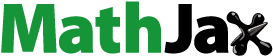
Abstract
The present study investigates the dynamic and asymmetric impacts of eco-innovation and human capital development on ambient pollution by validating the Environment Kuznets Curve (EKC) hypothesis in China from 1988Q1 to 2018Q4. The findings confirm non-normality and structural breaks in data. Thus, Quantile Autoregressive Distributive Lag (QARDL) model and Granger Causality-in-Quantiles are applied to address non-linearity and structural breaks. The long-run results exhibit that eco-innovation and human capital have a significant negative relationship with carbon emissions, mainly from lower (0.05) to medium (0.5) quantiles and medium (0.50) to higher (0.95) emissions quantile. Moreover, economic growth contributes to higher emissions across all quantiles. In contrast, the square of economic growth has a significant negative association with emissions, confirming the validity of EKC from medium (0.40) to higher (0.95) quantiles. Lastly, Granger causality confirms a two-way causality between eco-innovation, human capital, and carbon emissions, and a one-way causality from human capital, economic growth to carbon emissions. These findings offer valuable policy recommendations.
1. Introduction
Energy is a primary input in manufacturing, disseminating, and consuming nearly all goods and services worldwide (Sharif et al., Citation2019; Zhang et al., Citation2021). Energy is essential to increase economic growth and development, improve quality and standard of life, and offer many other benefits (Chiu & Lee, Citation2020). The increase in energy use for promoting industrialization causes global warming and greenhouse gases (GHGs) emissions in the atmosphere (Ozturk & Acaravci, Citation2013; Zhuang et al., Citation2021). Climatic change due to the greater concentration of GHG has been viewed as a key concern in the twenty-first century (Bano et al., Citation2018; Sharma et al., Citation2021). Global warming and the subsequent environmental concerns are extensively supposed to be the leading causes of future problems that will transact national borders (Sinha et al., Citation2020a, Citation2020b, Citation2020c; Yurdakul & Kazan, Citation2020). Likewise, GHG emissions of GHGs raise atmospheric temperature globally, which becomes a severe threat for both developing and developed nations (Saleem & Shujah-Ur-Rahman, Citation2019; Sinha & Rastogi, Citation2017). Gu and Wang (Citation2018) investigated that research and development (R&D) investment at the company level becomes a necessary tool for reducing GHGs emissions. Expenditure in R&D in the companies is reflected as an operative instrument to improve environmental tactics that frequently allow sustainable products and services (Frondel et al., Citation2007; Lee & Min, Citation2015; Nasiritousi, Citation2017; Porter & Van der Linde, Citation1995). Expenditure in physical assets, investment in R&D, and human capital training require a long-term commitment by implementing the idea of eco-innovation (Cucchiella et al., Citation2017; Fernández et al., Citation2018).
The notion of eco-innovation has been reflected as a root for anticipating environmental damages due to GHGs and is expected to decrease the quantity of waste, material resource usage, and air pollution (Hojnik & Ruzzier, Citation2016; Sun et al., Citation2021; Yurdakul & Kazan, Citation2020). Eco-innovation is defined as new ideas for improving processes and products and reducing environmental burden (Dangelico & Pujari, Citation2010; Fussler & James, Citation1996; Garcia-Granero et al., Citation2018; Mensah et al., Citation2018). According to Serrat (Citation2009), organizations can improve their product quality with the help of product innovation, and its cost can be reduced with the help of process innovation. Eco-product innovation can be achieved by improving existing or new products by reducing environmental concerns (Reid & Miedzinski, Citation2008). Likewise, eco-process innovation necessitates an amendment in existing business processes and systems by R&D investment, cutting resource costs and technological investment, and reducing greenhouse gas emissions (Cheng et al., Citation2014; Fethi & Rahuma, Citation2020; Mensah et al., Citation2018; Rennings, Citation2000). Eco-innovation could be a key pointer for executing a sound environmental tactic and help improve operational efficiency, future sustainability, and environmental performance (Fethi & Rahuma, Citation2020; Lopez-Gamero et al., Citation2010).
Human capital (HC) is deliberated as a substantial constituent that plays an imperative part in plummeting energy ingestion through improving energy efficiency (Bano et al., Citation2018). HC is one of the essential parameters for innovation, add value during the production process, plays an essential role in economic growth, and permitting developments in energy depletion competence and reduction in carbon emissions (CE) (Armstrong, Citation2016; Fang & Chang, Citation2016; Huang et al., Citation2021; Razzaq et al., Citation2021a). As an imperative cause of knowledge aggregation and technological revolution, HC is a significant component in improving green production through energy conservation, emission reduction, and environmental regulation (An et al., Citation2021). Pablo-Romero and Sánchez-Braza (Citation2015) have found that more educated laborers can act as a substitute for energy, as enhancement in HC maintains technological advancement, which moreover decreases the implementation cost of these technologies, whereas utilizing such technologies leads to more ecologically friendly production (Dasgupta et al., Citation2000; Kim & Lee, Citation2011). Some prior studies propose that technological advancement can supplant labor and capital speculation while, on the other hand, it increments the request energy as well (Jiao et al., Citation2018; Yang & Li, Citation2017). Accordingly, the progressive outcome of technological innovation on the environment can be found merely in nations with a greater mix of renewable energy.
On the contrary, some other studies suggested that environmental-related technologies (low carbon) can play a substantial part in diminishing CE (De Jesus et al., Citation2018; Lee & Min, Citation2015). Moreover, De Jesus et al. (Citation2018) also highlighted that eco-innovation could achieve environmental sustainability. Becker (Citation2009) divides HC venture into different practices such as health care, quality of life, social setting, school-level education, and work-related training. There are many benefits by investing in HC, such as HC adds to greater labor productivity, economic growth, and is concomitant with a variation of societal externalities likewise less inequalities, abidance of government rules, better health, and deterioration in crime ratios (Rist, Citation2019; Romer, Citation1990; Schultz, Citation1961; Sianesi & Reenen, Citation2003). Desha et al. (Citation2015) determined that improvement in HC with the help of knowledge, awareness, and education leads to increases renewable energy consumption. HC can be classified into three forms: first, the general HC, which is also known as HC stock and it is the amalgamation of education and general experience; the second one is called as firm-specific HC, which is the combination of skills, knowledge, and firm-level education; and third, task-specific HC, representing job-related training, knowledge, and skills (Au et al., Citation2008; Kwon, Citation2009).
Most of the prior studies used the Environmental Kuznets Curve (EKC) phenomena to analyze the relationship between economic growth and environmental change. The concept of EKC was presented by Kuznets (Citation1955). The EKC demonstrates that at the early economic growth, increases in the atmosphere due to greater use of economic resources in the production process, but when a certain level of threshold of economic development is achieved, it will improve the environment. Shafik and Bandyopadhyay (Citation1992) first analyze the association between economic growth and CE under EKC phenomena, and the results showed an increasing trend. A detailed review of this association is provided by Shahbaz and Sinha (Citation2019).
Likewise, Choi et al. (Citation2012) investigated the association between economic growth and CE under KEC hypothesis in developed economies. The study's findings revealed a positive relationship between and CE at an early stage of economic development, but later as the economic growth increases, the CE decreases. So, it implies that when economic growth is in the early stages, the natural resources are in plentitude with limited waste generation, but amid industrialization and advancement, the consumption of natural resources upsurges which come about in a significant positive relationship between economic growth and CE. As the nation gets in the developed stage of improved technology, it lessens the consumption of resources and results in an enhanced environment (Panayotou, Citation2016). The findings are also supported by some prior studies Dinda and Coondoo (Citation2006), Grossman and Krueger (Citation1995), Stern (Citation2004), and Sinha and Shahbaz (Citation2018).
China is one of the developing countries which shown tremendous economic growth over the last few decades; this growth is the result of increasing the consumption of fuel energy which will ultimately cause an increase in CE (Lapinskienė et al., Citation2017; Nguyen & Le, Citation2018; Tian et al., Citation2017). The government of China is taking Proactive initiatives to enhance energy efficiency and trying to develop renewable energy. Regardless of these incentives, CE in China is still high compared to other developed countries (Tan et al., Citation2011). The government of China commits at the Paris Climate Conference 2015 to reduce CE in a range between 60% and 65% till 2030 and try to incorporate this target in their long-term national projects respectively (Nguyen & Le, Citation2018).
From the above discussion, it can be said that eco-innovation and HC are important components for reducing CE. The silent feature of eco-innovation is that it helps in reducing environmental burden by giving new ideas for improving process and product manufacturing. HC ascertained to be a substantial component in improving green production through energy conservation and emission reduction. The said study analyzed the impact of eco-innovation and HC on environmental degradation by using QARDL approach. The motivation behind utilizing the QARDL is to test the long-term relationship over the quantiles of dependent variables, i.e., DJCM and DJIM, besides the conceivable asymmetric association with the exogenous variables in thought. Examination of asymmetries is the most advantage of QARDL over the linear ARDL approach (Xiao, Citation2009). Asymmetric association among the variables is due to the nonlinear relationship, which shows the changing impacts of regressors beneath various regressands (Cheng et al., Citation2021; Godil et al., Citation2020; Mishra et al., Citation2019; Shahbaz et al., Citation2018a, Citation2019a). In this study, asymmetries in relationship with eco-innovation and HC are analyzed regarding the aforementioned environmental degradation. The QADRL approach handles the long- and short-run association of eco-innovation and HC with environmental degradation and locating asymmetries under various quantiles.
The remaining paper is organized as takes after: The outline of the previous studies is created in the literature review section. The inquire about the research approach and data analysis techniques are debated in the methodology section. Data investigation and its discussion appear in result and interpretation, while the conclusion and policy implication appears in the conclusion segment.
2. Literature review
Eco-innovation has been recorded as an effective approach to addressing environmental problems (Lingyan et al., Citation2021; Zhang et al., Citation2017). Yurdakul and Kazan (Citation2020) examined the effect of eco-innovation on CE and firm financial performance on a sample of 219 Turkish manufacturing firms. The results from the SEM revealed that eco-innovation is significantly positively related to resource-saving, recycling, and pollution prevention. Razzaq et al. (Citation2021b) argued that eco-innovation has important implications for the environment. Sun et al. (Citation2021) investigated the role of eco-innovation and globalization in mitigating CE in the case of the USA. The study used QARDL approach to estimate the long-run and the short-run association between selected variables. The findings of the study revealed that eco-innovation acts as a mitigating factor of CE. The study also supports the existence of EKC for the USA. Ding et al. (Citation2021) determined the impact of eco-innovation, international trade, and energy on CE for G7 countries from 1990 to 2018. The findings from the panel causality test suggested that eco-innovation, trade, and energy are the primary factors of consumption-based CE in the G7 countries.
Erdoğan et al. (Citation2020) analyzed the importance of environmental innovation in reducing CE in G20 countries. The author found that environmental innovation in the industrial sector reduces CE. Ji et al. (Citation2021) used data from seven highly fiscally decentralized countries, Australia, Austria, Belgium, Canada, Germany, Spain, and Switzerland, from 1990 to 2018. The findings from econometric analysis revealed that eco-innovation reduces CE. This study recommends that any policy that targets green growth will affect CE. Yang and Li (Citation2017) supported the role of eco-innovation to achieve the low-carbon emissions target. The author found that eco-innovation is helpful in reducing CE by improving carbon emission efficiency. Ali et al. (Citation2021) examined the role of environmental innovation, trade, and renewable energy consumption in the nexus between trade and CE for the top 10 carbon emitter countries. The results of the Westerlund cointegration method suggest a long-term equilibrium relationship between environmental innovation, trade, and renewable energy consumption and CE.
Hojnik and Ruzzier (Citation2016) concluded that eco-innovation in the form of greener technology has a long-term capacity to improve environmental quality. Wang et al. (Citation2020) explored the effect of eco-innovation and export diversification on CE on panel data of G7 countries from 1990 to 2017. The result of the study shows that eco-innovation help in reducing CE in G7countries. Mensah et al. (Citation2018) explore the impact of eco-innovation on environmental degradation in 28 OCED nations at an individual level for the time period 1990–2014. The study used three models based on the economic-EKC development model, the STIRPAT model, and the innovation-EKC model. This research showed that innovation plays a noteworthy part in alleviating CE in most OECD nations.
Fethi and Rahuma (Citation2020) use the Porter hypothesis in the short run and long run to consider the dynamic influence of three eco-innovation indicators, namely HC training, investment, and R&D on CE in selected petroleum firms. The study applied second-generation panel regression on quarterly data over the period 2005–2016. The results demonstrate that investment as one of the gauges of eco-innovation altogether diminishes CE in the long-run, while R&D and HC make substantial reductions in CE in the short run. Garrone and Grilli (Citation2010), discovered the association between R&D and CE for thirteen advanced countries. The study used annual data over the period 1980–2004. The OLS regression and GMM difference estimator findings revealed that R&D spending is not adequate to stimulate innovation in energy. To extend the argument, Yii and Geetha (Citation2017) examined the relationship between technological innovation and CE for the Malaysian economy from 1971 to 2013. The results from ARDL approach and Granger causality test revealed a negative relationship between technological innovation and CE in the short run, while no such association is observed in the long run in the said economy.
Ramanathan et al. (Citation2017) scrutinized the relationship between eco-innovation, sustainable benefits in terms of pollution reduction, environmental regulations, and CE impact on 9 case studies of Chines and British companies. They perceived that corporations that depend on their assets and capabilities move their commitments towards sustainability through decreases in pollution and enhanced performance. Zhang et al. (Citation2017) examined the impact of eco-innovation on CE in China. The study utilized panel data of 30 provinces of China for the period 2000–2013. Empirical results from GMM estimation technique revealed that eco-innovation reduces CE in China effectively. Alam et al. (Citation2019) studied the impact of R&D investment on the environment in G6 countries from 2004 to 2016. The findings of study revealed a significant positive association between R&D investment and CE reductions.
Huang et al. (Citation2021) found the impact of HC on carbon emissions in 30 provinces in China from 1998 to 2017. The overall results from the quantile regression model and spatial panel lag model revealed that primary, knowledgeable, skilled, and institutional HC would offer assistance in decreasing carbon emissions in various selected regions. Shahbaz et al. (Citation2019b) found that HC plays a noticeable part in controlling energy consumption. HC also helps to increase renewable energy consumption because of education, awareness, and knowledge about energy security (Desha et al., Citation2015). HC promotes technology advancement and decreases the execution cost of these technologies (Kim & Lee, Citation2011). Ahmed and Wang (Citation2019) scrutinized the impact of HC on the ecological footprint in India over the period 1971–2014. It appears from the research findings that higher HC mitigates the ecological footprint in the long-run and short-run. Bano et al. (Citation2018) examined HC's short- and long-term impact on CE in Pakistan from 1971 to 2014 by employing an autoregressive distributed lag model and the vector error correction model. The study's finding shows a long-term association between HC and CE. It also shows a two-way causal relationship between HC and CE in the long run. Li and Ouyang (Citation2019) found the long-run cointegration linkage between HC and CE in China from 1978 to 2015 by using the ARDL approach. Mehrara et al. (Citation2015) expressed that HC measured by tertiary education is imperative for renewable energy consumption and maintains renewable energy through information sharing, skilled labor, and financial-economic development. Yao et al. (Citation2020) found the relationship between HC and CE for 20 OECD countries from 1870 to 2014. The result from cross-sectional dependence and structural breaks proposed that HC is related to a diminishment in CE. Furthermore, a higher level of human capital might also promote enterprises to strictly follow related environmental standards, which will also help reduce CO2 emission intensity (Li & Ouyang, Citation2019).
Yuan and Zhang (Citation2017) suggested that HC, as an essential source of knowledge accumulation and technological innovation, can promote green production through energy-saving and technological innovation. Iqbal et al. (Citation2021) examined the impact of trade openness, urbanization, and HC on environmental degradation using the panel data of 126 economies for the years 1971–2020. The study also extends the analysis for four sub-panels, namely, high-income economies (HIC), upper-middle-income economies (UMIC), lower-middle-income economies (LMIC), and low-income economies (LIC) by using fully modified least squares (FMOLS), dynamic ordinary least squares (DOLS), fixed effects (FEM), random effects (REM), and system GMM. The results show that enhancement in HC will lessen emissions in all economies. Therefore, economies should invest in human capital to combat emissions.
Besides, Yao et al. (Citation2019) centered on determinants of renewable energy consumption (REC) and non-renewable energy consumption (NREC) for high-income part nations by utilizing data from 1965 to 2014. They have found a negative relationship between HC and NREC, though a positive relationship between HC and REC. Adom (Citation2015) analyzed the asymmetric impact of the elements affecting energy intensity within the case of Nigeria from 1971 to 2011 and has found that HC improvement upgrades energy consumption in the case of Nigeria. Lin et al. (Citation2021) examined the effect of innovative HCl on CE in China. The provincial panel data of 30 Chinese provinces from 2003 to 2017 was analyzed utilizing the fixed effect, OLS, and the system GMM. The analysis revealed that innovative HC alleviates environmental deterioration in China. The outcomes unfold the presence of the EKC considering innovative HC in the model. Wang and Xu (Citation2021) observationally analyze the relationship between internet usage, HC, and CE beneath the diverse level of financial development by using system GMM and a threshold regression model on the panel data of 70 nations from 1995 to 2018. The outcomes appear internet usage and HC are basic drivers of low-carbon economy development, and HC can inversely regulate the impact of internet usage on CE.
3. Material and methods
3.1. Quantile ARDL approach
To investigate the asymmetric and dynamic association between eco-innovation, human capital, economic growth, and CE in China, the current study utilized Quantile Auto Regressive Distributed Lag (QARDL) approach, which was developed by Cho et al. (Citation2015). The QARDL approach is an extension of ARDL approach, and this strategy permits testing the asymmetries and long-term equilibrium relationship between eco-innovation (EI), human capital (HC), economic growth (GDP), square economic growth (SQGDP), and carbon emissions (CE). The study moreover utilized the Wald test to decide the cointegration affiliated among the said factors. The test permits checking the steadiness of integration coefficients through a range of quantiles. The equation for ARDL model is as follows:
(1)
(1)
where
is the error term,
and
and
refer to the eco-innovation, human capital, ECO_GRO, and square economic growth individually, whereas
signifies CE. Cho et al. (Citation2015) extended the model in EquationEq. (1)
(1)
(1) to a quantile setting and presented the following basic form of the QARDL (p, q, r, s, u) model:
(2)
(2)
where,
and 0 >
< 1 illustrate quantile (Kim & White, Citation2003). Owing to the chance of a sequential relationship, the QADRL demonstrate appeared in EquationEq. (2)
(2)
(2) is generalized as takes after:
(3)
(3)
The parameters in EquationEq. (3)(3)
(3) degree the short-term dynamics, whereas the long-term connections between eco-innovation, human capital, and carbon dioxide spread emanation can be captured by reformulating adaptation of EquationEq. (3)
(3)
(3) moreover to avoid the serial correlation of ε, we generalize the QARDL as takes after:
(4)
(4)
By utilizing the delta strategy, the aggregate short-run effect of previous carbon dioxide outflow on present carbon dioxide emanations is decided by:
Whereas the aggregate short term effect of the past and current levels of EI, HC, GDP, and SQGDP are determined by
The factor associated to long-run for eco-innovation, human capital, economic growth, and square economic growth is calculated as:
It might be noted that the ECM parameter out to be essentially negative. Wald test is applied to look at the validity of short and long-run asymmetric impact of parameters.
opposite to an alternative one
3.2. Data and variables
This research contains five variables, Carbon emissions (CE), Eco-innovation (EI), Human capital (HC), economic growth (GDP), and square economic growth (SQGRP). shows the detail of variables along with measurement, source, and empirical justifications.
Table 1. Detail and justification of variables.
By virtue of limited data, the annual data was further transformed into quarterly observations by using the quadratic match sum method used by Aziz et al. (Citation2020a), Godil et al. (Citation2020), and Shahbaz et al. (Citation2018b). The match sum quadratic method is very effective as this method converts the data from low frequency into high frequency. This method allows amendments for seasonal deviations by dropping end-to-end data deviation (Godil et al., Citation2020; Mishra et al., Citation2020). The graphical trend of carbon emissions, Eco-innovation, Human capital in China from 1988 to 2018 is provided in .
3.3. Descriptive statistics
Measures of central tendency are represented by mean value, median value, minimum value, and maximum value. shows the summary statistics of all the variables used in the study, the minimum value, mean value, and the maximum value of selected variables shows positive numbers i.e., CE (M = 2.030, Min= 4.461, Max = 7.060), EI (M = 4.918, Min = 7.107, Max = 10.286), HC (M = 1.847, Min = 2.252, Max = 2.581), GDP (M = 2.855, Min = 3.423, Max = 3.895), SQGDP (M = 5.710, Min = 6.846, Max = 7.789). In order to validate the normality of the data, this research study used the Jarque-Bera test. The test outcome showed that all the null hypotheses of data linearity were rejected, which instigated us to apply the QADRL approach (Batool et al., Citation2019; Godil et al., Citation2020; Sinha et al., Citation2021; Troster et al., Citation2018). The quantile distribution of data for each variable is presented in .
Table 2. Descriptive statistics.
4. Results and discussion
4.1. Unit root test
The unit root test was used to find the stationarity of the data. For QADRL approach, it is necessary to confirm the order on integration in a given data set. According to Li and Ouyang (Citation2019), all the regressors used in the QADRL approach must be integrated at level I (0) or at the first difference I (1). therefore, this research study applied two unit root tests, the first one is the Zivot and Andrews (Citation2002) unit root test (ZA), and the second one is Augmented Dickey-Fuller unit root test (ADF). The ZA test is essential as this test help to determine the structural breaks in the given data. shows the results of the ZA unit root test and ADF unit root test. The findings showed that all the selected time series variables are I (1) and stationary either at a 5% or 10% level of significance, except HC, which is found to be I (0) as well as I(1). The results revealed that all the variables have unique order of integration I (1). Hence, it is confirmed that the QADRL approach is an appropriate method that elaborates structural breaks, dynamic trends, and non-linearity in the data (Aziz et al., Citation2020b Sharif et al., Citation2020a,Citation2020b; Razzaq et al., Citation2021).
Table 3. Unit root test.
reports the outcomes of QARDL approach. The finding shows that the estimated parameter of dependency ρ is highly significant with a negative sign in all 11 quantiles (0.05–0.95). It shows the long-run equilibrium relationship between eco-innovation, human capital, economic growth, square economic growth, and CE. Eco-innovation βEI shows a significant negative relationship with CE at lower (0.05) to medium (0.50) levels of quantiles. These findings are supported by some prior studies (Ding et al., Citation2021; Ji et al., Citation2021; Sun et al., Citation2021; Wang et al., Citation2020) and inconsistent with (Ahmad et al., Citation2021; Ozcan & Apergis, Citation2018). The study considers that eco-innovation shall be helpful in support of environmental quality. The author contended that energy efficiency technologies accommodate swapping the financial structure to more sustainable energy sources, renewable energies. Likewise, human capital βHC indicates a significant negative relationship with CE at medium (0.50) to highest (0.95) level of quantiles. The findings showed the presence of long-run asymmetric relationship between HC and CE for China. The findings of this study are consistent with some prior studies (Bano et al., Citation2018; Bastola & Sapkota, Citation2015; Chengliang et al., Citation2017; Huang et al., Citation2021; Iqbal et al., Citation2021; Lin et al., Citation2021). As a fundamental source of information aggregation and technological innovation, human capital can advance green production through energy-saving and technological innovation. Economic growth βGDP, the results show a significant positive relationship with CE at lower (0.20) to highest (0.95) level of quantiles, and the results are supported by Alkhathlan and Javid (Citation2013), Begum et al. (Citation2015), Hussain et al. (Citation2012), Ji et al. (Citation2021) and Sun et al. (Citation2021). In contrast, the square of economic growth βSQGDP specifies a significant negative relationship with CE in lower (0.40) to highest (0.95) level of quantile. The βSQGDP indicate the EKC hypothesis, justified through the negative coefficient value at only lower (0.40) to highest (0.95) level of quantiles in the long run. These findings are echoed by recent literature (Lin et al., Citation2021; Saleem & Shujah-Ur-Rahman, Citation2019; Sun et al., Citation2021; Xuefeng et al., 2021).
Table 4. Results of quantile autoregressive distributed lag.
The short-run relationship among the selected variables for QARDL approach is also presented in . The study's findings show that the fluctuations in the current CE are significantly positively influenced by their own past at the grid of all quantiles from lower (0.05) to highest (0.95). In the short-run, eco-innovation (EI) indicates a significant negative relationship with CE at lower (0.40) to higher (0.70) level of quantiles and insignificant at the rest of the quantiles. Likewise, human capital (HC) also shows a significant negative relationship with CE at the grid of higher (0.70) to highest (0.95) level of quantiles. For economic growth, GDP shows a significant positive association with CE at lower (0.20) to highest (0.95) level of quantiles. Lastly, the findings for square economic growth (SQGDP) show an insignificant relationship with CE in the long run, indicating that the KEC hypothesis does not validate in the short run. Overall, the study's findings showed a long-run quantile integration relationship between EI, HC, GDP, SQGDP, and CE in China.
The findings of the Wald test are presented in . The Wald test results confirm that the consistency parameters and the linearity of the speed of adjustment parameters are not accepted, so that it will reject the null hypothesis. In addition, the consistency parameter hypothesis at all the 11 quantiles for the selected variables, i.e., βEI, βHC, βGDP, and βSQGDP are not accepted. These results indicate the presence of asymmetry as the results show that the parameters for all the variables used in this study are varied across qualities. Similarly, the outcomes of short-term dynamics are also presented in . The results rejected the null hypothesis of parameter consistency around the lattice of all the 11 quantiles. For the short-run impact, the Wald test rejects the null hypothesis of parameter consistency and indicates an asymmetric relationship for EI on CE, HC on CE, and GDP on CE. The Wald test failed to reject the null hypothesis of parameter consistency for SQGDP impact on CE, demonstrating the symmetric collective short-run impact of SQGDP on CE.
Table 5. Results of the Wald test for the constancy of parameters.
presented the result of the Granger-causality test. The given probability values at various quantiles confirm the casual link at a particular quantile. For eco-innovation and CE, the findings show two-way causality running from EI to CE and from CE to EI, respectively, across all the given quantiles at a 1 percent level of significance. This finding justified that eco-innovation causes CE, and as a result, CE also causes eco-innovation. Shabani and Shahnazi (Citation2019) support these findings, identifying two-way causality between eco-innovation and CE in China. Similar findings are endorsed by Razzaq et al. (Citation2021) in the BRICS sample. On the contrary, contrarily et al. (2018) find one-way causality running from eco-innovation to CE. Likewise, for the relationship between human capital and CE, it is confirmed that a one-way causality exists between human capital and CE for all quantiles at a 1 percent level of significance. For ECO_GRO, one-way causality was observed running from economic growth to CE. These findings are consistent with Ben Jebli and Hadhri (Citation2018). Whereas the results are contradicted with Zhang and Gao (Citation2016), indicating two-way causality between economic growth and CE. Lastly, two-way causality is observed for eco-innovation and human capital at a 1 percent level of significance. The overall findings of the Granger causality test result show the presence of causality at all the quantiles, respectively.
Table 6. Test results of granger causality in quantile.
5. Conclusion and policy implications
The key objective of this study is to ascertain the long-run and the short-run association among eco-innovation, human capital, and carbon emissions by concurrently determining the presence of EKC hypothesis in China. We applied Quantile ARDL and Granger causality test to estimate dynamic and asymmetric association among model variables using quarterly data from 1988Q1 to 2018Q4. In the long run, the findings indicate that eco-innovation (EI) and human capital (HC) significantly mitigate the level of carbon emissions (CE) at lower (0.05) to medium (0.50) levels of quantiles and medium (0.50) to highest (00.95) level of quantiles respectively. Economic growth (GDP) directs a positive asymmetric impact on CE at the lower (0.50) to highest (0.95) level of quantiles. Likewise, the square of economic growth (SQGDP) exerts a negative influence on CE at lower (0.40) to highest (0.95) level of quantiles, validating the existence of EKC hypothesis in the long run. Likewise, in the short run, EI and HC possess significant emissions mitigating effects mainly at lower (0.40) to higher (0.70) quantiles and higher (0.70) to highest (0.95) level of quantiles, respectively. While GDP indicates a significant positive impact on CE at lower (0.20) to highest (0.95) level of quantiles. Granger causality results confirm the presence of two-way causality between EI, HC, and CE at 1 percent significance level, while a one-way causality runs from HC to CE and GDP to CE at 1 percent level of significance, respectively.
These results imply that human capital development is the foundation of sustainable growth. It encourages innovation and increases overall productivity for firms/countries. Although several human capital development initiatives are already undertaken in China, it is reiterated to embark on another wave to develop human capital by introducing an integrated policy where an innovation-driven model is promoted at the firms and national level. Moreover, the Chinese government should revitalize the national innovation plan to encourage households and firms to adopt sustainable technologies by proposing lower interest rates for energy-efficient acquisitions like electric cars, vitality-efficient housing construction, solar system purchase, installation, etc. Moreover, the government should offer credits at a lower interest rate to private, commercial, and industrial clients for introducing solar energy, as this will quicken the move from non-renewable energy to renewable energy consumption. Policy practitioners ought to plan arrangements to contribute to environmental friendly technologies. In expansion, the government should start new ventures and encourage research and development in environmentally friendly technologies. It is imperative to energize unique and diverse sources of renewable energy at the household and commercial levels to manage the issues of environmental degradation. Lastly, China should be heightening endeavors to intensify efforts to foster innovative human capital. The Chinese central and regional government must do some strategic planning to nurture innovative human capital. In this respect, introducing different training programs for human capital and centering on expanding R&D staff by offering some subsidies and benefits on R&D in different economy divisions can provide assistance to foster innovative human capital.
Disclosure statement
No potential conflict of interest was reported by the authors.
Correction Statement
This article has been republished with minor changes. These changes do not impact the academic content of the article.
References
- Adom, P. K. (2015). Asymmetric impacts of the determinants of energy intensity in Nigeria. Energy Economics, 49, 570–580. https://doi.org/10.1016/j.eneco.2015.03.027
- Ahmad, M., Khan, Z., Rahman, Z. U., Khattak, S. I., & Khan, Z. U. (2021). Can innovation shocks determine CO2 emissions (CO2e) in the OECD economies? A new perspective. Economics of Innovation and New Technology, 30(1), 89–109. https://doi.org/10.1080/10438599.2019.1684643
- Ahmed, Z., & Wang, Z. (2019). Investigating the impact of human capital on the ecological footprint in India: An empirical analysis. Environmental Science and Pollution Research International, 26(26), 26782–26796.
- Alam, M. S., Atif, M., Chien-Chi, C., & Soytaş, U. (2019). Does corporate R&D investment affect firm environmental performance? Evidence from G-6 countries. Energy Economics, 78, 401–411. https://doi.org/10.1016/j.eneco.2018.11.031
- Ali, S., Dogan, E., Chen, F., & Khan, Z. (2021). International trade and environmental performance in top ten‐emitters countries: The role of eco‐innovation and renewable energy consumption. Sustainable Development, 29(2), 378–387. https://doi.org/10.1002/sd.2153
- Alkhathlan, K., & Javid, M. (2013). Energy consumption, carbon emissions and economic growth in Saudi Arabia: An aggregate and disaggregate analysis. Energy Policy, 62, 1525–1532. https://doi.org/10.1016/j.enpol.2013.07.068
- An, H., Razzaq, A., Nawaz, A., Noman, S. M., & Khan, S. A. R. (2021). Nexus between green logistic operations and triple bottom line: evidence from infrastructure-led Chinese outward foreign direct investment in Belt and Road host countries. Environmental Science and Pollution Research, 28, 51022–51045.
- Armstrong, M. (2016). Armstrong's handbook of management and leadership for HR: Developing effective people skills for better leadership and management.
- Au, A. K. M., Altman, Y., & Roussel, J. (2008). Employee training needs and perceived value of training in the Pearl River Delta of China. Journal of European Industrial Training, 32, 19–31.
- Aziz, N., Mihardjo, L. W., Sharif, A., & Jermsittiparsert, K. (2020a). The role of tourism and renewable energy in testing the environmental Kuznets curve in the BRICS countries: Fresh evidence from methods of moments quantile regression. Environmental Science and Pollution Research International, 27(31), 39427–39441.
- Aziz, N., Sharif, A., Raza, A., & Rong, K. (2020b). Revisiting the role of forestry, agriculture, and renewable energy in testing environment Kuznets curve in Pakistan: Evidence from Quantile ARDL approach. Environmental Science and Pollution Research International, 27(9), 10115–10128.
- Bano, S., Zhao, Y., Ahmad, A., Wang, S., & Liu, Y. (2018). Identifying the impacts of human capital on carbon emissions in Pakistan. Journal of Cleaner Production, 183, 1082–1092. https://doi.org/10.1016/j.jclepro.2018.02.008
- Bastola, U., & Sapkota, P. (2015). Relationships among energy consumption, pollution emission, and economic growth in Nepal. Energy, 80, 254–262. https://doi.org/10.1016/j.energy.2014.11.068
- Batool, R., Sharif, A., Islam, T., Zaman, K., Shoukry, A. M., Sharkawy, M. A., Gani, S., Aamir, A., & Hishan, S. S. (2019). Green is clean: The role of ICT in resource management. Environmental Science and Pollution Research International, 26(24), 25341–25358. https://doi.org/10.1007/s11356-019-05748-0
- Becker, G. S. (2009). Human capital: A theoretical and empirical analysis, with special reference to education. University of Chicago press.
- Begum, R. A., Sohag, K., Abdullah, S. M. S., & Jaafar, M. (2015). CO2 emissions, energy consumption, economic and population growth in Malaysia. Renewable and Sustainable Energy Reviews, 41, 594–601. https://doi.org/10.1016/j.rser.2014.07.205
- Ben Jebli, M., & Hadhri, W. (2018). The dynamic causal links between CO2 emissions from transport, real GDP, energy use and international tourism. International Journal of Sustainable Development & World Ecology, 25(6), 568–577. https://doi.org/10.1080/13504509.2018.1434572
- Chen, W., & Lei, Y. (2018). The impacts of renewable energy and technological innovation on environment-energy-growth nexus: New evidence from a panel quantile regression. Renewable Energy., 123, 1–14. https://doi.org/10.1016/j.renene.2018.02.026
- Chengliang, L., Qingbin, G., & Rui, Z. (2017). The dynamic effects of endogenous technological advancement on carbon emissions in China. China: An International Journal, 15(2), 192–207.
- Cheng, Y., Sinha, A., Ghosh, V., Sengupta, T., & Luo, H. (2021). Carbon tax and energy innovation at crossroads of carbon neutrality: Designing a sustainable decarbonization policy. Journal of Environmental Management, 294, 112957.
- Cheng, C. C., Yang, C-l., & Sheu, C. (2014). The link between eco-innovation and business performance: A Taiwanese industry context. Journal of Cleaner Production, 64, 81–90. https://doi.org/10.1016/j.jclepro.2013.09.050
- Chiu, Y.-B., & Lee, C.-C. (2020). Effects of financial development on energy consumption: The role of country risks. Energy Economics, 90, 104833. https://doi.org/10.1016/j.eneco.2020.104833
- Cho, J. S., Kim, T-h., & Shin, Y. (2015). Quantile cointegration in the autoregressive distributed-lag modeling framework. Journal of Econometrics, 188(1), 281–300. https://doi.org/10.1016/j.jeconom.2015.05.003
- Choi, Y., Zhang, N., & Zhou, P. (2012). Efficiency and abatement costs of energy-related CO2 emissions in China: A slacks-based efficiency measure. Applied Energy, 98, 198–208. https://doi.org/10.1016/j.apenergy.2012.03.024
- Cucchiella, F., Gastaldi, M., & Miliacca, M. (2017). The management of greenhouse gas emissions and its effects on firm performance. Journal of Cleaner Production, 167, 1387–1400. https://doi.org/10.1016/j.jclepro.2017.02.170
- Dangelico, R. M., & Pujari, D. (2010). Mainstreaming green product innovation: Why and how companies integrate environmental sustainability. Journal of Business Ethics, 95(3), 471–486. https://doi.org/10.1007/s10551-010-0434-0
- Dasgupta, S., Hettige, H., & Wheeler, D. (2000). What improves environmental compliance? Evidence from Mexican industry. Journal of Environmental Economics and Management, 39(1), 39–66. https://doi.org/10.1006/jeem.1999.1090
- De Jesus, A., Antunes, P., Santos, R., & Mendonça, S. (2018). Eco-innovation in the transition to a circular economy: An analytical literature review. Journal of Cleaner Production, 172, 2999–3018. https://doi.org/10.1016/j.jclepro.2017.11.111
- Desha, C., Robinson, D., & Sproul, A. (2015). Working in partnership to develop engineering capability in energy efficiency. Journal of Cleaner Production, 106, 283–291. https://doi.org/10.1016/j.jclepro.2014.03.099
- Dinda, S., & Coondoo, D. (2006). Income and emission: A panel data-based cointegration analysis. Ecological Economics, 57(2), 167–181. https://doi.org/10.1016/j.ecolecon.2005.03.028
- Ding, Q., Khattak, S. I., & Ahmad, M. (2021). Towards sustainable production and consumption: Assessing the impact of energy productivity and eco-innovation on consumption-based carbon dioxide emissions (CCO2) in G-7 nations. Sustainable Production and Consumption, 27, 254–268. https://doi.org/10.1016/j.spc.2020.11.004
- Erdoğan, S., Yıldırım, S., Yıldırım, D. Ç., & Gedikli, A. (2020). The effects of innovation on sectoral carbon emissions: Evidence from G20 countries. Journal of Environmental Management, 267, 110637.
- Fang, Z., & Chang, Y. (2016). Energy, human capital and economic growth in Asia Pacific countries – Evidence from a panel cointegration and causality analysis. Energy Economics, 56, 177–184. https://doi.org/10.1016/j.eneco.2016.03.020
- Fernández, Y. F., López, M. F., & Blanco, B. O. (2018). Innovation for sustainability: The impact of R&D spending on CO2 emissions. Journal of Cleaner Production, 172, 3459–3467.
- Fethi, S., & Rahuma, A. (2020). The impact of eco-innovation on CO2 emission reductions: Evidence from selected petroleum companies. Structural Change and Economic Dynamics, 53, 108–115. https://doi.org/10.1016/j.strueco.2020.01.008
- Frondel, M., Horbach, J., & Rennings, K. (2007). End‐of‐pipe or cleaner production? An empirical comparison of environmental innovation decisions across OECD countries. Business Strategy and the Environment, 16(8), 571–584. https://doi.org/10.1002/bse.496
- Fussler, C., & James, P. (1996). Driving eco-innovation: A breakthrough discipline for innovation and sustainability. Financial Times/Prentice Hall.
- Garcia-Granero, E. M., Piedra-Munoz, L., & Galdeano-Gomez, E. (2018). Eco-innovation measurement: A review of firm performance indicators. Journal of Cleaner Production, 191, 304–317.
- Garrone, P., & Grilli, L. (2010). Is there a relationship between public expenditures in energy R&D and carbon emissions per GDP? An empirical investigation. Energy Policy, 38(10), 5600–5613. https://doi.org/10.1016/j.enpol.2010.04.057
- Godil, D. I., Sarwat, S., Sharif, A., & Jermsittiparsert, K. (2020). How oil prices, gold prices, uncertainty and risk impact Islamic and conventional stocks? Empirical evidence from QARDL technique. Resources Policy, 66, 101638. https://doi.org/10.1016/j.resourpol.2020.101638
- Godil, D. I., Sharif, A., Agha, H., & Jermsittiparsert, K. (2020). The dynamic nonlinear influence of ICT, financial development, and institutional quality on CO2 emission in Pakistan: New insights from QARDL approach. Environmental Science and Pollution Research International, 27(19), 24190–24200.
- Grossman, G. M., & Krueger, A. B. (1995). Economic growth and the environment. The Quarterly Journal of Economics, 110(2), 353–377. https://doi.org/10.2307/2118443
- Gu, G., & Wang, Z. (2018). Research on global carbon abatement driven by R&D investment in the context of INDCs. Energy, 148, 662–675. https://doi.org/10.1016/j.energy.2018.01.142
- Hassan, S. T., Baloch, M. A., Mahmood, N., & Zhang, J. (2019). Linking economic growth and ecological footprint through human capital and biocapacity. Sustainable Cities and Society, 47, 101516.
- Hojnik, J., & Ruzzier, M. (2016). The driving forces of process eco-innovation and its impact on performance: Insights from Slovenia. Journal of Cleaner Production, 133, 812–825. https://doi.org/10.1016/j.jclepro.2016.06.002
- Huang, C., Zhang, X., & Liu, K. (2021). Effects of human capital structural evolution on carbon emissions intensity in China: A dual perspective of spatial heterogeneity and nonlinear linkages. Renewable and Sustainable Energy Reviews, 135, 110258. https://doi.org/10.1016/j.rser.2020.110258
- Hussain, M., Javaid, M. I., & Drake, P. R. (2012). An econometric study of carbon dioxide (CO2) emissions, energy consumption, and economic growth of Pakistan. International Journal of Energy Sector Management, 6(4), 518–533. https://doi.org/10.1108/17506221211282019
- Iqbal, M. A., Majeed, M. T., & Luni, T. (2021). Human capital, trade openness and CO2 emissions: Evidence from heterogeneous income groups. Pakistan Journal of Commerce and Social Sciences, 15(3), 559–585.
- Ji, X., Umar, M., Ali, S., Ali, W., Tang, K., & Khan, Z. (2021). Does fiscal decentralization and eco-innovation promote sustainable environment? A case study of selected fiscally decentralized countries. Sustainable Development, 29(1), 79–88.
- Jiao, J., Yang, Y., & Bai, Y. (2018). The impact of inter-industry R&D technology spillover on carbon emission in China. Natural Hazards, 91(3), 913–929. https://doi.org/10.1007/s11069-017-3161-3
- Kim, T., & Lee, J. (2011). Technological change, human capital structure, and multiple growth paths. The Japanese Economic Review, 62(3), 305–330.
- Kim, T., & White, H. (2003). Estimation, inference, and specification testing for possibly misspecified quantile regression. Emerald Group Publishing Limited.
- Kuznets, S. (1955). Economic growth and income inequality. The American Economic Review, 45(1), 1–28.
- Kwon, D. (2009). Human capital and its measurement. Paper presented at the The 3rd OECD World Forum on ‘Statistics, Knowledge and Policy’ Charting Progress, Building Visions, Improving Life.
- Lapinskienė, G., Peleckis, K., & Nedelko, Z. (2017). Testing environmental Kuznets curve hypothesis: The role of enterprise’s sustainability and other factors on GHG in European countries. Journal of Business Economics and Management, 18(1), 54–67. https://doi.org/10.3846/16111699.2016.1249401
- Lederman, D., Lesniak, J. T., Feenstra, R. C., Inklaar, R., & Timmer, M. P. (2017). The next generation of the Penn world table. Total factor productivity at constant national prices for Kenya. American Economic Review. www.ggdc.net/pwt
- Lee, K.-H., & Min, B. (2015). Green R&D for eco-innovation and its impact on carbon emissions and firm performance. Journal of Cleaner Production, 108, 534–542. https://doi.org/10.1016/j.jclepro.2015.05.114
- Li, P., & Ouyang, Y. (2019). The dynamic impacts of financial development and human capital on CO2 emission intensity in China: An ARDL approach. Journal of Business Economics and Management, 20(5), 939–957. https://doi.org/10.3846/jbem.2019.10509
- Lin, X., Zhao, Y., Ahmad, M., Ahmed, Z., Rjoub, H., & Adebayo, T. S. (2021). Linking innovative human capital, economic growth, and CO2 emissions: An empirical study based on Chinese provincial panel data. International Journal of Environmental Research and Public Health, 18(16), 8503. https://doi.org/10.3390/ijerph18168503
- Lingyan, M., Zhao, Z., Malik, H. A., Razzaq, A., An, H., & Hassan, M. (2021). Asymmetric impact of fiscal decentralization and environmental innovation on carbon emissions: Evidence from highly decentralized countries. Energy & Environment, https://doi.org/10.1177/0958305X211018453
- Lopez-Gamero, M. D., Molina-Azorín, J. F., & Claver-Cortés, E. (2010). The potential of environmental regulation to change managerial perception, environmental management, competitiveness and financial performance. Journal of Cleaner Production, 18(10–11), 963–974. https://doi.org/10.1016/j.jclepro.2010.02.015
- Mehrara, M., Rezaei, S., & Razi, D. H. (2015). Determinants of renewable energy consumption among ECO countries; based on Bayesian model averaging and weighted-average least square. International Letters of Social and Humanistic Sciences, 54, 96–109. https://doi.org/10.18052/www.scipress.com/ILSHS.54.96
- Mensah, C. N., Long, X., Boamah, K. B., Bediako, I. A., Dauda, L., & Salman, M. (2018). The effect of innovation on CO2 emissions of OCED countries from 1990 to 2014. Environmental Science and Pollution Research International, 25(29), 29678–29698. https://doi.org/10.1007/s11356-018-2968-0
- Mishra, S., Sharif, A., Khuntia, S., Meo, M. S., & Khan, S. A. R. (2019). Does oil prices impede Islamic stock indices? Fresh insights from wavelet-based quantile-on-quantile approach. Resources Policy, 62, 292–304. https://doi.org/10.1016/j.resourpol.2019.04.005
- Mishra, S., Sinha, A., Sharif, A., & Suki, N. M. (2020). Dynamic linkages between tourism, transportation, growth and carbon emission in the USA: Evidence from partial and multiple wavelet coherence. Current Issues in Tourism, 23(21), 2733–2755. https://doi.org/10.1080/13683500.2019.1667965
- Nasiritousi, N. (2017). Fossil fuel emitters and climate change: Unpacking the governance activities of large oil and gas companies. Environmental Politics, 26(4), 621–647. https://doi.org/10.1080/09644016.2017.1320832
- Nguyen, N. T. K., & Le, M. B. (2018). CO2 emissions and economic growth in Vietnam: An ARDL bound testing approach. Asian Journal of Economic Modelling, 6(1), 47–55. https://doi.org/10.18488/journal.8.2018.61.47.55
- Ozcan, B., & Apergis, N. (2018). The impact of internet use on air pollution: Evidence from emerging countries. Environmental Science and Pollution Research International, 25(5), 4174–4189.
- Ozturk, I., & Acaravci, A. (2013). The long-run and causal analysis of energy, growth, openness and financial development on carbon emissions in Turkey. Energy Economics, 36, 262–267. https://doi.org/10.1016/j.eneco.2012.08.025
- Pablo-Romero, M. d P., & Sánchez-Braza, A. (2015). Productive energy use and economic growth: Energy, physical and human capital relationships. Energy Economics, 49, 420–429. https://doi.org/10.1016/j.eneco.2015.03.010
- Panayotou, T. (2016). 14. Economic growth and the environment. New York University Press.
- Porter, M., & Van der Linde, C. (1995). Green and competitive: Ending the stalemate. The Dynamics of the Eco-Efficient Economy: Environmental Regulation and Competitive Advantage, 33, 120–134.
- Ramanathan, R., He, Q., Black, A., Ghobadian, A., & Gallear, D. (2017). Environmental regulations, innovation and firm performance: A revisit of the Porter hypothesis. Journal of Cleaner Production, 155, 79–92. https://doi.org/10.1016/j.jclepro.2016.08.116
- Razzaq, A., Ajaz, T., Li, J. C., Irfan, M., & Suksatan, W. (2021b). Investigating the asymmetric linkages between infrastructure development, green innovation, and consumption-based material footprint: Novel empirical estimations from highly resource-consuming economies. Resources Policy, 74, 102302. https://doi.org/10.1016/j.resourpol.2021.102302
- Razzaq, A., Sharif, A., Ahmad, P., & Jermsittiparsert, K. (2021). Asymmetric role of tourism development and technology innovation on carbon dioxide emission reduction in the Chinese economy: Fresh insights from QARDL approach. Sustainable Development, 29(1), 176–193. https://doi.org/10.1002/sd.2139
- Razzaq, A., Wang, Y., Chupradit, S., Suksatan, W., & Shahzad, F. (2021a). Asymmetric inter-linkages between green technology innovation and consumption-based carbon emissions in BRICS countries using quantile-on-quantile framework. Technology in Society, 66, 101656. https://doi.org/10.1016/j.techsoc.2021.101656
- Reid, A., & Miedzinski, M. (2008). Eco-innovation. Final report for sectoral innovation watch. Europe Innova. Technopolis Group.
- Rennings, K. (2000). Redefining innovation – Eco-innovation research and the contribution from ecological economics. Ecological Economics, 32(2), 319–332. https://doi.org/10.1016/S0921-8009(99)00112-3
- Rist, G. (2019). The history of development: From western origins to global faith. Zed Books Ltd.
- Romer, P. M. (1990). Endogenous technological change. Journal of Political Economy, 98(5, Part 2), S71–S102. https://doi.org/10.1086/261725
- Saleem, N., & Shujah-Ur-Rahman, J. Z. (2019). The impact of human capital and biocapacity on environment: Environmental quality measure through ecological footprint and greenhouse gases. Journal of Pollution Effects and Control, 7(2), 1–13.
- Schultz, T. W. (1961). Investment in human capital. The American Economic Review, 51(1), 1–17.
- Serrat, O. (2009). Harnessing creativity and innovation in the workplace. In Knowledge solutions (Vol. 61). Springer.
- Shabani, Z. D., & Shahnazi, R. (2019). Energy consumption, carbon dioxide emissions, information and communications technology, and gross domestic product in Iranian economic sectors: A panel causality analysis. Energy, 169, 1064–1078. https://doi.org/10.1016/j.energy.2018.11.062
- Shafik, N., & Bandyopadhyay, S. (1992). Economic growth and environmental quality: Time-series and cross-country evidence (Vol. 904): World Bank Publications.
- Shahbaz, M., Balsalobre-Lorente, D., & Sinha, A. (2019a). Foreign direct investment–CO2 emissions nexus in Middle East and North African countries: Importance of biomass energy consumption. Journal of Cleaner Production, 217, 603–614. https://doi.org/10.1016/j.jclepro.2019.01.282
- Shahbaz, M., Gozgor, G., & Hammoudeh, S. (2019b). Human capital and export diversification as new determinants of energy demand in the United States. Energy Economics, 78, 335–349. https://doi.org/10.1016/j.eneco.2018.11.016
- Shahbaz, M., Hye, Q. M. A., Tiwari, A. K., & Leitão, N. C. (2013). Economic growth, energy consumption, financial development, international trade and CO2 emissions in Indonesia. Renewable and Sustainable Energy Reviews, 25, 109–121. https://doi.org/10.1016/j.rser.2013.04.009
- Shahbaz, M., Lahiani, A., Abosedra, S., & Hammoudeh, S. (2018a). The role of globalization in energy consumption: A quantile cointegrating regression approach. Energy Economics, 71, 161–170. https://doi.org/10.1016/j.eneco.2018.02.009
- Shahbaz, M., & Sinha, A. (2019). Environmental Kuznets curve for CO2 emissions: A literature survey. Journal of Economic Studies, 46(1), 106–168. https://doi.org/10.1108/JES-09-2017-0249
- Shahbaz, M., Zakaria, M., Shahzad, S. J. H., & Mahalik, M. K. (2018b). The energy consumption and economic growth nexus in top ten energy-consuming countries: Fresh evidence from using the quantile-on-quantile approach. Energy Economics, 71, 282–301. https://doi.org/10.1016/j.eneco.2018.02.023
- Sharif, A., Baris-Tuzemen, O., Uzuner, G., Ozturk, I., & Sinha, A. (2020a). Revisiting the role of renewable and non-renewable energy consumption on Turkey’s ecological footprint: Evidence from quantile ARDL approach. Sustainable Cities and Society, 57, 102138. https://doi.org/10.1016/j.scs.2020.102138
- Sharif, A., Mishra, S., Sinha, A., Jiao, Z., Shahbaz, M., & Afshan, S. (2020b). The renewable energy consumption-environmental degradation nexus in Top-10 polluted countries: Fresh insights from quantile-on-quantile regression approach. Renewable Energy., 150, 670–690. https://doi.org/10.1016/j.renene.2019.12.149
- Sharif, A., Raza, S. A., Ozturk, I., & Afshan, S. (2019). The dynamic relationship of renewable and nonrenewable energy consumption with carbon emission: A global study with the application of heterogeneous panel estimations. Renewable Energy., 133, 685–691. https://doi.org/10.1016/j.renene.2018.10.052
- Sharma, R., Sinha, A., & Kautish, P. (2021). Does financial development reinforce environmental footprints? Evidence from emerging Asian countries. Environmental Science and Pollution Research International, 28(8), 9067–9083.
- Shoaib, H. M., Rafique, M. Z., Nadeem, A. M., & Huang, S. (2020). Impact of financial development on CO 2 emissions: A comparative analysis of developing countries (D 8) and developed countries (G 8). Environmental Science and Pollution Research, 27(11), 12461–12475. https://doi.org/10.1007/s11356-019-06680-z
- Sianesi, B., & Reenen, J. V. (2003). The returns to education: Macroeconomics. Journal of Economic Surveys, 17(2), 157–200. https://doi.org/10.1111/1467-6419.00192
- Sinha, A., Mishra, S., Sharif, A., & Yarovaya, L. (2021). Does green financing help to improve environmental & social responsibility? Designing SDG framework through advanced quantile modelling. Journal of Environmental Management, 292, 112751. https://doi.org/10.1016/j.jenvman.2021.112751
- Sinha, A., & Rastogi, S. K. (2017). Collaboration between central and state government and environmental quality: Evidences from Indian cities. Atmospheric Pollution Research, 8(2), 285–296. https://doi.org/10.1016/j.apr.2016.09.007
- Sinha, A., Sengupta, T., & Alvarado, R. (2020c). Interplay between technological innovation and environmental quality: Formulating the SDG policies for next 11 economies. Journal of Cleaner Production, 242, 118549. https://doi.org/10.1016/j.jclepro.2019.118549
- Sinha, A., Sengupta, T., & Saha, T. (2020b). Technology policy and environmental quality at crossroads: Designing SDG policies for select Asia Pacific countries. Technological Forecasting and Social Change, 161, 120317. https://doi.org/10.1016/j.techfore.2020.120317
- Sinha, A., & Shahbaz, M. (2018). Estimation of environmental Kuznets curve for CO2 emission: Role of renewable energy generation in India. Renewable Energy., 119, 703–711. https://doi.org/10.1016/j.renene.2017.12.058
- Sinha, A., Shah, M. I., Sengupta, T., & Jiao, Z. (2020a). Analyzing technology-emissions association in Top-10 polluted MENA countries: How to ascertain sustainable development by quantile modeling approach. Journal of Environmental Management, 267, 110602. https://doi.org/10.1016/j.jenvman.2020.110602
- Stern, D. I. (2004). The rise and fall of the environmental Kuznets curve. World Development, 32(8), 1419–1439. https://doi.org/10.1016/j.worlddev.2004.03.004
- Sun, Y., Duru, O. A., Razzaq, A., & Dinca, M. S. (2021). The asymmetric effect eco-innovation and tourism towards carbon neutrality target in Turkey. Journal of Environmental Management, 299, 113653.
- Sun, Y., Yesilada, F., Andlib, Z., & Ajaz, T. (2021). The role of eco-innovation and globalization towards carbon neutrality in the USA. Journal of Environmental Management, 299, 113568.
- Tamazian, A., Chousa, J. P., & Vadlamannati, K. C. (2009). Does higher economic and financial development lead to environmental degradation: Evidence from BRIC countries. Energy Policy, 37(1), 246–253. https://doi.org/10.1016/j.enpol.2008.08.025
- Tan, Z., Li, L., Wang, J., & Wang, J. (2011). Examining the driving forces for improving China’s CO2 emission intensity using the decomposing method. Applied Energy, 88(12), 4496–4504. https://doi.org/10.1016/j.apenergy.2011.05.042
- Tian, Y., Chen, W., & Zhu, S. (2017). Does financial macroenvironment impact on carbon intensity: Evidence from ARDL-ECM model in China. Natural Hazards, 88(2), 759–777. https://doi.org/10.1007/s11069-017-2925-0
- Troster, V., Shahbaz, M., & Uddin, G. S. (2018). Renewable energy, oil prices, and economic activity: A Granger-causality in quantiles analysis. Energy Economics, 70, 440–452. https://doi.org/10.1016/j.eneco.2018.01.029
- Wang, L., Chang, H.-L., Rizvi, S. K. A., & Sari, A. (2020). Are eco-innovation and export diversification mutually exclusive to control carbon emissions in G-7 countries? Journal of Environmental Management, 270, 110829.
- Wang, J., & Xu, Y. (2021). Internet usage, human capital and CO2 emissions: A global perspective. Sustainability, 13(15), 8268. https://doi.org/10.3390/su13158268
- Xiao, Z. (2009). Quantile cointegrating regression. Journal of Econometrics, 150(2), 248–260. https://doi.org/10.1016/j.jeconom.2008.12.005
- Xuefeng, Z., Razzaq, A., Gokmenoglu, K. K., & Rehman, F. U. (2021). Time varying interdependency between COVID-19, tourism market, oil prices, and sustainable climate in United States: Evidence from advance wavelet coherence approach. Economic Research-Ekonomska Istraživanja, 1–23. https://doi.org/10.1080/1331677X.2021.1992642
- Yang, L., & Li, Z. (2017). Technology advance and the carbon dioxide emission in China–Empirical research based on the rebound effect. Energy Policy, 101, 150–161. https://doi.org/10.1016/j.enpol.2016.11.020
- Yao, Y., Ivanovski, K., Inekwe, J., & Smyth, R. (2019). Human capital and energy consumption: Evidence from OECD countries. Energy Economics, 84, 104534. https://doi.org/10.1016/j.eneco.2019.104534
- Yao, Y., Ivanovski, K., Inekwe, J., & Smyth, R. (2020). Human capital and CO2 emissions in the long run. Energy Economics, 91, 104907–104914. https://doi.org/10.1016/j.eneco.2020.104907
- Yii, K.-J., & Geetha, C. (2017). The nexus between technology innovation and CO2 emissions in Malaysia: Evidence from granger causality test. Energy Procedia., 105, 3118–3124. https://doi.org/10.1016/j.egypro.2017.03.654
- Yuan, B., & Zhang, K. (2017). Can environmental regulation promote industrial innovation and productivity? Based on the strong and weak Porter hypothesis. Chinese Journal of Population Resources and Environment, 15(4), 322–336. https://doi.org/10.1080/10042857.2017.1416042
- Yurdakul, M., & Kazan, H. (2020). Effects of Eco-Innovation on Economic and Environmental Performance: Evidence from Turkey’s Manufacturing Companies. Sustainability, 12(8), 3167. https://doi.org/10.3390/su12083167
- Zhang, H., Razzaq, A., Pelit, I., & Irmak, E. (2021). Does freight and passenger transportation industries are sustainable in BRICS countries? Evidence from advance panel estimations. Economic Research-Ekonomska Istraživanja. https://doi.org/10.1080/1331677X.2021.2002708
- Zhang, L., & Gao, J. (2016). Exploring the effects of international tourism on China's economic growth, energy consumption and environmental pollution: Evidence from a regional panel analysis. Renewable and Sustainable Energy Reviews, 53, 225–234. https://doi.org/10.1016/j.rser.2015.08.040
- Zhang, Y.-J., Peng, Y.-L., Ma, C.-Q., & Shen, B. (2017). Can environmental innovation facilitate carbon emissions reduction? Evidence from China. Energy Policy, 100, 18–28. https://doi.org/10.1016/j.enpol.2016.10.005
- Zhuang, Y., Yang, S., Razzaq, A., & Khan, Z. (2021). Environmental impact of infrastructure-led Chinese outward FDI, tourism development and technology innovation: A regional country analysis. Journal of Environmental Planning and Management, 1–33.
- Zivot, E., & Andrews, D. W. K. (2002). Further evidence on the great crash, the oil-price shock, and the unit-root hypothesis. Journal of Business & Economic Statistics, 20(1), 25–44. https://doi.org/10.1198/073500102753410372