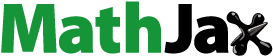
Abstract
The adoption of green technology is imperative to realise sustainable development. Considering the same, this study explores the drivers of Green Innovation (GI) based on the theoretical foundation of the Triple Bottom Line (environmental, social, and economic factors) with the integration of information and communication technologies (ICT) and institutional governance (INST) in Pakistan. This study employs a nonlinear autoregressive distributed lag (NARDL) framework on quarterly data from Q1-1996 to Q4-2019. The results reveal that positive shocks in human capital (HCI) instigate GI by 1.05%, while negative shock undermines GI by 0.93%. Similarly, positive shocks in carbon dioxide (CO2) emissions increase GI by 0.63%, while any negative shock undermines GI by 0.01%. On the other hand, positive shock in ICT leads to 0.55% advanced GI; however, this effect turned stronger in negative shocks, which leads to reduced GI by 0.78% in the long-run. These results confirm the asymmetricity because positive and negative shocks in HCI, CO2 emissions, and ICT instigated GI differently. Finally, INST and GDP contribute to enhancing GI by 0.12% and 1.69%, respectively. The results indicate that the Pakistan government should improve institutional governance, adapt, and focus on sustainable practices with ICT integration to promote green technologies.
1. Introduction
From the last decade, exponential economic growth and industrialisation have been witnessed in many countries yet failed to keep the environment sustainable due to various challenges related to resource depletion. These challenges urge policymakers to develop sustainable and practical solutions on a war footing basis (Khan et al., Citation2019). To mitigate environmental risks derived from the industrial revolution, green technologies (energy efficient) are expected to become a dominant factor that practically reduces over 60% of targeted CO2 emissions (Du et al., Citation2019). Green technologies include, for instance, controlling pollution, waste management, clean technology, and clean-up technology (Chen & Lee, Citation2020). Further, it might depend on the particular environment, social, and economic factors facilitating triple bottom line (TBL) (United Nations, Citation2018). Green innovation (GI) refers to generating new ideas, goods, services, processes, or management systems that can be used to deal with environmental problems (Shahzad et al., Citation2020a; Sun et al., Citation2021). However, understanding the comprehensive relationship between TBL and GI is imperative to realise Sustainable Development Goals (SDGs) (An et al., Citation2021).
Recently, technological innovation has become an essential means for reducing hazardous emissions worldwide (Maasoumi et al., Citation2020). Prior studies proposed that the impact of technological innovation on dangerous emissions (such as CO2 emissions) might be positive or negative due to the rebound effect (Du et al., Citation2019). Environmental deterioration is becoming a global threat leading to socio-economic problems, such as health problems, which are 91% climate-related. Almost 1.3 million people were killed and 4.4 billion injured from 1998 to 2017 (UNDP). To ensure environmental stewardship, human capital development, sequester allocation of R&D investment, institutional governance, and economic progression are deriving factors of new technologies that can be translated into environmental sustainability (Razzaq et al., Citation2021). Extant literature highlighted the importance of human capital development for education and awareness regarding pro-environmental behaviour that reduces environmental deterioration (Shahzad et al., Citation2020b). Further, it is also considered one of the most significant elements that positively affect total factor productivity and technological innovation (Hu, Citation2021).
Industrialised and advanced economies are now collaborating to cope with hazardous emission-related environmental deterioration and CO2 emissions (Maasoumi et al., Citation2020; Razzaq et al., Citation2021). Ahmad et al. (Citation2021) identified that overdependence on coal energy is the primary source of CO2 emissions. Stringency in environmental protection strategies, environmental tax, and reduced CO2 emissions positively affects GI (Maasoumi et al., Citation2020; Sharif et al., Citation2019). Further, ICT infrastructure, including innovative equipment, provides multiple channels to reduce CO2 emissions, energy consumption, and environmental pollution (Stucki & Woerter, Citation2019). Such channels are price effect, substitution effect, and use effect (Razzaq et al., Citation2021). Without proper legislation and implementation, it is hard to implement green growth agenda.
Previous studies identified that reducing production costs and GDP growth are also the key drivers of GI (Saunila et al., Citation2018). Hu (Citation2021) acknowledges that GDP has a positive effect on technological innovation. Previous scholars unveiled that integrating these sustainable strategies helps survival and smooth operations in the technologically changing business environment (Saunila et al., Citation2018; Yu et al., Citation2020). Awan et al. (Citation2021) argued that implementing GI strategy industries can reduce operating costs and improve processes and product innovation. Research on developing economies like Pakistan can provide better visions on how various factors control environmental deterioration and reform environmentally friendly environments. Pakistan has natural gas, coal, crude oil, iron, salt, copper, limestone, and water resources and has excellent growth potential (Sharif et al., Citation2019). As per GII, Pakistan is one of the least innovative countries, and its ranking is low (Global Innovation Index, Citation2018). The most valuable factors that hinder innovation and growth are institutional, infrastructure, and technology (Shahzad et al., Citation2020c; Zhuang et al., Citation2021).
Although several research studies have attempted to examine the relationship between targeted variables (Razzaq et al., Citation2021), existing studies mainly explored the link between GI and TBL using survey data. They produced inconclusive results due to subjectivity bias (Saunila et al., Citation2018). However, empirical evidence does not consider these core factors with the TBL assimilation in an encompassing framework. Despite ample literature on GI and SD, the aspect of how the TBL approach influences these are still not adequately engrossed (Zhu et al., Citation2019). Further, the previous literature on ICT and institutional governance is limited to conventional linear empirical methods such as OLS and ARDL with simplified and single dimension constructs, which resultantly shaped inconsistent results. Diverse with prior studies, we claim that carbon and other hazardous emissions, technological innovation, institutional governance, and ICT adoption affect GI through several factors. These factors may have a significant effect on GI at different levels. Prior scholars identified that the economic and financial factors validate a nonlinear dynamic trend over different times, which guides an asymmetric and nonlinear interactive relationship (Shahbaz et al., Citation2018). Further, Razzaq et al. (Citation2021) recommend employing novel econometric procedures to draw unbiased and consistent outcomes.
This study outspreads the prior literature in multiple ways. First, this study explores the nexus of ICT adoption, institutional governance, environmental, economic, and social sustainability with GI by testing the hypotheses in a multidimensional framework. Secondly, this study contributes to the literature by developing and using a novel index of ICT adoption and institutional governance index, which combines multiple constructs in a single composite index to eliminate the subjectivity bias. Thirdly, this study employs a nonlinear autoregressive distribution lag (NARDL) model to check the positive and negative shock, which has not been carried out in Pakistan in this context.
2. Theoretical background and hypotheses development
2.1. Triple bottom line and green innovation
Sustainable development (SD) has achieved substantial importance among researchers and practitioners due to its growing nature about conserving natural resources. The environmentalist and ecologists have praised integrating green thinking and environmental awareness into the organisational production process to yield the benefits of intensifying SD (Sarkis et al., Citation2011). The WCED defines SD as ‘development that meets the needs of the present without compromising the ability of future generations to meet their needs’ (World Commission on Environment and Development (WCED), Citation1987). These propositions established the TBL sustainable assessment framework, and it can evaluate the SD from a multidimensional aspect (Elkington, Citation1998). These three pillars (environmental, social, and economic) of SD are acknowledged as a TBL ‘that affects the present and future generations’ (Elkington, Citation1998). Further, these elements are interdependent and symbiotic; consequently, they could be viewed as an integrative theory of sustainability (Tseng et al., Citation2015). With increasing demands for SD, organisational competing criteria have been expanded to include conventional economic, social, and environmental sustainability (Delmas & Pekovic, Citation2013). North (Citation1990) conceptualised the institutional governance framework, which states that ‘institutions are the rules of the game in a society,’ indicating that how economic indicators are performed in the presence of different institutional settings. Hojnik and Ruzzier (Citation2016) argued that institutional and regulatory are key drivers for GI at both development and dissemination stages as institutions rule the game in any society. The effectiveness of institutional and government intervention is matured through institutional sequester quality. Strong institutional capacity stimulates innovation and growth, further implementing a green growth/sustainable agenda. The assessment of sustainability and its aspects are viewed as indispensable drivers of GI. GI could mean an organisation can decrease the unfavourable impact of its operations on the natural environment (Shahzad et al., Citation2020a).
Presently, several scholars have been analyzing the factors which have a substantial impact on GI. Chen and Lee (Citation2020) found that stakeholders’ pressure is an essential element influencing GI. Environmental and technological capabilities, environmental laws and regulations, market and customer green demand also have fundamental GI determinants (Chang, Citation2016). Further, creativity, knowledge management activities, and investment in environmental management also enable SD and GI (Awan et al., Citation2021). ICT infrastructure, including innovative equipment and materials, can reduce environmental impact by reducing CO2 emissions and building smart and sustainable cities (Stucki & Woerter, Citation2019). Further, Lim et al. (Citation2017) highlighted the TBL approach’s importance for achieving SD and organisational innovation. Innovation is an impulse for modern knowledge to develop sustainable materials, green products, and cleaner production processes (Yu et al., Citation2020). Despite ample literature on GI and SD, the aspect of how the TBL approach influences these are still not adequately engrossed (Zhu et al., Citation2019). Therefore, this research hypothesises that a TBL strategy might significantly predict GI with ICT infrastructure assimilation. The conceptual model is shown below in .
2.2. Hypotheses development
2.2.1. Economic sustainability (ECO)
Financial growth and human capital development are two diverse views associated with economic sustainability (Saunila et al., Citation2018). This SD dimension is most extensively studied after the international economic depression identified the risks of bankruptcy and insolvency. Economic sustainability depends on the export intensity, financial development, market capitalisation, and trade openness of any country, further improving foreign direct investment (FDI) (Maasoumi et al., Citation2020). Accordingly, Galbreath (Citation2019) acknowledged the positive relationship between export intensity and GI. Previous studies identified that reducing production cost and GDP growth are also the key drivers of GI (Saunila et al., Citation2018). Cost-saving and recycling are the most imperative instigator for the least utilisation of energy and raw material (Aboelmaged & Hashem, Citation2019). Investment in human capital development also influences green growth in the current digital economy-boosting competitiveness. Wanzala and Zhihong (Citation2016) claimed that green logistic operations help fight climate change while improving firms’ operational and economic performance by embracing green practices. Luthra et al. (Citation2016) stressed that employing recycling principles in organisational logistics contributes to waste reduction and energy saving, strengthening a sustainable economy. Shahzad et al. (Citation2020a) acknowledged that economic sustainability positively influences GI. Recently, Aboelmaged and Hashem (Citation2019) uncovered that sustainable organisational capabilities significantly affect corporate green performance. Accordingly, the below hypothesis was further proposed.
H1. Economic Sustainability has a positive influence on Green Innovation.
2.2.2. Environment sustainability (ENV)
Different economies have diverse motivations for GI execution as natural resource reclamation is a key challenge globally. The environmental facet of SD means transmogrifying industrial and production technologies (environmental regulations, energy consumption, technological changes, and recycling) to reduce the adverse effect on ecological growth and industrialisation (Maasoumi et al., Citation2020; Saunila et al., Citation2018). Countries with lower diffusion of the eco-innovative system need to consider exploiting innovative and eco-friendly technologies that point towards the effective utilisation of resources to enhance environmental conservation (Maasoumi et al., Citation2020). Leading economies have stringent environmental policies and regulations to improve green growth. The prior researcher proposed that environmental regulations positively influence GI by endorsing public interest and investment (Steinhorst & Matthies, Citation2016). In the green technology to pollution reduction nexus, past studies stressed that R&D, technology innovation, environmental regulations and quality, and CO2 emissions are interconnected (Amin et al., Citation2020; Khan et al., Citation2019). As adoption of green technology helps to decrease energy and fuel consumption which ultimately decreases CO2 emission. Recent research by Amin et al. (Citation2020) identified that technology innovation had reduced CO2 emissions in Asian countries. For developing countries, David and Grobler (Citation2020) assessed the effect of innovative measures on CO2 emissions and found an inverse association between innovation and CO2 emissions.
Further, Santra (Citation2017) inferred a positive relationship between innovation and CO2 emissions in BRICS countries. A recent research study by Hamdoun et al. (Citation2018) identified that green process and product innovation, green organisational innovation, and ecological research and development investment could be boosted by effective environmental management systems. Likewise, existing literature acknowledged that environmental sustainability is also one of the core elements for GI exploitation and investment (Saunila et al., Citation2018). Moreover, green creativity, organisational identity, and green strategy substantially impact GI (Soewarno et al., Citation2019; Song & Yu, Citation2018). Environmental policy stringency, environmental tax, and reduction in CO2 emissions affect GI positively (Maasoumi et al., Citation2020). According to Du et al. (Citation2019), enhancing the understanding of reduced CO2 emissions on GI needs further investigation. This uncertain situation motivates us to scrutinise the environmental sustainability relationship to GI in the current circular economy. Thus, the below hypothesis was proposed.
H2. Environment Sustainability has a positive influence on Green Innovation.
2.2.3. Social sustainability (SOC)
Social sustainability is acknowledged as state efficiency regarding the environment, focussing on human capital growth, job creation, and social wellbeing (Saunila et al., Citation2018). The internal knowledge capabilities and development initiatives were supposed to significantly enhance GI (Triguero et al., Citation2013). Through environmental training, human capital development could also help employees change their attitude and behaviour towards eco-friendly actions (Awan et al., Citation2021). Extant literature revealed that citizens’ environmental knowledge affects community wellbeing, social support, financial growth, and profitability (Delmas & Pekovic, Citation2013). Nevertheless, human capital development and internal learning stimulate the process of GI. Previous researchers have exhaustively studied the direct association between sustainable practice and GI (Chang, Citation2016; Hojnik & Ruzzier, Citation2016; Triguero et al., Citation2013). The development of Industry 4.0 is besides necessary, given its effect on the workforce and how work is industrialized, which can engender projections for growth and productivity (David & Grobler, Citation2020). In the social sustainability context, the influence of GI can be realised through variation in behaviour and attitude, especially by driving towards technology adoption for mutual cooperation consumption (Ganapathy et al., Citation2014). Consumers are also keen to pay more for GI and eco-friendly products to increase ecological performance through energy saving, minimising waste, and reducing natural hazards (Song & Yu, Citation2018). According to Maasoumi et al. (Citation2020), the welfare index, including investment in education, mortality rate, and R&D, positively affects GI. Prior literature recognised that social sustainability positively influences GI exploitation and investment (Saunila et al., Citation2018). It is determined that organisational requirements, green consumer demand, human capital, and internal knowledge capabilities influence GI. Therefore, the below hypothesis is proposed.
H3. Social Sustainability has a positive influence on Green Innovation.
2.2.4. Institutional sustainability (INST)
Institutional governance is the key driver for environmental quality and innovation in institutional theory (Azam et al., Citation2021). Several researchers have focussed on decent institutional governance and its importance for underdeveloped and developing countries after highlighting the lesser reassurance for productivity and profitability (Dasgupta & Cian, Citation2018). Further, the previous researcher identified some key factors such as creative entrepreneurs, knowledge improvement and its diffusion, market formation, and its growing influence on the productivity of innovative systems in the context of technologies and energy consumption while emphasising the necessity of efficient institutions and the rule of law at the core of influencing innovation (Dasgupta & Cian, Citation2018). North (Citation1990) established that the quality and structure of institutional have a substantial effect on innovation. Weak institutional structures upsurge uncertainty and are likely to harm innovation. Simultaneously, strong institutions may accelerate new patents, diffusion of knowledge, enforcement of laws, and uncertainty reduction (Azam et al., Citation2021). Extant literature recommended that improved institutions are likely to support GI (Aboelmaged & Hashem, Citation2019). Previous literature evaluates the impact of institutional quality on CO2 emissions across 47 developing countries. The results revealed that institutional quality matters for environmental growth and innovation. Regulation and its practical implementation by the institutional are the critical drivers for GI (Saunila et al., Citation2018). Thus, institutional sustainability is playing a magnificent role in achieving green growth outcomes through GI. Therefore, the following hypothesis is proposed.
H4. Institutional Sustainability has a positive influence on Green Innovation.
3. Materials and methods
3.1. Data description
The current study estimates the asymmetric impact of the human capital index (HCI), carbon dioxide (CO2) emissions, Information and communication technologies (ICT) adoption, institutional governance (INST), and GDP on green innovation (GI) in Pakistan using the NARDL model. The study contains two novel indicators: the ICT adoption index, institutional governance quality index, and TBL factors for Pakistan. Following Razzaq et al. (Citation2021); Shahzad et al. (Citation2017), this research employs quarterly data from 1996Q1 to 2019Q4 containing 92 observations. The ICT adoption constructs data contains mobile subscriptions per 100 people and percent of the population using internet retrieved from World development indicators (WDI). The institutional governance quality construct has government effectiveness, control of corruption, regulatory quality, the rule of law, voice and accountability, political stability, and absence of violence retrieved from World governance indicators (WGI). The details of the constructed index are given in sections 3.1.1 and 3.1.2.
For this study, we used environment-related technologies as a percentage of total technologies as a proxy of GI, GDP (USD Constant 2010), human capital index (Average years of schooling and returns to education) from Penn World Table, and carbon emissions (Metric tons per capita) were extracted from the Global Atlas Database. Data of all variables are transformed into log10 except indexes. For empirical estimation, we used EViews version 10. Further descriptions of data and sources are shown in .
Table 1. Data narrative.
Appendix A & B demonstrate the scatter plot and data distribution at the end, indicating a positive association between the HCI, CO2, ICT, INST, GDP, and GI shows the descriptive statistics of each variable, including mean, median, and skewness. Further, Jarque-Bera (JB) test results show that data distribution is not normal except INST rejecting the null hypothesis (Data is normally distributed). Thus, it is imperative to use the NARDL model.
Table 2. Descriptive statistics.
3.1.1. ICT adoption
Current literature has been hindered by the absence of appropriate technology adoption measures, which failed to practice comprehensive and quantifiable technology development measures (Márquez-Ramos & Martínez-Zarzoso, Citation2010). Thus, we constructed a cumulative index using Principal Component Analysis (PCA) by integrating different indicators such as mobile subscriptions per 100 people and percent of the population using the internet to capture the combined effect of ICT (Razzaq et al., Citation2021).
3.1.2. Institutional governance index
This study constructed a composite index of institutional quality through PCA by integrating all main governance measures such as government effectiveness, control of corruption, regulatory quality, the rule of law, voice and accountability, political stability, and absence of violence scaled from minus 2.5 to plus 2.5 lower to higher, respectively. It helps us to integrate the cumulative effect of institutional governance structure (Razzaq et al., Citation2021).
3.1.3. PCA index of ICT adoption and institutional governance
A brief explanation of the PCA index for ICT adoption and Institutional governance is given in and . The eigenvalue of the first principal component (PC) exceeds the one that implies the PC’s significance. For conciseness, the estimation of eigenvalues for second and third PCs is omitted by following the Kaiser criterion. The primary factor’s importance is anticipated by the significant % of the variance within the data that covered the first PC. The first PC individually illustrates more than 90% and 65% of the total variation for ICT adoption and Institutional governance, respectively.
Table 3. PCA index: ICT adoption.
Table 4. PCA index: INST quality.
3.2. Empirical methodology (nonlinear auto regressive distributed lag model - NARDL)
We employed a nonlinear autoregressive distributed lag model (NARDL) established by Shin et al. (Citation2014) to capture probable asymmetries that ascend due to positive and negative shocks in the human capital index CO2 emissions, ICT adoption. It is the extension of ARDL. Normally, this approach encompasses a dynamic error correction description that authorises clutching asymmetries that ascend because of shocks in targeted variables in both the long and short-run (Mensi et al., Citation2018). First, we propose the following linear equation.
(1)
(1)
Where HCI represents the human capital index, ICT represents ICT adoption, INST represents institutional governance index, CO2 emissions represent carbon dioxide emissions, and GDP represents per capita gross domestic product in Pakistan. These relationships carried out the linear relationship between the variables; however, this study’s main objective is to study the nonlinear association among these variables by employing the NARDL model presented by Shin et al. (Citation2014). This approach is flexible and straightforward since variables are not required to be stationary at the same level. It can also be applied regardless of the order of integration, except that the series is not integrated at I (2) (Granger & Yoon, Citation2002). Moreover, Granger and Yoon (Citation2002) proposed the hidden co-integration concept, by which co-integration relationships may be defined as the positive and negative constituents of underlying variables. Following the empirical work of Maasoumi et al. (Citation2020); Rahman et al. (Citation2019), accounting for the asymmetric relationship among the target construct, our model can be specified as;
(2)
(2)
Where θi is associated with the long-run parameters, and the asymmetric effect of HCI, CO2, and ICT is combined with a partial sum of the square of positive and negative changes. The impact of the projected variables, in the long-run, is mentioned in the above equation, and a positive sign indicates a positive shock, while a negative sign indicates a negative shock. For a detailed derivation of the NARDL model, please refer to Ling et al. (Citation2021).
4. Results and discussion
Before pursuing parameters estimation, it is imperative to perform a preliminary test to confirm data properties. Due to structural breaks identified in data, the BDS test for nonlinearity applies as a follow-up of the Jarque-Bera (JB) test. The BDS test’s z statistics confirmed the rejection of data normality at m = 2 to m = 6 at a 1% level of significance, indicating that all variables followed asymmetricity and non-linearity. Therefore, the NARDL model was preferred and most appropriate, which can cater structural breaks.
4.1. Unit root tests
Before analyzing the NARDL model, the affirmation of stationary characteristics of the variables is essential. This study primarily employs Augmented Dickey-Fuller (ADF) and Zivot and Andrews (Citation1992) (ZA) unit root test, which were given in . Both tests approve that all variables are stationary at the first difference with a 1% level of significance except HCI in the ZA test. ZA test is superior as it provides details of a structural break in data. ZA test suggests structural breaks (i.e. 2004Q1 (CO2), 2002Q1 (ICT), 2003Q4 (INST), and 2007Q1 (GDP)), which indicated that the targeted variables are affected by non-stationarity. These structural breaks are attributed to financial crises, technology reforms, and democracy regime shifts in Pakistan. Further, data non-normality guides us to apply the NARDL model, which can efficiently deal with structural breaks and dynamic stochastic tendencies (Rahman et al., Citation2019).
Table 5. Empirics for unit root tests.
4.2. Long-run results
The long-run results are reported in , suggesting that any positive shock in HCI stimulates GI by 1.0451%. In contrast, any negative shock in HCI undermines GI by 0.9347%. Interestingly, positive shocks are more persistent than negative ones, implying that adequate human capital and higher educational achievement lead to higher innovation performance by simultaneously setting an innovation base. In contrast, if any negative shock happens in HCI, then the marginal reduction in GI is less than the former due to the existing knowledge stock in the economy and society. This study signifies the result of previous studies, which argue that HCI improvement reinforces technological innovation (Hu, Citation2021; Marvel et al., Citation2020). The results infer that the countries with well-developed and upgraded HCI (education, R&D, skills, and experience) can reap significant benefits from knowledge spill-over, mainly by employing new technologies which ultimately enhance total factor productivity and economic growth. The human capital theory also specifies that individuals vary in knowledge, skills, and experience, which have an unequal effect on economic growth (Marvel et al., Citation2020).
Table 6. Dynamic estimation of NARDL results (long-run coefficients).
Further, a positive shock in CO2 emissions increases GI by 0.6308%, while any negative shock in CO2 emissions undermines GI by 0.0121%. Positive shock in emissions is attributed to higher economic growth or boom period in any economy as a directly proportional relationship exists between both variables. At the full peak of economic output, carbon emissions are also higher as a residual of growth. Thus, higher emissions create social pressure and provoke respective governments to take appropriate policy measures in higher R&D allocation and environmental regulations, translating into higher GI in the long-run. Ahmad et al. (Citation2021) identified that positive shock or change in innovation decrease CO2 emissions. On the other hand, our results signify that any negative shock in CO2 emissions leads to increased GI. Following the same rationality, negative shocks in CO2 are mainly attributed to uneven situations such as financial crises, pandemic situations, natural disasters, and other stringency measures that halt economic activities.
Further, in economic downfall, the government injects funds and resources for firms and industries to innovate and find efficient solutions to improve their growth by investing in new technologies. Moreover, new entrant vacillates to use the latest and costly technologies to minimise CO2 emissions at the initial level. Government support (tax rebate) is essential to elevate stakeholders’ confidence to generate economic activity for growth and development. Endogenous growth theory also postulates the same, which indicates that innovation endogenously improves economic output. Our study offered that relevant environmental policies and regulations have been made to scent firms to develop specific technologies or promote innovations and direct technological changes towards cleaner technologies. IPAT model also relates sustainable growth outputs with technological innovation, which supports our results. Additionally, technology stock (ICT capital) accumulates over time, and any negative shock in environmental indicators cannot instantly change GI. The same can be endorsed from our short-run results, where negative shock in CO2 emissions shows an insignificant effect on GI. Moreover, in today's world, investment in R&D, efficient energy solutions, digital manufacturing, process and product innovation is considered an alternative solution to give a big push to the economy, which can further reinforce GI (Song & Yu, Citation2018). Compliance with relevant environmental standards and regulations is the most critical factor to enhance efficiency and reduce the cost of innovation.
Third, positive shock in ICT stimulates GI by 0.5599%, while any negative shock in ICT undermines GI by 0.7824%. Through the digital revolution, the association between ICT and innovation has been relatively complex. Positive shocks in Pakistan are mainly attributed to 2002 and afterward, representing the installation and dissemination of ICT infrastructure (optical fibres, mobile towers, and computers). Improved ICT penetration increased local firms’ connectivity with global leaders, which helped them imitate global technologies virtually. Regarding the sources of innovation, prior studies consider ICT one of the driving factors of innovation (Higón, Citation2012). ICT influences GI by producing novel IT apparatuses that consume less energy consumption and reduce environmental pollution (Chen & Lee, Citation2020). Further, the adoption of ICT in the R&D process exerts positive pressure on GI outcomes. Using ICT in various departments is mainly 23% more likely to introduce new and innovative processes (Higón, Citation2012). Industrial production through new processes and energy saving module reduces energy and fuel consumption, which significantly boosts GI. These facts provide significant support to our results.
Furthermore, the institutional governance index also has a significant and positive effect on GI, which strongly specified that GI increases due to institutional quality improvement and stability. Improvement and stability of INST encompass adequate security and political stability, which have been a significant challenge in Pakistan. Though our results are substantial, continuous steps should be taken to improve the quality of INST, which will have a long-run positive impact on overall economic conditions and sustainable growth. Our results align with Saunila et al. (Citation2018), which found institutional sustainability (legislation and regulations) was operative for GI investment and exploitation, ultimately supporting sustainable development.
Lastly, GDP has a significant and positive effect on GI because higher GDP is evidence of higher productivity of labour and ability to sustain the potential of advanced technology development which offices GI. These results adhered to Hu (Citation2021) prior findings, who identified GDP stimulates technological innovation through higher R&D allocations and human capital development. However, Maasoumi et al. (Citation2020) identified a negative relationship between GDP and GI. The increased cost of innovation may explain this adverse effect. To overcome these deficiencies and minimise the innovation cost, such activities are outsourced or located in emerging economics. From these outcomes, we can conclude that the factors that regulate GI in Pakistan exert a nonlinear and asymmetric effect. Although short-run results () indicate a similar direction of relationship; however lower in magnitude, implying that the deriving factors of GI are more profound in the long-run than the short-run. It has theoretical rationality that GI is a long-term process. Moreover, the negative coefficient (66%) of the error correction term (ECT) confirms the convergence of long-run equilibrium in case of any shock.
Table 7. Short-run asymmetric relationship.
Further, the bounds test presented in confirmed the existence of a cointegrating relationship among the targeted variables as the F-statistics value is greater than the critical value of 5 percent. The Wald test also confirmed the presence of long-term asymmetric relationships. The serial correlation was checked through the LM test, heteroscedasticity was checked through Breusch-Pagan Godfrey (BPG) test, and Ramsey reset test. The outcomes of these tests recommended that the computed model was free from autocorrelation and heteroscedasticity. Furthermore, the cumulative sum of recursive residuals (CUSUM) and the square of CUSUM were used to examine the stability of the parameters used in the model.
Table 8. Diagnostic inspection.
provides the summary of our core hypotheses, which confirmed that the TBL approach positively affects the green growth agenda through GI.
Table 9. Hypotheses results.
5. Conclusion and policy implications
5.1. Conclusion
The world is challenged by climate change and global warming-induced by environmental pollution and hazardous emissions in the current era. The current research is the pioneer in evaluating the effect of sustainable practices (environmental, economic, social, and institutional) with the ICT revolution for SD to pursue GI outcomes. This study primary aim was to examine what drives GI in terms of the TBL and ICT adoption from 1996 to 2019 by using a novel NARDL approach in Pakistan. This study employed the novel indexes of ICT adoption and institutional governance, which integrates the effects of multiple constructs into a single composite index using PCA. This study extends the literature on GI by using a nonlinear methodology that allows examining the potential asymmetric impact in both long and short-run spheres, which further helps to test the positive and negative shocks by employing the NARDL model. The results indicate that positive shock in HCI significantly positively affects GI (1.0451%) in the long-run, but the negative shock doesn’t undermine with the same magnitude. Secondly, a positive shock in CO2 emissions excites GI (0.6308%), but the negative shock doesn’t significantly harm the GI. As GI is an ongoing process, it can’t decrease readily after achieving a certain innovation threshold level. These results are vital because any policy that intends to reduce CO2 emissions will affect the innovation process and provide the solution to improve environmental quality and economic growth. Third, a positive shock in ICT adoption positively affects GI (0.5599%), while any negative shock in ICT emasculates GI (0.7824%). As ICT is a double-edged sword, growth in ICT adoption positively affects the innovation process, while negative shock adversely affects the environment by increasing disposal. Moreover, institutional governance quality plays a significant role in achieving GI economic growth and sustainable development outcomes. Further, GDP per capita positively affects GI, which confirms prior research results (Rahman et al., Citation2019); sustainable environmental strategies have a vital role in economic growth. The GI dream will never come true without adopting sustainable practices (environmental, economic, social, and institutional) and the latest ICT technologies.
5.2. Research implications
From the policy perspective, the current study’s outcomes help us respond to the complicated query, the critical role of sustainable practices, institutional governance, and ICT adoption for GI in the forthcoming years. First, human capital and individuals’ welfare has been acknowledged as the essential drivers of economic growth. Organisations should provide favourable working conditions and policies to fulfil their potential while encouraging them to acquire more advanced knowledge through education and training. More investment should be allocated for R&D in education and skill-building regarding GI. The strategists must implement diverse approaches to balance supply and demand for resources to maintain the environment quality. Thus, government and policymakers should focus on bridging the gap among resources, competencies, and goals.
Second, to reap the benefits of the sustainable development plan 2030, innovation strategy and the latest ICT adoption must be aligned. Underdeveloped economies such as Pakistan must undertake technological and GI to compete with developed economies in the current era. As Awan et al. (Citation2021) specified, sustainable practices improve GI by assimilating innovative and cleaner production technologies. Auxiliary, innovative technologies ensure appropriate raw material consumption, prevent environmental pollution, and enhance competitive edge. Adopting sustainable technologies that transform garbage into energy can substitute fossil fuels and reduce environmental pollution and hazardous emissions needed in the digital era. It further addressed the ecological and economic development objectives, GI, and ICT development together. The policymaker can improve the awareness of eco-friendly processes in ICT adoption.
Third, considering environmental deprivation, the Pakistani government should support and efficiently work on green business environment programs and facilitate the organisations to lessen their dependency on fossil fuel and exploit renewable energy resources (World Bank, Citation2018). That will surge the environmental awareness among the industries and enhance economic growth. Additionally, with the support of the business community government of Pakistan should complete the plantation of over a billion tree Tsunami to enhance the beauty of the natural environment, air quality and to promote a sustainable climate culture in Pakistan (World Economic Forum, Citation2018), which is prerequisite for green transformation. For example, developed countries like China have achieved rapid economic growth while suffering from severe ecological pollution and resource exhaustion (Zhu et al., Citation2010). Lately, they have recognised the importance of GI-related to significant environmental challenges and stringent environmental laws. Last but not least, effective and efficient formulation and implementation of laws, rules, and regulations are beneficial to provide stable and consistent political support for sustainable development.
Concludingly, an integrated policy is imperious to implement a green growth agenda at the national level, where sequester institutions aligned other socio-economic objectives of Pakistan, such as ICT adoption and penetration that may reinforce growth and translate into green technologies. This research unveils the association between HCI, CO2, ICT, INST, GDP, and GI in Pakistan. Future researchers can observe these relationships in other countries and specify the policies to execute in their countries and areas to ensure sustainability. They can examine the targeted variables among other Asian countries using panel data to widen the prospects further.
Disclosure statement
No potential conflict of interest was reported by the authors.
Additional information
Funding
References
- Aboelmaged, M., & Hashem, G. (2019). Absorptive capacity and green innovation adoption in SMEs: The mediating effects of sustainable organisational capabilities. Journal of Cleaner Production, 220, 853–863. https://doi.org/10.1016/j.jclepro.2019.02.150
- Ahmad, M., Khan, Z., Rahman, Z. U., Khattak, S. I., & Khan, Z. U. (2021). Can innovation shocks determine CO2 emissions (CO2e) in the OECD economies? A new perspective. Economics of Innovation and New Technology, 30(1), 89–109. https://doi.org/10.1080/10438599.2019.1684643
- Amin, A., Aziz, B., & Liu, X. H. (2020). The relationship between urbanization, technology innovation, trade openness, and CO2 emissions: Evidence from a panel of Asian countries. Environmental Science and Pollution Research International, 27(28), 35349–35363. https://doi.org/10.1007/s11356-020-09777-y
- An, H., Razzaq, A., Nawaz, A., Noman, S. M., & Khan, S. A. R. (2021). Nexus between green logistic operations and triple bottom line: evidence from infrastructure-led Chinese outward foreign direct investment in Belt and Road host countries. Environmental Science and Pollution Research, 28, 51022–51045.
- Awan, U., Arnold, M. G., & Golgeci, I. (2021). Enhancing green product and process innovation: Towards an integrative framework of knowledge acquisition and environmental investment. Business Strategy and the Environment, 30(2), 1213–1283. https://doi.org/10.1002/bse.2684
- Azam, M., Liu, L., & Ahmad, N. (2021). Impact of institutional quality on environment and energy consumption: Evidence from developing world. Environment, Development and Sustainability, 23(2), 1646–1667. https://doi.org/10.1007/s10668-020-00644-x
- Chang, C.-H. (2016). The determinants of green product innovation performance. Corporate Social Responsibility and Environmental Management, 23(2), 65–76. https://doi.org/10.1002/csr.1361
- Chen, Y., & Lee, C. C. (2020). Does technological innovation reduce CO2 emissions? Cross-country evidence. Journal of Cleaner Production, 263, 121550. https://doi.org/10.1016/j.jclepro.2020.121550
- Dasgupta, S., & Cian, E. D. (2018). The influence of institutions, governance, and public opinion on the environment: Synthesized findings from applied econometrics studies. Energy Research & Social Science, 43, 77–95. https://doi.org/10.1016/j.erss.2018.05.023
- David, O. O., & Grobler, W. (2020). Information and communication technology penetration level as an impetus for economic growth and development in Africa. Economic Research-Ekonomska Istraživanja, 33(1), 1394–1418. https://doi.org/10.1080/1331677X.2020.1745661
- Delmas, M. A., & Pekovic, S. (2013). Environmental standards and labor productivity: Understanding the mechanisms that sustain sustainability. Journal of Organizational Behavior, 34(2), 230–252. https://doi.org/10.1002/job
- Du, K., Li, P., & Yan, Z. (2019). Do green technology innovations contribute to carbon dioxide emission reduction? Empirical evidence from patent data. Technological Forecasting and Social Change, 146, 297–303. https://doi.org/10.1016/j.techfore.2019.06.010
- Elkington, J. (1998). Partnerships from Cannibals with Forks: The triple bottom line of 21st century business. Environmental Quality Management, 8(1), 37–51. https://doi.org/10.1002/tqem.3310080106
- Galbreath, J. (2019). Drivers of green innovations: The impact of export intensity, women leaders, and absorptive capacity. Journal of Business Ethics, 158(1), 47–61. https://doi.org/10.1007/s10551-017-3715-z
- Ganapathy, S. P., Natarajan, J., Gunasekaran, A., & Subramanian, N. (2014). Influence of eco-innovation on Indian manufacturing sector sustainable performance. International Journal of Sustainable Development & World Ecology, 21(3), 198–209. https://doi.org/10.1080/13504509.2014.907832
- Global Innovation Index. (2018). Key findings report. https://www.globalinnovationindex.org/about-gii#keyfindings
- Granger, C. W. J., & Yoon, G. (2002). Hidden cointegration. University of California. https://doi.org/10.2139/ssrn.313831
- Hamdoun, M., Chiappetta Jabbour, C. J., & Ben Othman, H. (2018). Knowledge transfer and organizational innovation: Impacts of quality and environmental management. Journal of Cleaner Production, 193, 759–770. https://doi.org/10.1016/j.jclepro.2018.05.031
- Higón, D. A. (2012). The impact of ICT on innovation activities: Evidence for UK SMEs. International Small Business Journal: Researching Entrepreneurship, 30(6), 684–699. https://doi.org/10.1177/0266242610374484
- Hojnik, J., & Ruzzier, M. (2016). What drives eco-innovation? A review of an emerging literature. Environmental Innovation and Societal Transitions, 19, 31–41. https://doi.org/10.1016/j.eist.2015.09.006
- Hu, G. (2021). Is knowledge spillover from human capital investment a catalyst for technological innovation? The curious case of fourth industrial revolution in BRICS economies. Technological Forecasting and Social Change, 162, 120327. https://doi.org/10.1016/j.techfore.2020.120327
- Khan, Z., Sisi, Z., & Siqun, Y. (2019). Environmental regulations an option: Asymmetry effect of environmental regulations on carbon emissions using non-linear ARDL. Energy Sources, Part A: Recovery, Utilization and Environmental Effects, 41(2), 137–155. https://doi.org/10.1080/15567036.2018.1504145
- Lim, M. K., Tseng, M.-L L., Tan, K. H., & Bui, T. D. (2017). Knowledge management in sustainable supply chain management: Improving performance through an interpretive structural modelling approach. Journal of Cleaner Production, 162, 806–816. https://doi.org/10.1016/j.jclepro.2017.06.056
- Ling, G., Razzaq, A., Guo, Y., Fatima, T., & Shahzad, F. (2021). Asymmetric and time-varying linkages between carbon emissions, globalization, natural resources and financial development in China. Environment, Development and Sustainability, 1–29. https://doi.org/10.1007/s10668-021-01724-2
- Luthra, S., Garg, D., & Haleem, A. (2016). The impacts of critical success factors for implementing green supply chain management towards sustainability: An empirical investigation of Indian automobile industry. Journal of Cleaner Production, 121, 142–158. https://doi.org/10.1016/j.jclepro.2016.01.095
- Maasoumi, E., Heshmati, A., & Lee, I. (2020). Green innovations and patenting renewable energy technologies. Empirical Economics, 60(1), 1–26. https://doi.org/10.1007/s00181-020-01986-1
- Márquez-Ramos, L., & Martínez-Zarzoso, I. (2010). The effect of technological innovation on international trade. A nonlinear approach. Economics, 4(2010-11), 1–37. https://doi.org/10.5018/economics-ejournal.ja.2010-11
- Marvel, M. R., Wolfe, M. T., & Kuratko, D. F. (2020). Escaping the knowledge corridor: How founder human capital and founder coachability impacts product innovation in new ventures. Journal of Business Venturing, 35(6), 106060. https://doi.org/10.1016/j.jbusvent.2020.106060
- Mensi, W., Hussain Shahzad, S. J., Hammoudeh, S., & Al-Yahyaee, K. H. (2018). Asymmetric impacts of public and private investments on the non-oil GDP of Saudi Arabia. International Economics, 156, 15–30. https://doi.org/10.1016/j.inteco.2017.10.003
- North, D. C. (1990). Institutions, institutional change and economic performance. Cambridge University Press.
- Rahman, Z. U., Chongbo, W., & Ahmad, M. (2019). An (a)symmetric analysis of the pollution haven hypothesis in the context of Pakistan: a non-linear approach. Carbon Management, 10(3), 227–239. https://doi.org/10.1080/17583004.2019.1577179
- Razzaq, A., Sharif, A., Ahmad, P., & Jermsittiparsert, K. (2021). Asymmetric role of tourism development and technology innovation on carbon dioxide emission reduction in the Chinese economy: Fresh insights from QARDL approach. Sustainable Development, 29(1), 118–176. https://doi.org/10.1002/sd.2139
- Razzaq, A., Sharif, A., Najmi, A., Tseng, M. L., & Lim, M. K. (2021). Dynamic and causality interrelationships from municipal solid waste recycling to economic growth, carbon emissions and energy efficiency using a novel bootstrapping autoregressive distributed lag. Resources, Conservation and Recycling, 166, 105372. https://doi.org/10.1016/j.resconrec.2020.105372
- Santra, S. (2017). The effect of technological innovation on production-based energy and CO2 emission productivity: Evidence from BRICS countries. African Journal of Science, Technology, Innovation and Development, 9(5), 503–512. https://doi.org/10.1080/20421338.2017.1308069
- Sarkis, J., Zhu, Q., & Lai, K. H. (2011). An organizational theoretic review of green supply chain management literature. International Journal of Production Economics, 130(1), 1–15. https://doi.org/10.1016/j.ijpe.2010.11.010
- Saunila, M., Ukko, J., & Rantala, T. (2018). Sustainability as a driver of green innovation investment and exploitation. Journal of Cleaner Production, 179, 631–641. https://doi.org/10.1016/j.jclepro.2017.11.211
- Shahbaz, M., Lahiani, A., Abosedra, S., & Hammoudeh, S. (2018). The role of globalization in energy consumption: A quantile cointegrating regression approach. Energy Economics, 71, 161–170. https://doi.org/10.1016/j.eneco.2018.02.009
- Shahzad, M., Qu, Y., Javed, S., Zafar, A., & Rehman, S. (2020a). Relation of environment sustainability to CSR and green innovation: A case of Pakistani manufacturing industry. Journal of Cleaner Production, 253, 119938. https://doi.org/10.1016/j.jclepro.2019.119938
- Shahzad, M., Qu, Y., Zafar, A. U., Ding, X., & Rehman, S. U. (2020b). Translating stakeholders’ pressure into environmental practices - The mediating role of knowledge management. Journal of Cleaner Production, 275, 124163. https://doi.org/10.1016/j.jclepro.2020.124163
- Shahzad, M., Qu, Y., Zafar, A. U., Rehman, S. U., & Islam, T. (2020c). Exploring the influence of knowledge management process on corporate sustainable performance through green innovation. Journal of Knowledge Management, 24(9), 2079–2106. https://doi.org/10.1108/JKM-11-2019-0624
- Shahzad, S. J. H., Shahbaz, M., Ferrer, R., & Kumar, R. R. (2017). Tourism-led growth hypothesis in the top ten tourist destinations: New evidence using the quantile-on-quantile approach. Tourism Management, 60, 223–232. https://doi.org/10.1016/j.tourman.2016.12.006
- Sharif, A., Raza, S. A., Ozturk, I., & Afshan, S. (2019). The dynamic relationship of renewable and nonrenewable energy consumption with carbon emission: A global study with the application of heterogeneous panel estimations. Renewable Energy, 133, 685–691. https://doi.org/10.1016/j.renene.2018.10.052
- Shin, Y., Yu, B., & Greenwood-Nimmo, M. (2014). Modelling asymmetric cointegration and dynamic multipliers in a nonlinear ARDL framework. In R. Sickles & W. Horrace (Eds.), Festschrift in Honor of Peter Schmidt (pp. 281–314). Springer. https://doi.org/10.1007/978-1-4899-8008-3_9
- Soewarno, N., Tjahjadi, B., & Fithrianti, F. (2019). Green innovation strategy and green innovation: The roles of green organizational identity and environmental organizational legitimacy. Management Decision, 57(11), 3061–3078. https://doi.org/10.1108/MD-05-2018-0563
- Song, W., & Yu, H. (2018). Green innovation strategy and green innovation: The roles of green creativity and green organizational identity. Corporate Social Responsibility and Environmental Management, 25(2), 135–150. https://doi.org/10.1002/csr.1445
- Steinhorst, J., & Matthies, E. (2016). Monetary or environmental appeals for saving electricity? Potentials for spillover on low carbon policy acceptability. Energy Policy, 93, 335–344. https://doi.org/10.1016/j.enpol.2016.03.020
- Stucki, T., & Woerter, M. (2019). The private returns to knowledge: A comparison of ICT, biotechnologies, nanotechnologies, and green technologies. Technological Forecasting and Social Change, 145, 62–81. https://doi.org/10.1016/j.techfore.2019.05.011
- Sun, Y., Duru, O. A., Razzaq, A., & Dinca, M. S. (2021). The asymmetric effect eco-innovation and tourism towards carbon neutrality target in Turkey. Journal of Environmental Management, 299, 113653.
- Triguero, A., Moreno-Mondéjar, L., & Davia, M. A. (2013). Drivers of different types of eco-innovation in European SMEs. Ecological Economics, 92, 25–33. https://doi.org/10.1016/j.ecolecon.2013.04.009
- Tseng, M. L., Lim, M., & Wong, W. P. (2015). Sustainable supply chain management: A closed-loop network hierarchical approach. Industrial Management & Data Systems, 115(3), 436–461. https://doi.org/10.1108/IMDS-10-2014-0319
- United Nations. (2018). Transforming our world: The 2030 agenda for sustainable development. Transforming our world: The 2030 agenda for sustainable development.
- Wanzala, W. G., & Zhihong, J. (2016). Integration of the extended gateway concept in supply chain disruptions management in East Africa-Conceptual paper. International Journal of Engineering Research in Africa, 20, 235–247. https://doi.org/10.4028/www.scientific.net/JERA.20.235
- World Bank. (2018). World Bank project: Punjab green development program. Retrieved March 2, 2021, from https://projects.worldbank.org/en/projects-operations/project-detail/P165388?lang%2525C2%2525BCen=
- World Commission on Environment and Development (WCED). (1987). Our common future. Oxford University Press.
- World Economic Forum. (2018). Pakistan has planted over a billion trees. World Economic Forum. Retrieved March 2, 2021, from https://www.weforum.org/agenda/2018/07/pakistan-s-billion-tree-tsunami-is-astonishing/
- Yu, W., Chavez, R., Jacobs, M., & Wong, C. Y. (2020). Innovativeness and lean practices for triple bottom line: testing of fit-as-mediation versus fit-as-moderation models. International Journal of Operations & Production Management, 40(10), 1623–1647. https://doi.org/10.1108/IJOPM-07-2019-0550
- Zhuang, Y., Yang, S., Razzaq, A., & Khan, Z. (2021). Environmental impact of infrastructure-led Chinese outward FDI, tourism development and technology innovation: A regional country analysis. Journal of Environmental Planning and Management, 1–33. https://doi.org/10.1080/09640568.2021.1989672
- Zhu, Q., Geng, Y., & Lai, K. h. (2010). Circular economy practices among Chinese manufacturers varying in environmental-oriented supply chain cooperation and the performance implications. Journal of Environmental Management, 91(6), 1324–1331. https://doi.org/10.1016/j.jenvman.2010.02.013
- Zhu, Q., Zou, F., & Zhang, P. (2019). The role of innovation for performance improvement through corporate social responsibility practices among small and medium-sized suppliers in China. Corporate Social Responsibility and Environmental Management, 26(2), 341–350. https://doi.org/10.1002/csr.1686
- Zivot, E., & Andrews, D. W. K. (1992). Further evidence on the great crash, the oil-price shock, and the unit-root hypothesis. Journal of Business and Economic Statistics, 10(3), 251–270. https://doi.org/10.1080/07350015.1992.10509904