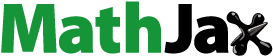
Abstract
This study investigates the influence of ecological innovations and sustainable financing in promoting the Chinese economy's green total factor productivity (GTFP) from Q1-2004 to Q4-2018. Initially, this study measures GTFP using Data Envelopment Analysis and applies Bootstrap Auto-regressive Distributed Lagged (BARDL) model for dynamic effects. The preliminary results confirm the existence of unit root and a long-term cointegrating relationship among the model variables. The long-run results demonstrate that ecological innovations and sustainable financing have promoted GTFP in China. Moreover, government intervention is imperative to support green productivity growth at the macro level. However, there is a need to control the adverse effect of unemployment in impeding GTFP. Notably, the long-run results reflect the more substantial impact of the stated variables compared to the short run with the convergence towards long-run equilibrium. The policymakers are suggested to prioritise the development of the financial market and technological innovations.
1. Introduction
Over the past four decades, the Chinese economy has maintained growth in different sectors while achieving the title of second in the world in terms of total economic output (Wang et al., Citation2021). Moreover, China's average annual growth rate has witnessed remarkable growth that is covered by a three-phase superposition; the stage of growth shift, adjustment pain, and initial policy digestion (Liu et al., Citation2021). Although the growth in the Chinese region is quite impressive, however, issues like environmental pollution, ecological harm, wasting of different natural resources, higher levels of traditional energy consumption, and low sustainable growth are pretty imperative (Ling et al., Citation2022; Liu & Lin, Citation2019). Meanwhile, there is an imbalance between sustainable and traditional economic growth where more emissions from economic activities cannot be neglected (Le & Ozturk, Citation2020; Sharif et al., Citation2020; Shan et al., Citation2021). Under the constant threat to the natural environment, there is a strong need to achieve a green and low-carbon economy. The role of green financing is a powerful track that helps put the growth engine in a sustainable direction. In the same pursuits, China is currently trying to achieve net zero-emission or carbon neutrality by 2060.
Academicians and policymakers focus on China and its green financial market to achieve sustainable targets. In this regard, by the end of 2020, the scale of green credit in the Chinese economy has increased to 10 trillion marks, where the stock of green bonds was about to touch 813.2 trillion yuan (Yoshino et al., Citation2019). At the same time, the communication capacity of international green financing has also been strengthened, which shows an ascendant growth of sustainable finance in China. Moreover, green financing (GFIN) also helps to fill the gap for green financial products in the form of green bonds and green funds, which provide reasonable guarantees towards sustainable efforts. Additionally, GFIN helps construct a green financial system while lowering the adverse utilisation of natural and financial resources. The traditional research on the relationship between green finance and economic growth mainly focuses on the direct association. These variables are highly integrated with green total factor productivity (GTFP) and interesting research area to be explored. The term GTFP helps consider the utilisation of resources and environmental cost in the traditional measure of total factor productivity (Ren, Citation2020). Therefore, effectively measuring the GTFP from the context of China under the shadow of green financing is much needed. Exploring this relationship between the utilisation of green funding and its role in justifying the GTFP will help policymakers to initiate effective policy measures. GTFP can be attained by integrating environmental loss and resource input based on the original total factor productivity (TFP) (Ren, Citation2020). Measurement of GTFP can be made from the economy's reliance on natural resources within a country. The TFP index between 1997 and 2019 in green development was found to be 1.0173, which states an average annual growth rate of 1.73% in the case of China (Zhong et al., Citation2021).
In addition, green innovation (GINO) has become prevalent as global warming and increasing environmental threats threaten the world (Sun et al., Citation2022). The sustainability horizon in terms of green growth has been ever more urgent, where the role of technological progress in promoting green development is generally accepted now. From the context of China, remarkable economic growth has been achieved while opining some significant discussion on sustainable policies and practices (Sun et al., Citation2022). Meanwhile, China's economic development depends on traditional technologies and energy sources. Therefore, in response to adverse environmental impacts, the 13th Five-year plan in the last decade has mainly focussed on green technological innovation and development as the core principles for the future outlook (Sun & Razzaq, Citation2022; Wang et al., Citation2021). Besides, the nexus between green innovations and green growth has also been under the researchers' observations. Technological progression, specifically in the Chinese economy, helps achieve sustainable development (Wang et al., Citation2021). However, the association between green innovation and GTFP in China has been investigated with less attention, specifically while applying the advanced timer series estimations. Based on the motivation from this gap, the current study has contributed significantly to the literature while claiming that green financing and innovations have stimulated GTFP over the past two decades.
The rest of the article is organised in the following way: section 2 covers the literature review while focussing on the relationship between the variables. Section 3 demonstrates the research methodology and econometric models. Section 4 discusses the results, whereas the last section covers the conclusion and policy implications.
2. Literature review
2.1. Nexus between green financing and green factor productivity
Lee and Lee (Citation2022) explore different factors affecting green productivity growth. The research work analyses the link between green finance (GFIN) development and GTFP based on the extensive index of GFIN between 2006 and 2018 for Chinese provinces. They explore the impact of GFIN on GTFP, revealing that the development in GFIN significantly improves the GTFP. The more beneficial effect is estimated in the case of provinces with high levels of economic growth, improved environmental protection, and green development. It is further stated that efficient green energy policies can increase the impact of GFIN on GTFP. China has made various policy implications for attaining higher productivity with less environmental damage. To achieve carbon neutrality goals, developing economies must determine the constraints of GFIN on the production of green energy. Liu et al. (Citation2021) employ the panel datasets from 30 provinces of China for the period 2009 and 2019 to determine the GTFP and development level of GFIN. They utilise the entropy method and explosion-based measurement model to determine the relationship between GFIN and GTFP. The empirical research undertakes technological innovation, industrial development, higher economic progress, and environmental supervision in the framework of GTFP for Chinese provinces. The research outcomes reveal that the topological development of GFIN in China provides a decreasing trend from East to Middle to West and coastal to the island.
The correlation between GFIN and GTFP has gained much importance among researchers and policymakers in countries with higher carbon emissions like China. Zhong and Li (Citation2020) attempt to disclose the distribution of GFTP in Chinese provinces. The study further states the impact of GFIN on GTFP by employing the Green Malmquist-Luenberger (GML) index based on the Spatial Durbin Model (SDM) between 1996 and 2015. Their findings reflect that the mean values of China’s GTFP show a U-shaped relationship for the data between 1996 and 2015. Development in GFIN promotes the growth of GTFP through innovative technologies. The study states that GFIN boosts environmental-friendly innovation and green technology development, leading towards a sustainable environment. Zhou et al. (Citation2019) measure the GTFP for China based on provincial panel data to empirically investigate the impact of GFIN development on GTFP and its threshold effect. The research study determines different factors related to GFIN, including foreign direct investment, green innovation, and environmental regulations affecting the GTFP in Chinese provinces. Results of the empirical study show that enhanced GFIN positively impact the China’s GTFP with an overall upward tendency. It further states that GFIN development has a double-threshold impact on overall GTFP with a non-linear relationship. Moreover, a positive U-shaped correlation is estimated between GFIN and GTFP for Chinese provinces and a positive effect of technology innovation that can bring sustainable development.
To promote sustainable development in the economy, a critical analysis of the impacts of green credit under GFIN on GTFP is conductive. The literature contribution by Hu et al. (Citation2021) explores the heterogeneous effects of GFIN on GTFP based on the data of 30 provinces from 2008 to 2017 in China. The results determine that greenhouse gas emissions enhance the GTFP based on the panel regression model. Secondly, the panel quantile approach results show that improvement in GTFP improves the significant impact of GFIN on GTFP in Chinese provinces. Moreover, the study states that green credit has a diversified effect on GTFP that can be estimated from economic growth. The datasets between the given period reveal that green innovation through enhanced GFIN positively impacts the GTFP. In addition, the research study of Liu et al. (Citation2022) employs the chain-mediated approach to determine the impact of the issuance of GFIN on GTFP of different provinces of China based on the data from 2008 to 2016. The empirical results through heterogenous analysis reveal that due to vast differences in regional industrial infrastructures, the impact of GFIN on GTFP is more substantial in the Western region than in the other areas of China. Development in GFIN is on the rise in China, but this progress is found to be insufficient for different provinces. Hence, it can be stated that with increased investment in green innovation, more productivity can be obtained in all sectors with sustainable development (Razzaq et al., Citation2021).
2.2. Nexus between Green Innovation (GINO) and Green Factor Productivity (GTFP)
As the biggest emitter of Greenhouse gases (GHGs), the Chinese government aimed to promote green innovation to be carbon neutral by 2030 (IIAS, Citation2022). Rapid development in China has created a higher energy demand, putting more pressure on the environment. To achieve a green economy, the Chinese government devised environmental regulations to reduce GHG emissions by 17%, energy utilisation by 16%, and increase the forecast coverage by 21.66% per unit of GDP (OECD, Citation2022). China’s government seeks to improve public environmental services and strengthen ecological risk prevention and control. The federal government is aimed to provide enhanced economic support through metrics such as federal grants and ecological remuneration to develop environmental public services in western regions, areas less developed, and other underprivileged areas.
Green technology innovation can effectively reduce fossil fuel consumption's negative impacts. Green technology innovation is one of the essential methods to enhance the GTFP and achieve sustainable development. The research study of Wang et al. (Citation2022) estimates the provincial-based GTFP by analysing the available data from 2000 to 2016 in China. The study employs the Malmquist index method and stochastic frontier analysis to determine the spatial impact of GINO on GTFP for Chinese provinces. The results show an overall upward trend in GTFP. Still, the Eastern region shows the highest values and the Western part with the lowest increase in GTFP in China.
Moreover, the Spatial Durbin Model reveals a positive cointegration between GINO and GTFP in China. It is further stated that GINO of China's Central and Eastern regions negatively impacts the GTFP. Government should develop policies regarding the increased green technology innovation for sustainable development in all sectors. Given China’s increased productivity, its economy requires a shift from non-renewable to renewable energy development. GINO is considered a vital tool for achieving increased GTFP. Hence, Lee et al. (Citation2022) investigate the impact of GINO in the industrial sector on GTFP. The study provides robust empirical outcomes from data from different provinces in China from 2006 to 2017. Their results show that increased GINO in technology and production effectively enhance the GTFP. Moreover, the innovation capability of the government plays a mediating role in attaining improved GTFP. The government of China must enhance and optimise green technology innovation to achieve higher levels of GTFP along with sustainable development.
Based on the technological diversity, there is a need for green technology innovation that can reduce the environmental impacts of non-renewable energy technologies. Song et al. (Citation2022) analysed the GTFP of Chinese provinces from 2006 to 2017, employing the Meta-frontier and data envelopment model. The study explores the cointegration between GINO and GTFP in China. Their findings show that technological development through GINO can bring improvement inGTFP. The Malmquist-Luenberger index also provides the unidirectional relationship of given variables regarding green growth in the Chinese industrial sector. The increased investment in green technology innovation significantly impacts GTFP in various sectors. China's government should incentivize organisations to improve GINO based on renewable energy development and utilisation. It should also encourage industries to invest more in GINO for a sustainable future with improved GTFP. Green technology innovation can alleviate the climate crisis with enhanced adoption and improved investment. Gao et al. (Citation2022) show that GINO can be only effective in high-income countries, while low-income countries do not offer a positive correlation between GINO and GTFP. Digitalisation and GINO can positively impact the GTFP with increased investment in green technology and renewable energy production. The results show clarifying impacts on GTFP in case of Chinese cities. It is critical to draw attention to the need for significant technological upgrades by incorporating green technology advances into new investments. Their findings also point to strategies that promote global green technology collaboration, such as expediting green technology transfer across countries.
2.3. Control variables
This study also controls other factors of GTFP. Thus include control variables such as Government Intervention (Gov) and Unemployment Rate (UNER).
2.3.1. Government intervention and green factor productivity
Government Intervention (GINT) is the ratio of government expenses as % of GDP. The GINT plays a mediating role in developing environmental regulation and economic growth through administrative techniques. Thus, GINT can propose strategies for GFIN for higher economic development in various sectors. The GINT impacts the GTFP while devising policies for a secure and sustainable environment. Some of the administrative strategies taken by the government may include credit subsidies to develop backward industries, regulating industries to build their resource effectiveness, and reducing GHG emissions (Dong et al., Citation2016). The government of developing nations like China may encourage the development of GINT through renewable means of energy production and shifting industries to green technologies for a sustainable environment. Government can also provide tax cuts, financial subsidies, and green investment to improve the development of renewable energy production and other eco-friendly technologies at the industrial level. Xie et al. (Citation2021) analyses that the GINT can guide industries towards GINO and economic growth to achieve sustainable development.
Wang and Yan (Citation2022) explore the impact of intervention from the government in regulating the green economy based on optimal regulations. The study employs an SBM-Malmquist model to determine the effectiveness of the GINT in raising the GTFP in Chinese provinces. Their results reveal that improved environmental regulations significantly impact the GTFP with a mediating effect on GINT in China. Li et al. (Citation2022) provides the impact of GINT and financial policies to trigger economic growth and improve environmental quality in Chinese cities. The mediating role of GINT positively impacts the GTFP in the industrial sector of China. Another research study by Huang (Citation2022) provides the concept of GINO and GFIN with the mediating role of GINT to increase GTFP in the case of high-income BRI economies. The study utilises GMM methodology and data envelopment techniques to determine the control impact of GINT in increasing or decreasing the GTFP. Their findings show that the lack of significant government measures is the prime cause for volatility in the BRI countries' green economic performance score. Furthermore, the econometric results suggest that government spending has a strong positive impact on green economic growth. Hence, in terms of curbing carbon emissions and green innovation development, the GINT can bring enhanced GTFP in the case of higher-income countries.
2.3.2. Unemployment rate and green factor productivity
The unemployment rate (UNER) indicates the employment condition in any economy (WorldBank, Citation2022). The research contribution of Fangming et al. (Citation2021) employs the total unemployment index as a percentage of the labour force to determine the UNER. UNER plays a mediating role in determining the levels of GTFP based on the close relationship between economic development and UNER. Increased UNER negatively impacts economic growth, hence decreasing the overall GTFP. They employ Okun’s Law, which states that UNER infers the underutilisation of production factors and leads to a reduction in GDP. Furthermore, with an increase in UNER, the number of manufactured products and services also decrease in economic activity (Khan et al., Citation2022). When more people are severely unemployed within an economy, the GTFP also falls eventually in the long and short run. Thus, UNER must be considered a control variable in exploring the level of GTFP in an economy for enhanced development in the industrial sector with a sustainable future.
In addition, the empirical work of Ng et al. (Citation2022) examines the EKC hypothesis employing the GINT as a mediating factor in indicating the GTFP in selected OCED economies. It further aims to determine the effectiveness of GFIN on GTFP levels. The study analyses the panel data for 36 OECD economies from 1995 to 2015 (Sarkodie & Ozturk, Citation2020). Cointegration and second-generation unit root tests were employed to explore the cross-section interrelationship between the given variables after controlling the role of UNER. Their findings reveal that developing GINO could be a key aspect in maintaining sustainable growth and improved environmental quality in OECD nations. Moreover, the study recommends focussing on UNER for OECD economies for innovative GFIN and promoting green energy utilisation, implying that employment rate, green policies, and economic growth are interlinked. Song et al. (Citation2022) employ the GML productivity data to determine the GTFP in China from 2000 to 2014. The study states that UNER can be effectively decreased through GINT while creating job opportunities. Labour market policies devised by governmental departments also impact the total productivity at an industrial scale in terms of enhanced productivity and green technology innovation. Improved implementation of green energy policies and ambitions can also improve environmental quality, maintain economic growth, and reduce UNER and enhance the GTFP levels.
3. Methodology
3.1. The bootstrap ARDL
The study employs ‘Bootstrap Auto-regressive Distributed Lag’ (BARDL) model with a recently established Bound Testing technique to analyse the long-term association between the given variables (McNown et al., Citation2018). This study effectively deals with the power attributes and lower size issues, which have not been discussed in the general ARDL model (Pesaran et al., Citation2001). In addition, the BARDL approach is an addition to the simple ARDL bound test that involves a new correlation test to enhance the power of the F-test and T-test. The general cointegration test employs two constraints while exploring the correlation between given variables. The first requirement of the cointegration test is the information about error-correction terms (ECTs). Secondly, significant factors are also required for lagged independent variables. Pesaran et al. (Citation2001) proposed that no critical bounds are present for the first condition, while lower and upper bounds are considered for the cointegration test for the second case. The ECTs are analytically significant for examining the first situation, while the bound test is employed only in the case if the model carries the order of one variable. Hence, the general ARDL model provides weak power and explanatory attributes (McNown et al., Citation2018).
BARDL model utilises an extra F-test for lagged factors of independent variables (Goh et al., Citation2017). The independent variables discussed in this study include green financing and innovation, government intervention, and the unemployment rate as control variables affecting the green factor productivity. The BARDL model affects the output response by recognising the factors with mixed integration order that is more suitable for dynamic approaches based on more than one independent variable. It also discusses the issue of indefinite evidence from the traditional model of bound testing (McNown et al., Citation2018). Following the research contribution of Goh et al. (Citation2017), EquationEquation (1)(1)
(1) provides the mathematical description of the conventional ARDL bound testing methodology with four descriptive variables.
(1)
(1)
Here, lag variables are represented by i, j, k and l that vary from 0 to p, 0 to q, 0 to r and 0 to s. The term t denotes the time, GTFP is the dependent variable, and
terms are the independent variables. The term
is a dummy for break year based on the unit root test provided by Kim and Perron (Citation2009). The factors of lag independent variables are denoted by
is the value for dummy coefficient,
denotes the error value with constant deviation. The procedure for error correction for the BARDL model is provided as follows:
(2)
(2)
The variables from the above equation, including
and
contain the concerned function from the EquationEquation (1)
(1)
(1) . The unconditional model is obtained by employing constant term (
) such as:
(3)
(3)
To determine the correlation between the given variables and
rejection is required for the following null hypotheses in the study:
Error correction terms (ECTs) employed in the study are calculated by F-1 Test.
H0: =
=
= 0 whereas H1: At least one (
are not zero.
The explanatory variable values are determined using the F-2 test.
H0: =
= 0 against H1: At least one (
3) Lagged variable based on other control variables is provided as:
H0: = 0 against H1:
zero.
Table 1. Variables’ description.
Similarly, output measures like economic output, carbon dioxide emission, waste gasses, and solid waste can be considered. However, it is pretty complex to view all the stated input and output while measuring the GTFP. Therefore, the selection of both input and output measures is reasonably necessary. Johnes (Citation2006) also says that DEA analysis focuses on various input and output measures where the model has no functional requirement. Therefore, many research studies have utilised the DEA approach to measure productivity and efficiency (Kulshreshtha & Parikh, Citation2002; Li et al., Citation2019).
To overcome the issue of a shorter period, we have utilised a quadratic match-sum approach to transform yearly data into quarterly data by following Razzaq et al. (Citation2021a). The quadratic match-sum method is quite inspective because the adjustment of cyclical variation in data and seasonality issues are avoided as this procedure decreases the point-to-point data variations.
4. Results and discussion
Descriptive statistics are reported in , showing that the unemployment rate reflects the highest mean score during the study duration, whereas the average trend in GTFP was 1.436, respectively. Moreover, green innovations and green financing have their highest scores of 2.97 and 3.73, whereas the green productivity dynamic reflects the lowest maximum trend. In addition, current research also focuses on the JB test for which H0 for the normal distribution is tested. The results show that JB test statistics show insignificant p-values for green factor productivity, green financing, green innovation, governmental intervention, and unemployment. Therefore, these variables have been normally distributed over the past two decades.
Table 2. Descriptive statistics and normality testing.
As stated in the research methodology, it is obvious to examine the unit root properties of the study variables, for which results are shown in through ADF and ZA tests considering the structural breaks. However, at the level of both ADF and ZA tests, the results report the unit root problem. Therefore, we have taken the first difference for GTFP, GFIN, GINO, GINT and UNER, respectively. While taking the first difference, these variables are integrated at I(1), where both ADF and ZA tests report that null findings are highly significant at 1%. More specifically, the structural breaks are presented for 2006Q2, 2004Q1, 2010Q3, 2014Q2 and 2008Q3 for the stated variables. This confirms a different order of integration for the selected variables. Hence, this research applies cointegration techniques.
Table 3. Results of Unit root test.
Our study applies BARDL modelling as McNown et al. (Citation2018) suggested based on the detailed explanation under the research methodology. BARDL is admitted to providing more reliable results than the traditional ARDL models. Moreover, a joint F-test through lagged values of the study variables has been utilised in BARDL estimation with the traditional T-test statistics. It has superiority over traditional ARDL models based on the above claims. The findings have been covered in , showing the estimated model, lag levels, break year, Fpss T for DV and IV, and the diagnostics output. The results show that the break year was 2008Q2, where the Fpss score was 19.305, significant at 1%.
Table 4. Bootstrapped ARDL cointegration analysis.
Similarly, the T values for DV and IV also reflect a highly significant output at 1%, confirming that long-term cointegration is presented among the study variables at 1% and 5%, respectively. The diagnostic result confirms the explained variation in GTFP as 82.0%, which is indeed a good model's explanatory power. Finally, the Null for the JB test reports the normal distribution of the data, whereas H1 rejects it. As the JB test statistics' findings are insignificant, it confirms the presence of normal distribution that supports H0.
For the long-run association, the findings are reported in . We observe that GFIN helps in improving the GTFP. The coefficient of GFIN is 0.357 with a T-score of 4.205. It shows that a 1% increase in green financing stimulates green productivity by 0.357%. These results are highly significant as the p-value is 0.000. Over the past couple of decades, considerable growth has been observed in green financing, which helps improve the financial infrastructure and sustainable finance (Liu et al., Citation2021).
Table 5. Results bootstrapped ARDL cointegration analysis (long run).
Additionally, the financial reforms under the shadow of green products and green services have also been observed in the Chinese economy. Moreover, green financing in China results from evaluating the financial market through quantitative efforts and efficiency effects (Fakher et al., Citation2021; Nizam et al., Citation2020). Liu et al. (Citation2021) claim that green financing contributes to high-quality economic growth in green factor productivity. Chi-Chuan Lee et al. (Citation2022) have covered similar empirical findings while taking the provincial-level data from China during 2006–2018. Their study states that the beneficial effect of green financing on green productivity is higher and stronger among those provinces with significant social and economic conditions.
Moreover, implementing green financial policies may help improve green finance development. Kong et al. (Citation2021) examine the role of green financing in determining the green productivity for the urban areas during the outbreak of COVID-19 in the Chinese economy. It is inferred that green financing plays a significant role in green development. Naeem et al. (Citation2021) also observe that green productivity efficiency is significantly influenced by green finance. Moreover, financial institutions working in any region may influence and encourage the utilisation of green energy among different enterprises, which can improve production efficiency (Yoshino et al., Citation2019). Based on the above arguments, our study infers that GFIN and related facilities in growing or developed financial markets would generate sustainable results while controlling the adverse environmental impact.
In terms of control variables, the results show that government intervention positively influences green productivity, whereas unemployment negatively affects China. Several reasons have been found in the current literature to justify the positive role of GINT in explaining higher GTFP. For example, a significant role is observed from the government, specifically in economic construction and environmental governance, which helps to boost the green output. In this regard, government intervention would help generate green economic development. Another viewpoint regarding the positive nexus between government intervention and green productivity reflects that government may help in different sectors like energy and financial growth to align economic direction.
However, contrary to a positive association between governmental intervention and GTFP, the role of unemployment is negatively significant in the long-run analysis. It implies that higher unemployment reflects a tight economic situation which reflects weak stability in the economy. Ebersberger and Pyka (Citation2002) state that a higher level of unemployment in any economy negatively impacts economic development, hence impedes it. Meanwhile, with the higher UNER, there is a reduction in the production of goods and services, lower utilisation of natural resources, and low social productivity. Therefore, our study has added UNER as a second control variable to examine the real green productivity trend from China's context. Besides, the dummy variable year 2010 also reflects a positively significant impact on GTFP.
Additionally, also reports stability findings and covers that the error terms' normal distribution has been found, followed by no serial correlation in the study model while taking the GTFP as a key dependent variable. The stability analysis also reports no white heterogeneity in the study model while assuming the role of green financing and green innovation as key explanatory variables. In contrast, government intervention and unemployment are control variables. At the same time, the presence/absence of autocorrelation was tested through the Durbin Watson test, where the findings show a score of 2.008. As the value of DW states is near 2, it confirms no autocorrelation in the study data. Besides, the findings of CUSUM and CUSUMsq endorse the stability of the model.
The short-run results and presented in . The overall results are similar to long run, and suggest that GFIM and GINO positively influence GTFP in short-run. . It reconfirms that green financing and innovations help enhance green productivity. More specifically, it shows that a 1% increase in green financing and green innovation help in increasing the GTFP with the coefficients size of 0.208 and 0.119, respectively. However, both control variables, entitled governmental intervention and unemployment, show a positive(negative) impact on GTFP It indicates that both explanatory and control variables significantly determine the trends in green productivity of China. Xie et al. (Citation2021) argue that higher technological innovations increase production capacity in any economy by providing a pathway for the energy transition. Simultaneously, such technologies also help to improve the utilisation of efficient energy sources, leading to more green output. Therefore, with the positive nexus between technological innovation and efficient energy utilisation, the final outcome will be green productivity.
Table 6. Results bootstrapped ARDL cointegration analysis (short run).
On the other hand, Lee et al. (Citation2022) justify the positive impact of green financing on GTFP. Likewise, Liu et al. (Citation2021) explain that green financing promotes sustainable economic development where the role of technological innovation and industrial development is quite evident. Zhong and Li (Citation2020) focussed on the China’s economy and studied the association between financial development and GTFP using China’s provincial data. The results confirm that higher financial growth and government interventions facilitate the GTFP at the provincial level. Additionally, the short-run results show the coefficient for ECM is −0.208, significant at 1%. It justifies that any deviation from the steady state will be adjusted by 20.8% quarterly. Finally, diagnostic findings are presented in , where the Durbin Watson test reflects no autocorrelation in the study data. Meanwhile, there is a normal distribution, no issues of serial correlation, and heteroscedasticity among the study variables in the short run. Besides, both CUSUM and CUSUMsq also confirm the parameters' stability in the long run.
Finally, our results report the Granger Causality analyses between the key variables. found bidirectional causality between green financing and GTFP, green innovation and GTFP, governmental intervention, and GTFP. However, unidirectional causality exists between unemployment and GTFP, respectively.
Table 7. Results of Granger causality.
5. Conclusion and policy implications
This paper puts forward theoretical and empirical discussions based on green financing and green innovations while controlling the effect of governmental intervention and unemployment in determining the trends of China’s green factor productivity. A quarterly data from 2004 to 2018 was collected and empirically tested through advanced time-series estimations. Unit root properties have been initially examined through ADF and ZA tests, confirming that data become stationary with structural breaks at ADF (Δ) and ZA (Δ). Afterwards, cointegration analysis through BARDL estimation confirms that Fpss, TDV and TIV are statistically significant at 1%. Meanwhile, the excellent explanatory power of the model has been achieved, and the JB tests confirm the normal distribution of the data. Moreover, the long-run results demonstrate that green financing and innovation significantly enhanced green productivity in China over the past two decades. More specifically, historical reviews confirm that green funding in China is in the emerging phase, and still, there is significant potential for its growth. At the same time, green financing plays a direct role in promoting high-quality economic development. It is observed that higher development of green finance leads to robust allocation of ecological and financial resources and their economic regulations.
In addition, the role of green and ecological innovations is also a productive component through which green productivity growth is observed. Therefore, seeking a path of green growth is crucial for China's sustainable development, which is possible by focussing on green innovations both in the long and short run. Although a considerable amount of investment has been made by the Chinese government towards green innovations and related research and development, the critical question arises whether these efforts are consistent at the macro and micro levels. Based on the above findings, few relevant contributions and policy implications can be derived. Firstly, investment in green projects like clean energy at the macro and micro level by the government and individual firms is a consistent and strategic tool for green productivity. It can also motivate stakeholders to participate in ecological innovations and environmental sustainability. The benefits of green financing and innovations are observed in green productivity; policymakers and governmental representatives should add more incentives to promote this nexus while integrating the private sectors into their strategic policy formulation. These efforts will create an immense horizon for investors in sustainable finance, environmental activists, and communities to contribute to achieving green productivity growth.
Besides, this study also highlights some fundamental limitations. Firstly, the current research considers a limited period from 2004 to 2018 with quarterly observations. Secondly, only two explanatory variables (i.e., green financing and green innovations) have been added to the model to explore green total factor productivity trends. Thirdly, an overall perspective of green total factor productivity has been measured as a key dependent variable. Fourthly, only the Chinese economy has been considered a sample observation unit. Fifthly, linear association between the stated variables has been observed where there is a possibility of non-linear trends. Future studies are recommended to expand the study time, adding more explanatory variables like energy transition, different proxies of green financing and innovations, environmental regulations, and regional-level data for green productivity. Besides, it is also proposed that advanced-panel estimations for the various provinces of China or other regional economies may also be implemented to achieve a better policy formulation.
Disclosure Statement
No potential conflict of interest was reported by the author(s).
References
- Dong, K., Shu, L., & Ting, C. (2016). Financial structure, firm size and industry growth. International Review of Economics & Finance, 41, 23–39.
- Ebersberger, B., & Pyka, A. (2002). Innovation and sectoral employment: a trade–off between compensation mechanisms. Labour, 16(4), 635–665. https://doi.org/10.1111/1467-9914.00211
- Fakher, H. A., Panahi, M., Emami, K., Peykarjou, K., & Zeraatkish, S. Y. (2021). Investigating marginal effect of economic growth on environmental quality based on six environmental indicators: does financial development have a determinative role in strengthening or weakening this effect? Environmental Science and Pollution Research International, 28(38), 53679–53699. https://doi.org/10.1007/s11356-021-14470-9
- Fangming, X., Bing, Z., & Ning, W. (2021). Non-linear relationship between energy consumption transition and green total factor productivity: A perspective on different technology paths. Sustainable Production and Consumption, 28, 28–104. https://doi.org/10.1016/j.spc.2021.03.036.
- Gao, D., Li, G., & Yu, J. (2022). Does digitisation improve green total factor energy efficiency? Evidence from Chinese 213 cities. Energy, 247, 123395. https://doi.org/10.1016/j.energy.2022.123395
- Goh, C., Leong, C., Kasmin, K., Hii, P., & Tan, O. (2017). Students’ experiences, learning outcomes and satisfaction in e-learning. Journal of E-Learning and Knowledge Society, 13(2)
- Hu, Q., Feng, Y., & Xu, M. (2021). Are there heterogeneous impacts of air pollution on mental health? Frontiers in Public Health, 9, 33698–33712. https://doi.org/10.3389/fpubh.2021.780022
- Huang, D. (2022). Green finance, environmental regulation, and regional economic growth: from the perspective of low-carbon technological progress. Environmental Science and Pollution Research, 29(22), 33615–33698. https://doi.org/10.1007/s11356-022-18582-8
- IIAS. (2022). Green innovation in China. https://www.iias.asia/the-review/green-innovation-china.
- Johnes, J. (2006). Data envelopment analysis and its application to the measurement of efficiency in higher education. Economics of Education Review, 25(3), 273–288. https://doi.org/10.1016/j.econedurev.2005.02.005
- Khan, S. A. R., Yu, Z., & Umar, M. (2022). A road map for environmental sustainability and green economic development: An empirical study. Environmental Science and Pollution Research International, 29(11), 16082–16090.
- Kim, D., & Perron, P. (2009). Unit root tests allowing for a break in the trend function at an unknown time under both the null and alternative hypotheses. Journal of Econometrics, 148(1), 1–13. https://doi.org/10.1016/j.jeconom.2008.08.019
- Kong, Q., Shen, C., Li, R., & Wong, Z. (2021). High-speed railway opening and urban green productivity in the post-COVID-19: Evidence from green finance. Global Finance Journal, 49, 100645. https://doi.org/10.1016/j.gfj.2021.100645
- Kulshreshtha, M., & Parikh, J. K. (2002). Study of efficiency and productivity growth in opencast and underground coal mining in India: A DEA analysis. Energy Economics, 24(5), 439–453. https://doi.org/10.1016/S0140-9883(02)00025-7
- Le, H. P., & Ozturk, I. (2020). The impacts of globalisation, financial development, government expenditures, and institutional quality on CO2 emissions in the presence of environmental Kuznets curve. Environmental Science and Pollution Research International, 27(18), 22680–22697.
- Lee, C.-C., & Lee, C.-C. (2022). How does green finance affect green total factor productivity? Evidence from China. Energy Economics, 107, 105863. https://doi.org/10.1016/j.eneco.2022.105863
- Lee, C.-C., Zeng, M., & Wang, C. (2022). Environmental regulation, innovation capability, and green total factor productivity: New evidence from. China. Environmental Science and Pollution Research, 1–16.
- Li, H., He, H., Shan, J., & Cai, J. (2019). Innovation efficiency of semiconductor industry in China: A new framework based on generalised three-stage DEA analysis. Socio-Economic Planning Sciences, 66, 136–148. https://doi.org/10.1016/j.seps.2018.07.007
- Ling, G., Razzaq, A., Guo, Y., Fatima, T., & Shahzad, F. (2022). Asymmetric and time-varying linkages between carbon emissions, globalization, natural resources and financial development in China. Environment, Development and Sustainability, 24(5), 6702–6730.
- Li, X., Shu, Y., & Jin, X. (2022). Environmental regulation, carbon emissions and green total factor productivity: A case study of China. Environment, Development and Sustainability, 24(2), 2577–2597. https://doi.org/10.1007/s10668-021-01546-2
- Liu, Y., Lei, J., & Zhang, Y. (2021). A study on the sustainable relationship among the green finance, environment regulation and green-total-factor productivity in China. Sustainability, 13(21), 11926. https://doi.org/10.3390/su132111926
- Liu, K., & Lin, B. (2019). Research on influencing factors of environmental pollution in China: A spatial econometric analysis. Journal of Cleaner Production, 206, 356–364. https://doi.org/10.1016/j.jclepro.2018.09.194
- Liu, Y., Yang, Y., Li, H., & Zhong, K. (2022). Digital economy development, industrial structure upgrading and green total factor productivity: Empirical evidence from China’s cities. International Journal of Environmental Research and Public Health, 19(4), 2414. https://doi.org/10.3390/ijerph19042414
- McNown, R., Sam, C. Y., & Goh, S. K. (2018). Bootstrapping the autoregressive distributed lag test for cointegration. Applied Economics, 50(13), 1509–1521. https://doi.org/10.1080/00036846.2017.1366643
- Naeem, M. A., Nguyen, T. T. H., Nepal, R., Ngo, Q.-T., & Taghizadeh–Hesary, F. (2021). Asymmetric relationship between green bonds and commodities: Evidence from extreme quantile approach. Finance Research Letters, 43, 101983. https://doi.org/10.1016/j.frl.2021.101983
- Ng, C.-F., Yii, K.-J., Lau, L.-S., & Go, Y.-H. (2022). Unemployment rate, clean energy, and ecological footprint in OECD countries. Environmental Science and Pollution Research, 1–10. https://doi.org/10.1007/s11356-021-17966-6
- Nizam, R., Karim, Z. A., Rahman, A. A., & Sarmidi, T. (2020). Financial inclusiveness and economic growth: New evidence using a threshold regression analysis. Economic Research-Ekonomska Istraživanja, 33(1), 1465–1484. https://doi.org/10.1080/1331677X.2020.1748508
- OECD (2022). Green growth in action: China.
- Pesaran, M. H., Shin, Y., & Smith, R. J. (2001). Bounds testing approaches to the analysis of level relationships. Journal of Applied Econometrics, 16(3), 289–326. https://doi.org/10.1002/jae.616
- Razzaq, A., An, H., & Delpachitra, S. (2021). Does technology gap increase FDI spill-overs on productivity growth? Evidence from Chinese outward FDI in Belt and Road host countries. Technological Forecasting and Social Change, 172, 121050. https://doi.org/10.1016/j.techfore.2021.121050
- Razzaq, A., Sharif, A., Najmi, A., Tseng, M. L., & Lim, M. K. (2021a). Dynamic and causality interrelationships from municipal solid waste recycling to economic growth, carbon emissions and energy efficiency using a novel bootstrapping autoregressive distributed lag. Resources, Conservation and Recycling, 166, 105372. https://doi.org/10.1016/j.resconrec.2020.105372
- Ren, Y. (2020). Research on the green total factor productivity and its influencing factors based on system GMM model. Journal of Ambient Intelligence and Humanised Computing, 11(9), 3497–3508. https://doi.org/10.1007/s12652-019-01472-2
- Sarkodie, S. A., & Ozturk, I. (2020). Investigating the environmental Kuznets curve hypothesis in Kenya: A multivariate analysis. Renewable and Sustainable Energy Reviews, 117, 109481. https://doi.org/10.1016/j.rser.2019.109481
- Shan, S., Genç, S. Y., Kamran, H. W., & Dinca, G. (2021). Role of green technology innovation and renewable energy in carbon neutrality: A sustainable investigation from Turkey. Journal of Environmental Management, 294, 113004.
- Sharif, A., Baris-Tuzemen, O., Uzuner, G., Ozturk, I., & Sinha, A. (2020). Revisiting the role of renewable and non-renewable energy consumption on Turkey’s ecological footprint: Evidence from Quantile ARDL approach. Sustainable Cities and Society, 57, 102138. https://doi.org/10.1016/j.scs.2020.102138
- Song, M., Peng, L., Shang, Y., & Zhao, X. (2022). Green technology progress and total factor productivity of resource-based enterprises: A perspective of technical compensation of environmental regulation. Technological Forecasting and Social Change, 174, 121276. https://doi.org/10.1016/j.techfore.2021.121276
- Sun, Y., Guan, W., Razzaq, A., Shahzad, M., & An, N. B. (2022). Transition towards ecological sustainability through fiscal decentralisation, renewable energy and green investment in OECD countries. Renewable Energy, 190, 385–395. https://doi.org/10.1016/j.renene.2022.03.099
- Sun, Y., & Razzaq, A. (2022). Composite fiscal decentralisation and green innovation: Imperative strategy for institutional reforms and sustainable development in OECD countries. Sustainable Development. https://doi.org/10.1002/sd.2292
- Wang, K.-L., Pang, S.-Q., Zhang, F.-Q., Miao, Z., & Sun, H.-P. (2022). The impact assessment of smart city policy on urban green total-factor productivity: Evidence from China. Environmental Impact Assessment Review, 94, 106756. https://doi.org/10.1016/j.eiar.2022.106756
- Wang, K.-H., Umar, M., Akram, R., & Caglar, E. (2021). Is technological innovation making world "Greener"? An evidence from changing growth story of China. Technological Forecasting and Social Change, 165, 120516. https://doi.org/10.1016/j.techfore.2020.120516
- Wang, L., & Yan, Y. (2022). Environmental Regulation Intensity, Carbon Footprint and Green Total Factor Productivity of Manufacturing Industries. International Journal of Environmental Research and Public Health, 19(1), 553. https://doi.org/10.3390/ijerph19010553
- Wang, J., Zhang, S., & Zhang, Q. (2021). The relationship of renewable energy consumption to financial development and economic growth in China. Renewable Energy, 170, 897–904. https://doi.org/10.1016/j.renene.2021.02.038
- WorldBank (2022). Unemployment, total (% of total labour force) (modeled ILO estimate).
- Xie, F., Zhang, B., & Wang, N. (2021). Non-linear relationship between energy consumption transition and green total factor productivity: A perspective on different technology paths. Sustainable Production and Consumption, 28, 91–104. https://doi.org/10.1016/j.spc.2021.03.036
- Yoshino, N., Taghizadeh–Hesary, F., & Nakahigashi, M. (2019). Modelling the social funding and spill-over tax for addressing the green energy financing gap. Economic Modelling, 77, 34–41. https://doi.org/10.1016/j.econmod.2018.11.018
- Zhong, J., & Li, T. (2020). Impact of financial development and its spatial spill-over effect on green total factor productivity: Evidence from 30 Provinces in China. Mathematical Problems in Engineering, 2020, 1–11. https://doi.org/10.1155/2020/5741387
- Zhong, S., Li, Y., Li, J., & Yang, H. (2021). Measurement of total factor productivity of green agriculture in China: Analysis of the regional differences based on China. PLoS One, 16(9), e0257239. https://doi.org/10.1371/journal.pone.0257239
- Zhou, Y., Xu, Y., Liu, C., Fang, Z., Fu, X., & He, M. (2019). The threshold effect of China’s financial development on green total factor productivity. Sustainability, 11(14), 3776. https://doi.org/10.3390/su11143776