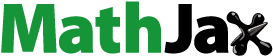
Abstract
This study evaluates the relevancy of economic globalization, financial development, and clean energy, in strengthening the environmental sustainability of the next 11 economies over a time period pertaining to 1995–2018. In order to achieve the objective of this study, the advanced panel estimation techniques of unit root testing, and the cointegration analysis have been applied due to the presence of the cross-sectional-dependence and heterogeneity of the slope parameters in the panel data. The long-run output coefficients have been estimated through the Cross-Sectional Autoregressive Distributive Lag Model (CS-ARDL). Moreover, the causality test for a heterogeneous panel has also been employed in order to determine the causal relationships among the variables that are under study. Our empirical findings of these tests indicate that financial development and economic globalization tend to contribute to the deterioration of environmental quality, but clean energy is productive for its improvement. The bi-directional causal relationship is recognized to exist between CO2 emission and all the variables. Based on these findings, the study recommends adopting economic growth policies that are aligned with the defined environmental regulations, thus promoting the use of more clean energy resources. These include resources such as renewable energy and incorporating the environmental welfare goals into financial development plans in N11 economies.
1. Introduction
Over the last few decades, environmental issues have received a great amount of attention (Anwar et al., Citation2021; Bibi et al., Citation2021; Ji et al., Citation2021; Umar, Ji, Kirikkaleli, & Xu, Citation2020). In this regard, ensured economic growth, and the protection of the environment simultaneously have become a major development priority around the world (Umar, Ji, Kirikkaleli, Shahbaz, et al., Citation2020; K.-H. Wang et al., Citation2021; Xu et al., Citation2020; J. Zhang et al., Citation2021). The Next 11 (N11) countries are no exemption in this regard. The N11 countries, and the BRICs countries are among the fastest-growing economies of the worldFootnote1. Although N11 is not qualified to be a competitor of the BRIC’s anytime in the short run, their GDP is expected to reach to the G7 levels by the year 2050 (Goldman, Citation2007). It is noteworthy that the N11 countries accounted for 9 percent of the global GDP in 2016 (Group, Citation2016). Hence, the N11 countries have been anticipated to experience a significant level of economic growth, which can further harm the environment in these very countries (Nathaniel, Citation2021). Moreover, the extant of industrialization in the N11 countries is responsible for deteriorating the level of environmental degradation (Pata & Yilanci, Citation2021). Therefore, these countries need to match their economic growth plans with the environmental welfare goals (Naqvi et al., Citation2021; Su, Khan, et al., Citation2021; Su, Yuan, et al., Citation2021). Hence, understanding and addressing the causal factors of environmental degradation is vital for the N11 nations (Nathaniel, Citation2021).
There are several variables that are considered to be responsible for the environmental deterioration in general. These variables need to be taken into close account by the N11 economies during the development process. One such variable from this list is that of financial development. For numerous reasons, the expanding part of financial development, in increasing the carbon dioxide emissions, has been amplified in an economic entity with the continuously deepening financial sectors. First, foreign direct investment (FDI) is attracted through financial development, in order to boost the economic production and CO2 emissions (Frankel & Romer, Citation1999). Second, successful and effective financial development appears to be favourable to the consumers’ accessibility to loans, thus making it simpler for them to purchase expensive items such as refrigerators, automobiles, housing property, washing machines, air conditioners, and so on, and therefore contribute towards the generation of more CO2 (Sadorsky, Citation2010). Furthermore, the development of the stock market assists publicly traded companies in lowering the costs of financing, expanding financing channels, and optimizing structures of assets and liabilities. This allows them to gain access to investments in advanced and new projects, and also to buy more installations, thereby increasing the consumption of energy and carbon emissions (Su et al., Citation2020; Taghizadeh-Hesary et al., Citation2021; Q.-S. Wang et al., Citation2021; Yan et al., Citation2021).
However, several authors disagree with the points that have been presented above. For instance, (Tamazian et al., Citation2009) argue that financial development helps the firms to promote technology advancements, adopt new strategies for efficiency in energy consumption, and promote development with low carbon emissions. As a result, the intensity of CO2 emissions have the potential to experience colossal reductions. Furthermore, Claessens and Feijen (Citation2007) also discovered that firms with improved governance are more likely to consider development with reduced carbon. Hence, financial development can enhance enterprise functioning, thus resulting in lower consumption of energy and CO2 emissions (Y.-J. Zhang, Citation2011).
Similarly, energy is another factor considered as a key driver of the growth of an economy, in both advanced, as well as emerging/developing countries (Taghizadeh-Hesary & Yoshino, Citation2020; Umar, Ji, Kirikkaleli, et al., Citation2021; Umar, Ji, Mirza, et al., Citation2021). The energy demand is considerably high for it to realistically achieve sustainable growth, which poses many environmental stability threats (e.g. deforestation, air pollution, global warming, and water contamination). However, unfortunately, in order to meet this demand, energy is still mostly produced from traditional energy sources, including gas, coal, and oil. These traditional fuels produce massive amounts of GHG (greenhouse gases), which are the primary contributing factors towards global warming and climate change in the recent decades (Cai et al., Citation2018; Nawaz et al., Citation2021). Environmental policymakers frequently expect that the expansion of clean energy sources, such as solar energy and renewable energy (hydro, solar, biomass, geothermal, and wind), will help to decouple economic growth from carbon emissions (Baloch et al., Citation2021; Mohsin et al., Citation2021; Piłatowska & Geise, Citation2021; Sun et al., Citation2021; Razzaq et al. Citation2021b). Hence, it can be asserted that clean energy can potentially reduce energy dependence, and also improve energy security, while also improving the environmental quality. Moreover, clean energy provides a solution to ecological concerns, such as global warming, acid rain, biodiversity loss, climate change, air, and water pollution, by reducing carbon and other pollutant gas emissions (Apergis & Payne, Citation2012; Georgescu et al., Citation2011; Nawaz et al., Citation2021; Ullah et al., Citation2020; B. Zhang et al., Citation2017). Furthermore, clean energy can also help to promote long-term economic growth by enhancing agricultural, industrial, and service expansion. As a result, clean energy can generate employment opportunities, and also raise the standards of living by improving economic conditions, and reducing poverty in developing countries (Z. Wang, Citation2019). As a result, it is not surprising that the mounting prices of fossil fuels, macro-level instability, energy security, and emissions have elevated the importance of clean energy in larger scaled energy issues and environmental discussions (Usman et al., Citation2020; Zhuang et al., Citation2021).
Regardless of the increasing level of globalization, the importance of the sustainability of the environment, particularly the influence of economic globalization on global climate change, has been vastly underestimated. Through capital movements, open trading, and many other channels, economic globalization improved the well-being of many countries and unveiled a more contented approach to life (Gozgor et al., Citation2020). Many scholars have presented diverse perspectives on economic globalization’s role in climatic changes, divided into two groups. Some belief in the ‘Pollution Haven Hypothesis’, which claims that industrialized countries with strict environmental rules typically move polluting or energy-demanding processes of production to countries having less stringent environmental controls (Destek & Okumus, Citation2019; Copeland & Taylor, Citation2004). FDI and commercial flows, according to proponents, are abandoning environment quality in emerging economies (Ghosh, Citation2010). Indeed, some countries are being compelled to compromise their environmental standards to magnetize more international investment due to the demands of expanding economic globalization. Economic activities and more energy inputs in many emerging countries contribute to environmental damage (Gozgor & Can, Citation2017). Excessive consumption of resources is incompatible with the aim of sustainable economic development.
In contrast, the other group supports that industry, money movements, technology innovation, and globalization support the bearer of ‘green growth’ and are key factors for dealing with pollution and climate changes (Chien, Kamran, et al., Citation2021; Chien, Sadiq, et al., Citation2021; Shahbaz et al., Citation2016). Countries having fewer environmental restrictions might gain access to modern environment protection technology through the cross-regional movement of the factors of production, FDI, and patent transfers because of the multinational firms’ practices and experiences that are helpful for the betterment of the environmental quality. In order to address this contentious issue, the study identifies economic globalization as one of the vital elements influencing emissions in N11 countries in order to substantiate this claim and make policy recommendations (Yang et al., Citation2021).
The primary goal of our work is to examine whether the development of the financial sector, economic growth, clean energy, and economic globalization help strengthen environmental prevention over the period 1995–2018. The study’s focus group is N11 countries, including Egypt, Bangladesh, Indonesia, Mexico, Iran, Philippines, South Korea, Pakistan, Turkey, Nigeria, and Vietnam, as the included variables are understudied in these economies. The study is going to add to the already existing empirical researches in two ways. (i) like Ampofo et al. (Citation2021) and Nathaniel (Citation2021), this study also analyzes the environmental impacts of economic growth and financial development in N11 economies but also takes clean energy and economic globalization into consideration as these two variables also have significant influences on environmental quality (ii) Ampofo et al. (Citation2021) disregard the structure breaks in data that we are considering in our analysis. Bai and Carrion-I-Silvestre (Citation2009) approach is applied in our study to overcome this issue. In addition, we employed the Westerlund and Edgerton (Citation2008) as well as the Banerjee and Carrion-i-Silvestre (Citation2017) techniques, where the earlier takes the breaks at the structure with cointegration into consideration. The empirical results of our study imply that financial development, economic growth, and economic globalization decline the environmental quality. However, clean energy helps to improve it. Nonetheless, in the long run, the coefficients of the variables are greater than in the short run, indicating that these variables’ environmental effects transmit more significantly in the long term. Finally, the DH panel causality analysis indicates that bi-directional causality existed between the explanatory variables and CO2 emission.
The remaining study is ordered as follows. Reviews of the existing literature are given in section 2. Section 3 describes and data and specification of the model and estimation strategy. The empirical findings and their discussion is provided in section 4. Finally, section 5 provides the conclusion and policy recommendations of the study
2. Literature review
As a result of rapid economic development, globalization, and financial development, CO2 emissions have become a significant issue globally, as globalization helps fulfil the growing demand for goods and services across borders. Environmental pollution is the subject matter of a large number of researches. For instance, Kirikkaleli and Adebayo (Citation2021) explored the influence of financial development, technological innovation, renewable energy, and growth on CO2 emission globally over the period of 185–2017. Using the econometric techniques of Fully Modified Least Square, dynamic OLS, Canonical Cointegrating Regression, and Frequency Domain Causality Tests, the authors confirmed the presence of the long-term relationship among the variables. It was also found that renewable energy consumption and the development of the financial sector negatively affected CO2 emission, whereas economic growth influenced CO2 emission positively in the global framework (Kirikkaleli & Adebayo, Citation2021).
Rafique et al. (Citation2021) inspected the nexus between financial development, economic growth, trade liberalization, use of energy, and carbon dioxide emissions from 1980 to 2016 in Bangladesh. Using the ARDL bound technique, the results showed that energy usage significantly impacted carbon dioxide emissions. Economic growth was found to have a significant effect only in the long run and had no significant effect in the short run. The effect of openness of trade and financial development was negative but insignificant in both time periods. The study suggested adopting strategies to substitute energy sources that do not release significant carbon emissions (Rafique et al., Citation2021).
Likewise, Özsoy and Ozpolat (n.d.) tried to estimate how clean energy, GDP per capita, and technological development affected per capita CO2 emission in Turkey for the 1990–2014 period. For this purpose, the study used ARDL bound test on parameters and VECM Granger causality analysis. ARDL results indicated that technology development was affecting carbon emissions significantly and positively, whereas cleaner energy had an adverse impact on emissions. Clean energy was proved to be more efficient than technology development in reducing CO2 emission in the long run. Both clean energy and technological development had a negative impact on carbon emissions in the short run. Moreover, the incidence of the EKC curve was confirmed by short and long-run results in Turkey (Özsoy & Ozpolat, n.d.).
Murshed et al. (Citation2021) evaluated the influences of clean energy, urbanization, trade liberalization, and GDP on carbon footprint in Bangladesh over the 1975–2016 period, and FMOLS and Vector Autoregressive model results indicated that consumption of total energy, fossil fuel energy, and natural gas increased the carbon footprint. In contrast, the consumption of non-fossil fuel and hydroelectricity decreased the carbon footprint. International trade and economic growth were evidenced to be a source of an increase in the carbon footprints. The study’s findings suggested that a transition to clean energy could be a solution to the aggravating environmental problems of the country (Murshed et al., Citation2021). Kalayci (Citation2019) investigated how trade liberalization and economic globalization affected CO2 emissions in NAFTA countries using annual data over the 1990–2015 period. The findings revealed a positive relationship between trade liberalization economic growth and economic globalization and emissions. The validation of the Environmental Kuznets Curve was also tested for the targeted countries, and the researchers identified a positive relationship of linear and squared GDP with CO2 emissions (Kalayci, Citation2019).
You and Lv (Citation2018) analyzed how economic globalization affected carbon dioxide emissions for a panel of eighty-three countries from 1985 to 2013. The estimation results of the spatial panel estimation technique proved the presence of spatial correlations in CO2 emissions among countries. It was found that economic globalization had a considerable adverse impact on CO2 emissions despite the direct positive effect, implying a large and significant total effect. Finally, a piece of strong evidence was found for the EKC relationship between income and CO2 emissions (You & Lv, Citation2018).
A study by X. Yao et al. (Citation2021) compared the BRICS and the Next 11 economies from 1995 to 2014 in energy efficiency, financial development, corruption control, and ecological footprint. The GMM results revealed that if corruption were managed, financial development, resource rents, and technology innovations would enhance energy efficiency and environment stability by decreasing ecological footprints. The feedback hypothesis linking ecological footprint, energy efficiency, corruption control, financial development, technical innovation, natural resource rent, trade was highlighted by the result of causality, and these findings are confirmed by some other studies in the literature as well (Li et al., Citation2021; Shair et al., Citation2021; X. Yao et al., Citation2021).
According to the findings, Yang et al. (Citation2021) studied the effect of population aging and economic globalization on CO2 emissions in OECD countries, and economic globalization and ageing reduced long-term CO2 emissions. The existence of the EKC in countries was verified by the inverted U-shaped link between growth and environmental degradation. The study also found a unidirectional causal relationship between globalization and the aging population to CO2 emissions (Yang et al., Citation2021).
Over the period 1972–2013, Ampofo et al. (Citation2021) examined the causal relationships and asymmetric cointegration between carbon emissions, economic growth, and energy consumption in N11 countries. Applying the NARDL bounds approach and Non-Pragmatic Granger Causality tests affirmed that non-linear cointegration existed among all variables in Turkey, Iran, Bangladesh, and Vietnam. It was indicated that a boom in economic growth increased the CO2 emission significantly in Turkey but declined it in Vietnam. Furthermore, an increase in energy consumption raised CO2 emissions in Iran, Bangladesh, and Turkey but reduced emissions in Vietnam. Bidirectional causality existed between CO2 emission and economic growth in Turkey and Bangladesh, and a unidirectional causality between growth and CO2 emission in South Korea and Egypt (Ampofo et al., Citation2021). Likewise, Nawaz et al. (Citation2021) found a positive impact of energy consumption and economic growth on carbon emissions using quantile regression estimation in the context of BRICS and OECD regions.
Far Eastern countries (Anwar et al., Citation2020) investigated the primary factors influencing CO2 emissions from 1980 to 2017. Applying the Fixed Effect model, the study found that economic growth, urbanization, and trade liberalization determined CO2 emission significantly in these countries (Anwar et al., Citation2020). Nathaniel (Citation2021) probed into globalization, financial development, natural resources, human well-being, and ecological footprint linkage in N11 countries by applying the latest estimation techniques well-suited with cross-sectional dependence and endogeneity across countries. The results indicated that biocapacity and financial development increased the ecological footprints, whereas globalization and natural resources were found to reduce it. Human well-being was observed to increase the ecological footprint in all the countries except Egypt. According to the study, strong institutions could assist in minimizing trade-offs and make it easier to achieve both environmental protection and greater human well-being at the same time (Nathaniel, Citation2021).
Cai et al. (Citation2018) used the newly introduced bootstrap ARDL bounds test with breaks at the structure to analyze the relationship between economic development, use of renewable energy, and carbon dioxide emissions in G7 countries. In Canada, France, Italy, the United States, and the United Kingdom, no correlation was found between real per capita GDP, consumption of clean energy, and carbon emissions. However, cointegration existed in Japan and Germany. According to the causality test results, clean energy use was found to cause real per capita GDP in Germany, Canada, and the United States. In contrast, CO2 emissions caused clean energy consumption in Germany. The Study suggested energy efficiency as a strategy to reduce CO2 emissions in G 7 economies (Cai et al., Citation2018).
It has been derived from the literature mentioned above that the bulk of the studies focused on CO2 emissions, financial growth, clean energy, economic globalization, and economic growth in the recent time period. These studies either considered the individual countries or different panels of the countries. For instance, Rafique et al. (Citation2021), A. G. Khan et al. (Citation2021), and Murshed et al. (Citation2021) studied these determinants of CO2 emission in individual economies, whereas Cai et al. (Citation2018), Anwar et al. (Citation2020), Kalayci, (Citation2019), and You and Lv (Citation2018) in different panels of the countries. However, except Ampofo et al. (Citation2021) and Nathaniel (Citation2021), none of the previous studies analyzed the effect of financial development and economic growth in a panel of N11 countries. Like the previous studies, we also look at the impact of financial development, clean energy, economic globalization, and economic growth on CO2 emission in N11 countries.
Nonetheless, we investigate the problem using some novel ways. The structural breaks in the data were ignored by the study of Ampofo et al. (Citation2021), and the environmental impact of economic growth was measured by ecological footprints in the study of Nathaniel (Citation2021). Considering these issues, this study is proceeding to measure the environmental effects of the variables in terms of CO2 emission by applying more advanced second-generation econometric techniques. We can construct a robust relationship in the presence of structural breaks by using robust second-generation econometric tools.
3. Data and model specification
On the basis of the support from the existing empirical findings, CO2 emission is used as the dependent variable, and clean energy, financial development, economic globalization, and economic growth as independent variables in the study. The data for CO2 emissions and clean energy (renewable energy) is taken from British Petroleum Website (BP, 2021). Data on economic globalization is taken from KOF Swiss Economic Institute, whereas data for financial development and GDP are gained from The World Bank (Bank, Citation2021). The model is specified in its general form as below:
(1)
(1)
Where ‘i’ denotes the cross-sections, ‘i.e. Egypt, Bangladesh, Indonesia, Mexico, Iran, Philippines, South Korea, Pakistan, Turkey, Nigeria, and Vietnam, while t stands for time-period from 1995 to 2018’. EquationEq. (1)
(1)
(1) in its regression form is given as follows:
(2)
(2)
Where CO2 is the carbon dioxide emission measured as million tons of carbon dioxide, GDPit is a gross domestic product with constant US dollar unit for a given period, 2010. FDit is the financial development measured as domestic credit to the private sector (percentage of GDP). EGLO is the economic globalization measured as the index based on actual flows of FDI, trade, portfolio investments, and restrictions, e.g. tariffs, import barriers, and taxes, and CLEANitx is the clean energy measured as renewable energy tetra watt-hours.
3.1. Estimation techniques
3.1.1. Cross-sectional dependence (CSD) testing
Our empirical analysis begins with cross-sectional dependence (CSD) testing between the cross-sectional units. Testing CSD is beneficial to select appropriate tests for stationarity/unit root from different generation tests that treat the issue of CSD. Various elements drive CSD, including economic and financial integrations, residual interdependence, shocks in oil prices, the global economic crisis, unobserved and observed omitted common factors, and globalization. There is no way to ignore the issue of CSD, as its ignorance can lead to spurious findings, biased stationarity, and cointegration. Pesaran et al. (Citation2004) CSD test is used to determine whether CSD problems exist in our data or not. (H0= no CSD and H1= CSD)
The CSD test is given as:
(a)
(a)
(b)
(b)
(c)
(c)
Shows the coefficient of residual pair-wise correlation of the Least Square residuals. In this situation, the null hypothesis is accepted if the panel data does not show any cross-sectional dependency.
3.1.2. Unit root tests
After getting the estimates for the CSD test, the second step is the unit root testing for the panel data. Panel data literature classifies non-stationarity in data into three generation categories of tests for unit root that can further be defined based on the issues that each strategy addresses. Choi (Citation2001), Levin et al. (Citation2002), and Maddala and Wu (Citation1999) address the problem of homogenous panel non-stationarity and Im et al. (Citation2003) of the heterogeneous panel. It is imperative to note that the tests of the second generation for panel data unit root established by Pesaran (Citation2007), Moon and Perron (Citation2012), and Choi (2006) address the parameter heterogeneity and solve the issue of CSD between cross-sectional units. However, when structural breaks in the series occur as a result of global or local circumstances, both the second and first-generation tests fail to function well. Third-generation panel tests deal with structural breaks in data and CSD and heterogeneity issues if structural breaks are present in data (Bai & Carrion-I-Silvestre, Citation2009). Because the presence of CSD makes it inappropriate to apply first-generation stationary tests, the study adopts Bai and Carrion-I-Silvestre (Citation2009) and Pesaran (Citation2007) techniques to address the stationarity in the presence of CSD (Jalil, Citation2014).
The Pesaran (Citation2007) test statistics are given as follows:
(d)
(d)
Where
shows the average cross-section and is represented as:
(e)
(e)
The CIPS test statistics is stated as
(f)
(f)
3.1.3. Slope homogeneity and cointegration tests
After verifying the stationarity or unit root in data, we applied (Pesaran & Yamagata, Citation2008) test to determine whether the slope is homogeneous or heterogeneous. The null hypothesis assumes that slopes are homogenous, and the alternative hypothesis assumes that slopes are heterogeneous.
The model of the test is given below:
(d)
(d)
(e)
(e)
Where
= Swamy test statistic and k = number of explanatory variables
The first generation cointegration techniques of Westerlund (Citation2005), Pedroni (Citation2004), Larsson et al. (Citation2001), and McCoskey and Kao (Citation1998) do not offer good predictions because of size parameters distortion arising because of the existence of CSD. We apply heterogeneous estimating approaches such as Westerlund and Edgerton (Citation2008) and Banerjee and Carrion-i-Silvestre (Citation2017) in CSD, heterogeneity of slope parameters non-stationarity problems in the data. These approaches address the difficulties mentioned earlier and spot structural breaks when cointegration is present. Westerlund (Citation2005) considers the problems of slope heterogeneity and CSD but neglects the impact of probable structural breaks, which may lead to accepting the H0 of no cointegration. However, Westerlund and Edgerton (Citation2008), unlike other tests, handle the problems of CSD, heterogeneous slopes, and serially correlated errors as well as probable structural breaks at various positions for every cross-section. Banerjee and Carrion-i-Silvestre (Citation2017) created a similar approach which is based on CCEMG. This approach is employed in the study to examine the cointegration among variables. This technique is best in dealing with CSD, non-stationarity, and parameters constantly estimated in the specious regression framework.
3.1.4. CS-ARDL analysis
A number of causes create common shocks, such as rising oil- prices and the worldwide economic crisis that can lead to CSD. If unknown factors are associated with independent variables in the model, this might lead to misleading results. CS-ARDL model is appropriate to apply in the case of the problem of slope heterogeneity and CSD. These problems can be overcome with the help of the CS- ARDL common correlated dynamic effects estimator (Y. Yao et al., Citation2019). The following is the beginning point for CS-ARDL as shown in Eq. (f):
(f)
(f)
Equation (f) is Autoregressive Distributed Lags (ARDL) model. In the presence of CSD, following Eq. (j) leads to misleading results. Eq. (g) is an expanded version of Eq. (f) that uses the cross-section mean of every regressor to avoid the unsatisfying inference generated by CSD about the existence of the threshold effect.
(g)
(g)
Where,
are the mean of the explained and explanatory variables under consideration, px, pw, pz, denotes lags of every variable. Further, Wit is the dependent variable which is CO2 emission, and Zit includes all the independent variables such as FD, CLEAN, GDP, and EGLO. X represents cross-sectional means (not only including time dummy variables and trends) in order to avoid CSD originated by spillover impacts (Liddle, Citation2018). The long-run coefficients are estimated from short-run coefficients in the CS-ARDL process. The MG estimation and long-run coefficient are represented as below:
(h)
(h)
The mean group is provided in Eq. (i)
(i)
(i)
Short-run coefficients are given as:
(j)
(j)
Where ΔI = t–(t–1)
(k)
(k)
(l)
(l)
(m)
(m)
ECM shows the adjustment speed towards equilibrium or the period of time an economy requires to attain the equilibrium level similar to the pooled mean group.
3.1.5. Heterogeneous panel causality test
Dumitrescu and Hurlin (Citation2012) state that ‘If each individual in the sample has a different economic behaviour than the rest, a homogenous specification of the relationship between the variables x and y makes it impossible to evaluate causality relationships’. As a result, they suggest a mean Wald statistic that tests the H0 of no causal association for any of the cross-sections, (H0 == 0, (i = 1 to N)) contrary to the H1 of one subgroup (at least) of the panel having causal relationships (H1 =
= 0, (i = 1 to N1);
when N1 = 0, rejecting H0 means that x granger causes y for all ‘i’, whereas when N1 > 0, rejecting H0 shows that the causal relationships and model differ from one sample or individual to another. In case of these conditions, the mean of the Wald statistic given by Dumitrescu and Hurlin (Citation2012) is as the following:
(n)
(n)
where WiT shows the Wald statistic ith cross-section.
4. Empirical findings and discussion
The empirical result of the CSD test is given in . CSD in the data must be addressed. Otherwise, spurious unit root and cointegration analyses may result. Based on empirical findings, we rejected the H0 of no CSD for all our variables, i.e. FD, EGLO, GDP CLEAN, at a 1% significance level. This evidences the presence of CSD in the panel data.
Table 1. CSD test results.
In the context of CSD, structural breaks, and slope heterogeneity, we used Bai and Carrion-I-Silvestre (Citation2009) and Pesaran (Citation2007) tests for panel unit root to assess the variable’s stationarity features.
The empirical results of both tests are presented in . These tests results that consider the structural breaks, CSD, and heterogeneity reject the H0 of unit root at the level. Bai and Carrion-I-Silvestre (Citation2009) Results were the only ones that accept the H0 of non-stationarity or unit root even after accounting for possible structural breaks. All the variables are stationary at the level in the Pesaran (Citation2007) test. That is why bai and carrion I Silvestre test is applied at first difference. All the variables such as FD, EGLO, CLEAN, and GDP are confirmed to be stationary at 1st difference.
Table 2. Results of unit root test: With and without structural break (Pesaran, Citation2007).
After using these tests, Pesaran and Yamagata (Citation2008) devise using an advanced version of Swamy (Citation1970) estimation for homogeneity of the slopes. As the assumption of slope homogeneity would produce deceptive estimation results, this approach checks for heterogeneous or homogeneous slope coefficients (Alam et al., Citation2018). It assumes homogenous slope coefficients for the H0 and reverses for the H1. provides the test results, which show that H0 is rejected at a 1 percent significance level.
Table 3. Slope heterogeneity test results.
Table 4. Westerlund and Edgerton (Citation2008) Panel cointegration results.
The empirical results of Westerlund and Edgerton (Citation2008) with H0 of no long-run cointegrating association between the variables are presented in . The empirical findings refute the H0 without a break at the mean and regime shift. The results confirm that cointegration exists between EGLO, CLEAN, GDP, and FD.
Table 5. Banerjee and Carrion-i-Silvestre (Citation2017) results.
summarizes the empirical findings of Banerjee and Carrion-i-Silvestre (Citation2017) technique and confirms that a cointegrating link exists between EGLO, CLEAN, GDP and FD for the whole sample and individual countries: Egypt, Bangladesh, Indonesia, Mexico, Iran, Philippines, South Korea, Pakistan, Turkey, Nigeria, and Vietnam.,.
The empirical results of the CS-ARDL model are summarized in . Economic growth is found to affect CO2 emissions positively, having a significant coefficient of 1%.
Table 6. Long run CS-ARDL results.
This denotes that for a 1 unit rise in GDP, CO2 emissions increase by 0.467 units. GDP measures a country’s health, including government expenditures, investment, consumption, net exports, etc. Consumption is a major component of GDP, and an increase in consumption is linked to rising carbon emissions. When income levels rise, government, businesses, and households may consume more, causing more CO2 emissions (Hasanov et al., Citation2018). This finding is not surprising, given that emerging countries, like the N11, focus on achieving economic growth in the preliminary stages of their development and tend to disregard the deteriorating environmental quality. The N11 countries are currently in the growth stage, integrating their economic expansion with poor environmental quality. This means that these countries have yet to reach the growth threshold beyond which there will be no such trade-off. A similar finding is provided by Sinha and Shahbaz (Citation2018), Mikayilov et al. (Citation2018), Li et al. (Citation2021), and Ampofo et al. (Citation2021).
Similarly, financial development is also positively related to carbon dioxide emissions. It is indicated from the findings that a one-unit rise in the development of the financial sector leads to a rise of 0.196 units in CO2 emissions. Our findings corroborate that bank loans help businesses acquire external financing and expand their scale of investment. This improves economic growth and accelerates CO2 emissions, both of which are dependent on bank asset growth (Y.-J. Zhang, Citation2011). Business firms and households both benefit from increased access to financial capital as a result of financial development. This raises demand for machines, automobiles, and other goods, which boosts manufacturing and transportation activity. As energy use rises, it leads to more emissions of CO2 into the atmosphere and pollutants into the water (Shahzad et al., Citation2017). This result has been noticed before by Nathaniel (Citation2021), Boutabba (Citation2014), Y.-J. Zhang (Citation2011), and Shahzad et al. (Citation2017).
Like financial development and GDP, economic globalization is also observed to increase CO2 emissions in N11 economies. An increase of 1 unit in economic globalization causes CO2 emission to increase by 0.235 units. One explanation of the finding is that with economic globalization, it becomes easier for multinational corporations to transfer their dirty manufacturing operations to lower-income countries to give lower wages and take advantage of easy environmental regulations, resulting in environmental deterioration (Ahmed et al., Citation2021). The use of dirty technologies and low-cost production strategies by foreign investors exploit the natural resources. Moreover, an increase in energy intensity following an increase in the rate of globalization, including increased transportation, human activities, and industrial output, fuels the positive relationship between globalization and CO2emissions. Our results support the results of Kirikkaleli and Adebayo (Citation2021), Kalayci (Citation2019), and Nguyen and Le (Citation2020).
Last, unlike other variables, clean energy is found to affect CO2 emissions negatively in our empirical analysis. If clean energy increases by one unit, it causes CO2 emissions to reduce by 0.25 units. To put it another way, using clean energy helps to enhance the environment by decreasing energy dependency and energy security as clean energy provide alternative resources of energy that are less polluting (e.g. biomass, geothermal, hydropower, wind, nuclear, biofuels, solar, respectively) (Usman et al., Citation2020). Clean energy, as state-of-the-art acknowledges, helps to minimize CO2 emissions as countries use more renewable energy and emit lesser CO2 in the environment. The finding of our study seconds the findings of Dogan and Seker (Citation2016), Murshed et al. (Citation2021), and Usman et al. (Citation2020).
The short-run CS-ARDL results are provided in , and a favourable relationship was observed between GDP, FD, EGLO, and CO2 emissions. Contrarily, CLEAN energy affects CO2 emissions negatively in the short run. The ECM shows the CS-ARDL’s adjustment pace toward equilibrium which is −0.234%. FD, EGLO, CLEAN, and GDP have short-run coefficients of 0.039, 0.024, 0.103, and 0.051. Coefficients in the short run are lower than long-run coefficients. This is primarily because these economies are developing ones that are currently growing, particularly having more industrial growth, which affects CO2 emissions positively (Umar, Su, Rizvi, et al., Citation2021).
Table 7. CS-ARDL analysis results (Short-run).
Last, the (Dumitrescu & Hurlin, Citation2012) test of panel causality results is given in . All the test statistics are highly significant, indicating that co2 emissions and variables have a bi-directional causal relationship. For N11 countries, a causality relationship exists from FD to CO2 and CO2 to FD, from EGLO to CO2 and CO2 to EGLO, from CLEAN to CO2 and CO2 to CLEAN, and last, from GDP to CO2 and CO2 to GDP.
Table 8. Dumitrescu and Hurlin (Citation2012) panel causality test results.
5. Concluding remarks and policy recommendations
Ensured economic growth and protection of the environment simultaneously have become a major development priority around the world. Energy is the factor that is considered the key driver of the growth of an economy in both advanced and emerging/developing countries. However, energy is also the cause of the imbalance situation between economics and the environment. On the other hand, due to globalization which undoubtedly contributes significantly to the global economic and financial development, however, it has also been reported as the strategy by which the developed countries shifts their environment polluting industries to the countries that are having least on no enforcement of ecology and environment protection laws and regulations. The case of N-11 countries are extremely crucial because of being account for 9 percent of global GDP in 2016 and are also anticipated to experience significant economic growth, which can further harm the environment in these countries.
Hence the present study evaluated the role of economic globalization, clean energy, financial development, and economic growth in climate prevention for a panel of N11 nations, namely, Egypt, Bangladesh, Indonesia, Mexico, Iran, South Korea, Pakistan, Philippines, Turkey, Vietnam and Nigeria throughout 1995–2018. Pesaran et al. (Citation2004) test for CSD estimation and tests of Pesaran (Citation2007) and Bai and Carrion-I-Silvestre (Citation2009) for unit root are applied to the data. In addition, the Pesaran and Yamagata (Citation2008) technique was used to evaluate slope homogeneity in this study while relying on Westerlund and Edgerton (Citation2008) and Banerjee and Carrion-i-Silvestre (Citation2017) approaches to estimate the long term association among all the variables. All the variables are found to have a long-run association in cointegration test results. The coefficients estimated through the CS-ARDL test established that economic growth, financial development, economic globalization positively impacted, while clean energy negatively impacted CO2 emissions for the Next 11 countries.
Moreover, the magnitude of all the long-run coefficients is higher than the magnitude of the short-run coefficients. This is mainly because these countries are growing in industrial terms that have a high impact on CO2 emission. Last, (Dumitrescu & Hurlin, Citation2012) test for panel causality is applied, and the bidirectional relationship between dependent and explanatory variables is found.
5.1. Policy recommendations
The study’s findings are robust to various policy recommendations for the policymakers and governments in N11 countries. The study recommends that economic growth policies be adopted that can accelerate economic growth, but these policies must be aligned with achieving environmental sustainability targets. These policies will be helpful to reduce the trade-off between economic expansion and environmental sustainability in the earlier stages of growth. Moreover, consumption levels must be targeted to minimize the impact of growth on CO2 emission, particularly in more energy-intensive sectors, resulting in higher carbon emissions. Secondly, the N11 countries must move away from their conventional reliance on fossil fuels for energy. The long-run incentives must be provided, and the governments must pursue the policies, which are helpful to the production of clean energy. The economies should gradually integrate renewable energy resources into their energy systems to improve the overall quality of the environment. The policymakers must pay sufficient attention to make the public aware of clean energy and its role in the green and clean economy.
Third, the N11 countries should strengthen their financial development strategies by incorporating environmental welfare goals into financial development plans. It is recommended that the considerably cleaner industries operating inside the N11 countries receive a bigger proportion of total domestic credit provided to the private sector. Simultaneously, governments must support credits used to invest in ecologically sustainable initiatives, which would help to mitigate the negative environmental effects of financial development. Governments should also introduce green bonds to incentivize borrowers to have an investment in ecologically sustainable initiatives. As a result, financial growth in these countries should preferably make green funding schemes more accessible. Finally, we propose that governments utilize appropriate and efficient policy coordination to reduce globalization’s environmental costs. Given the negative environmental implications of globalization, we believe that when developing a significant and long environmental policy framework in N11 countries, policymakers should consider the role that globalization plays in the trends of CO2 emissions. We also propose that policymakers consider globalization as a significant economic factor in the framework of economic policies to improve long-term environmental quality. Aside from that, there is a need to improve research related to energy and consider the broader globalization role in the demand for energy and emission.
5.2. Limitations and future recommendations
Similar to other researchers, the present research also comprises certain limitations that provide an avenue for the researchers to explore further. Firstly, the present study is based on the panel research methodology, whereas there is a possibility that the focused countries from this panel may behave differently from each other. Therefore, it is suggested to explore the similar phenomena country-wise individually. Secondly, the current study is based on the panel data of N-11 countries only. More exploration in terms of better understanding the pollution needs to be done in higher polluting countries.
Moreover, a comparison between developed countries and developing countries can also significantly contribute to the existing literature. Statistically, the application of CS-ARDL is based on integrating the average of the independent variable with the dependent variables. However, there is a possibility that the data behaves differently at different quantiles. For that purpose, applying the quantiles-based estimation technique can also lead to an in-depth understanding of the findings. Lastly, the employment of regulation oriented phenomena is also suggested to evaluate compliance to the environmental rules and regulations among the economies for environment preservation and protection.
Disclosure statement
No potential conflict of interest was reported by the authors.
Notes
1 Goldman Sachs Group (2007) defined the N11 countries as the next BRICs.
References
- Ahmed, Z., Cary, M., & Le, H. P. (2021). Accounting asymmetries in the long-run nexus between globalization and environmental sustainability in the United States: An aggregated and disaggregated investigation. Environmental Impact Assessment Review, 86, 106511. https://doi.org/10.1016/j.eiar.2020.106511
- Alam, M. S., Miah, M. D., Hammoudeh, S., & Tiwari, A. K. (2018). The nexus between access to electricity and labour productivity in developing countries. Energy Policy, 122, 715–726. https://doi.org/10.1016/j.enpol.2018.08.009
- Ampofo, G. K. M., Cheng, J., Ayimadu, E. T., & Asante, D. A. (2021). Investigating the asymmetric effect of economic growth on environmental quality in the next 11. Energies, 14(2), 491. https://doi.org/10.3390/en14020491
- Anwar, A., Sharif, A., Fatima, S., Ahmad, P., Sinha, A., Khan, S. A. R., & Jermsittiparsert, K. (2021). The asymmetric effect of public private partnership investment on transport CO2 emission in China: Evidence from quantile ARDL approach. Journal of Cleaner Production, 288, 125282. https://doi.org/10.1016/j.jclepro.2020.125282
- Anwar, A., Younis, M., & Ullah, I. (2020). Impact of urbanization and economic growth on CO2 emission: A case of far east Asian countries. International Journal of Environmental Research and Public Health, 17(7), 2531. https://doi.org/10.3390/ijerph17072531
- Apergis, N., & Payne, J. E. (2012). Renewable and non-renewable energy consumption-growth nexus: Evidence from a panel error correction model. Energy Economics, 34(3), 733–738. https://doi.org/10.1016/j.eneco.2011.04.007
- Bai, J., & Carrion-I-Silvestre, J. L. (2009). Structural changes, common stochastic trends, and unit roots in panel data. Review of Economic Studies, 76(2), 471–501. https://doi.org/10.1111/j.1467-937X.2008.00530.x
- Baloch, Z. A., Tan, Q., Kamran, H. W., Nawaz, M. A., Albashar, G., & Hameed, J. (2021). A multi-perspective assessment approach of renewable energy production: Policy perspective analysis. Environment, Development and Sustainability, 1–29. https://doi.org/10.1007/s10668-021-01524-8
- Banerjee, A., & Carrion-i-Silvestre, J. L. (2017). Testing for panel cointegration using common correlated effects estimators. Journal of Time Series Analysis, 38(4), 610–636. https://doi.org/10.1111/jtsa.12234
- Bank, W. (2021). World development report 2021: Data for better lives. The World Bank.
- Bibi, A., Zhang, X., & Umar, M. (2021). The imperativeness of biomass energy consumption to the environmental sustainability of the United States revisited. Environmental and Ecological Statistics, 28(4), 821–841. https://doi.org/10.1007/s10651-021-00500-9
- Boutabba, MA (2014). The impact of financial development, income, energy and trade on carbon emissions: evidence from the Indian economy. Economic Modeling, 40, 33–41.
- Cai, Y., Sam, C. Y., & Chang, T. (2018). Nexus between clean energy consumption, economic growth and CO2 emissions. Journal of Cleaner Production, 182, 1001–1011. https://doi.org/10.1016/j.jclepro.2018.02.035
- Chien, F., Kamran, H. W., Nawaz, M. A., Thach, N. N., Long, P. D., & Baloch, Z. A. (2021). Assessing the prioritization of barriers toward green innovation: Small and medium enterprises Nexus. Environment, Development and Sustainability, 1–31. https://doi.org/10.1007/s10668-021-01513-x
- Chien, F., Sadiq, M., Kamran, H. W., Nawaz, M. A., Hussain, M. S., & Raza, M. (2021). Co-movement of energy prices and stock market return: Environmental wavelet nexus of COVID-19 pandemic from the USA, Europe, and China. Environmental Science and Pollution Research, 1–15. https://doi.org/10.1007/s11356-021-12938-2
- Choi, I. (2001). Unit root tests for panel data. Journal of International Money and Finance, 20(2), 249–272. https://doi.org/10.1016/S0261-5606(00)00048-6
- Choi, I. (2006). Econometric theory and practice: Frontiers of analysis and applied research (pp. 311–333).
- Claessens, S., & Feijen, E. (2007). Financial sector development and the millennium development goals. (Issue 89). World Bank Publications.
- Copeland, B. R., & Taylor, M. S. (2004). Trade, growth, and the environment. Journal of Economic Literature, 42(1), 7–71. https://doi.org/10.1257/.42.1.7
- Destek, M. A., & Okumus, I. (2019). Does pollution haven hypothesis hold in newly industrialized countries? Evidence from ecological footprint. Environmental Science and Pollution Research International, 26(23), 23689–23695.
- Dogan, E., & Seker, F. (2016). Determinants of CO2 emissions in the European Union: The role of renewable and non-renewable energy. Renewable Energy., 94, 429–439. https://doi.org/10.1016/j.renene.2016.03.078
- Dumitrescu, E.-I., & Hurlin, C. (2012). Testing for Granger non-causality in heterogeneous panels. Economic Modelling, 29(4), 1450–1460. https://doi.org/10.1016/j.econmod.2012.02.014
- Frankel, J. A., & Romer, D. H. (1999). Does trade cause growth?. American economic review, 89(3), 379–399.
- Georgescu, M., Lobell, D. B., & Field, C. B. (2011). Direct climate effects of perennial bioenergy crops in the United States. Proceedings of the National Academy of Sciences, 108(11), 4307–4312. https://doi.org/10.1073/pnas.1008779108
- Ghosh, S. (2010). Examining carbon emissions economic growth nexus for India: A multivariate cointegration approach. Energy Policy, 38(6), 3008–3014. https://doi.org/10.1016/j.enpol.2010.01.040
- Goldman, S. (2007). BRICS and Beyond. Goldman Sachs Global Economics Group. http://www.goldmansachs.com/japan/ideas/brics/book/bric-full.pdf.
- Gozgor, G., & Can, M. (2017). Does export product quality matter for CO2 emissions? Evidence from. Environmental Science and Pollution Research, 24(3), 2866–2875. https://doi.org/10.1007/s11356-016-8070-6
- Gozgor, G., Mahalik, M. K., Demir, E., & Padhan, H. (2020). The impact of economic globalization on renewable energy in the OECD countries. Energy Policy, 139, 111365. https://doi.org/10.1016/j.enpol.2020.111365
- Group, W. B. (2016). World development report 2016: Digital dividends. World Bank Publications.
- Hasanov, F. J., Liddle, B., & Mikayilov, J. I. (2018). The impact of international trade on CO2 emissions in oil exporting countries: Territory vs consumption emissions accounting. Energy Economics, 74, 343–350. https://doi.org/10.1016/j.eneco.2018.06.004
- Im, K. S., Pesaran, M. H., & Shin, Y. (2003). Testing for unit roots in heterogeneous panels. Journal of Econometrics, 115(1), 53–74. https://doi.org/10.1016/S0304-4076(03)00092-7
- Jalil, A. (2014). Energy–growth conundrum in energy exporting and importing countries: Evidence from heterogeneous panel methods robust to cross-sectional dependence. Energy Economics, 44, 314–324. https://doi.org/10.1016/j.eneco.2014.04.015
- Ji, X., Zhang, Y., Mirza, N., Umar, M., & Rizvi, S. K. A. (2021). The impact of carbon neutrality on the investment performance: Evidence from the equity mutual funds in BRICS. Journal of Environmental Management, 297, 113228. https://doi.org/10.1016/j.jenvman.2021.113228
- Kalayci, C. (2019). The impact of economic globalization on CO2 emissions: The case of NAFTA countries. International Journal of Energy Economics and Policy, 9(1), 356.
- Kirikkaleli, D., & Adebayo, T. S. (2021). Do renewable energy consumption and financial development matter for environmental sustainability? New global evidence. Sustainable Development, 29(4), 583–594. https://doi.org/10.1002/sd.2159
- Larsson, R., Lyhagen, J., & Löthgren, M. (2001). Likelihood‐based cointegration tests in heterogeneous panels. The Econometrics Journal, 4(1), 109–142. https://doi.org/10.1111/1368-423X.00059
- Levin, A., Lin, C.-F., & Chu, C.-S J. (2002). Unit root tests in panel data: Asymptotic and finite-sample properties. Journal of Econometrics, 108(1), 1–24. https://doi.org/10.1016/S0304-4076(01)00098-7
- Li, W., Chien, F., Hsu, C.-C., Zhang, Y., Nawaz, M. A., Iqbal, S., & Mohsin, M. (2021). Nexus between energy poverty and energy efficiency: Estimating the long-run dynamics. Resources Policy, 72, 102063. https://doi.org/10.1016/j.resourpol.2021.102063
- Liddle, B. (2018). Consumption-based accounting and the trade-carbon emissions nexus. Energy Economics, 69, 71–78. https://doi.org/10.1016/j.eneco.2017.11.004
- Maddala, G. S., & Wu, S. (1999). A comparative study of unit root tests with panel data and a new simple test. Oxford Bulletin of Economics and Statistics, 61(S1), 631–652. https://doi.org/10.1111/1468-0084.0610s1631
- McCoskey, S., & Kao, C. (1998). A residual-based test of the null of cointegration in panel data. Econometric Reviews, 17(1), 57–84. https://doi.org/10.1080/07474939808800403
- Mikayilov, J. I., Galeotti, M., & Hasanov, F. J. (2018). The impact of economic growth on CO2 emissions in Azerbaijan. Journal of Cleaner Production, 197, 1558–1572. https://doi.org/10.1016/j.jclepro.2018.06.269
- Mohsin, M., Kamran, H. W., Nawaz, M. A., Hussain, M. S., & Dahri, A. S. (2021). Assessing the impact of transition from nonrenewable to renewable energy consumption on economic growth-environmental nexus from developing Asian economies. Journal of Environmental Management, 284, 111999.
- Moon, H. R., & Perron, B. (2012). Beyond panel unit root tests: Using multiple testing to determine the nonstationarity properties of individual series in a panel. Journal of Econometrics, 169(1), 29–33. https://doi.org/10.1016/j.jeconom.2012.01.008
- Murshed, M., Ahmed, Z., Alam, M. S., Mahmood, H., Rehman, A., & Dagar, V. (2021). Reinvigorating the role of clean energy transition for achieving a low-carbon economy: Evidence from Bangladesh. Environmental Science and Pollution Research, 1–22. https://doi.org/10.1007/s11356-021-15352-w
- Naqvi, S. A. A., Shah, S. A. R., Anwar, S., & Raza, H. (2021). Renewable energy, economic development, and ecological footprint nexus: Fresh evidence of renewable energy environment Kuznets curve (RKC) from income groups. Environmental Science and Pollution Research International, 28(2), 2031–2051.
- Nathaniel, S. P. (2021). Ecological footprint and human well-being nexus: Accounting for broad-based financial development, globalization, and natural resources in the Next-11 countries. Future Business Journal, 7(1), 1–18. https://doi.org/10.1186/s43093-021-00071-y
- Nawaz, M. A., Seshadri, U., Kumar, P., Aqdas, R., Patwary, A. K., & Riaz, M. (2021). Nexus between green finance and climate change mitigation in N-11 and BRICS countries: Empirical estimation through difference in differences (DID) approach. Environmental Science and Pollution Research International, 28(6), 6504–6519.
- Nguyen, T., & Le, Q. (2020). Impact of globalization on CO2 emissions in Vietnam: An autoregressive distributed lag approach. Decision Science Letters, 9(2), 257–270.
- Özsoy, F., & Ozpolat, A. (n.d.) Do technological development and clean energy effect environmental awareness?: An empirical analysis for Turkey. Gaziantep University Journal of Social Sciences, 19(3), 789–804.
- Pata, U. K., & Yilanci, V. (2021). Investigating the persistence of shocks on the ecological balance: Evidence from G10 and N11 countries. Sustainable Production and Consumption, 28, 624–636. https://doi.org/10.1016/j.spc.2021.06.027
- Pedroni, P. (2004). Panel cointegration: Asymptotic and finite sample properties of pooled time series tests with an application to the PPP hypothesis. Econometric Theory, 20(03), 597–625. https://doi.org/10.1017/S0266466604203073
- Pesaran, M. H. (2007). A simple panel unit root test in the presence of cross‐section dependence. Journal of Applied Econometrics, 22(2), 265–312. https://doi.org/10.1002/jae.951
- Pesaran, M. H., Schuermann, T., & Weiner, S. M. (2004). Modeling regional interdependencies using a global error-correcting macroeconometric model. Journal of Business & Economic Statistics, 22(2), 129–162. https://doi.org/10.1198/073500104000000019
- Pesaran, M. H., & Yamagata, T. (2008). Testing slope homogeneity in large panels. Journal of Econometrics, 142(1), 50–93. https://doi.org/10.1016/j.jeconom.2007.05.010
- Piłatowska, M., & Geise, A. (2021). Impact of clean energy on CO2 emissions and economic growth within the phases of renewables diffusion in selected european countries. Energies, 14(4), 812. https://doi.org/10.3390/en14040812
- Rafique, A., Quddoos, M. U., Ali, S., Aslam, F., Ahmad, M., Güzel, A. E., Akin, C. S., Mushtaq, M., Ahmed, S., Taheri, A., Nessabian, S., Moghaddasi, R., Arbabi, F., Damankeshideh, M., Dang, V. D., Huynh, J., de Salles, A. A., Ananwude, A. C., Ibenta, S. N., … & Trivedi, P. (2021). Monetary policy transmission: Balance sheet channel and investment behavior of firms in Pakistan. Economic Journal of Emerging Markets, 13(1), 1–108. file:///C:/Users/Mg/Downloads/1444-255-PB.pdf
- Razzaq, A., Ajaz, T., Li, J. C., Irfan, M., & Suksatan, W. (2021). Investigating the asymmetric linkages between infrastructure development, green innovation, and consumption-based material footprint: Novel empirical estimations from highly resource-consuming economies. Resources Policy, 74, 102302. https://www.bp.com/content/dam/bp/business-sites/en/global/corporate/pdfs/energy-economics/statistical-review/bp-stats-review-2021-full-report.pdf
- Sadorsky, P. (2010). The impact of financial development on energy consumption in emerging economies. Energy Policy, 38(5), 2528–2535. https://doi.org/10.1016/j.enpol.2009.12.048
- Shahbaz, M., Solarin, S. A., & Ozturk, I. (2016). Environmental Kuznets curve hypothesis and the role of globalization in selected African countries. Ecological Indicators, 67, 623–636. https://doi.org/10.1016/j.ecolind.2016.03.024
- Shahzad, S. J. H., Kumar, R. R., Zakaria, M., & Hurr, M. (2017). Carbon emission, energy consumption, trade openness and financial development in Pakistan: A revisit. Renewable and Sustainable Energy Reviews, 70, 185–192. https://doi.org/10.1016/j.rser.2016.11.042
- Shair, F., Shaorong, S., Kamran, H. W., Hussain, M. S., Nawaz, M. A., & Nguyen, V. C. (2021). Assessing the efficiency and total factor productivity growth of the banking industry: Do environmental concerns matters? Environmental Science and Pollution Research International, 28(16), 20822–20838.
- Sinha, A., & Shahbaz, M. (2018). Estimation of environmental Kuznets curve for CO2 emission: Role of renewable energy generation in India. Renewable Energy., 119, 703–711. https://doi.org/10.1016/j.renene.2017.12.058
- Su, C.-W., Khan, K., Umar, M., & Zhang, W. (2021). Does renewable energy redefine geopolitical risks? Energy Policy, 158, 112566. https://doi.org/10.1016/j.enpol.2021.112566
- Sun, H., Awan, R. U., Nawaz, M. A., Mohsin, M., Rasheed, A. K., & Iqbal, N. (2021). Correction to: Assessing the socio-economic viability of solar commercialization and electrification in south Asian countries. Environment, Development and Sustainability, 23(8), 12659–12660. https://doi.org/10.1007/s10668-020-01145-7
- Su, C.-W., Qin, M., Tao, R., & Umar, M. (2020). Does oil price really matter for the wage arrears in Russia? Energy, 208, 118350. https://doi.org/10.1016/j.energy.2020.118350
- Su, C.-W., Yuan, X., Tao, R., & Umar, M. (2021). Can new energy vehicles help to achieve carbon neutrality targets? Journal of Environmental Management, 297, 113348. https://doi.org/10.1016/j.jenvman.2021.113348
- Swamy, P. A. (1970). Efficient inference in a random coefficient regression model. Econometrica, 38(2), 311–323. https://doi.org/10.2307/1913012
- Taghizadeh-Hesary, F., Rasoulinezhad, E., Yoshino, N., Sarker, T., & Mirza, N. (2021). Determinants of the Russia and Asia–Pacific energy trade. Energy Strategy Reviews, 38, 100681. https://doi.org/10.1016/j.esr.2021.100681
- Taghizadeh-Hesary, F., & Yoshino, N. (2020). Sustainable solutions for green financing and investment in renewable energy projects. Energies, 13(4), 788. https://doi.org/10.3390/en13040788
- Tamazian, A., Chousa, J. P., & Vadlamannati, K. C. (2009). Does higher economic and financial development lead to environmental degradation: Evidence from BRIC countries. Energy Policy, 37(1), 246–253. https://doi.org/10.1016/j.enpol.2008.08.025
- Ullah, S., Ozturk, I., Usman, A., Majeed, M. T., & Akhtar, P. (2020). On the asymmetric effects of premature deindustrialization on CO2 emissions: Evidence from Pakistan. Environmental Science and Pollution Research International, 27(12), 13692–13702.
- Umar, M., Ji, X., Kirikkaleli, D., & Alola, A. A. (2021). The imperativeness of environmental quality in the United States transportation sector amidst biomass-fossil energy consumption and growth. Journal of Cleaner Production, 285, 124863. https://doi.org/10.1016/j.jclepro.2020.124863
- Umar, M., Ji, X., Kirikkaleli, D., Shahbaz, M., & Zhou, X. (2020). Environmental cost of natural resources utilization and economic growth: Can China shift some burden through globalization for sustainable development? Sustainable Development, 28(6), 1678–1688. https://doi.org/10.1002/sd.2116
- Umar, M., Ji, X., Kirikkaleli, D., & Xu, Q. (2020). COP21 Roadmap: Do innovation, financial development, and transportation infrastructure matter for environmental sustainability in China? J Environ Manage, 271, 111026. https://doi.org/10.1016/j.jenvman.2020.111026
- Umar, M., Ji, X., Mirza, N., & Rahat, B. (2021). The impact of resource curse on banking efficiency: Evidence from twelve oil producing countries. Resources Policy, 72, 102080. https://doi.org/10.1016/j.resourpol.2021.102080
- Umar, M., Su, C.-W., Rizvi, S. K. A., & Lobonţ, O.-R. (2021). Driven by fundamentals or exploded by emotions: Detecting bubbles in oil prices. Energy, 231, 120873. https://doi.org/10.1016/j.energy.2021.120873
- Usman, A., Ullah, S., Ozturk, I., Chishti, M. Z., & Zafar, S. M. (2020). Analysis of asymmetries in the nexus among clean energy and environmental quality in Pakistan. Environmental Science and Pollution Research International, 27(17), 20736–20747.
- Wang, Z. (2019). Does biomass energy consumption help to control environmental pollution? Evidence from BRICS countries. Science of the Total Environment, 670, 1075–1083. https://doi.org/10.1016/j.scitotenv.2019.03.268
- Wang, Q.-S., Su, C.-W., Hua, Y.-F., & Umar, M. (2021). How can new energy vehicles affect air quality in China?—From the perspective of crude oil price. Energy & Environment, 0958305X2110443. https://doi.org/10.1177/0958305X211044388
- Wang, K.-H., Umar, M., Akram, R., & Caglar, E. (2021). Is technological innovation making world “Greener”? An evidence from changing growth story of China. Technological Forecasting and Social Change, 165, 120516. https://doi.org/10.1016/j.techfore.2020.120516
- Westerlund, J. (2005). New simple tests for panel cointegration. Econometric Reviews, 24(3), 297–316. https://doi.org/10.1080/07474930500243019
- Westerlund, J., & Edgerton, D. L. (2008). A simple test for cointegration in dependent panels with structural breaks. Oxford Bulletin of Economics and Statistics, 70(5), 665–704. https://doi.org/10.1111/j.1468-0084.2008.00513.x
- Xu, Q., Umar, M., & Ji, X. (2020). Time-frequency analysis between renewable and nonrenewable energy consumption, economic growth, and CO2 emissions in the United States: Evidence from the transportation sector. 2964–2974. https://doi.org/10.1061/9780784483053.250
- Yan, L., Mirza, N., & Umar, M. (2021). The cryptocurrency uncertainties and investment transitions: Evidence from high and low carbon energy funds in China. Technological Forecasting and Social Change, 121326. https://doi.org/10.1016/j.techfore.2021.121326
- Yang, X., Li, N., Mu, H., Pang, J., Zhao, H., & Ahmad, M. (2021). Study on the long-term impact of economic globalization and population aging on CO2 emissions in OECD countries. Science of the Total Environment, 787, 147625. https://doi.org/10.1016/j.scitotenv.2021.147625
- Yao, Y., Ivanovski, K., Inekwe, J., & Smyth, R. (2019). Human capital and energy consumption: Evidence from OECD countries. Energy Economics, 84, 104534. https://doi.org/10.1016/j.eneco.2019.104534
- Yao, X., Yasmeen, R., Hussain, J., & Shah, W. U. H. (2021). The repercussions of financial development and corruption on energy efficiency and ecological footprint: Evidence from BRICS and next 11 countries. Energy, 223, 120063. https://doi.org/10.1016/j.energy.2021.120063
- You, W., & Lv, Z. (2018). Spillover effects of economic globalization on CO2 emissions: A spatial panel approach. Energy Economics, 73, 248–257. https://doi.org/10.1016/j.eneco.2018.05.016
- Zhang, Y.-J. (2011). The impact of financial development on carbon emissions: An empirical analysis in China. Energy Policy, 39(4), 2197–2203. https://doi.org/10.1016/j.enpol.2011.02.026
- Zhang, J., Dai, Y., Su, C.-W., Kirikkaleli, D., & Umar, M. (2021). Intertemporal change in the effect of economic growth on carbon emission in China. Energy & Environment, 32(7), 1207–1225. https://doi.org/10.1177/0958305X211008618
- Zhang, B., Wang, B., & Wang, Z. (2017). Role of renewable energy and non-renewable energy consumption on EKC: evidence from Pakistan. Journal of Cleaner Production, 156, 855–864.
- Zhuang, Y., Yang, S., Chupradit, S., Nawaz, M. A., Xiong, R., & Koksal, C. (2021). A nexus between macroeconomic dynamics and trade openness: Moderating role of institutional quality. Business Process Management Journal, 27(6), 1703–1719. https://doi.org/10.1108/BPMJ-12-2020-0594