https://doi.org/10.1016/j.omega.2013.09.005
- Yang, W., Sui, X. P., & Qi, Z. (2021). Can fintech improve the efficiency of commercial banks?-An analysis based on big data. Research in International Business and Finance, 55, 101338. https://doi.org/10.1016/j.ribaf.2020.101338.
- Zhang, Y., & Zhao, L. (2015). Government support and financial development, social capital and the financing efficiency of S&T innovation enterprises. Science Research Management, 36(11), 55–63.
- Zhao, G., & Zhang, Z. (2010). Uncovering the Relationship between FDI, Human Capital and Technological Progress in Chinese High-technology Industries. China & World Economy, 18(1), 82–98. https://doi.org/10.1111/j.1749-124X.2010.01182.x
- Zhu, X. Y., Asimakopoulos, S., & Kim, J. (2020). Financial development and innovation-led growth: Is too much finance better? Journal of International Money and Finance, 100, 102083. 2020online. https://doi.org/10.1016/j.jimonfin.2019.102083