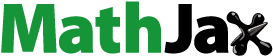
Abstract
Under the control of multi-dimensional factors such as industry and enterprise characteristics, this paper examines the impact of government R&D subsidies on enterprise R&D activities, both theoretically and empirically. Theoretically, on the basis of Symeonidis model, this paper establishes a three-stage dynamic game model by introducing the government R&D subsidy, in order to expand the existing theory. Taking the data of the listed enterprises in China as the research sample, the Spatial Quantile Autoregressive Regression method, which has the ability to examine both spatial effect and quantile effect, is used to test the theoretical results. It is found that R&D subsidies play a significant positive role in stimulating the R&D activities of enterprises, and the incentive effect of subsidies is more obvious with the increase of R&D investment and R&D efficiency. Furthermore, the spillover effect can improve R&D efficiency, and this effect will be gradually strengthened with the increase of quantile.
1. Introduction
R&D plays a significant role in economic growth (Blanco et al., Citation2020). According to the latest data released by OECD, the total amount of R&D investment in China ranks the second in the world, and the growth rate of R&D investment has far exceeded that of developed countries such as the United States, Germany and Japan. The IMD World Competitiveness Yearbooks published by Lausanne Institute of International Management, reveal that the competitiveness gap has narrowed significantly between China and the United States. However, there is still a huge gap in innovation environment, the leading role of R&D in China is still relatively insufficient (Zhang et al., Citation2020). To make breakthrough achievements, China needs to rely on the substantial improvement in R&D of enterprises.
Cultivating and upgrading the independent innovation capabilities of the enterprises is related to the micro-foundation of one country's sustainable economic growth (Hsiao, Citation2014). From the perspective of internal incentive mechanism of micro-enterprise R&D investment, the reasons why enterprises show insufficient R&D motivation are as follows: first, the fundamental research has public externalitiesFootnote1; second, the intellectual property protection system is relatively imperfect; third, the innovation of enterprise's core technology has the characteristics of high investment and high uncertainty. Therefore, the governments in various countriesFootnote2 have to correct the ‘market failure’ and stimulate enterprises' enthusiasm for innovation by means of R&D subsidiesFootnote3 (Cappelen et al., Citation2012; Broekel, Citation2015).
Although government R&D subsidies could make up for market failures, they often distort market incentive mechanism and reduce market allocation efficiency (Chen & Yang, Citation2016). Under some situations, R&D subsidies may even decrease R&D investment due to the crowding-out effect (Acemoglu et al., Citation2018). In view of the complexity and uncertainty of the impact of government R&D subsidies on R&D activities of enterprises, it has always been a hot and cutting-edge issue in the field of economics to explore the actual effect of government subsidies on micro-enterprises' R&D investment and R&D efficiency. It is necessary to start from the perspectives of ‘government-enterprise’, and try to give a theoretical underpinning to the empirical analysis. Therefore, this paper analyzes in-depth the actual impact of government subsidies on R&D investment and R&D efficiency of enterprises, both theoretically and empirically.
The rest of the paper proceeds as follows. Section 2 describes the literature review. Section 3 discusses the theoretical model construction and shows the main theoretical results. Section 4 describes the research data and the variable selection. Section 5 conducts empirical analysis and discusses the empirical results. Finally, conclusions are drawn in section 6.
2. Literature review
The related literature can be classified into ‘government R&D subsidy and enterprise R&D investment’ and ‘government R&D subsidy and enterprise R&D efficiency’.
2.1. Government R&D subsidy and enterprise R&D investment
To understand the actual impact of government R&D subsidy on enterprise R&D investment, it is necessary to clarify the influence mechanism of R&D subsidy. The influence mechanism is divided into ‘incentive effect’ (Czarnitzki & Hussinger, Citation2018; Zawalińska et al., Citation2018) and ‘inhibition effect’ (Wang et al., Citation2014).
There are three aspects in terms of ‘incentive effect’ mechanism. First, government R&D subsidies bear certain risks for enterprises to carry out R&D activities, and the decrease of innovation risks encourages enterprises to increase R&D investment (Hall, Citation2002). Second, government R&D subsidies are often used in technological transformation projects. These projects not only prolong the service life of enterprises' R&D equipment, but also greatly improve its operation efficiency and indirectly reduce the R&D cost of other R&D equipment, thus accumulating funds for enterprises' R&D activities (Montmartin & Herrera, Citation2015). Third, the ‘halo’ effect of government R&D subsidies can release a positive signal, which is conducive to attracting the participation of external universities and research institutions. This can not only improve enterprises' innovation capabilities, but also encourage enterprises to increase their R&D investment (Lu et al., Citation2018; Takalo & Tanayama, Citation2010).
There are two aspects in terms of ‘inhibition effect’ mechanism. First, the government R&D subsidy will crowd out the R&D activities of enterprises when there is information asymmetry between government and enterprises (Boeing, Citation2016). Second, large-scale enterprises and state-owned enterprises with political background have easier access to government subsidies due to their economies of scale and political connections, which makes them face fewer financial constraints and gives negative feedback on subsidies (Chen & Yang, Citation2016).
2.2. Government R&D subsidy and enterprise R&D efficiency
Government R&D subsidy should not only encourage enterprises to increase R&D investment, but also improve R&D efficiency of enterprises (Xiong, Citation2011). However, up to now, there is still no consensus on the impact of government R&D subsidies on R&D efficiency of enterprises. Dong and Han (Citation2016) find that there is the nonlinear relationship between R&D subsidy and R&D efficiency by using the data of listed enterprises in China. Bronzini and Piselli (Citation2016) consider that government R&D subsidies can significantly promote the R&D efficiency of enterprises by using the panel data of 612 manufacturing industries in Italy. Based on the data of private enterprises in Beijing, Zheng (Citation2016) confirms that government R&D subsidies can effectively increase R&D investment, but they have no significant impact on R&D efficiency of enterprises. Guo et al. (Citation2018) find that the government tend to support enterprises with high productivity, and the efficiency of enterprises will be significantly improved after receiving the R&D subsidy. Yan and Huang (Citation2020) find that government R&D subsidies have no significant impact on R&D efficiency of enterprises, they could even aggravate overcapacity of enterprises. The inconsistency of existing research indicates the possible complex relationship between government R&D subsidy and enterprise R&D efficiency, which is often affected by multi-dimensional factors such as industry, enterprise, region and other key factors from different perspectivesFootnote4.
2.3. Contributions
This paper has the following contributions. Theoretically, by constructing a three-stage dynamic game, this paper addresses the dynamic interaction between government R&D subsidies and enterprise R&D activities in the co-existence of horizontal and vertical differentiations of product. This makes up for the deficiency that the existing literature ignores the multi-dimensional differentiation of products. Empirically, this paper uses the Spatial Quantile Autoregressive Regression (henceforth, SQAR) method, to study the impact of government R&D subsidies on enterprise R&D activities. On the one hand, through the advantages of SQAR in analyzing ‘spatial effect’, the geographical location of enterprises is organically linked with the R&D spillover of theoretical model, which improves the connection between theoretical and empirical parts of this paper. On the other hand, through the advantages of SQAR in analyzing ‘quantile effect’, the marginal effect of government R&D subsidy on enterprise R&D at specific quantile can be studied in depth, so as to dig out richer information ‘loosely’.
3. Theoretical analysis
On the basis of Symeonidis model (Symeonidis, Citation2003), the theoretical part introduces the government R&D subsidy, and establishes a three-stage dynamic game model to study the impact of government R&D subsidy on enterprise R&D strategy.
3.1. Enterprises and consumers
Suppose an industry is composed of enterprise and enterprise
and the outputs they produce are characterized by both ‘vertical differentiation’ and ‘horizontal differentiation’. The utility function of representative consumers takes the form of EquationEquation (1)
(1)
(1) , following Sutton (Citation1997). Note that EquationEquation (1)
(1)
(1) is a quality-augmented version of the standard quadratic utility function used by Qiu (Citation1997).
(1)
(1)
where
and
are the consumption quantities of product
and product
and
represent the quality of product
and product
respectively, product quality can not only characterize the vertical differentiation between products, but also measure the R&D efficiency of the enterprise;
represents the possible ‘substitute or complementary’ relationship between productsFootnote5, which can be used to measure the horizontal differentiation of products (the greater the value of
the stronger the product substitutability, and the weaker the level of horizontal differentiation);
represents the consumption of goods other than product
and product
namely,
and
represents the price of the product. Based on the first-order condition of maximization of consumer utility, the demand function can be obtained
(2)
(2)
3.2. Enterprise R&D activities and government R&D subsidy
Assume enterprises in the industry are engaged in R&D, and there is R&D spillovers between enterprises. Enterprises invest in R&D to improve their product quality and market competitiveness. Product quality (R&D efficiency) is defined as where
represents the level of R&D spillover,
represents the level of R&D investment. The function linking product quality
to R&D investment
and
is a modified version of the one used by Motta (Citation1992).
Suppose the unit production cost of an enterprise is a fixed and uniform constant and the government decides the R&D subsidy rate
according to the R&D investment level of enterprises. The profit function of enterprise
can be described as:
(3)
(3)
3.3 ‘Government-enterprise’ dynamic game
The government and enterprises engage in a three-stage dynamic game. The first stage is ‘government R&D subsidy’, in which the government decides the optimal R&D subsidy rate with the goal of maximizing social welfare. The second stage is ‘enterprise R&D’, in which enterprises decide their respective R&D investment level to maximize their profits when the government R&D subsidy rate is determined. The third stage is the ‘production competition’, in which enterprises choose their own output levels in the product market to maximize their profits.
The above game can be solved by backward induction. First of all, beginning with the ‘production competition’ stage, enterprises decide their output according to the principle of profit maximization. Taking enterprise as an example, given the government R&D subsidy and enterprise R&D behavior, the optimal output level is chosen based on Cournot competition. According to
the optimal output of enterprise
is
(4)
(4)
It can be known that and when the ratio of R&D efficiency between enterprise
and its competitor is higher than a critical threshold (
), the enterprise can earn more profit by increasing its output; when R&D efficiency ratio is lower than this threshold, enterprises should reduce production as much as possible.
Furthermore, in the ‘enterprise R&D’ stage, enterprises choose the level of R&D investment to maximize their own profit. Since the model is symmetric, the optimal R&D investment of the enterprise can be obtained by solving It is easy to find
(5)
(5)
Finally, in the ‘government R&D subsidy’ stage, the government decides the optimal R&D subsidy rate in order to realize the maximization of social welfare. Assume that social welfare is composed of producer surplus consumer surplus
and government expenditure
namely
Producer surplus
is the sum of profits of enterprises in the industry (
); consumer surplus
is
government expenditure is government subsidies for R&D (
). Through the first-order condition of social welfare maximization, the government's optimal R&D subsidy rate can be found
(6)
(6)
It is shown that within the allowable range of spillover effect and horizontal differentiation
the government R&D subsidy rate is always greater than zero. This means that the government R&D subsidy can not only effectively stimulate enterprises to engage in R&D activities, but also achieve the goal of improving the social welfare.
Result 1: R&D investment of enterprises can be affected by spillover effect. When the spillover is high and the degree of horizontal differentiation is low, the spillover effect will inhibit the R&D investment of enterprises; when the product is highly differentiated, or when the spillover level is low, or there is high spillover and low horizontal differentiation, the spillover effect will encourage enterprises to increase R&D investment.
Proof:
According to EquationEquation (5)(5)
(5) , we have
When
spillover effect is beneficial to increase R&D investment of enterprises. When
the sign of
cannot be determined. The spillover effect on enterprise R&D investment has two possibilities (incentive or inhibition). To be precise, when
and
we have
when
or
and
we have
■
Result 2: Government R&D subsidies always play an incentive role in R&D investment and R&D efficiency (or product quality).
Proof:
Since it is known that
is always true. Furthermore, since
we have the equilibrium expression
and it is easy to find
is always true. ■
4. Data and variable selection
The listed enterprises in China from 2010 to 2014 are selected as the research sampleFootnote6. Related data mainly stem from Wind database, CSMAR database and ‘JUCHAO’ information website (www.cninfo.com.cn). To ensure the integrity and accuracy of the data, the following steps are adopted: (1) the samples with missing values in enterprise R&D expenses and patent are eliminated; (2) data with negative values are eliminated; (3) the extreme samples of each variable above 99.5% quantile and below 0.5% quantile are deleted. Finally, 7207 valid observations are obtained from 273 cities in 31 provinces of China.
The explained variables are the enterprise R&D investment () and R&D efficiency (
) involved in the theoretical part. Consistent with existing studies, this paper uses the logarithm of R&D expenditure to measure the R&D investment level of enterprisesFootnote7, the data are derived from the R&D expenditure disclosed in the enterprise's annual report. R&D efficiency reflects the ratio between R&D output and R&D input (Zhang et al., Citation2017). This paper uses the ratio of patent application number to R&D investment to measure the R&D efficiency of listed enterprises.
The core explanatory variable is the government R&D subsidy (). Referring to the data acquisition method of Wang et al. (Citation2014), the government subsidies are distinguished according to the details of government subsidies disclosed in the annual reports of listed enterprises. The government subsidies directly related to the R&D activities of listed enterprises, such as the detailed items containing ‘science and technology’, ‘scientific research’, ‘research and development’, ‘innovation’ and other keywords, will be regarded as R&D subsidies, and add up various types of R&D subsidies for each enterprise in the same year. Considering the possible endogenous problems and the lagging effect of R&D subsidy on enterprises' R&D activities, this paper adopts the lag of one period of government R&D subsidy (logarithm) as the measurement index of
Control variables are divided into enterprise control variables and other control variables. Enterprise control variables mainly include the proportion of researchers (), enterprise age (
), enterprise size (
), degree of nationalization (
), foreign shareholding ratio (
), liquid assets (
), long and short term loans (
and
) and equity financing (
). Other control variables include industry (
), year (
) and location (
). The specific definitions of each variable are shown in , and presents the descriptive statistics and the Jarque-Bera statistics of variables in this study. It is worthwhile to note that the null hypothesis for the normality test is rejected, and the quantile approach is more robust for estimation.
Table 1. Variable description.
Table 2. Descriptive statistics.
5. Empirical discussion
5.1. In the absence of spatial effect
In this subsection, the quantile regression (QR) model is used to explore the heterogeneous reaction of government R&D subsidies to enterprises with different R&D activities. The advantages of quantile regression are as follows: first, it can capture the tail characteristics of the distribution of R&D activities of listed enterprises, especially in the situation where the R&D investment and efficiency of enterprises may be skewed; second, compared with ordinary least square regression (OLS), it can describe the distribution characteristics of enterprises' R&D activities more comprehensively, so as to obtain a more comprehensive result that government R&D subsidies affect enterprise R&D activities. The specific form of quantile regression is shown below
(7)
(7)
where
is a series of enterprise control variables, and
is the corresponding coefficient of enterprise control variables.
lists the estimated results of OLS regression and quantile regression (QR) at and models 1 to 4 analyze the impact of government R&D subsidy on R&D investment of listed enterprises. The estimated coefficient of government R&D subsidy (
) in model 1 is 1.6520, which is significant at the level of 1% statistically, indicating that subsidy has a significant positive impact on stimulating listed enterprises to increase R&D investment. In models 2 to 4, three quantiles of 10%, 50% and 90% are used for quantile regression, and the estimated coefficients of R&D subsidy are 0.1883, 1.4166 and 4.1215, respectively. It can be inferred that the incentive effect of government R&D subsidy on R&D investment of listed enterprises becomes stronger with the increase of quantile. Besides, the influence of the proportion of researchers (
) and the scale of liquid assets (
) on R&D investment is similar to that of subsidies. A higher proportion of scientific research personnel is beneficial for enterprises to increase R&D investment. With the improvement of R&D investment level, scientific research teams will play a more and more positive role in promoting R&D investment of enterprises (David et al., Citation2000). And with the increase of liquidity assets, the endogenous resources of enterprises become more abundant, which makes enterprises easier to promote R&D investment (Lerner, Citation1999).
Table 3. Government subsidy and enterprise R&D investment.
In addition, shows that although the enterprise scale () has a positive impact on R&D investment, this impact is not obvious for the enterprises with low R&D investment; the older an enterprise is, the more conscious the enterprise invests in R&D activities; the degree of nationalization (
) has a negative impact on listed enterprises with medium and high level of R&D investment. Besides, different capital structures often determine the R&D investment and R&D efficiency of listed enterprises. According to , equity financing (
) cannot generate a significant impact on the R&D investment of enterprises, in other words, direct financing of listed enterprises will not affect their R&D investment. However, the indirect financing (including long-term loans and short-term loans) plays an inhibitory role at
and
which makes debt significantly reduce the R&D investment of listed enterprises (Chiao, Citation2002).
shows the estimated results of OLS and QR regressions with listed enterprises' R&D efficiency () as the explained variable. The estimated results of model 5 show that government R&D subsidy (
), age (
), nationalization (
) and liquid assets (
) can significantly promote the R&D efficiency of listed enterprises. Furthermore, by using QR estimation (model 6 to 8), it is shown that the influence of government subsidy and liquidity asset on R&D efficiency increases greatly with the increase of quantile, while the effect of enterprise age and nationalization degree on R&D efficiency presents an ‘inverted U-shape’.
Table 4. Government subsidy and enterprise R&D efficiency.
To describe more specifically the details of the quantile regression at each quantile and show the trend and confidence interval of the coefficient of the explanatory variable at 99 quantiles (
).
Figure 1. Variation of estimated coefficient (R&D investment as the explained variable).
Source: The authors.
Note: The curve composed of black dots in the figure is the quantile regression estimation result of each explanatory variable, and the shaded part is the confidence interval of quantile regression estimation value (the confidence level is 95%); The red straight line represents the OLS regression estimation of explanatory variables, and the area between two thin red dotted lines represents the confidence interval of the OLS regression estimation (the confidence level is 95%); The horizontal axis represents the different quantiles of R&D investment level, and the vertical axis represents the regression coefficient of each variable.
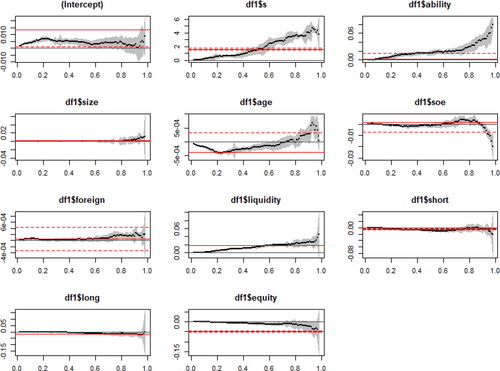
Figure 2. Variation of estimated coefficient (R&D efficiency as the explained variable).
Source: The authors.
Note: The curve composed of black dots in the figure is the quantile regression estimation result of each explanatory variable, and the shaded part is the confidence interval of quantile regression estimation value (the confidence level is 95%); The red straight line represents the OLS regression estimation of explanatory variables, and the area between two thin red dotted lines represents the confidence interval of the OLS regression estimation (the confidence level is 95%); The horizontal axis represents the different quantiles of R&D investment level, and the vertical axis represents the regression coefficient of each variable.
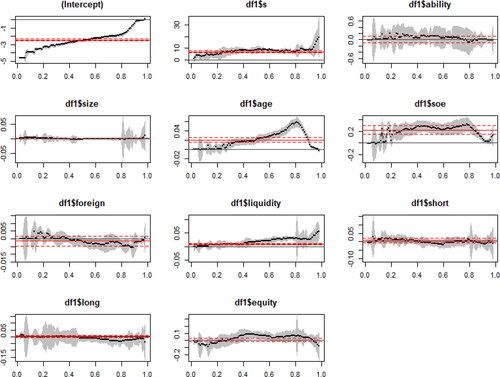
According to and 2, the estimated coefficients of government R&D subsidy are always greater than 0 no matter whether the explained variable is the level of enterprise R&D investment () or enterprise R&D efficiency (
). Hence, as long as there is government R&D subsidy, it will definitely generate positive incentives for enterprises' R&D investment and efficiency. This not only confirms the positive role of government R&D subsidies in promoting enterprises' R&D activities, but also verifies the theoretical result (Result 2) from an empirical perspective.
5.2. In the presence of spatial effect
Spatial dependence may be produced by a diffusion process, when spatial spillovers cause the R&D activities of listed enterprise to depend on the R&D activities of neighboring listed enterprises. This type of spatial dependence, known as the spatial lag model, has much more serious implications. One of the motivations for using the spatial quantile autoregressive regression (SQAR) model is the possibility for varying (across the sample) the degree of spatial dependence (Kostov, Citation2009).
SQAR model can be regarded as a combination of spatial autoregressive regression (SAR) model and quantile regression (QR) model. The SAR model expands the standard linear regression into a spatial model by adding a spatial lag variable to explain the spatial effects which the linear regression model may ignore. The specific form of SAR is as follows
(8)
(8)
where
is the explained variable,
is the
order matrix of the explanatory variable,
is the coefficient vector of the explanatory variable,
is the error vector, and
is the
order matrix of the spatial relationship between each value of the explanatory variable
and its adjacent values.
Similarly, the SQAR model is based on the SAR model and endowed with the ability to investigate the quantile effect. By referring to the ideas of Trzpiot and Orwat-Acedanska (Citation2016), the SAR model (EquationEq. 8(8)
(8) ) and the QR model (EquationEq. 7
(7)
(7) ) are combined to construct the SQAR model shown in EquationEquation (9)
(9)
(9)
(9)
(9)
where
and
is the spatial autoregressive parameter at the quantile
and
is the coefficient vector of each explanatory variable, vector
is a random variable which is independent at different quantiles.
In the estimation process of the model, due to the existence of endogenous problems (caused by ) and the use of instrumental variables (
etc.) for each quantile
the model estimation is very complex. To this end, this paper uses a simple extension of 2SLS to determine the form of instrumental variables required in different quantile regression. The advantage of this approach is to greatly simplify the model estimation, which is not necessary to solve the inverse
of
order matrix. The detailed estimation process is as follows:
In the first stage, EquationEquation (10)(10)
(10) is estimated
(10)
(10)
In the second stage, for each value of
and
are used for estimating
according to EquationEquation (11)
(11)
(11) , and the predicted value of quantile regression is
(11)
(11)
In the third stage, the second quantile regression estimation is performed for the same value of according to EquationEquation (12)
(12)
(12) . At this stage,
is taken as the explained variables,
and
are considered as the explanatory variables
(12)
(12)
Before using SQAR for analysis, it should be clear whether the explained variables have spatial correlation. In general, Moran’s index (EquationEq.13(13)
(13) ) can be used to determine whether variables have spatial correlation.
(13)
(13)
where
is the observed value of the R&D investment or the R&D efficiency of listed enterprise
and
is the observed value of listed enterprise
is the observed number of listed enterprises,
represents the average R&D investment or R&D efficiency of listed enterprises (
),
is the matrix element in the spatial weight matrix
According to the office address of listed enterprises in the Financial Terminal Database ‘Oriental Wealth Choice’ (http://choice.eastmoney.com), the geographical coordinates of listed enterprises are collected, and the spatial weight matrix
is constructed based on the ‘geographical distance’. The results of Moran’s I test show that the Moran index of R&D investment and that of R&D efficiency of listed enterprises are always significantly positive, indicating that the correlation between R&D investment (or R&D efficiency) among enterprises becomes more and more obvious with the agglomeration of spatial distribution positions of listed enterprises.
Furthermore, SQAR model is used to conduct regression for and the estimated results are shown in .
Table 5. Estimation results of SQAR model.
shows that when enterprise R&D investment is taken as the explained variable (models 9 to 11), the estimated coefficients of spatial lag variable at
are significantly different, and the spillover effect between enterprises R&D investment gradually decreases with the increase of quantile. Specifically, when
and
the estimated coefficients
of spatial spillover effect are significantly positive (0.0220 and 0.0081, respectively), indicating that spillover effects are beneficial to encourage enterprises to increase R&D investment. However, when
the coefficient
is significantly negative (-0.0629), which indicates that the spillover effect plays a role in inhibiting R&D investment of listed enterprises. To some extent, the above estimation results verify the main result of the theoretical part (Result 1), the spillover effect on the R&D investment of enterprises manifest two possibilities: incentive or inhibition. When enterprise R&D efficiency is considered as the explained variable, at different quantile (models 12 to 14), there is always ‘mutual incentive’ between enterprises close to each other, and the ‘mutual incentive’ will gradually become stronger with the increase of quantiles. Besides, also shows that the effects of government subsidy on R&D investment and R&D efficiency of listed enterprises at
are always positive, and the estimated coefficient of
increases with the increase of quantile.
By comparing the case in the absence of spatial effect (subsection 5.1) to the case in the presence of spatial effect (subsection 5.2), it can be inferred that whether using QR or SQAR, government R&D subsidies always play an incentive role in R&D investment and R&D efficiency of listed enterprises, which further verify the theoretical result of this paper (Result 2).
6. Conclusion
Existing research focuses on theoretical analysis or empirical discussion. This paper adopts the method which combines theoretical analysis with empirical discussion, to realize the consistency between theory and practice. On the one hand, based on the ‘government-enterprise’ three-stage dynamic game model, this paper makes a theoretical discussion on the interaction between government R&D subsidies and enterprise R&D activities in order to realize ‘theory guiding practice’. On the other hand, using data of listed enterprises in China, this paper tests the theoretical results through empirical analysis, to achieve the purpose of ‘practice correcting theory’.
By the three-stage dynamic game, the theoretical part studies the effect of government R&D subsidy on enterprise R&D strategy and the relationship between spillover effect and enterprise R&D activities. It is found that when the spillover level is high and the degree of product horizontal differentiation is low, the spillover effect will inhibit the R&D investment of enterprises; when the horizontal differentiation is high, or the spillover level is low, or there is high spillover and low horizontal difference, the spillover effect will encourage enterprises to increase R&D investment. Government R&D subsidy can not only encourage enterprises to increase R&D investment, but also help enterprises to improve R&D efficiency and product quality. The empirical part makes an in-depth analysis of the influence of government R&D subsidies on R&D investment and R&D efficiency of enterprises by using QR and SQAR models. By comparing the case in the presence of spatial effect to that in the absence of spatial effect, it is shown that (1) government R&D subsidies always have a significant positive incentive effect on the R&D investment and R&D efficiency of listed enterprises in China, and the incentive effect of R&D subsidy becomes more and more stronger with the increase of the R&D investment and R&D efficiency of listed enterprises. (2) from the viewpoint of enterprise R&D investment, spillover effect has two possibilities: incentive or inhibition; form the viewpoint of enterprise R&D efficiency, there is always ‘mutual incentive’ between listed enterprises that are close to each other, and this ‘mutual incentive’ will gradually become stronger with the increase of the quantile.
This study has a number of limitations, proving further developments for future research. First, on the basis of the three-stage dynamic game model proposed in this paper, it is possible to further explore the optimal value of subsidy rate or subsidy scale, and the optimal timing of policy intervention, namely, when government subsidies enter and when to exit during the enterprise R&D project. Second, considering the differences in technological requirements of different industries, it can be reasonably speculated that the optimal scale of subsidies is not the same among different industries. For example, high-tech enterprises have higher demand for innovative resources compared with enterprises in other industries. If the scale of subsidies is determined according to the average level, it may not achieve good results. Therefore, how to calculate the optimal subsidy scale applicable to different industries according to the technical requirements of different industries, the development degree of local markets and other relevant characteristics can become a future research direction.
Disclosure statement
No potential conflict of interest was reported by the authors.
Additional information
Funding
Notes
1 For example, technological spillovers can greatly reduce the enthusiasm of enterprises engaged in R&D activities.
2 According to statistical information from Wind database, 99% of China's A-share listed enterprises have received government R&D subsidies. Moreover, the development of software industry in the United States, pharmaceutical industry in India, LSI (Large-Scale Integration) industry in Japan, benefits from a large amount of subsidy funds provided by the government.
3 Government R&D subsidy is an uncompensated asset provided by the government for enterprise R&D activities. As a direct means to stimulate innovation, government R&D subsidy focuses on ‘ex-ante incentive’. Due to the uncertainty and externality of R&D, enterprises' R&D investment may be lower than the social optimal level, which provides theoretical support for the government to carry out R&D subsidy.
4 For example, the heterogeneity of enterprises in scale and liquid assets, as well as the differences in industries and regions, and even the quality of their products, make enterprises have great differences in ‘receiving subsidy’ and ‘using subsidy’.
5 We assume in order to avoid that the demand becomes negative.
6 In view of the availability of data, it is not possible to incorporate the latest data (from 2015 to 2019) into the empirical analysis framework, which is the shortcoming of this study.
7 There are mainly two methods to measure enterprise R&D investment: the first one is to directly use the R&D investment (usually logarithm); the second one is to use the ratio of the R&D investment to the main business income. This paper adopts the first method to measure enterprise R&D investment for three reasons. First, the data of main business income in the sample of listed enterprises is missing to some extent, so it is difficult to use the ratio of the two to measure the intensity of R&D investment. Second, the measurement methods of enterprise R&D investment and government R&D subsidy should be consistent. Third, the authors have used the ‘intensity’ of R&D investment as a measurement, and the results are not significantly different.
8 Due to the limited disclosure of foreign shareholding by listed enterprises, the data of foreign shareholders’ shareholding only takes the top ten shareholders.
References
- Acemoglu, D., Akcigit, U., Alp, H., Bloom, N., & Kerr, W. (2018). Innovation, reallocation, and growth. American Economic Review, 108(11), 3450–3491. https://doi.org/10.1257/aer.20130470
- Blanco, F. A., Delgado, F. J., & Presno, M. J. (2020). R&D expenditure in the EU: convergence or divergence? Economic Research-Ekonomska Istraživanja, 33(1), 1685–1710. https://doi.org/10.1080/1331677X.2020.1756371
- Boeing, P. (2016). The allocation and effectiveness of China’s R&D subsidies – evidence from listed firms. Research Policy, 45(9), 1774–1789. https://doi.org/10.1016/j.respol.2016.05.007
- Broekel, T. (2015). Do cooperative research and development (R&D) subsidies stimulate regional innovation efficiency? Evidence from Germany. Regional Studies, 49(7), 1087–1110. https://doi.org/10.1080/00343404.2013.812781
- Bronzini, R., & Piselli, P. (2016). The impact of R&D subsidies on firm innovation. Research Policy, 45(2), 442–457. https://doi.org/10.1016/j.respol.2015.10.008
- Cappelen, A., Raknerud, A., & Rybalka, M. (2012). The effects of R&D tax credits on patenting and innovations. Research Policy, 41(2), 334–345. https://doi.org/10.1016/j.respol.2011.10.001
- Chen, L., & Yang, W. H. (2016). Do government subsidies stimulate enterprises’ innovation? Evidence from China’s listed companies. Studies in Science of Science, 34(3), 433–442.
- Chiao, C. (2002). Relationship between debt, R&D and physical investment, evidence from US firm-level data. Applied Financial Economics, 12(2), 105–121. https://doi.org/10.1080/09603100110102709
- Czarnitzki, D., & Hussinger, K. (2018). Input and output additionality of R&D subsidies. Applied Economics, 50(12), 1324–1341. https://doi.org/10.1080/00036846.2017.1361010
- David, P., Hall, B., & Toole, A. (2000). Is public R&D a complement or substitute for private R&D? A review of the econometric evidence. Research Policy, 29(4-5), 497–529. https://doi.org/10.1016/S0048-7333(99)00087-6
- Dong, M. F., & Han, X. F. (2016). Does R&D input affect the technical efficiency of the strategic emerging industries. Science of Science and Management of S.& T, 1, 95–106.
- Guo, D., Guo, Y., & Jiang, K. (2018). Governance and effects of public R&D subsidies: Evidence from China. Technovation, 74, 18–31.
- Hall, B. H. (2002). The financing of research and development. Oxford Review of Economic Policy, 18(1), 35–51. https://doi.org/10.1093/oxrep/18.1.35
- Hsiao, S. H. (2014). PTE, innovation capital and firm value interactions in the biotech medical industry. Journal of Business Research, 67(12), 2636–2644. https://doi.org/10.1016/j.jbusres.2014.04.001
- Kostov, P. (2009). A spatial quantile regression hedonic model of agricultural land prices. Spatial Economic Analysis, 4(1), 53–72. https://doi.org/10.1080/17421770802625957
- Lerner, J. (1999). The government as venture capitalist: the long-run impact of the SBIR program. The Journal of Business, 72(3), 285–318. https://doi.org/10.1086/209616
- Lu, X., He, X. H., Dai, X. T., & Ding, Y. P. (2018). Financial development, government subsidies and R&D investment of companies: Evidence from the listed companies in strategic emerging industries. Journal of Capital University of Economics and Business, 20(1), 49–58.
- Montmartin, B., & Herrera, M. (2015). Internal and external effects of R&D subsidies and fiscal incentives: Empirical evidence using spatial dynamic panel models. Research Policy, 44(5), 1065–1079. https://doi.org/10.1016/j.respol.2014.11.013
- Motta, M. (1992). Co-operative R&D and vertical product differentiation. International Journal of Industrial Organization, 10(4), 643–661. https://doi.org/10.1016/0167-7187(92)90064-6
- Qiu, L. D. (1997). On the dynamic efficiency of Bertrand and Cournot equilibria. Journal of Economic Theory, 75(1), 213–229. https://doi.org/10.1006/jeth.1997.2270
- Sutton, J. (1997). One smart agent. The Rand Journal of Economics, 28(4), 605–628. https://doi.org/10.2307/2555778
- Symeonidis, G. (2003). Comparing Cournot and Bertrand equilibria in a differentiated duopoly with product R&D. International Journal of Industrial Organization, 21(1), 39–55. https://doi.org/10.1016/S0167-7187(02)00052-8
- Takalo, Y., & Tanayama, T. (2010). Adverse selection and financing of innovation: is there a need for R&D subsidies? The Journal of Technology Transfer, 35(1), 16–41. https://doi.org/10.1007/s10961-009-9112-8
- Trzpiot, G., & Orwat-Acedanska, A. (2016). Spatial quantile regression in analysis of healthy life years in the European Union countries. Comparative Economic Research, 19(5), 179–199.
- Wang, Q. M., Han, Q. X., & Yang, C. (2014). Government subsidies and corporate behavior in strategic emerging industries: A perspective of dynamic game based on government regulation. Journal of Finance and Economics, 40(7), 43–53.
- Xiong, W. Q. (2011). A theoretical and empirical study on the effects of taxation and subsidy policies on the R&D efficiency and scale. Studies in Science of Science, 29(5), 698–706.
- Yan, X. C., & Huang, G. T. (2020). Government subsidies, enterprise economic and innovation performance, and overcapacity: An empirical research based on strategic emerging industries. Nankai Economic Studies, 1, 176–198.
- Zawalińska, K., Tran, N., & Płoszaj, A. (2018). R&D in a post centrally-planned economy: The macroeconomic effects in Poland. Journal of Policy Modeling, 40(1), 37–59. https://doi.org/10.1016/j.jpolmod.2017.09.007
- Zhang, X. W., Wang, L. X., & Chen, F. (2020). R&D subsidies, executive background and innovation of Chinese listed companies. Economic Research-Ekonomska Istraživanja, https://doi.org/10.1080/1331677X.2020.1792324
- Zhang, Y., Chen, K. H., & Qiao, W. G. (2017). Chinese large-and-medium enterprises’ R&D efficiency measurement and fiscal incentive polices’ effects. The Journal of Quantitative & Technical Economics, 5, 38–54.
- Zheng, Y. B. (2016). The impact of government funding on R&D expenditure and efficiency of private firms in Beijing. Studies in Science of Science, 34(7), 1036–1043.