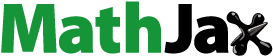
Abstract
This investigation is the first to analyze how civil unrest impacts herding behavior in an emerging economy. Using series of prices and daily traded volumes of the companies that make up the IGPA of the Santiago Stock Exchange between 2010 and 2020, it was found that civil unrest causes reverse herding behavior in the Chilean stock market. Thus, herding behavior and inverse herding behavior are more complex behaviors than the financial literature has reported to date, especially in a period of civil unrest. Different robustness tests support the findings.
JEL Codes:
1. Introduction
Civil unrest consists of acts of protest and/or attacks by certain groups of civilians and/or the mass population against the government. These riots can take the form of peaceful, disruptive, or violent demonstrations, strikes and acts of violence (Lancaster, Citation2018). The consequences of civil unrest are diverse, and can be disastrous, and cover various areas such as human rights, economy, education, health, and infrastructure, among many others.
In financial markets, one way for investors to overcome periods of crisis is adopting herding behavior (Andrikopoulos et al., Citation2017; Omay & Iren, Citation2019). This behavior allows them to imitate the actions of others using the same sources of information and interpreting signals sent to the market in an identical way, and, consequently, making similar financial decisions (Hirshleifer et al., Citation1994). In this way, acting in a “herd,” investors would safeguard the value of their assets in periods of crisis. Furthermore, when the social interactions among traders are strong, an extremely small bubble may cause a sufficiently large number of traders to engage in herd behavior (Chang, Citation2014).
But can an economic-financial crisis be considered similar to civil unrest? Definitely not. During civil unrest human rights violations and even deaths occur. The consequences of these acts shape new behaviors of people that even span generations. These situations do not occur in periods of economic-financial crisis. Furthermore, it is known that in serious economic-financial crises the government has operated as a lender of last resort to rescue the market. However, during civil unrest it is the government that is questioned. Considering that civil unrest is a different situation from that of an economic-financial crisis, it is possible to expect two situations in the stock markets. First, that the people who participate in the stock markets interpret this situation as a crisis and favor herding behavior; or second, that some of them go ahead and act individually, quickly causing a reverse herding behavior. Until today it has not been reported in the financial literature how civil unrest impacts herding behavior
The main objective of this article is to investigate how civil unrest impacts herding behavior. For this, series of prices and daily traded volumes of the companies that make up the General Index of Stock Prices of the Santiago Stock Exchange (S&P/CL IGPA) are used from January 1, 2010 to February 04, 2020 and the model proposed by Chang et al. (Citation2000), which is a modification of the model proposed by Christie and Huang (Citation1995). The period of civil unrest is considered from October 19, 2019 onwards since this day President Sebastián Piñera declares a State of Emergency in the capital of Chile, Santiago, due to the increasingly violent riots since October 7. The results show that civil unrest caused reverse herding behavior in the Chilean stock market. Robustness tests support the findings. In turn, the results are not in line with those of Nath and Brooks (Citation2020) as well as with those of Christie and Huang (Citation1995) who point out that herding behavior should appear during extreme and crisis periods. This confirms that civil unrest is different from a traditional economic-financial crisis and its impact on the stock market is also different.
The findings of this study are relevant to the financial sector (investors, regulators, brokers), the political sector (government, congress), analysts and academics. This study is structured as follows. Section 2 briefly reviews the literature and research hypotheses. Section 3 presents the methodology and data used in this paper. Section 4 presents and discusses the empirical findings and highlights limitations of the current study. Section 5 concludes the paper.
2. Literature review and research hypotheses
2.1. Herding behavior in financial markets
Herding behavior is a process where investors imitate the actions of others. It is a tendency for investors to follow the same sources of information, interpreting signals sent to the market in an identical way, and, consequently, making similar financial decisions (Hirshleifer et al., Citation1994).
It can be found an extensive literature on herding behavior in financial markets over developed countries (USA, Nicolis and Sumpter (Citation2011); Central and East European, Pochea et al. (Citation2017); Germany, Mueller and Brettel (Citation2012); and Spain, Blasco et al. (Citation2012); among others). Similarly, there is extensive evidence on various financial markets among which the stock markets stand out (Economou et al., Citation2011), the gold market (Boako et al., Citation2019), hedge fund (Ennis & Sebastian, Citation2003) and bank markets (Waheed & Mathur, Citation1993) among others.
From developed markets the evidence has spread to countries in Asia (Najmudin et al., Citation2017) and various emerging countries such as Malaysia, South Korea (Chung et al., Citation2016), Pakistan (Chaudhry & Sam, Citation2018), the Gulf Arab stock markets Abu Dhabi, Dubai, Kuwait, Qatar and Saudi Arabia (Balcilar et al., Citation2013), the Asian and Latin American markets (Kabir & Shakur, Citation2018), Israel (Andronikidi & Kallinterakis, Citation2010), Russia (Indārs et al., Citation2019) and Greek (Economou et al., Citation2016), among many others. These countries use different techniques and incorporate some specific characteristics of these markets such as agency, information, efficiency, behavioral problems, expansionary monetary policy, increases in foreign portfolio investment and contagion factors in financial markets, among others, to explain herding behavior.
In this context, studies on herding behavior surrounding events such as civil unrest are those that consider times of crisis, such as the subprime crisis and the Asian crisis (Andrikopoulos et al., Citation2017 and Omay & Iren, Citation2019, respectively). Andrikopoulos et al. (Citation2017) consider a sample of Euronext member countries reporting intraday herding behavior before, during and after the period of said financial crisis (2007–09), with its presence being less prominent during the crisis. Omay and Iren (Citation2019) report herding behavior for Malaysia during the Asian crisis for both foreign and domestic investors. In addition to that, there is also research that incorporates social aspects to explain herd behavior. In this vein, this behavior would occur when strong enough social interactions are present in individual investors (Chang, Citation2014).
In summary, the evidence is overwhelming pointing out that herding behavior is present in the financial markets. However, there is little evidence on how social interactions can influence this behavior and as far as we know there are no studies investigating how civil unrest impacts herding behavior.
2.2. Civil unrest in financial markets
An exploratory review of articles on civil unrest in financial markets in the database of Web of Science (WOS),Footnote1 that searches in titles, abstracts, and keywords made on May 30, 2020, returned 4 articles and only 1 of them, which investigates the relationship between armed and civil conflicts and oil prices, has some relationship with civil unrest. In this article, Noguera (Citation2016) reports three civil-political unrests, among 32 geopolitical events in his sample, which correspond to the countries of Nigeria and Venezuela and are focused, like all the selected ones, on observing how these events impact the price of oil.
A broader search in the same WOS database that included the keyword “Economy” yielded 48 articles during the period from 1995 to 2019, although in their review no more than 10 articles have any relation to the financial sector. In this group of articles, Brunnschweiler and Lujala (Citation2019) show that a relative economic backwardness is related to a greater probability of the appearance of violent and non-violent forms of civil unrest for a sample during the period between 1946 and 2011 including 163 independent countries. Although the authors do not propose that economic backwardness is the main explanation for mass demonstrations or armed civil conflict, the results suggest that civil unrest may appear more naturally in economies that have a delay in their economic development, such as emerging economies, which is in line with studies reporting evidence that civil unrest is more likely to arise in countries with weak economic conditions (Collier & Hoeffler, Citation2004; Elbadawi & Sambanis, Citation2002) and where their economies are more based on extraction and production of commodities (Collier & Hoeffler, Citation2005; Dunning, Citation2005). On another note, Chapman and Reinhardt (Citation2013) found that increases in the sovereign risk of 59 undeveloped countries for the period 1990-2004 are positively related to higher probabilities of civil unrest. Weinberg and Bakker (Citation2015) investigated the relationship between food prices inside a country and social unrest, reporting a positive relationship between them. Barry et al. (Citation2014), who use a cross-national sample of 125 countries from 1981 to 2007, find that freedom of foreign movement and the existence of economic opportunities abroad reduce civil unrest. Kibria et al. (Citation2020) using a sample of 34 countries in Sub Saharan Africa (SSA) between 1972 and 2013 found that an increase in foreign direct investment reduces the risk of civil violence for skilled-labor intensive fuel-resource rich SSA countries. Power (Citation2018), from a relative-deprivation-theory perspective, reports for Ireland that the gap between expectations and lived experiences galvanized and legitimized protest and civic discontent. Power (Citation2018) shows that civil unrest arises even when there is objective information about economic growth, therefore there is a subjective aspect that leads people to social discontent.
Other research has investigated how specifically financial markets have reacted to scenarios of war, economic turbulence, and geopolitical unrest. For example, Leigh et al. (Citation2003) compared price movements in Oil Futures Markets with the Saddam Security prior to the Iraq war in order to assess how it would affect the evolution of oil prices. The authors found that the probability of war increases the price of oil in the short run, and that USA stock market is extremely sensitive to changes in the likelihood of war. Antonakakis et al. (Citation2017) investigated the dynamic structural connectedness between oil price shocks and stock market returns and volatility of 11 major stock markets around the world (net oil–importing and net oil–exporting countries) for the period 1995–2013. Among their findings they report a peak in the connectedness during periods of economic turbulence and geopolitical unrest, such as, the Great Recession, the 2nd war in Iraq and the Start of the Arab Spring. Suzuki and Miah (Citation2010) report that frequent civil unrest which leads to high level of uncertainty is one of the causes of shallow financial development in Georgia and Hong et al. (Citation1999) report that South African boycott had little valuation effect on the financial sector. The authors of this article studied the financial effects of the most visible and successful instance of social activism in investment policies, the boycott of South Africa designed to speed the end of the apartheid regime in 1986. No evidence was found that the anti-apartheid shareholder and legislative boycotts affected the financial sector adversely.
2.3. Civil unrest and herding behavior in Chile
Considering the literature on herding behavior and civil unrest, this research focuses on Chile. This is done for two reasons. First, Chile is a small and open economy that has a well-developed capital market, a significant number of companies participate in it, besides, it has low levels of corruption, a good judicial system, and open and regulated financial markets (Securities Market Law, Public Offerings and Corporate Governance Law). Furthermore, Chile was the first country in the region to establish a private pension fund system which participates in the stock market, giving it greater liquidity and depth. Finally, it is a member of the Latin American Integrated Market (MILA) which gives companies that participate in the capital market greater financing opportunities. As a consequence, investors find greater investment opportunities. All this allows testing herding behavior in a market without intervention and relatively developed.
Second, although various civil unrests have occurred in Chile in the last decade (Aysén, 2012; Magallanes, 2011; Los Lagos, 2016), the most recent one called “Estallido social en Chile” or “Revolución de los 30 pesos” is the most important in the history of the country in democracy. Its origin was on October 7, 2019, manifested in the mass evasion of payment in the Santiago Metro by hundreds of secondary students in response to the rise of 30 pesos (0.05 USD) in the fare of the public transport system of Santiago. In the following days, these acts increased, and people of different natures got involved. On October 18, different demonstrations and attacks were carried out on the Santiago Metro stations, which led to the complete closure of its service on its two main lines. The demonstrations continued with clashes between civilians and the police, barricades in some sectors, incendiary attacks and “cacerolazos” (pots-and-pans banging) in much of Santiago. In the early morning of the following day, President Sebastián Piñera declares a “State of Emergency” in Santiago and the Chacabuco region. This same day the protests continued, including looting that was spread throughout the country. From the following day, massive demonstrations began throughout the country. On October 25, a big march was held, calling more than 1.2 million people in Santiago alone. The consequences of civil unrest in Chile have been diverse and in different areas such as human rights, economy, education, health, and infrastructure, among many others. In economic terms, the Monthly Economic Activity Indicator (Imacec) for the month of October 2019 was −3.4%, being the lowest in the last 10 years. In November it was −3.3%. All of the above makes Chile a natural setting that allows recent observation of how civil unrest can impact herding behavior in stock market.
Considering that Indārs et al. (Citation2019) point out that herding behavior is more pronounced in emerging economies than in developed economies, which is supported by less transparency and quality of information that exists in emerging economies (Balcilar et al., Citation2013), which was reported by Chang and Lin (Citation2015) who investigated 50 international markets for the period 1989–2011 reported herding behavior in the Chilean stock market. In the same line, Nath and Brooks (Citation2020) as well as Christie and Huang (Citation1995) point out that herding behavior should appear during extreme and crisis periods, and that an increase in herding behavior in stock market of Chile during civil unrest would be expected. However, two situations cast doubt on this hypothesis. First, Chiang and Zheng (Citation2010) who investigated 18 international markets including Chile for the period 1989–2009 reported reverse herding behavior, which compares with the study by Chang and Lin (Citation2015) that herding behavior is sensitive to the selected period; and second, the current civil unrest in Chile, due to its magnitude, consequences and duration, is different and more profound than other civil unrests in the past and from various economic and financial crises that have occurred in the country. Thus, the behavior of investors participating in the stock market during this period is uncertain. On the one hand, they could share information and make joint decisions to protect their investments. On the other hand, they could, based on the information available, make individual decisions trying to safeguard their assets. Therefore, and finally considering that there is no evidence regarding how civil unrest impacts herding behavior, the following alternative hypothesis is proposed:
H1a: Civil unrest causes herding behavior in Chile.
H1b: Civil unrest causes reverse herding behavior in Chile.
3. Data and methodology
3.1. Data
The data correspond to the series of daily prices and volumes traded by the companies that make up the General Index of Stock Prices of the Santiago Stock Exchange (S&P/CL IGPA) from January 1, 2010 to February 04, 2020. Companies that during this time were delisted or that did not have data since the beginning of the selected period were not considered. Data from 2010 were considered to observe herding behavior over a long period and to isolate the effect of the subprime crisis. Likewise, data were considered until February 4, 2020 to isolate a potential effect of COVID-19 in Chile, where the first case of contagion was reported on March 05, 2020. Thus, 95 companies and 2,241 observations make up the final sample. Specifically, the research focuses on determining the effect of civil unrest on herding behavior in the short term considering as reference date October 19, 2019 (State of Emergency declaration in the capital of Chile, Santiago) onwards. Different robustness tests are performed around this date.
reports information from the sample. Overall, the sample represents 46% of the companies listed on the Santiago Stock Exchange and 75% of the volume traded in the stock market in 2019. The Financial and Investments sector groups the largest number of companies in the sample, followed by the Industry and Services sector (General and Utilities). These three sectors contain 50% of the sample companies and 55% of the volumes traded during 2019. Considering that herding behavior has been reported in the services sector, specifically in the Pension Fund Administrators (Bravo & Ruiz, Citation2015; Raddatz & Schmukler, Citation2013), and in the Financial sector (Lavin & Magner, Citation2014), specifically in the Mutual Funds industry, there is likely to be herding behavior in the Chilean stock market.
Table 1. Sample information at the end of 2019.
3.2. Methodology
Like Chiang and Zheng (Citation2010) and Tan et al. (Citation2008) the stock returns is calculated as for firms listed on the Santiago Stock Exchange (S&P/CL IGPA) over the period from January 01, 2010 to Febrary 04, 2020 is obtained. This frecuency is used since herding is more evident with daily data than with weekly or monthly (Tan et al., Citation2008).
To detect herding behavior the model proposed by Chang et al. (Citation2000) is used, which is a modification of the model proposed by Christie and Huang (Citation1995). This method has been widely used in the financial literature (Batmunkh et al., Citation2020; Lao & Singh, Citation2011; Mobarek et al., Citation2014; Tan et al., Citation2008; Yao et al., Citation2014). Specifically, Chang et al. (Citation2000) suggest using the following cross-sectional absolute deviation (CSAD) model to facilitate the detection of herding over the entire distribution of market return:
(1)
(1)
where
is the market return (equal-weighted average stock return) and CSADt is a measure of return dispersion calculated as:
(2)
(2)
where
and
are the absolute value of market return and individual stock return of stock i, respectively.
To assess the effect of Civil Unrest on herding the following specification of EquationEquation (1)(1)
(1) is estimated:
(3)
(3)
where
is the cross-sectional absolute deviation define in EquationEquation (2)
(2)
(2) ,
is the market return,
is a dummy variable that takes the value of 1 counting from October 19, 2019 (State of Emergency declaration in the capital of Chile, Santiago) onwards and zero in any other case,
is the standard deviation of market return of the last 30 days and Volume is the logarithm of the daily traded volume in the local market. Significantly negative values of
and
would indicate the presence of herding after (before) of Civil Unrest.
4. Conducting the investigation
4.1. Visual exploration of the sample
First, a visual examination of the data is performed. shows the evolution of the S&P/CL IGPA and CSAD Index during the entire period of the sample. An increase in CSAD is observed during different periods in the last decade, especially when there are strong changes in the direction of the index (green circles).
shows both series during the civil unrest period. There is a sharp drop in the S&P/CL IGPA index from mid-October 2019 (State of emergency) which runs until mid-November 2019 (declaration for social peace and a new constitution). During this same period there is a significant increase in CSAD. This may indicate reverse herding behavior, although this is only exploratory.
4.2. Descriptive statistics of sample
Statistical analysis of data is now performed. reports the descriptive statistics for the CSAD measure and the market return for the complete sample, before and since civil unrest. The mean values (0.410) and standard deviations (0.159) of CSAD are high in the total sample and higher in after civil unrest period (0.490 and 0.281 respectively).
Table 2. Descriptive statistics of CSAD and market return of stock markets (%).
The results for the market returns are overwhelming. Civil unrest has caused a significant drop in the profitability of the Chilean market, confirming the virtual analysis. The average return for the period after civil unrest is −7.8% with a volatility that almost doubles that of the total period before civil unrest. A higher mean value of CSAD suggests significantly higher market variations across stock returns which may suggest that markets have unusual cross-sectional variations due to unexpected events (Chiang & Zheng, Citation2010).
4.3. Civil unrest effect on herding behavior
With the previous information, it is investigated whether civil unrest has an effect on herding behavior. For this the model of EquationEquation (3)(3)
(3) is estimated. The results are reported in . Column 1 reports the general model without control variables and column 2 includes the variables volatility (standard deviation of market return of the last 30 days) and volume (logarithm of the daily traded volume in the local market) as control variables. A positive and statistically significant (at the 1% level) coefficient
= 0.168 is found. The VIF (variance inflation factors) statistic does not report multicollinearity and the errors are calculated using Huber-White robust standard errors.
Table 3. Civil unrest effect on herding behavior.
The results are clear. After civil unrest, considering the date on which President Sebastián Piñera decrees a State of Emergency in the country's capital, there is an inverse herding behavior. The difference between the and
estimates is significant in both cases. Visual, statistical, and econometric analysis confirm that civil unrest caused a significant drop in the return of the stock market and a reverse herding behavior supporting hypothesis H1b: Civil unrest causes a reverse herding behavior in Chile.
4.4. Robust results
4.4.1. Asymmetric effects
4.4.1.1. Asymmetric effects of market return
Pagan and Sossounov (Citation2003) report that stock performs is different in bull runs and bear markets due to fear of further potential loss when the market is lower versus making a potential profit when the market is booming (Mobarek et al., Citation2014). In this context, Demirer and Kutan (Citation2006) point out that the dispersions of the return of the shares are significantly higher during periods of great changes in the aggregate market index. Demirer et al. (Citation2010) find that herding is more prominent during periods of market loss. Lao and Singh (Citation2011) report that herding is higher when the Chinese market is down and, in turn, in India when sudden changes in market conditions occur.
To asymmetric effects of market return is examined whether there is any asymmetry in herd behavior when the market is rising or falling, before and after civil unrest. The results are reported in , columns 1 and 2.
Table 4. Civil unrest effect on herding behavior incorporating asymmetric effects.
4.4.1.2. Asymmetric effects of volatility
Several studies analyze herding behavior in high and low volatility states (Lam & Qiao, Citation2015; Vo and Phan Citation2019; among others). Vo and Phan (Citation2019) find herding behavior under different portfolios depending on the levels of volatility. Tan et al. (Citation2008) finds herding behavior in periods of high volatility in the Chinese market, contrary to what was reported by Batmunkh et al. (Citation2020) for Mongolia.
Considering the above, the possible asymmetric effects of herding behavior with respect to volatility in market performance are examined. It is considered high volatility when the observed volatility becomes higher than the moving average of volatility over the previous 30 days and low volatility when it does not exceed the moving average over the same period (Chang et al., Citation2000). The volatility is calculated as the standard deviation of market return times the square root of 252 trading days. The results are reported in , columns 3 and 4.
4.4.1.3. Asymmetric effects of trading
The literature has reported that the level of herding behavior may be associated with trading volume. Tan et al. (Citation2008) report herding behavior in high-volume states for A-share in China for the period 1994–2003. Lao and Sing (2011) find a similar result for during the period 1999–2009 for China but not for India.
Considering the above, the possible asymmetric effects of herding behavior with respect to domestic market trading volume is examined. High domestic market trading volume is considered when the observed volume is higher than the moving average of volume trading over the previous 30 days and low volume when it does not exceed the moving average over the same period. The results are reported in , columns 5 and 6.
In summary, reports the results of estimating EquationEquation (3)(3)
(3) incorporating the different asymmetric effects previously described. Column 1 and 2 report the results considering the asymmetric effects of market return (Rm,t>0 and Rm,t<0 respectively). Column 3 and 4 show the results considering high and low volatility states (σHIGH > σMAt-30 and σHIGH < σMAt-30 respectively). Column 5 and 6 report the results considering asymmetric effects of high and low domestic market trading volume (volHIGH > volMAt-30 and volLOW < volMAt-30 respectively).
Statistically significant inverse herding behavior () is found in all civil unrest models (except when Rm,t<0) . Regarding the effect of Rm, it is observed that inverse herding behavior is greater when the market is on the rise. In the case of volatility states, reverse herding behavior is stronger in the case of low volatility. Finally, in the case of traded volume, inverse herding behavior is stronger when the traded volumes are lower.
In summary, civil unrest is found to cause reverse herding behavior in the Chilean stock market; second, inverse herding behavior is asymmetric in this period. And third, this behavior is stronger when the market is on the rise, with low volatility and low trading volumes.
4.4.2. Rolling estimation
An asymmetric inverse herding behavior infers that this behavior varies under certain market conditions and could also vary over time. In this context, the contrary results reported by Chiang and Zheng (Citation2010) and Chang and Lin (Citation2015) for Chile confirm that herding behavior is sensitive to the selected period. In fact, Chiang and Zheng (Citation2010) reported herding behavior inverse in the Chilean stock market during the period 1989–2009 while Chang and Lin (Citation2015) reported herding behavior for the period 1989–2011. Thus, for a long period of time it is possible that in the same market there will be herding behavior and reverse herding behavior. To investigate this point and observe the herding behavior dynamics from the beginning of civil unrest in Chile, the process of rolling window regression methodology to detect potential time-varying parameters is applied. Specifically, the following model is estimated:
(4)
(4)
The description of the variables corresponds to those indicated in EquationEquations (1)(1)
(1) and Equation(3)
(3)
(3) . I use a 100-day window. Errors are calculated using Huber-White robust standard errors. Interest is placed on the dynamics of
during the period from October 7, 2019 (first evasion of the Santiago metro) to November 15, 2019 (Agreement for social peace and a new constitution), with special attention to October 19 (State of emergency). A statistical summary of the main results are reported in .
Table 5. Reverse herding behavior during civil unrest.
The S&P/CL IGPA rank is over 4000 points. Similarly, the average return for this period is −12.1%. CSAD presents high and highly volatile values. Specifically, the average of is positive for the period. The models present a good fit and the individual coefficients of
are statistically significant at the 5% level and less since October 21, 2019. shows the evolution of
and S&P/CL IGPA during this period.
Starting on October 7, the date on which the first mass evasion took place in the Santiago Metro and during the following days, reverse herding behavior began to increase until October 19, the day on which a State of Emergency is declared in Santiago. From this date, this behavior declined slightly until November 15, when an agreement for social peace and a new constitution were announced. Since then, reverse herding behavior has increased again, and the same trend tends to remain.
This result shows that in the most critical period of civil unrest these caused a change in inverse herding behavior, first increasing it, and then experiencing a fall as an agreement was advanced to finally resume the values it had before civil unrest.
4.4.3. Herding behavior analysis for period windows
A final robustness test corresponds to estimating the model of EquationEquation (4)(4)
(4) in different time windows. The aim is to observe if inverse herding behavior is present in the most critical period of civil unrest and from here expand the time window to observe if this behavior is maintained or changed. If indeed civil unrest causes inverse herding behavior, a statistically significant positive coefficient
is expected in the most concentrated windows to civil unrest. At the same time, as the size of the window is enlarged, it is expected that this behavior incorporates more information and, therefore, inverse herding behavior would no longer be significant or even, according to the previously reported evidence, it could be negative. In this way, a 6-time windows around October 19 was built, 2019: −30 + 30; −60 + 60; −90 + 90; −120 + 120; −150 + 150; and −180 + 180 days. Finally, the results are compared to those resulting from applying the same procedure around an earlier date in which market conditions were absent from civil unrest (January 02, 2019). Errors are calculated using Huber-White robust standard errors. The results are reported in .
Table 6. Herding behavior analysis for period windows.
Herding behavior is found to exist around civil unrest (Panel A). Column 2 (-60 + 60), column 3 (-90 + 90) and column 4 (-120 + 120) show statistically significant inverse herding behavior. As of the window (-150 + 150) is no longer statistically significant and even when more observations are considered, herding behavior begins to appear, although this is not statistically significant. In Panel B, coefficients close to zero are observed, they are not statistically significant and without a clear pattern. In this period inverse herding behavior disappears.
5. Discussion
Herding behavior and reverse herding behavior are more complex behaviors than the financial literature has reported to date. First, Nath and Brooks (Citation2020) as well as with Christie and Huang (Citation1995) point out that herding behavior should appear during extreme and crisis periods. However, during civil unrest the opposite arises, a reverse herding behavior. In a financial crisis, herding behavior appears which is interpreted as if the market participants act in a “herd” to protect themselves from the potential losses that the crisis may generate. Thus, the least informed, facing an uncertain scenario, follow the behavior of the most informed. On the contrary, in a social crisis, specifically under civil unrest, inverse herding behavior appears which represents just the opposite. That is, the agents that participate in the market make individual decisions, probably questioning the decisions made by the most informed, and discriminating the available information to protect their assets.
Second, the results show that herding behavior does not always occur in emerging economies and leaves some doubt that this behavior is relatively stronger in emerging markets compared to developed markets (Indārs et al., Citation2019). Indeed, on the one hand, Chiang and Zheng (Citation2010) had already reported reverse herding behavior in Chile; and on the other hand, the inverse herding/herding behavior dynamics can vary over a relatively short time horizon. Taking into account that the transparency of a market is the consequence of a set of measures, regulations, and interactions, it is being triggered and incorporated into the market in a longer-term horizon. However, reverse herding/herding behavior has variations not only on long time horizons but also on short horizons. The literature is not conclusive when considering herding behavior over time, with which we cannot affirm that this behavior is relatively stronger in emerging markets compared to developed markets.
Third, the literature on civil unrest has reported that this arises in countries with relative economic backwardness (Brunnschweiler & Lujala, Citation2019) and weak economic conditions (Collier & Hoeffler, Citation2004; Elbadawi & Sambanis, Citation2002). In the last two decades, Chile has been highlighted as a solid economy that has a well-developed capital market, with a significant number of companies participating in it, as well it has low levels of corruption and a good judicial system that, among other characteristics, makes institutions operate relatively effectively. So, how is a civilian unrest of the magnitude of OCT-19 even possible? Power (Citation2018) provides an answer from the perspective of relative deprivation theory documenting the existence of a gap between expectations and lived experiences. This dissociation can lead certain people to put aside objective information and subjective aspects emerge that may lead to social discontent and those who participate in the stock market to reverse herding behavior. The deeper analysis on this point is proposed as a future line of research.
Fourth, civil unrest is more likely in economies that are more based on the extraction and production of commodities (Collier & Hoeffler, Citation2005; Dunning, Citation2005) and in countries where the excess risk increases (Chapman & Reinhardt, Citation2013), among other factors. In turn, the freedom of foreign movement and the existence of economic opportunities abroad (Weinberg & Bakker, Citation2015) as well as an increase in foreign direct investment (Kibria et al., Citation2020) reduce civil unrest. These factors, among others, could be triggers of civil unrest and, therefore, of the results presented in this article. However, the analysis reported show that inverse herding/herding behavior has variations not only in long time horizons but also in short time horizons with which more long-term elements such as those described could hardly provoke changes in short duration herding.
In summary, herding behavior and reverse herding behavior during civil unrest are complex and new behaviors in the literature, therefore more research is required to have a better understanding of how, for example, civil social interactions are related to social interactions in financial markets where there seems to be a dissociation between these interactions, which could be moderated by the value that each individual assigns to their well-being (wealth).
6. Conclusion
This article investigates how civil unrest impact herding behavior in an emerging economy like Chile. Using series of prices and daily traded volumes of the companies that make up the General Index of Stock Prices of the Santiago Stock Exchange (S&P/CL IGPA) from January 1, 2010 to February 04, 2020 civil unrest is found to cause reverse herding behavior in the Chilean stock market. Three different robustness tests support the findings.
This article offers a new area of research to better understand how civil social interactions relate to social interactions in financial markets. Various dimensions and future lines of research arise in this context, such as knowing whether this behavior is similar in countries with a different culture to that of Chile, as countries with a Confucian, Muslim, or other culture. This work also aims at quantifying the determinants of civil unrest to identify how the stock market internalizes it as well as observing different origins of types of civil unrest to have a more complete picture on its extent and effect on the stock markets, among others. Additionally, this work contributes to the social-political literature by reporting that civil unrest not only arises in countries with relative economic backwardness (Brunnschweiler & Lujala, Citation2019) and weak economic conditions (Collier & Hoeffler, Citation2004; Elbadawi & Sambanis, Citation2002). Likewise, it shows how civil unrest has a direct consequence in the stock markets, thus inviting researchers to explore other dimensions that may receive an impact.
Disclosure statement
No potential conflict of interest was reported by the author.
Additional information
Funding
Notes
1 Command: TS= (("civil unrest*") AND ("stock market*" OR "capital market*" OR "capital markets*" OR “financial markets*”)).
References
- Andrikopoulos, P., Kallinterakis, B., Leite, M., & Thanos, V. (2017). Intraday herding on a cross-border exchange. International Review of Financial Analysis, 53, 25–36. https://doi.org/10.1016/j.irfa.2017.08.010
- Andronikidi, A., & Kallinterakis, V. (2010). Thin trading and its impact upon herding: The case of Israel. Applied Economics Letters, 17 (18), 1805–1810. https://doi.org/10.1080/13504850903357327
- Antonakakis, N., Chatziantoniou, I., & Filis, G. (2017). Oil shocks and stock markets: Dynamic connectedness under the prism of recent geopolitical and economic unrest. International Review of Financial Analysis, 50, 1–26. https://doi.org/10.1016/j.irfa.2017.01.004
- Balcilar, M., Demirer, R., & Hammoudeh, S. (2013). Investor herds and regime-switching: Evidence from Gulf Arab stock markets. Journal of International Financial Markets, Institutions and Money, 23, 295–321. https://doi.org/10.1016/j.intfin.2012.09.007
- Barry, C. M., Clay, K. C., Flynn, M. E., & Robinson, G. (2014). Freedom of foreign movement, economic opportunities abroad, and protest in non-democratic regimes. Journal of Peace Research, 51(5), 574–588. https://doi.org/10.1177/0022343314537860
- Batmunkh, M., Choijil, E., Vieito, J., Espinosa-Méndez, C., & Wong, W. (2020). Does herding behavior exist in the Mongolian stock market? Pacific-Basin Finance Journal, 62, 101352. https://doi.org/10.1016/j.pacfin.2020.101352
- Blasco, N., Corredor, P., & Ferreruela, S. (2012). Does herding affect volatility? Implications for the Spanish stock market. Quantitative Finance, 12 (2), 311–327. https://doi.org/10.1080/14697688.2010.516766
- Boako, G., Tiwari, A. K., Ibrahim, M., & Ji, Q. (2019). Analysing dynamic dependence between gold and stock returns: Evidence using stochastic and full-range tail dependence copula models. Finance Research Letters, 31, 391–397. https://doi.org/10.1016/j.frl.2018.12.008
- Bravo, F., & Ruiz, J. L. (2015). Herding behavior and default in funded pension schemes: The Chilean case. Emerging Markets Finance and Trade, 51 (6), 1230–1243. https://doi.org/10.1080/1540496X.2015.1080526
- Brunnschweiler, C., & Lujala, P. (2019). Economic backwardness and social tension. The Scandinavian Journal of Economics, 121(2), 482–516. https://doi.org/10.1111/sjoe.12281
- Chapman, T., & Reinhardt, E. (2013). Global credit markets, political violence, and politically sustainable risk premia. International Interactions, 39(3), 316–342. https://doi.org/10.1080/03050629.2013.782302
- Chang, C. H., & Lin, S. J. (2015). The effects of national culture and behavioral pitfalls on investors' decision-making: Herding behavior in international stock markets. International Review of Economics & Finance, 37, 380–392. https://doi.org/10.1016/j.iref.2014.12.010
- Chang, E. C., Cheng, J. W., & Khorana, A. (2000). An examination of herd behavior in equity markets: An international perspective. Journal of Banking & Finance, 24(10), 1651–1679. https://doi.org/10.1016/S0378-4266(99)00096-5
- Chang, S. K. (2014). Herd behavior, bubbles and social interactions in financial markets. Studies in Nonlinear Dynamics and Econometrics, 18(1), 89–101. https://doi.org/10.1515/snde-2013-0024
- Chaudhry, M. I., & Sam, A. G. (2018). Herding behavior and the declining value relevance of accounting information: Evidence from an emerging stock market. Applied Economics, 50(49), 5335–5353. https://doi.org/10.1080/00036846.2018.1486989
- Chiang, T., & Zheng, D. (2010). An empirical analysis of herd behavior in global stock markets. Journal of Banking & Finance, 34(8), 1911–1921. https://doi.org/10.1016/j.jbankfin.2009.12.014
- Christie, W. G., & Huang, R. D. (1995). Following the pied piper: Do individual returns herd around the market? Financial Analysts Journal, 51(4), 31–37. https://doi.org/10.2469/faj.v51.n4.1918
- Chung, C. Y., Liu, C., & Wang, K. N. (2016). Institutional investor trading in a short investment horizon: Evidence from the Korean Stock Market. Emerging Markets Finance and Trade, 52(4), 1002–1012. https://doi.org/10.1080/1540496X.2015.1025648
- Collier, P., & Hoeffler, A. (2004). Greed and grievance in civil war. Oxford Economic Papers, 56(4), 563–595. https://doi.org/10.1093/oep/gpf064
- Collier, P., & Hoeffler, A. (2005). Resource rents, governance, and conflict. Journal of Conflict Resolution, 49(4), 625–633. https://doi.org/10.1177/0022002705277551
- Demirer, R., & Kutan, A. (2006). Does herding behavior exist in Chinese stock markets? Journal of International Financial Markets, Institutions and Money, 16(2), 123–142. https://doi.org/10.1016/j.intfin.2005.01.002
- Demirer, R., Kutan, A., & Chen, C. (2010). Do investors herd in emerging stock markets?: Evidence from the Taiwanese market. Journal of Economic Behavior & Organization, 76(2), 283–295. https://doi.org/10.1016/j.jebo.2010.06.013
- Dunning, T. (2005). Resource dependence, economic performance, and political stability. Journal of Conflict Resolution, 49(4), 451–482. https://doi.org/10.1177/0022002705277521
- Economou, F., Katsikas, E., & Vickers, G. (2016). Testing for herding in the Athens Stock Exchange during the crisis period. Finance Research Letters, 18, 334–341. https://doi.org/10.1016/j.frl.2016.05.011
- Economou, F., Kostakis, A., & Philippas, N. (2011). Cross-country effects in herding behavior: Evidence from four south European markets. Journal of International Financial Markets, Institutions and Money, 21(3), 443–460. https://doi.org/10.1016/j.intfin.2011.01.005
- Elbadawi, I., & Sambanis, N. (2002). How much war will we see? Explaining the prevalence of civil war. Journal of Conflict Resolution, 46 (3), 307–334. https://doi.org/10.1177/0022002702046003001
- Ennis, R. M., & Sebastian, M. D. (2003). A critical look at the case for hedge funds - Lessons from the bubble. The Journal of Portfolio Management, 29(4), 103–112. https://doi.org/10.3905/jpm.2003.319899
- Hirshleifer, D., Subrahmanyam, A., & Titman, S. (1994). Security analysis and trading patterns when some investors receive information before others. The Journal of Finance, 49(5), 1665–1698. https://doi.org/10.1111/j.1540-6261.1994.tb04777.x
- Hong, S. H., Welch, I., & Wazzan, P. (1999). The effect of socially activist investment policies on the financial markets: Evidence from the South African boycott. The Journal of Business, 72(1), 35–89.
- Indārs, E. R., Savin, A., & Lublóy, Á. (2019). Herding behavior in an emerging market: Evidence from the Moscow Exchange. Emerging Markets Review, 38, 468–487. https://doi.org/10.1016/j.ememar.2018.12.002
- Kabir, M. H., & Shakur, S. (2018). Regime-dependent herding behavior in Asian and Latin American stock markets. Pacific-Basin Finance Journal, 47, 60–78. https://doi.org/10.1016/j.pacfin.2017.12.002
- Kibria, K., Oladi, R., & Kibria, S. (2020). Foreign direct investment and civil violence in Sub‐Saharan Africa. The World Economy, 43(4), 948–981. https://doi.org/10.1111/twec.12904
- Lam, K. S., & Qiao, Z. (2015). Herding and fundamental factors: The Hong Kong experience. Pacific-Basin Finance Journal, 32, 160–188. https://doi.org/10.1016/j.pacfin.2014.11.002
- Lancaster, L. (2018). Unpacking discontent: Where and why protest happens in South Africa. South African Crime Quarterly, 64(64), 29–43. https://doi.org/10.17159/2413-3108/2018/v0n64a3031
- Lao, P., & Singh, H. (2011). Herding behavior in the Chinese and Indian stock markets. Journal of Asian Economics, 22(6), 495–506. https://doi.org/10.1016/j.asieco.2011.08.001
- Lavin, J., & Magner, N. (2014). Herding in the mutual fund industry: Evidence from Chile. Academia Revista Latinoamericana de Administración, 27(1), 10–29. https://doi.org/10.1108/ARLA-09-2013-0137
- Leigh, L., Wolfers, J., & Zitzewitz, R. (2003). What do financial markets think of war in Iraq? NBER Working Paper No. 9587. National Bureau of Economic Research, Inc.
- Mobarek, A., Mollah, S., & Keasey, K. (2014). A cross-country analysis of herd behavior in Europe. Journal of International Financial Markets, Institutions and Money, 32, 107–127. https://doi.org/10.1016/j.intfin.2014.05.008
- Mueller, A., & Brettel, M. (2012). Impact of biased pecking order preferences on firm success in real business cycles. Journal of Behavioral Finance, 13(3), 199–213. https://doi.org/10.1080/15427560.2012.708372
- Najmudin, N., Syarif, D. H., Wahyudi, S., & Muharam, H. (2017). Applying an international CAPM to herding behaviour model for integrated stock markets. Journal of International Studies, 10(4), 47–62. https://doi.org/10.14254/2071-8330.2017/10-4/3
- Nath, H., & Brooks, R. (2020). Investor-herding and risk-profiles: A state-space model-based assessment. Pacific-Basin Finance Journal, 62, 101383. https://doi.org/10.1016/j.pacfin.2020.101383
- Nicolis, S. C., & Sumpter, D. J. T. (2011). A dynamical approach to stock market fluctuations. International Journal of Bifurcation and Chaos, 21(12), 3557–3564. https://doi.org/10.1142/S0218127411030726
- Noguera, J. (2016). Geopolitics and the oil price. Economic Modelling, 52, 301–309.
- Omay, T., & Iren, P. (2019). Behavior of foreign investors in the Malaysian stock market in times of crisis: A nonlinear approach. Journal of Asian Economics, 60, 85–100. https://doi.org/10.1016/j.asieco.2018.11.002
- Pagan, A. R., & Sossounov, K. A. (2003). A simple framework for analyzing bull and bear markets. Journal of Applied Econometrics, 18(1), 23–46. https://doi.org/10.1002/jae.664
- Pochea, M. M., Filip, A. M., & Pece, A. M. (2017). Herding behavior in CEE Stock Markets under asymmetric conditions: A quantile regression analysis. Journal of Behavioral Finance, 18(4), 400–416. https://doi.org/10.1080/15427560.2017.1344677
- Power, S. (2018). The deprivation-protest paradox, how the perception of unfair economic inequality leads to civic unrest. Current Anthropology, 59(6), 765–789. https://doi.org/10.1086/700679
- Raddatz, C., & Schmukler, S. (2013). Deconstructing herding: Evidence from pension fund investment behavior. Journal of Financial Services Research, 43(1), 99–126. https://doi.org/10.1007/s10693-012-0155-x
- Suzuki, Y., & Miah, M. D. (2010). The civil unrest as an obstacle toward financial development in Georgia, 1991–2007. Communist and Post-Communist Studies, 43(4), 425–438. https://doi.org/10.1016/j.postcomstud.2010.10.009
- Tan, L., Chiang, T., Mason, J., & Nelling, E. (2008). Herding behavior in Chinese stock markets: An examination of A and B shares. Pacific-Basin Finance Journal, 16(1–2), 61–77. https://doi.org/10.1016/j.pacfin.2007.04.004
- Vo, X. V., & Phan, D. B. A. (2019). Herd behavior and idiosyncratic volatility in a frontier market. Pacific-Basin Finance Journal, 53, 321–330.
- Waheed, A., & Mathur, I. (1993). The effects of announcements of bank lending agreements on the market values of united-states banks. Financial Management, 22(1), 119–127. https://doi.org/10.2307/3665971
- Weinberg, J., & Bakker, R. (2015). Let them eat cake: Food prices, domestic policy and social unrest. Conflict Management and Peace Science, 32(3), 309–326. https://doi.org/10.1177/0738894214532411
- Yao, J., Ma, C. C., & He, W. P. (2014). Investor herding behavior of Chinese stock market. International Review of Economics & Finance, 29, 12–29. https://doi.org/10.1016/j.iref.2013.03.002