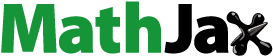
Abstract
The ongoing climate changes have created various challenges for each industry to achieve sustainable environmental goals. Like other extensively growing industries, China's film and drama industry and inbound tourism are also responsible for contributing effectively toward a sustainable environment. Despite its significant importance, there is a dearth of empirical evidence that analyses these industry's environmental performance. Thus, this study fills the gap by applying the novel bootstrap ARDL approach and found that Inbound Tourism and Film and Drama industry increased environmental performance (reducing ecological footprint) in China. These results imply that entertainment industries and inbound tourism increased social awareness and encouraged environmental protection in societies and industries. Manifestly, the coefficient's magnitude of the Film and Drama industry is relatively lower due to a smaller share of the overall economy. However, it shows significant potential for sustainability by creating awareness about sustainable practices and corporate social responsibility, leading to higher environmental performance. Moreover, a feedback effect (two-way causality) exists between Film and Drama industry, Inbound Tourism, and environmental performance. These results offer valuable policy recommendations.
1. Introduction
Due to the regular increase in the global temperature, human life and health is also getting affected because of the un-wanted modifications to the global atmospheric conditions, leading to the restrictions by the people in accessing quality food, water, and crops, which also worsen the respective use of soil and land for the production and reproduction (Danish & Wang, Citation2019; Zhuang et al., Citation2021). Environmental researchers have urged to safeguard nature, fauna and flora. In contrast, if neglected, there will be an abnormality in the rainfalls, droughts, storms, etc., which is also expected to be more lethal and destructive because of the imbalance caused to the environment (Guo et al., Citation2021; Razzaq et al., Citation2021; Sharif et al., Citation2020). Such imbalance leading to destruction can be controlled by the reduction and the just elimination of pollution (Danish et al., Citation2019; Najmi et al., Citation2019), which itself is extremely difficult because of the ongoing continuation of the traditional operational processes and policies (Ahmed et al., Citation2018; Godil et al., Citation2021; Sharif et al., Citation2021).
Among the few potential solutions that could contribute to environmental conservation by eliminating pollution is to create awareness among the masses and the public (Khan et al., Citation2019a, Citation2021; Najmi et al., Citation2021a). This is because the initiatives for environment preservation is not only the extended responsibility of the manufacturers where they should take care of the environment not only in the production stage but also after the product’s consumption phase (Khan et al., Citation2019b; Najmi et al., Citation2021b). It is also the responsibility of the consumers to have responsible consumption (Najmi et al., Citation2021a). Through this, not just the organizations can have optimized consumption of resources (Najmi & Khan, Citation2017), but the individuals from the general public can also play their part for environment protection (Najmi et al., Citation2019; Zhang et al., Citation2021).
For creating awareness in the masses and public, the potential of the role and the significance of contribution by the film and drama industry (FI) cannot be ignored (Chen & Valdmanis, Citation2019; Lopera-Mármol & Jiménez-Morales, Citation2021). They are considered the ear and mouth of society as they highlight the issue that requires attention and consideration of the institutions and play the role of the watchdog of society and people. However, the FI itself plays a dual role in the society. Firstly, the role of the watchdog is already discussed, and secondly, the consumer of the resources, which is of significant importance (Lopera-Mármol & Jiménez-Morales, Citation2021). It is a known fact that in this industry, every project is different with a different storyline, genre and script involving characters played by the individuals with different measurements and dimensions, thus this industry potentially consumes large amount of resources than any other industry operating with similar size and scale (Liu, Citation2020).
Secondly, being the consumer of the resources, the FI is found to be involved in the practices that waste the resources and but also cause and account for severe damage to the environment. For instance, the FI is found in abusing the resources in several ways, including excessive making of costumes, extra utilization of fuels, electricity, and any other type of energy resources, utilization of decorations, props, and sets which are made up of material and resources that are non-recyclable in nature, etc. however, these violations and abuses are majority of times get un-noticed and unheeded (Lopera-Mármol & Jiménez-Morales, Citation2021). On the other hand, certain examples have documented the possibilities of optimizing the resources and conserving the environment by adopting and implementing green practices through which many finances have been saved.
Some of the examples that have been covered by Fitzpatrick (Citation2019) regarding unsustainable practices observed in FI include disposition of chemical waste without treating into the landfills and water during the shooting of “Pirates of the Caribbean: Dead Men Tell No Tales”; and destruction to the natural sites located across the coastline of African Atlantic during the picturization of “Mad Max: Fury Road”. On the contrary, this industry has also reported and set certain examples for the other partners and players by which they can save the resources and environment as well. For instance, while shooting for the 10th season of the “X-Files” which is of criminal genre, the production house recycles the metals and aluminum and saves more than USD 35,000; reduce the consumption of plastic bottles by 45,740 and reduce 33 tons of carbon emissions (O’Grady, Citation2019). Furthermore, the industry has also taken the initiative of appreciating movies and their respective production houses by introducing “EMA Green Seal Award” of which the famous recipients include “Fifty Shades Freed” for the year 2016; “Yesterday” and “Downtown Abbey” for the year 2019, thus directing and encouraging other production houses for incorporating green practices in their projects.
The other industry which is highly dependent on the quality of the environment and accordingly earns revenue from it is reported to be tourism industry which is not just profitable and lucrative but is also one of the fastest-growing industries around the world (Jawad et al., Citation2017). The potential of this sector can be gauged with the fact that this industry surpasses the speed and rapidness of the goods exporting concerns by exceeding the value of USD 1.7 trillion, which also bring other economic benefits to the society, including employment opportunities, socio-economic development, improvement in quality of infrastructure, increasing the number entrepreneurship and foreign remittances, etc. (Eyuboglu & Uzar, Citation2020; Ozturk et al. Citation2021).
Despite the potential benefits that significantly contribute to the economy, whether it is developed or developing, this industry is also found to be the offender of unsustainable practices and also blamed for the continuous damage that is being done to the natural sites and environmental quality through the emissions of greenhouse gases (Robaina-Alves et al., Citation2016). This detrimental effect and nature of relationships have been reported by several researchers using multiple measurement proxies. For instance, Fethi and Senyucel (Citation2020) have reported the direct connection between the number of tourists arrivals and emissions of greenhouse gases, where more tourist arrivals lead to more greenhouse emissions, which was also supported by other researchers (Aziz, Mihardjo, et al., Citation2020; Koçak et al., Citation2020; Razzaq et al., Citation2021a; Shi et al., Citation2020; Xuefeng et al. Citation2021). Similarly, some researchers have used the measurement of tourism revenues and receipts for measuring the same phenomena but somehow reach the same conclusion that although there would be an improvement in the revenue and receipts but this could lead to more deterioration to the environment (Anser et al., Citation2020; Balli et al., Citation2019a; Koçak et al., Citation2020; Wang et al., Citation2017; Zha et al., Citation2020). On the other hand, Khan et al. (Citation2020) used tourism development measurement and reported that more development leads to more greenhouse emissions (An et al., Citation2021b). Sun et al. (Citation2021) argued the emissions mitigating effects of tourism in Malaysia. Nevertheless, despite all of these measurements gauging the different aspects of tourism, they all agree in terms of its relationship with the destruction and damage to the quality of the environment.
The literature related to tourism and its potential contribution to the economy along with the devastation it caused to the ecology can be categorized into two groups. The first category reflects the studies that tourism led to further destruction to the environment due to increased consumption of energy, fuels, resources, hoteling, transportation, and so on, which also damage the natural sites due to the increased number of arrivals of the tourists (Aziz, Sharif, et al., Citation2020; Khan et al., Citation2020; Tang et al., Citation2018). The second category reflects to the studies according which the tourism can be a potential solution to the environmental destruction reduction due to the green initiatives and the investments made in the tourists' destinations, which includes investment made in the preservation of the natural sites, making infrastructure following the principles of sustainability and so on (Dogan & Aslan, Citation2017; Fethi & Senyucel, Citation2020; Imran et al., Citation2014; Jebli & Hadhri, Citation2018; Paramati, Alam, et al., Citation2017; Paramati, Shahbaz, et al., Citation2017).
China being the most populous country in the world, is also a potential market in terms of both FI and tourism. In terms of FI, the country accounts for major viewerships, which constitutes 21% of the total viewership; with an anticipated estimation to reach the worth of USD 437 billion by 2025; collects revenue from the box office amounted to CNY 20 billion and constitutes 25.4% of market share of the box office across the globe (Statista, Citation2021). In terms of tourism, China is reported to be at the 4th rank among the most visited tourism market around the world and is considered to be low greenhouse generating sector (Tang et al., Citation2014), however the country is reported to have increased the level of greenhouse gases emissions by six times since 1978 (Zheng et al., Citation2020). Moreover, since the country is regarded as one of the highest emitters of carbon which makes it contributing 30% of the global emissions, the development of the sector could also increase the possible need and demands of the fuels and energy, which can further enhance the level of pollution and the destruction to the ecology respectively (Shan et al., Citation2018).
There are multiple contributions that the current study attempts to make. Firstly, the present study explores the effects of film and dram industry (FI) and tourism together on the environmental performance (EP) in China, which possess the potential both in terms of FI and tourism but also emits pollution significantly. Secondly, the current study also contributes the literature related to tourism by utilizing the Tourism Development Index (TDI), which is the most comprehensive and sophisticated measure for tourism and also helps in removing the ambiguity and biases of the literature which may arise due to different selection of proxies and measurement (Razzaq et al., Citation2021). Thirdly, the current study is statistically sound, based on the employment of the unique and recent technique suggested by McNown et al. (Citation2018) named as “Bootstrap Autoregressive Distributed Lag” which is far robust and rigorous in terms of predictability, especially when the dataset possesses weak statistical power or low sample size.
The remainder of the study is arranged as the next section comprises details related to data, followed by the methodology and estimations. The last section presents the conclusion, and recommendations.
2. Data
The current study is an attempt to explore the role of FI, and TDI in determining EP. In addition to this, the inclusion of GDP was made as the control variable because of its repetitiveness and relevancy among the literature exploring precisely TDI (Balli et al., Citation2019a; Khan et al., Citation2020; Wang et al., Citation2017) and studying EP (Awan et al., Citation2020; De Vita et al., Citation2015; Shahbaz et al., Citation2017; Suki et al., Citation2020). Precisely, EP is measured by the index of per capita of ecological footprint extracted from the Global Footprint Network, FI is measured by the index reflecting stocks prices of the companies belongs to the same activity which was extracted from the database of DataStream, whereas TDI is reflected through an index cumulatively developed by expenditure, tourists’ arrivals and the revenues. Lastly, GDP is reflected through real gross product measured in USD. The data for TDI and GDP is extracted from WDI, whereas the observations for all of variables are taken from 2000 to 2019. The details of the focused variables of the current study is shown in .
Table 1. Variable’s description and data source.
3. Bootstrap autoregressive distributed lag (BARDL) technique
For assessment of cointegration among the focused variables, a recent technique is applied that is suggested by McNown et al. (Citation2018) named as “Bootstrap Autoregressive Distributed Lag” (BARDL) technique. This is superior then other traditional estimations techniques predominately in capturing the association and relative significance despite of the possession of data sets’ weak prediction power and comparatively having low sizes, whereas if any data possess similar characteristics, then the application of BARDL will still consider legitimate. The traditional estimations techniques cannot ascertain and accordingly address these statistical issues ( refer Pesaran et al., Citation2001; Pesaran & Shin, Citation1999 ). In addition to this, in order to improve the data’s prediction power and the subsequent statistical scores computed from it for the measure of F-statistic and T-statistic, the methodology of BARDL is designed in a way that the bound testing is excluded and added back (as operationalized in typical ARDL) and lead to the computation within the cointegration outline through the assistance of newest test for cointegration. Pesaran et al. (Citation2001), have highlighted two considerations that should be noted for the recognition of cointegration. These include the statistical significance of coefficients of the error terms and the respective corrections they make, whereas the second consideration is the lagged coefficients for predictor variables that need to be statistically significant.
Furthermore, for decision making, the critical bound should be considered, which includes both lower and higher bounds, in the case of lagged coefficients for predictor variables, however the measure of critical bound should not be considered for the coefficients of error terms and their respective corrections. In addition to this, the application of this technique is dependent on the significance of coefficients of error terms and their respective corrections and needs to be employed if they are found significant after all of the focused variables are termed cointegrated at 1st level. On the other hand, researchers also suggested not evaluating the stationarity or unit root issues in the datasets with weak predictable power and minimal sample size leading to weak characteristics for illustration (Goh et al., Citation2017). Hence based on the recommendation by McNown et al. (Citation2018) the application of BARDL is made to address the methodological and statistical issues mentioned earlier.
Another advantage of BARDL over typical estimations techniques is the possession of no dependency in outcome generation on the order of integration and their characteristics. In contrast, it is also preferred in the context of dynamic models based on time-series data (McNown et al., Citation2018). Furthermore, McNown et al. (Citation2018) stated that BARDL also addresses the apprehension of observations’ inconclusiveness, which generally arise during the employment of traditional techniques based on the framework of ARDL. In addition to this, the BARDL is capable of the generation of the critical values through eliminating the cases and areas that are probably indecisive, which also makes BARDL superior to other traditional techniques for testing bounds of the dynamic models having more than a single independent variable. The mathematical representation of a general BARDL model as discussed by Goh et al. (Citation2017) that comprised of three constructs are mentioned below:
(1)
(1)
Referring equation 1, i, j, k, and l represents lags which are i = 1, 2, …p; j = 0, 1, 2, …, q; k = 1, 2, …, r; l = 1, 2, …, s; t represents the time; criterion variable is yt; xt and zt represents predictor variables; is the dummy variable which represents the break year in accordance with the considerations of unit root as highlighted by Kim and Perron (Citation2009);
and
represents the explanatory variables’ lagged;
represents the coefficient of the variables which was dummy;
represents the error term that is having a variance that is infinite and has a mean equal to zero. The error-correcting arrangement of the current model can be mathematically represented as:
(2)
(2)
Referring Equationequation 2(2)
(2) ,
At this point, the functions that are associated are captured by
and
Moreover, due to the transforming of vector of auto-regression and their levels towards error-correcting arrangement, the derivation of Equationequation 2
(2)
(2) is made. Furthermore, the computation of Equationequation 2
(2)
(2) is made through the assistance of
which is a term of constant and is unrestricted as mentioned under:
(3)
(3)
The pre-requisite of Equationequation 3(3)
(3) states that there should be the rejection of three null hypotheses that eventually lead to the assurance of the cointegration of the variables that are yt, xt and zt. The respective hypotheses are mentioned under:
The test of F1 is grounded on the error terms and their individual corrections, whereas the statement of the null hypothesis states
and the statement of the alternate hypothesis states
which represents the existence of the difference and is not equivalent to zero.
The test of F2 is grounded on the terms of independent variables, whereas the statement of the null hypothesis states
and the statement of the alternate hypothesis states
which represents the existence of the difference and is not equivalent to zero.
The T-test is grounded on the dependent variable, whereas the statement of the null hypothesis states
and the statement of the alternate hypothesis states
which represents the existence of the difference and is not equivalent to zero.
On the other hand, it should also be noted that in the typical ARDL techniques, the bound testing and their respective critical values are generated only for T-test and F1 test whereas the generation of the critical values of the independent variables are represented by F2 test is ignored. Hence, the application of BARDL is made because of the generation of statistic scores of all three tests and their respective critical values as discussed and highlighted by McNown et al. (Citation2018).
4. Estimations and results
At the initial stage, the descriptive statistics of the dataset were evaluated. The criterion variable in the present study is EP is found to have a mean average of 0.657, whereas its minimum value was found to be 0.258 and the maximum value was found to be 0.697 while having a deviation from the mean was found to be 0.484. On the other hand, the current study has three predictor variables: FI, TDI, and GDP. Precisely, FI is found to have a mean average of 0.202. In contrast, its minimum value was found to be 0.685, and maximum value was found to be 1.541, while having a deviation from the mean was found to be 0.026; TDI is found to have the mean average of 0.420, whereas its minimum value was found to be −0.314, and maximum value was found to be 0.654, while having a deviation from the mean was found to be 0.103; GDP is found to have the mean average of 0.690, whereas its minimum value was found to be 0.0212, and maximum value was found to be 1.013, while having a deviation from the mean was found to be 0.017. The outcome of the descriptive statistics is mentioned in .
Table 2. Variable’s description.
In addition to this, the unit root assessment was also made by the help of ADF test as suggested by Dickey and Fuller (Citation1981). The hypothesis statements of this test states that when the outcome is insignificant, it shows the acceptance of the null hypothesis, which means that the dataset possesses unit root whereas when the outcome is statistically significant, it shows the acceptance of the alternate hypothesis, which means that the dataset is free from the unit root and is stationary. The outcome mentioned in revealed that as per the ADF test, all of the variables are found to be stationary at first difference because at level they were having the unit root problem.
In the following stage, the application of BARDL was made for evaluating the cointegration as proposed by McNown et al. (Citation2018) and recommended by other researchers based on its predictability and robustness, which is far better than traditional ARDL techniques (Shahbaz et al. (Citation2018). As already discussed in greater detail in the methodology section that BARDL leads to the generation of outcome through the assessment of three criteria and test-statistics which evaluate three different facets of the outcome, including assessment through T-statistics, which is made for assessing the model as the whole; F-statistics which is made for assessing criterion variable’s lagged scores; and another F-statistics which is a novel and unique aspect of BARDL and is made for assessing predictor variables’ lagged scores, hence gives an edge to BARDL over other ARDL techniques. Although selecting appropriate lag and length is mandatory for bound testing to determine the precise and correct level of the dataset’s cointegration, which, if not ascertained, questions the legitimacy of the outcome. Therefore, to have enhanced quality in predictability power characteristics, the selection of “Akaike Information Criteria” (AIC) is made, which is appropriate to ascertain the order of the lag (Lütkepohl, Citation2006). The selected lag length of the model is shown in 2nd column of .
Table 3. Results of bootstrapped ARDL cointegration analysis.
In addition to this, the assessment of cointegration’s significance in the longer period of time was examined by the statistics, namely FPSS, TDV and TIV, which is accordingly bootstrapped by the propositions by McNown et al. (Citation2018) and compute the significance outcome at significance level of 1% and 5%. Based on the outcome reported in , the significance level revealed that an alternate hypothesis had been accepted, which shows the presence of cointegration in the longer period of time. Furthermore, populace equivalency is ensured by an investigative test which is Q-stat. This test confirms the presence of normality of the dataset, confirming and validating the generation of the output through Jarque-Bera test. On the other hand, the nonappearance of multicollinearity was also ensured, which if not confirmed then could lead to vague outcome (Pesaran et al., Citation2001). The outcome of the statistics is summarized and shown in .
After complying with all parameters for robustness and legitimacy, the application of BARDL was made for assessing the cointegration in the longer and shorter period, considering EP as the criterion variable and FI, TDI and GDP as predictor variables. The application of BARDL has revealed similar results for both longer and shorter period time in terms of nature and significance. Precisely, while focusing the level of cointegration between FI on EP, the FI was reported to be negative and significant at 5% level of significance in the long run and negative and significant at 10% level of significance in the short run
It means that an increment in the FI will improve the environmental performance by decreasing the per capita level of ecological footprint by 5.2% in the long run and 1.6% in the short run. These empirical findings highlight the importance of the film and drama industry in eradicating ecological footprint and improving environmental performance. As this industry plays the role of the ear and mouth of the society and is highly crucial in creating awareness regarding the environment improvement, therefore, its utilization should be efficiently managed as by creating awareness, the general public and masses can be made conscious regarding the responsible consumption and the consequences of irresponsible consumption to the environment (Khan et al., Citation2019b; Najmi et al., Citation2021a) and human health (Khan et al., Citation2019a; Najmi et al., Citation2021b). The outcome of the assessment of cointegration through BARDL between FI and EP in both longer and shorter periods of time is summarized and shown in .
Table 4. Results bootstrapped ARDL cointegration analysis.
While focusing on the level of cointegration between TDI on EP, the TDI was reported to be negative and significant at 1% level of significance in the long run and negative and significant at 5% level of significance in the short run
It means that an increment in the TDI will improve the environmental performance by decreasing the per capita level of ecological footprint by 10.2% in the long run and 8.6% in the short run. These findings also urge the prominence of TDI in reducing the ecological footprint along with the confirmation of the green practices that are being followed in making tourism sector more environmentally sustainable (An et al., Citation2021a; Ling et al., Citation2021). These findings are echoed by Paramati et al. (Citation2018) and Razzaq et al. (Citation2021); however, it contradicts the outcome of Tang et al. (Citation2018). The outcome of the assessment of cointegration through BARDL between TDI and EP in both longer and shorter period of time is summarized and shown in .
On the other hand, while focusing on the level of cointegration between GDP on EP, the GDP was reported to be positive and significant at 1% level of significance in the long run and positive and significant at 1% level of significance in the short run
It means that an increment in the GDP will worsen the environment performance by increasing the per capita level of ecological footprint by 61.5% in the long run and 24.5% in the short run. The relationship bears the highest coefficient value as GDP is the accumulated and desired objective for any financial and economic activity generation. Hence despite increasing economic activities causing an increment in the level of GDP it destroys the quality of the environment. The relationship between GDP and EP is always crucial as economic activities damage environmental quality; however, one economy cannot reduce its level of economic activities just for environmental preservation. Therefore, they opt for practices and operations that are environment friendly and generate maximum level of economic activities at the minimal cost to the environment. These findings are in accordance with the results of the studies by numerous researchers ( refer Awan et al., Citation2020; Balsalobre-Lorente et al., Citation2020; Khan et al., Citation2020; Razzaq et al., Citation2021 ). The outcome of the assessment of cointegration through BARDL between GDP and EP in both longer and shorter periods is summarized and shown in .
In addition to these, while evaluating the model's predictability, all three predictors can explain the EP by 70.9%, which is referred to as the explanation of the variation in the criterion variable reflected by the value of R-square. Apart from that, the model was also reported to be free from autocorrelation, which is gauged by the measure of Durbin Watson. Lastly, while assessing the robustness and rigorousness of the model, the values of CUSUM and CUSUMsq clearing reflects that the model is legitimate and appropriate in terms of stability. All of the aforementioned statistical measures are also reported in .
In the last step, the direction of causality was assessed by the employment of Granger Causality test. Though the direct of causality or dependency from predictor to criterion is already explained and can be judged by the outcome of BARDL or any other regression bases estimation technique, however, this test help is revealing the direction of causality from dependent to independent variables (if any). Similarly, the direction of effects and significance from all independent variables to dependent variables is already discussed and listed in . Apart from that, the findings from Granger Causality test reveal a dual-directional causality between all of the predictors and criterion variable, which is statistically significant. The outcome of the Granger Causality test is shown in .
Table 5. Results of Granger Causality.
5. Conclusions and recommendations
Environmental researchers have urged to safeguard nature, fauna and flora, whereas if neglected, there will be abnormalities in the rainfalls, droughts, and storms, etc. That is also expected to be more lethal and destructive because of the imbalance caused to the environment. Among the few potential solutions that could contribute to environment conservation by eliminating pollution is to create awareness among the masses and public, for which the potential of the role and the significance of contribution by the film and drama industry cannot be ignored.
The FI itself plays a dual role in society. Firstly, the role of watchdog and secondly, being the consumer of the resources where FI is found in abusing the resources in several ways including the excessive making of costumes, extra utilization of fuels, electricity and any other type of energy resources, utilization of decorations, props, and sets which are made up of material and resources that are non-recyclable, etc. On the other hand, tourism is also highly dependent on the quality of the environment and accordingly earns revenue from it is reported to be tourism industry which is not just profitable and lucrative but is also one of the fastest-growing industries around the world. Moreover, tourism is caused destruction to the environment due to increased consumption of energy, fuels, resources, hoteling, transportation, and so on, which also damage the natural sites due to the increased number of tourists arrivals.
Therefore, based on the significance of the two sectors, they are jointly explored in the context of China, which itself is a huge potential market for both of the sectors, whereas it is also one of the greatest emitters of greenhouse gases. Statistically, the current study has made BARDL employ a novel technique for estimation purposes, whereas the direction of causality was also evaluated. Based on the dataset coving observations from 2000 to 2019, the FI was reported to be negative and significant at 5% level of significance in the long run and negative and significant at 10% level of significance in the short run; TDI was reported to be negative and significant at 1% level of significance in the long run and negative and significant at 5% level of significance in the short run whereas GDP was reported to be positive and significant at 1% level of significance in the long run and positive and significant at 1% level of significance in the short run. While comparing the beta coefficients, GDP was reported to be a higher predictor of EP followed by TDI and FI, following this sector's contribution to China's economy. On the other hand, the findings from the Granger Causality test reveal a dual-directional causality between all of the predictors and criterion variables.
Based on the findings, the first recommendation is to make and build institutional pressures by the law enforcement agencies, which not just make the law in accordance with the environment but also implement it and enable the FI and Tourism sector to follow it. Precisely, the environmental violations made in the FI are not regularly reported and highlighted, which has the potential of improvements not just in the consumption of the resources, but recycling it, reusing it, and accordingly disposing it in a more environmental friendly manner. Moreover, there is a need to promote innovation in both sectors where resource consumption is not just optimized. Still, the implementation of green initiatives and environment-friendly processes and operations are implemented, which are often ignored because of being hefty and minimal short term benefits. Lastly, both industries are recommended to move towards more digital and technological advancements through which the imbalance between economic growth and environmental destructions can be minimized.
Following the limitations, there are specific future recommendations to the researchers, which can also lead to potential contributions in the literature. Firstly, there is a need to explore the role of technology innovation in both sectors studied either jointly or separately, which could lead to more insights from both industries. Moreover, the market of other regions, either as a single specifically USA, which is supposed to have great potential in terms of both sectors or as a panel data which could be formed based on the frequency of tourism-related visits or market potential of FI, need to be explored. Lastly, the data exploration needs to be done by employing other statistical techniques based on the framework of linking relationships with quantiles through QARDL or through the machine learning-based methods, which can further explain the detailed insights between the relationships of the studied phenomena.
Disclosure statement
No potential conflict of interest was reported by the authors.
References
- Ahmed, W., Ahmed, W., & Najmi, A. (2018). Developing and analyzing framework for understanding the effects of GSCM on green and economic performance. Management of Environmental Quality: An International Journal, 29(4), 740–758. https://doi.org/10.1108/MEQ-11-2017-0140
- An, H., Razzaq, A., Haseeb, M., & Mihardjo, L. W. (2021). The role of technology innovation and people’s connectivity in testing environmental Kuznets curve and pollution heaven hypotheses across the Belt and Road host countries: New evidence from Method of Moments Quantile Regression. Environmental Science and Pollution Research International, 28(5), 5254–5270.
- An, H., Razzaq, A., Nawaz, A., Noman, S. M., & Khan, S. A. R. (2021). Nexus between green logistic operations and triple bottom line: Evidence from infrastructure-led Chinese outward foreign direct investment in Belt and Road host countries. Environmental Science and Pollution Research, 28, 1–24.
- Anser, M. K., Yousaf, Z., Awan, U., Nassani, A. A., Qazi Abro, M. M., & Zaman, K. (2020). Identifying the carbon emissions damage to international tourism: Turn a blind eye. Sustainability, 12(5), 1937. https://doi.org/10.3390/su12051937
- Awan, A. M., Azam, M., Saeed, I. U., & Bakhtyar, B. (2020). Does globalization and financial sector development affect environmental quality? A panel data investigation for the Middle East and North African countries. Environmental Science and Pollution Research International, 27(36), 45405–45418. https://doi.org/10.1007/s11356-020-10445-4
- Aziz, N., Mihardjo, L. W. W., Sharif, A., & Jermsittiparsert, K. (2020). The role of tourism and renewable energy in testing the environmental Kuznets curve in the BRICS countries: Fresh evidence from methods of moments quantile regression. Environmental Science and Pollution Research, 27(31), 39427–39441.
- Aziz, N., Sharif, A., Raza, A., & Rong, K. (2020). Revisiting the role of forestry, agriculture, and renewable energy in testing environment Kuznets curve in Pakistan: Evidence from Quantile ARDL approach. Environmental Science and Pollution Research International, 27(9), 10115–10128.
- Balli, E., Sigeze, C., Manga, M., Birdir, S., & Birdir, K. (2019a). The relationship between tourism, CO2 emissions and economic growth: A case of Mediterranean countries. Asia Pacific Journal of Tourism Research, 24(3), 219–232. https://doi.org/10.1080/10941665.2018.1557717
- Balsalobre-Lorente, D., Driha, O. M., Shahbaz, M., & Sinha, A. (2020). The effects of tourism and globalization over environmental degradation in developed countries. Environmental Science and Pollution Research International, 27(7), 7130–7144. https://doi.org/10.1007/s11356-019-07372-4
- Chen, X., & Valdmanis, V. (2019). The content analysis of Chinese media report on the issue of waste incineration power plants. Management of Environmental Quality: An International Journal, 30(2), 456–466. https://doi.org/10.1108/MEQ-05-2018-0091
- Danish, & Wang, Z. (2019). Investigation of the ecological footprint's driving factors: What we learn from the experience of emerging economies. Sustainable Cities and Society, 49, 49–76. https://doi.org/10.1016/j.scs.2019.101626
- Danish, Baloch, M. A., & Wang, B. (2019). Analyzing the role of governance in CO2 emissions mitigation: The BRICS experience. Structural Change and Economic Dynamics, 51, 119–125.
- De Vita, G., Katircioglu, S., Altinay, L., Fethi, S., & Mercan, M. (2015). Revisiting the environmental Kuznets curve hypothesis in a tourism development context. Environmental Science and Pollution Research, 22(21), 16652–16663. https://doi.org/10.1007/s11356-015-4861-4
- Dickey, D. A., & Fuller, W. A. (1981). Likelihood ratio statistics for autoregressive time series with a unit root. Econometrica, 49(4), 1057–1072. https://doi.org/10.2307/1912517
- Dogan, E., & Aslan, A. (2017). Exploring the relationship among CO2 emissions, real GDP, energy consumption and tourism in the EU and candidate countries: Evidence from panel models robust to heterogeneity and cross-sectional dependence. Renewable and Sustainable Energy Reviews, 77, 239–245. https://doi.org/10.1016/j.rser.2017.03.111
- Eyuboglu, K., & Uzar, U. (2020). The impact of tourism on CO2 emission in Turkey. Current Issues in Tourism, 23(13), 1631–1645. https://doi.org/10.1080/13683500.2019.1636006
- Fethi, S., & Senyucel, E. (2020). The role of tourism development on CO2 emission reduction in an extended version of the environmental Kuznets curve: Evidence from top 50 tourist destination countries. Environment, Development and Sustainability, 23, 1499–1524. https://doi.org/10.1007/s10668-020-00633-0
- Fitzpatrick, R. K. (2019). Behind every film production is a mess of environmental wreckage. Vice. https://www.vice.com/en_us/article/3kxjvk/behind-every-film-production-is-a-mess-of-environmental-wreckage?fbclid=IwAR0-w24u0V4wPEUP26o8lZKYau7zZ0vcrXhT_iwxl6xHZtQqKEt4R6QBW4Q
- Godil, D. I., Sharif, A., Ali, M. I., Ozturk, I., & Usman, R. (2021). The role of financial development, R&D expenditure, globalization and institutional quality in energy consumption in India: New evidence from the QARDL approach. Journal of Environmental Management, 285, 112208. https://doi.org/10.1016/j.jenvman.2021.112208
- Goh, S. K., Yong, J. Y., Lau, C. C., & Tang, T. C. (2017). Bootstrap ARDL on energy-growth relationship for 22 OECD countries. Applied Economics Letters, 24(20), 1464–1467. https://doi.org/10.1080/13504851.2017.1284980
- Guo, J., Zhou, Y., Ali, S., Shahzad, U., & Cui, L. (2021). Exploring the role of green innovation and investment in energy for environmental quality: An empirical appraisal from provincial data of China. Journal of Environmental Management, 292, 112779.
- Imran, S., Alam, K., & Beaumont, N. (2014). Environmental orientations and environmental behaviour: Perceptions of protected area tourism stakeholders. Tourism Management, 40, 290–299. https://doi.org/10.1016/j.tourman.2013.07.003
- Jawad, S., Shahzad, H., Shahbaz, M., & Ravinesh, R. (2017). Tourism-led growth hypothesis in the top ten tourist destinations: New evidence using the quantile-on-quantile approach. Tourism Management, 60, 223–232. https://doi.org/10.1016/j.tourman.2016.12.006
- Jebli, M. B., & Hadhri, W. (2018). The dynamic causal links between CO2 emissions from transport, real GDP, energy use and international tourism. International Journal of Sustainable Development & World Ecology, 25(6), 568–577. https://doi.org/10.1080/13504509.2018.1434572
- Khan, F., Ahmed, W., & Najmi, A. (2019a). Understanding consumers’ behavior intentions towards dealing with the plastic waste: Perspective of a developing country. Resources, Conservation and Recycling, 142, 49–58. https://doi.org/10.1016/j.resconrec.2018.11.020
- Khan, A., Chenggang, Y., Hussain, J., Bano, S., & Nawaz, A. A. (2020). Natural resources, tourism development, and energy-growth-CO2 emission nexus: A simultaneity modeling analysis of BRI countries. Resources Policy, 68, 101751. https://doi.org/10.1016/j.resourpol.2020.101751
- Khan, M., Najmi, A., Ahmed, W., & Aman, A. (2019b). The role of consumer willingness to pay for halal certification in Pakistan. Journal of Islamic Marketing, 10 (4), 1230–1244. https://doi.org/10.1108/JIMA-09-2018-0155
- Khan, S. A. R., Razzaq, A., Yu, Z., & Miller, S. (2021). Industry 4.0 and circular economy practices: A new era business strategies for environmental sustainability. Business Strategy and the Environment, 30(8), 4001–4014. https://doi.org/10.1002/bse.2853
- Kim, D., & Perron, P. (2009). Unit root tests allowing for a break in the trend function at an unknown time under both the null and alternative hypotheses. Journal of Econometrics, 148(1), 1–13. https://doi.org/10.1016/j.jeconom.2008.08.019
- Koçak, E., Ulucak, R., & Ulucak, Z. Ş. (2020). The impact of tourism developments on CO2 emissions: An advanced panel data estimation. Tourism Management Perspectives, 33, 100611. https://doi.org/10.1016/j.tmp.2019.100611
- Ling, G., Razzaq, A., Guo, Y., Fatima, T., & Shahzad, F. (2021). Asymmetric and time-varying linkages between carbon emissions, globalization, natural resources and financial development in China. Environment, Development and Sustainability, 1–29.
- Liu, Z. (2020). The global film industry index research report, 2019. In Development of the global film industry (pp. 14–33). Routledge.
- Lopera-Mármol, M., & Jiménez-Morales, M. (2021). Green shooting: Media sustainability, a new trend. Sustainability, 13(6), 3001. https://doi.org/10.3390/su13063001
- Lütkepohl, H. (2006). Structural vector autoregressive analysis for cointegrated variables. Allgemeines Statistisches Archiv, 90(1), 75–88. https://doi.org/10.1007/s10182-006-0222-4
- McNown, R., Sam, C. Y., & Goh, S. K. (2018). Bootstrapping the autoregressive distributed lag test for cointegration. Applied Economics, 50(13), 1509–1521. https://doi.org/10.1080/00036846.2017.1366643
- Najmi, A., Kanapathy, K., & Aziz, A. A. (2019). Prioritising factors influencing consumers' reversing intention of e-waste using analytic hierarchy process. International Journal of Electronic Customer Relationship Management, 12(1), 58–74. https://doi.org/10.1504/IJECRM.2019.098981
- Najmi, A., Kanapathy, K., & Aziz, A. A. (2021a). Understanding consumer participation in managing ICT waste: Findings from two-staged Structural Equation Modeling-Artificial Neural Network approach. Environmental Science and Pollution Research International, 28(12), 14782–14796. https://doi.org/10.1007/s11356-020-11675-2
- Najmi, A., Kanapathy, K., & Aziz, A. A. (2021b). Exploring consumer participation in environment management: Findings from two‐staged structural equation modelling‐artificial neural network approach. Corporate Social Responsibility and Environmental Management, 28(1), 184–195. https://doi.org/10.1002/csr.2041
- Najmi, A., & Khan, A. A. (2017). Does supply chain involvement improve the new product development performance? A partial least square-structural equation modelling approach. International Journal of Advanced Operations Management, 9(2), 122–141. https://doi.org/10.1504/IJAOM.2017.086680
- O’Grady, M. (2019). It's a Good Thing: Green Spark Group puts the spotlight on the environment. BCBUSINESS. https://www.bcbusiness.ca/Its-a-Good-Thing-Green-Spark-Group-puts-the-spotlight-on-the-environment
- Ozturk, I., Aslan, A., & Altinoz, B. (2021). Investigating the nexus between CO2 emissions, economic growth, energy consumption and pilgrimage tourism in Saudi Arabia. Economic Research-Ekonomska Istraživanja, 1–16. https://doi.org/10.1080/1331677X.2021.1985577
- Paramati, S. R., Alam, S., & Chen, C. (2017). The effects of tourism on economic growth and CO2 emissions: A comparison between developed and developing economies. Journal of Travel Research, 56(6), 712–724. https://doi.org/10.1177/0047287516667848
- Paramati, S. R., Alam, S., Keung, C., & Lau, M. (2018). The effect of tourism investment on tourism development and CO2 emissions: Empirical evidence from the EU nations. Journal of Sustainable Tourism, 26(9), 1587–1607. https://doi.org/10.1080/09669582.2018.1489398
- Paramati, S. R., Shahbaz, M., & Alam, M. S. (2017). Does tourism degrade environmental quality? A comparative study of eastern and Western European Union. Transportation Research Part D: Transport and Environment, 50, 1–13. https://doi.org/10.1016/j.trd.2016.10.034
- Pesaran, M. H., Shin, Y., & Smith, R. J. (2001). Bounds testing approaches to the analysis of level relationships. Journal of Applied Econometrics, 16(3), 289–326. https://doi.org/10.1002/jae.616
- Pesaran, M. H., & Shin, Y. (1999). An autoregressive distributed lag modelling approach to cointegration analysis. In S. Strom (Ed.), Econometrics and Economic Theory in the 20th Century: The Ragnar Frisch Centennial Symposium. Cambridge University Press.
- Razzaq, A., Ajaz, T., Li, J. C., Irfan, M., & Suksatan, W. (2021a). Investigating the asymmetric linkages between infrastructure development, green innovation, and consumption-based material footprint: Novel empirical estimations from highly resource-consuming economies. Resources Policy, 74, 102302. https://doi.org/10.1016/j.resourpol.2021.102302
- Razzaq, A., Sharif, A., Ahmad, P., & Jermsittiparsert, K. (2021). Asymmetric role of tourism development and technology innovation on carbon dioxide emission reduction in the Chinese economy: Fresh insights from QARDL approach. Sustainable Development, 29(1), 176–193. https://doi.org/10.1002/sd.2139
- Razzaq, A., Wang, Y., Chupradit, S., Suksatan, W., & Shahzad, F. (2021). Asymmetric inter-linkages between green technology innovation and consumption-based carbon emissions in BRICS countries using quantile-on-quantile framework. Technology in Society, 66, 101656. https://doi.org/10.1016/j.techsoc.2021.101656
- Robaina-Alves, M., Moutinho, V., & Costa, R. (2016). Change in energy related CO2 (carbon dioxide) emissions in Portuguese tourism: A decomposition analysis from 2000 to 2008. Journal of Cleaner Production, 111, 520–528. https://doi.org/10.1016/j.jclepro.2015.03.023
- Shahbaz, M., Khan, S., Ali, A., & Bhattacharya, M. (2017). The impact of globalization on CO2 emissions in China. The Singapore Economic Review, 62(04), 929–957. https://doi.org/10.1142/S0217590817400331
- Shahbaz, M., Nasir, M. A., & Roubaud, D. (2018). Environmental degradation in France: The effects of FDI, financial development, and energy innovations. Energy Economics, 74, 843–857. https://doi.org/10.1016/j.eneco.2018.07.020
- Shan, Y., Guan, D., Zheng, H., Ou, J., Li, Y., Meng, J., Mi, Z., Liu, Z., & Zhang, Q. (2018). China CO2 emission accounts 1997-2015. Scientific Data, 5, 170201 https://doi.org/10.1038/sdata.2017.201
- Sharif, A., Baris-Tuzemen, O., Uzuner, G., Ozturk, I., & Sinha, A. (2020). Revisiting the role of renewable and non-renewable energy consumption on Turkey’s ecological footprint: Evidence from Quantile ARDL approach. Sustainable Cities and Society, 57, 102138. https://doi.org/10.1016/j.scs.2020.102138
- Sharif, A., Bhattacharya, M., Afshan, S., & Shahbaz, M. (2021). Disaggregated renewable energy sources in mitigating CO2 emissions: New evidence from the USA using quantile regressions. Environmental Science and Pollution Research, 1–20.
- Shi, H., Li, X., Zhang, H., Liu, X., Li, T., & Zhong, Z. (2020). Global difference in the relationships between tourism, economic growth, CO2 emissions, and primary energy consumption. Current Issues in Tourism, 23(9), 1122–1137. https://doi.org/10.1080/13683500.2019.1588864
- Statista. (2021). https://www.statista.com/
- Suki, N. M., Sharif, A., Afshan, S., & Suki, N. M. (2020). Revisiting the environmental Kuznets curve in Malaysia: The role of globalization in sustainable environment. Journal of Cleaner Production, 264, 121669. https://doi.org/10.1016/j.jclepro.2020.121669
- Sun, Y., Duru, O. A., Razzaq, A., & Dinca, M. S. (2021). The asymmetric effect eco-innovation and tourism towards carbon neutrality target in Turkey. Journal of Environmental Management, 299, 113653.
- Tang, Z., Bai, S., Shi, C., Liu, L., & Li, X. (2018). Tourism-Related CO2 Emission and Its Decoupling Effects in China: A spatiotemporal perspective. Advances in Meteorology, 2018, 1–9. https://doi.org/10.1155/2018/1473184
- Tang, Z., Shang, J., Shi, C., Liu, Z., & Bi, K. (2014). Decoupling indicators of CO2 emissions from the tourism industry in China: 1990-2012. Ecological Indicators, 46, 390–397. https://doi.org/10.1016/j.ecolind.2014.06.041
- Wang, K., Shao, H., Zhou, T., & Liu, H. (2017). Analysis of impact factors of CO2 emissions from tourism in China based on STIRPAT model. Environmental Science, 37, 1185–1192. https://doi.org/10.13671/j.hjkxxb.2016.0259
- Xuefeng, Z., Razzaq, A., Gokmenoglu, K. K., & Rehman, F. U. (2021). Time varying interdependency between COVID-19, tourism market, oil prices, and sustainable climate in United States: Evidence from advance wavelet coherence approach. Economic Research-Ekonomska Istraživanja, 1–23.
- Zha, J., Tan, T., Yuan, W., Yang, X., & Zhu, Y. (2020). Decomposition analysis of tourism CO2 emissions for sustainable development: A case study of China. Sustainable Development, 28(1), 169–118. https://doi.org/10.1002/sd.1980
- Zhang, H., Razzaq, A., Pelit, I., & Irmak, E. (2021). Does freight and passenger transportation industries are sustainable in BRICS countries? Evidence from advance panel estimations. Economic Research-Ekonomska Istraživanja, 1–21. https://doi.org/10.1080/1331677X.2021.2002708
- Zheng, X., Lu, Y., Yuan, J., Baninla, Y., Zhang, S., Stenseth, N. C., Hessen, D. O., Tian, H., Obersteiner, M., & Chen, D. (2020). Drivers of change in China's energy-related CO2 emissions. Proceedings of the National Academy of Sciences of the United States of America, 117(1), 29–36. https://doi.org/10.1073/pnas.1908513117
- Zhuang, Y., Yang, S., Razzaq, A., & Khan, Z. (2021). Environmental impact of infrastructure-led Chinese outward FDI, tourism development and technology innovation: A regional country analysis. Journal of Environmental Planning and Management, 1–33. https://doi.org/10.1080/09640568.2021.1989672