Abstract
Stakeholders in hospitality and tourism industries are involved in many decision-making scenarios. Multi-criteria decision-making (MCDM) methods have been widely used in hospitality and tourism industries. Although some articles summarised the applications of MCDM models in hospitality and tourism industries, they ignored the fuzziness of individual cognition in an uncertain environment. In addition, these surveys lacked a comprehensive overview from the perspective of bibliometrics analysis and content analysis regarding the whole hospitality and tourism industries. To analyse the applications of fuzzy MCDM methods in hospitality and tourism industries and further explore future research directions, this article reviews 85 selected papers published from 1997 to 2022 regarding fuzzy MCDM models applied in hospitality and tourism industries. Through analysing the results of bibliometric analysis, methodologies and applications, we found that analytic hierarchy process (AHP) and TOPSIS methods are the most widely used MCDM methods, and tourism evaluation, hotel evaluation and selection, tourism destination evaluation and selection are the most attractive research issues in hospitality and tourism industries. Finally, future research directions are proposed from three aspects. This article provides insights for researchers and practitioners who have interest in fuzzy MCDM models in hospitality and tourism industries.
1. Introduction
The hospitality and tourism industries are business patterns in which efforts are placed by decision-makers to attract visitors to support the sector’s developments (Kaushal & Srivastava, Citation2021). They make significant contribution to national economies by bringing much-needed investment, employment and tax revenue (Scheyvens & Hughes, Citation2019). According to the World Travel and Tourism Council, the hospitality and tourism industries provided about 330 million jobs worldwide and created more than 10% of the global GDP in 2019 (Huang & Baker, Citation2021). The hospitality and tourism industries have attracted much attention from researchers and practitioners (Zolfani et al., Citation2015; Litvin et al., Citation2018; Wut et al., Citation2021; Chen et al., Citation2022).
Multi-criteria decision-making (MCDM) is a process of evaluating a group of alternatives regarding multiple criteria according to the opinions of experts. It has been widely used to solve complicated decision-making problems (Chiao, Citation2021; Torkayesh et al., Citation2021; Baydaş et al., Citation2022). Due to the complex practical problems involved in hospitality and tourism industries, decision-makers need to compare multiple alternatives according to their performance and select the optimal scheme to maximise benefits. In this sense, MCDM methods are useful approaches to tackle decision-making problems in hospitality and tourism industries, which helps tourism institutions, hotel managers and tourists make reasonable decisions.
Considering the complexity of decision-making problems and the fuzziness of human cognition, decision-makers are often unable to express their opinions accurately. Zadeh (Citation1965) proposed fuzzy sets to characterise uncertain information. Atanassov (Citation1986) introduced the non-membership degree and extended fuzzy sets to intuitionistic fuzzy sets (IFSs). Atanassov (Citation1999) further proposed interval IFSs that used intervals to represent membership, non-membership and hesitancy degrees. Torra (Citation2010) proposed hesitant fuzzy sets, which argued that the membership degree is not a certain value but several possible values. Chen et al. (Citation2013) proposed interval hesitant fuzzy sets applying multiple intervals to denote membership degrees. Zadeh (Citation1975) introduced the concept of linguistic variables and advocated using natural language instead of exact values to express qualitative information. Nevertheless, the fuzzy linguistic method based on single linguistic terms is difficult to accurately describe the views of decision-makers. Since then, various forms of fuzzy linguistic sets, such as the hesitant fuzzy linguistic sets (Rodriguez et al., Citation2012), probabilistic linguistic term sets (Pang et al., Citation2016), and probabilistic double hierarchy linguistic term sets (Gou et al., Citation2021) have been proposed. Henceforth, many fuzzy technologies have been introduced into MCDM models, which expand the applications of MCDM methods. The hospitality and tourism industries have no exception. The hospitality and tourism industries now risk losing 98 − 197 million jobs worldwide due to the Covid-19 pandemic, which seriously affects economic development (Huang & Baker, Citation2021). How to use effective means to deal with decision-making problems involved in hospitality and tourism industries is a key issue in an unstable economic environment, especially for the stakeholders who are concerned about how to make scientific decisions to avoid losses and maximise benefits. Therefore, researching the current situation and future directions of fuzzy MCDM methods applied in hospitality and tourism industries is now a more critical issue than ever.
Using fuzzy MCDM methods to deal with management problems in hospitality and tourism industries has been researched a lot, but few studies have systematically reviewed the state-of-the-art research in this direction. Mardani, Jusoh, et al. (Citation2016) summarised the applications of MCDM methods in the tourism and hospitality industries. Duan et al. (Citation2021) overviewed the applications of MCDM methods in ecotourism and sustainable tourism. However, the first article only reviewed the papers published in 2014 and before. Since MCDM methods have received extensive attention in hospitality and tourism industries in recent years, it is necessary to update the number of publications. The second paper only summarised the applications of MCDM methods in ecotourism and sustainable tourism, without considering the whole hospitality and tourism industries. Moreover, both reviews ignored the uncertainty of decision-making problems and the subjectivity of human cognition, without considering fuzziness in decision-making problems. In addition, these surveys lacked a comprehensive overview from the perspective of bibliometrics analysis and content analysis.
To fill these research gaps, this study not only considers the fuzziness and uncertainty of the evaluation information provided by decision makers, but also takes into account the whole hospitality and tourism industries based on the work of Mardani, Jusoh, et al. (Citation2016) and Duan et al. (Citation2021). The primary concerns of our study are as follows:
What are the development status and research hotpots of fuzzy MCDM in hospitality and tourism industries?
What fuzzy MCDM methods have been used in hospitality and tourism industries?
What are the application domains of fuzzy MCDM in hospitality and tourism industries?
What are the future directions of fuzzy MCDM in hospitality and tourism industries?
The above issues are critical to learn the overall situation about fuzzy MCDM methods applied in hospitality and tourism industries. In view of the increasing academic attention to the applications of fuzzy MCDM methods in the unstable environment of the hospitality and tourism industries, it is necessary to summarise fuzzy MCDM methods applied in hospitality and tourism industries in order to obtain a systematic understanding in this research branch and find out hotspots and future development directions.
This article uses bibliometrics analysis and content analysis to reveal the research status and development trend of the applications of fuzzy MCDM models in hospitality and tourism industries. The retrieval formula TS = (‘tourism’ or ‘hospitality’) AND TS = (‘MCDM’ OR ‘Multi-Criteria’ OR ‘Multi Criteria’ OR ‘Multiple Criteria’ OR ‘Multiple-Criteria’ OR ‘MADM’ OR ‘Multi-Attribute’ OR ‘Multi Attribute’ OR ‘Multiple Attribute’ OR ‘Multiple-Attribute’ OR ‘MODM’ OR ‘Multi-Objective’ OR ‘Multi Objective’ OR ‘Multiple Objective’ OR ‘Multiple-Objective’ OR ‘MCDA’) AND TS = (‘fuzzy’) are used to search literature in the Web of Science Core Collection (WoSCC) database. It is worth noting that the main focus database is the WoSCC in this article, which includes several sub-databases such as Science Citation Index, and Conference Proceedings Citation Index. The database covers more than 15 thousand journals and 50 million papers and collects almost all the known scientific research (Merigó et al., Citation2015). Only considering the WoSCC database may result in omissions; however, other factors should also be taken into account, such as the simplicity of bibliometric analysis. Therefore, the WoSCC database was used to collect the data. It returned 320 records from 1992 to 2022 on 2 March 2022. The literature reviewed in this article must cover three topics simultaneously: fuzzy, MCDM and the hospitality and tourism industries. Therefore, the literature that meets only one or two part(s) of them is filtered out by analysing the keywords and abstracts of each article, and 85 records were finally obtained. We combine the articles talking about MCDM with the keywords ‘MCDM’ or ‘Multi criteria’ or ‘Multi criteria’ or ‘Multiple criteria’ or ‘Multiple criteria’ into one category. Similarly, those articles with the keywords ‘MADM’ or ‘Multi attribute’ or ‘Multi attribute’ or ‘Multiple attribute’ and ‘Multiple attribute’ are related to multi-attribute decision-making problems. The publications with the keyword ‘MODM’ or ‘Multi-Objective’ or ‘Multi Objective’ or ‘Multiple Objective’ or ‘Multiple-Objective’ are merged into one category about multi-objective decision-making. To conduct a bibliometric analysis, the title, author(s), keywords, source journal, field, abstract and references of publications are downloaded in the form of text.
In this article, we devote to reviewing these 85 articles about applications of fuzzy MCDM models applied in hospitality and tourism industries. The analysis procedure of this article is presented in . First, we conduct a bibliometrics analysis about publication and citation trends, region and institution distributions of reviewed publications, highly cited articles, categories of reviewed articles and keyword co-occurrence analysis. In addition, from the perspective of fuzzy MCDM methods and their applications, content analysis is used to conduct a systematical and comprehensive survey of fuzzy MCDM models applied in hospitality and tourism industries. From the method level, the selected publications are divided into those containing individual fuzzy MCDM methods and those with hybrid fuzzy MCDM methods according to the number of fuzzy MCDM methods used in the literature. From the application level, the application domains of fuzzy MCDM methods in hospitality and tourism industries are surveyed. Finally, future directions are proposed to provide reference and enlightenment for researchers and practitioners in this field.
The main goal of this article is to present a comprehensive literature review on the application of fuzzy MCDM methods in hospitality and tourism industries. Compared with existing studies, the main contributions of this article can be summarised into three aspects: (1) it reviews fuzzy MCDM methods in the whole hospitality and tourism industries; (2) the bibliometric and content analysis are combined to conduct a comprehensive overview of fuzzy MCDM methods and their applications in hospitality and tourism industries; (3) future research directions are proposed based on such a survey to provide insights for researchers and practitioners. The findings should make a contribution from a theoretical and practical perspective. From a theoretical viewpoint, the study contributes to establish ‘state-of-the-art’ academic research into the use of fuzzy MCDM methods in hospitality and tourism industries. To be specific, the results of bibliometrics analysis facilitate scholars to understand the development and research trends in this field and lay a foundation for further research. Second, by analysing the applications of fuzzy MCDM methods in hospitality and tourism industries, scholars can further expand fuzzy MCDM methods and use more advanced methods to solve problems in hospitality and tourism industries. Third, it is helpful to explore what hospitality and tourism problems were unsolved by fuzzy MCDM methods, and future research can focus on solving these problems. Fourth, research hotspots are also found through content analysis, so that scholars can understand the cutting-edge directions and keep up with research trends. From a practical perspective, the findings and suggestions will be of interest to industry practitioners looking for suggestions and best practices to deal with the challenges in hospitality and tourism industries.
The article is structured as follows: Section 2 introduces and analyses the results of bibliometric analysis, including publication trend, citation structure, the region and institution with the most publications, categories of reviewed papers, highly cited papers (CPs), and keywords co-occurrence. Section 3 reviews fuzzy MCDM methods applied in hospitality and tourism industries. Section 4 summarises various application areas in hospitality and tourism industries by means of fuzzy MCDM methods. Section 5 proposes future research directions and Section 6 closes this paper with concluding remarks.
2. Bibliometrics
Bibliometric analysis is an effective means to reveal the research status and development trend (Tang et al., Citation2021). It mainly uses mathematical and statistical methods combined with detailed information from publications to conduct a comprehensive analysis of a research field. Since there is no bibliometric study to analyse the applications of fuzzy MCDM models in hospitality and tourism industries, this section uses the bibliometric tool VOSviewer (Van Eck & Waltman, Citation2010) to study the research status and trend of fuzzy MCDM methods in hospitality and tourism industries.
2.1. Publications and citation trends
Analysing the quantity of publications and citations over time is helpful to grasp the development status and trend of a research field. reflects the number of publications (TP), CPs, the total number of citations (TC), citations per paper (C/P), citation of each CP (C/CP) and quantity of citations with different thresholds (200, 100, 50, 25 and 1). shows the development trends of publications and the citation of each CP.
Figure 2. Publication and citation trends from 1997 to 2022 (by 2 March 2022).
Source: Created by the authors based on the WoSCC database.
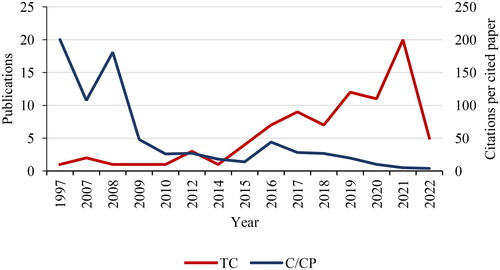
Table 1. Publication trend and citation structure from 1997 to 2 March 2022.
As shown in , the first article on the applications of MCDM models in hospitality and tourism industries appeared in 1997 with the highest citation of each CP. It may be due to the small number of papers in the early stage and the extensive attention paid to this research. Although the paper was highly cited, the research field has not been widely studied in the following 10 years since the first article was published. It was not until 2007 that experts began to revisit this research direction. In 2008, there was only one article and the number of citations was up to 185, leading the citations of each CP being raised again to a high level. In the following 7 years, the number of publications remained small. Until 2014, the quantity of publications increased significantly. The growth rate decreased in 2018. From 2020 to 2021, the growth rate reached the maximum, and the number of publications reached the highest in 2021. Although the number of publications decreased in 2018 and 2020, it showed an upward trend on the whole, which represented that this research field has gradually attracted wide attention. The quantity of citations shows a gradual downward trend despite small fluctuations. The citations of each CP are not directly related to the number of publications. Since 2014, the number of publications has shown an increasing trend, but the citations have gradually decreased. In 2021, the number of publications reached the maximum, but the citations are very small. As shown in , between 1997 and 2021 (2022 is excluded from the analysis as the citations do not cover the entire year), 2 papers published in 1997 and 2007 were cited more than 200 times, 3 papers were cited more than 100 times, 9 papers were cited more than 50 times, 21 papers were cited more than 25 times, and 71 papers were cited more than once.
2.2. Region and institution distributions of reviewed publications
This section implements a bibliometric analysis from the perspectives of regions and institutions to find out the geographical distribution of authors, which is beneficial for deep cooperation between scholars. displays the top 9 regions with the most publications.
Table 2. Top nine regions with the most publications.
As can be seen from , among the countries and regions with the most publications, China, Taiwan, and Spain rank in the top three. It is worth noting that among the 85 selected records, there is no publication from Hong Kong and Macao region. Thus, here China means China Mainland. China has the largest number of papers (28), accounting for 32.94% of the total publications, and the number of citations is up to 571, which proves that the research field is widely researched in China and has great influence in the world. Taiwan published 19 papers which account for 22.35% of all publications, and the number of citations reached the maximum (662). The next ones are Spain (10), Iran (9), Turkey (7), Lithuania (6), India (6), Serbia (5) and Malaysia (4). It is worth noting that China, Iran, Turkey, India, Serbia and Malaysia are developing countries, which proves that developing countries have made outstanding contributions to this research field.
shows the distribution of publications from different research institutions. From , it can be seen that the Vilnius Gediminas Technical University (5), Las Palmas Gran Canaria University (5) and Cent South University (5) have the largest number of publications. Combined with the publications of each country in , it is found that the Vilnius Gediminas Technical University is the main source of published papers in Lithuania. The publications in Spain are mainly from the Las Palmas Gran Canaria University. The following are Islamic Azad University (4), Southwestern University of Finance and Economics (4), Ming Chuan University (4), Sichuan Normal University (4) and Zhejiang University of Finance and Economics (4). On the whole, there is little difference in the number of publications among institutions, which proves that there are no particularly prominent research institutions in this research branch, and the number of publications is evenly distributed.
Table 3. Top 22 institutions with the most publications.
2.3. Categories of reviewed papers
In this section, we analyse the categories of reviewed publications. shows the categories of publications and the frequency of their occurrence. The category with the most publications is business and economics (22), which confirms the characteristics that the hospitality and tourism industries can promote economic development (Hu et al., Citation2021). The second frequent category is environmental sciences and ecology (21), which reflects the interdependent relationship between tourism and natural ecology (Liu & Suk, Citation2021). The third frequent category is computer science (20), which is related to the theoretical knowledge involved in fuzzy MCDM. The following frequent categories are social science (17), engineering (10), science and technology (9), mathematics (9), operations research and management science (7), public administration and public (3), and environmental and occupational health (3).
Table 4. Categories of reviewed papers.
exports by VOSviewer demonstrates publication volume and connections between categories. First, it can be seen that the circles of ‘business & economics’, ‘environmental sciences & ecology’ and ‘computer science’ are bigger than others, indicating that the number of papers in those categories is larger than those in other categories, which is consistent with the contents in . However, the publication volumes of ‘operations research & management’, ‘engineering’ and ‘science & technology’ are less, representing the applications of research in these fields have not been popularised and could be considered in future research. From the perspective of connections, we can find that the lines around ‘business & economics’ and ‘computer science’ are denser, which denotes that most papers regarded them as the center to conduct research. In addition, the categories of ‘operations research & management’, ‘environmental sciences & ecology’, and ‘sociology’ are linked with ‘business & economics’ closely and the categories related to ‘computer science’ mainly include ‘engineering’ and ‘operations research & management’, which shows that these fields are well integrated. In future research, scholars can focus on cross integration of other fields like ‘business & economics’ and ‘computer science’.
2.4. Top 10 highly cited publications
The number of citations reflects the dissemination and recognition of a paper in the research field (Lu et al., Citation2021). lists the title, authors, region, journal, year and citations of the top 10 highly CPs. The top three papers were published in 2007, 1997 and 2008, indicating that these papers published in the early stage attracted extensive attention and belong to the pioneering work in this field. The fourth and fifth-ranked papers were from China published in 2017 and 2018, respectively. Yu et al. (Citation2017, Citation2018) have contributed two highly CPs, which were published on International Transactions in Operational Research and International Journal of Fuzzy Systems, respectively. Besides, Wu et al. (Citation2018, Citation2019) have published two highly CPs which are ranked eighth and ninth. In terms of publication time, fie papers were published in the last five years, which proves that the research in recent years has received widespread concerns. In terms of publication region, China (Mainland) published four papers, which have made outstanding contributions in this field. There are three papers from Taiwan, and one paper from Spain, Vietnam, and Serbia, respectively. From the published journals, it is found that the top three papers were published in Tourism Management, Annals of Tourism Research and International Journal of Hospitality Management, which shows that these highly cited studies focused more on the applications in the field of hospitality and tourism industries.
Table 5. Top 10 highly cited publications.
2.5. Keywords co-occurrence
In this section, we extract the keywords’ co-occurrence information to analyse the current status and hotspots of this research direction. High-frequency words can help scholars understand what researchers are most concerned about, while low-frequency words provide a reference for researchers to uncover new ideas. In , the larger the circle is, the more frequently the keyword appears. The most frequent keywords are model, decision-making, tourism and management, indicating that many papers used decision-making models to solve tourism management problems. The frequent occurrence of fuzzy and fuzzy logic confirms the theme of this study. From the perspective of MCDM methods, TOPSIS appeared most frequently, which shows that the TOPSIS method has been widely used among all MCDM methods, followed by the analytic hierarchy process (AHP) and analytic network process (ANP) methods. Aggregation operators have also been mentioned many times, indicating that many papers used or innovated in aggregation operators. In terms of applications, service quality appeared more frequently, which means that fuzzy MCDM models have been applied to evaluate the service quality of hotels and tourism more often. The keyword selection also appeared many times, showing that there was literature regarding selection problems, such as hotel selection (Wang et al., Citation2021), destination selection (Hameed et al., Citation2022) and location selection (Ronizi et al., Citation2020). From the types of tourism, ecological tourism appears more frequently and represents the current research hotspot, followed by medical tourism.
3. Classification of fuzzy MCDM methods applied in hospitality and tourism industries
After investigating MCDM models used in reviewed papers, these models can be divided into individual fuzzy MCDM models and hybrid fuzzy MCDM models. The acronym of MCDM methods is given in .
Table 6. The acronym of MCDM methods.
3.1. Individual fuzzy MCDM methods
Individual fuzzy MCDM models can be divided into fuzzy MADM and fuzzy MODM methods. In the process of fuzzy MADM, experts select, classify, rank or prioritise a finite number of alternatives, while fuzzy MODM methods involve an infinite number of choices. shows the categories of fuzzy MCDM methods and corresponding references.
Table 7. Individual fuzzy MCDM models applied in hospitality and tourism industries.
Fuzzy MADM methods can be divided into seven categories, including pairwise comparison methods (16), distance-based methods (15), aggregation operator-based methods (8), interaction-based methods (5), compromise methods (2), value and utility measurement methods (1) and other methods (12).
3.1.1. Pairwise comparison methods
Sixteen papers were related to pairwise comparison methods.
Fourteen papers associated with fuzzy AHP method. Sheng Hshiung et al. (1997) used the fuzzy AHP method to rank tourism risks. They used linguistic term sets to express criteria performance, converted them into triangular fuzzy numbers (TFNs), applied AHP method to obtain weights of criteria by pairwise comparisons among criteria, and ranked the intensities of tourism risks of alternatives based on the COA method. Chou et al. (Citation2008) used the fuzzy AHP method to select hotel locations. They used TFNs to characterise linguistic values, combined fuzzy sets theory and hierarchical structure analysis to develop a fuzzy MCDM model. Lin et al. (Citation2010) proposed a fuzzy MCDM method to evaluate marketing strategy. They used TFNs to capture the uncertainty and fuzziness of expert knowledge and applied the AHP method to determine the weights of criteria. Chen et al. (Citation2014) proposed a two-step FAHP approach to evaluate the atmosphere of international hotels. They used the Delphi method to find out the factor influencing the atmosphere of international hotels, applied the FAHP method to assess the relative importance between criteria, and obtained the weights and ranks of criteria. Erfani et al. (Citation2015) applied AHP to obtain the weights of criteria. Finally, the weighted linear combination (WLC) was employed to calculate the suitability index and rank alternatives. Chen and Bau (Citation2016) used the fuzzy AHP model to calculate the weights of factors and associated attributes, which is helpful to reduce the subjectivity of decision-makers. Jusoh et al. (Citation2018) used the fuzzy Delphi method to obtain the evaluation information of experts, and then applied the FAHP method to determine the weights of criteria. Büyüközkan et al. (Citation2019) used IFSs to express evaluation information for eliminating uncertainties and vagueness, and applied the AHP method to determine the weights of criteria. Andria et al. (Citation2021) proposed a two-step fuzzy framework based on the FAHP to solve the problem of sustainability assessment. Vatankhah et al. (Citation2021) used TFNs to express experts’ opinion, and applied the fuzzy AHP method to obtain the weights of criteria and sub-criteria. Bueno et al. (Citation2021) incorporated a multi-granular fuzzy linguistic model into the recency, frequency and helpfulness (RFH) model to obtain customer opinion values, and used the fuzzy AHP method to rank a set of hotels. Erdenejargal et al. (Citation2021) combined a spatial MCDM method and the AHP method based on Boolean and fuzzy logic to assess natural landscape aesthetics. Omarzadeh et al. (Citation2022) proposed a geographic information systems (GISs)-based multi-criteria decision analysis model to evaluate ecotourism sustainability. They embedded the fuzzy logic theory into an MCDA process, and used the AHP method to determine the weights of criteria. Guru et al. (Citation2022) used the fuzzy AHP method to prioritise the medical tourism destination.
Two papers related to fuzzy ANP method. Aliani et al. (Citation2016) integrated GIS, fuzzy logic and ANP to determine suitable areas for ecotourism activities. The fuzzy logic and ANP were applied to weight criteria. Aliani et al. (Citation2017) combined the fuzzy logic and ANP method to evaluate the development of ecotourism, in which the ANP method was used to determine the weights of criteria and the WLC operator was applied to calculate the suitability to divide the region according to the ecotourism potential.
3.1.2. Distance-based methods
Fifteen papers dealt with distance-based methods.
Twelve papers associated with fuzzy TOPSIS method. Benítez et al. (Citation2007) used TFNs to represent linguistic terms, and applied the fuzzy TOPSIS method to rank the service quality of hotels. Huang and Peng (Citation2012) proposed an approach that applied the fuzzy Rasch model (Rasch, Citation1960) in TOPSIS to measure the competitiveness of tourism destinations. Chou et al. (Citation2012) used the fuzzy TOPSIS method to select medical providers, in which TFNs were used to express the importance of criteria and the distances of alternatives to the positive-ideal and negative-ideal solutions were calculated to determine the closeness coefficient for ranking alternatives. Saayman et al. (Citation2016) used TFNs to represent linguistic terms, and employed the fuzzy TOPSIS method to address the issue of service quality evaluation. Martín and Román (Citation2017) used TFNs to overcome the defects of Likert scales, and applied the TOPSIS method to rank alternatives. Skondras et al. (Citation2018) proposed a fuzzy MCDM method to select heritage route, where interval-valued trapezoidal fuzzy numbers were used to characterise the fuzzy evaluation information, and the trapezoidal fuzzy TOPSIS was applied to rank alternatives according to closeness coefficients. Pahari et al. (Citation2018) used IFSs to describe the vague information of decision-makers, and employed the TOPSIS method to select the most appropriate hotel. Carlos Martin et al. (2019) used TFNs to represent the information provided by respondents, and applied the TOPSIS method to obtain a synthetic index of satisfaction with tourist destinations for each segment. Martín et al. (Citation2020) used TFNs to characterise linguistic terms and the fuzzy TOPSIS method to calculate positive and negative ideal solutions. Martin et al. (Citation2021) transformed the information provided by the questionnaire sample into TFNs to overcome information distortion, and then used the TOPSIS method to calculate the satisfaction indicator for visitors. Hameed et al. (Citation2022) used the fuzzy TOPSIS method to select tourism destinations, and TFNs were used to represent the five-point scale of linguistic variables. Forouzandeh et al. (Citation2022) combined the artificial bee colony (ABC) algorithm and fuzzy TOPSIS method to develop an approach of recommendation in the tourism industry. The fuzzy TOPSIS method was used to calculate the positive ideal solution while the ABC algorithm was applied to search the destinations according to the distance to the positive ideal solution.
Two papers were related to the fuzzy VIKOR method. Wu et al. (Citation2019) considered the bounded rationality of decision-makers in the VIKOR method, and extended the VIKOR method to the MCDM framework under the interval-valued intuitionistic fuzzy (IVIF) environment. Krishnan et al. (Citation2021) developed a method named interval type 2 trapezoidal‐fuzzy weighted with zero inconsistency (IT2TR‐FWZIC) to identify the significant impact of each smart key concept, and then combined it with the VIKOR method to rank benchmarked applications in each category.
One paper was associated with fuzzy MABAC method. Yu et al. (Citation2017) defined the likelihood of IT2FNs (interval type-2 fuzzy sets). On this basis, they proposed an MABAC model to address MCDM problems.
3.1.3. Aggregation operator-based methods
There were eight papers related to aggregation operator-based methods. Qin (Citation2017) extended the Hamy mean operator into the interval type-2 fuzzy environment and developed the symmetric triangular interval type-2 fuzzy Hamy mean (STIT2FHM) and weighted symmetric triangular interval type-2 fuzzy Hamy mean (WSTIT2FHM) operators to aggregate interval type-2 fuzzy information, and ranked alternatives according to the overall performance value. Liu and Wang (Citation2017) developed a dependent hesitant fuzzy linguistic geometric Bonferroni mean (DHFLGBM) operator to solve MCDM problems under the hesitant fuzzy linguistic environment. Wu et al. (Citation2018) used the Hamy mean operator and Dombi operators to develop Hamy mean operators under the IVI fuzzy environment, and proposed two models based on the interval-valued intuitionistic fuzzy weighted Dombi Hamy mean (IVIFWDHM) and interval-valued intuitionistic fuzzy weighted dual Dombi Hamy mean (IVIFWDDHM) operators to solve MCDM problems. Peng et al. (Citation2019) developed the generalised weighted single-valued neutrosophic power Shapley Choquet average (GWSVNPSCA) and generalised weighted single-valued neutrosophic power Shapley Choquet geometric (GWSVNPSCG) operators based on the power aggregation operator and Shapley fuzzy measure, which considered the correlation between criteria and reduced the impact of abnormal evaluation values. Meanwhile, they proposed a fuzzy MCDM method to solve the problem of single-valued neutrosophic with incompletely known weights. Ling (Citation2019) used the hesitant fuzzy linguistic weighted average (HFLWA) operator to develop a fuzzy MCDM framework for measuring government administrative power. Sheng et al. (Citation2019) proposed an fuzzy Hamacher correlated geometric (FHCG) operator to rank leisure tourism cities. He et al. (Citation2020) used the power average operator to overcome the negative effect of extreme values, introduced the Bonferroni mean operator into the q-rung orthopair fuzzy environment to investigate the interactive relations between criteria, and developed an MCDM model using the q-rung orthopair power Bonferroni mean (q-ROPBM) operator to select investment partners. Wu et al. (Citation2020) proposed Dombi Heronian operators based on IVIFNs, and developed two MADM models with IVIFWDHM and IVIFWDGHM operators.
3.1.4. Interaction-based methods
Five papers were related to fuzzy DEMATEL methods. Jeong et al. (Citation2016) used the fuzzy DEMATEL method to evaluate the suitability of rural housing. Gigović et al. (Citation2016) developed a model combining GIS and MCDM methods to evaluate the suitability classes of ecotourism potentials in the Dunavski kljuc region, where the fuzzy DEMATEL method was used to determine the relative importance of criteria. Ozturkoglu et al. (Citation2021) employed the fuzzy DEMATEL method to explore the causal relations among criteria and identify the order of importance of criteria. MerdİVencİ and KarakaŞ (Citation2020) used linguistic terms to describe the relative importance between criteria, and then employed the DEMATEL method to explore the causality between criteria. Kaymaz et al. (Citation2021) used the GIS and fuzzy DEMATEL method to evaluate the suitability of Uzundere in terms of ecotourism, in which the fuzzy DEMATEL model was used to measure the causal relations between criteria.
3.1.5. Value and utility measurement methods
One paper was associated with fuzzy TODIM methods. Abrishamchi et al. (Citation2019) developed a decision-making framework combining the Delphi method, fuzzy set theory, and TODIM method to evaluate sustainable island-based tourism development. The Delphi method was adopted to determine the criteria by experts, while the TODIM method considering the risk preference of decision makers was used to rank alternatives.
3.1.6. Compromise methods
Two papers dealt with fuzzy MARCOS methods. Mijajlović et al. (Citation2020) presented a hybrid MCDM framework combining the full consistency method (FUCOM) and fuzzy MARCOS model to evaluate spas service, where the FUCOM model was used to determine the weights of criteria and the MARCOS model was applied to rank the spas. Puška et al. (Citation2021) used the FUCOM model to determine the weights of criteria, and applied the MARCOS model to rank rural settlements in terms of tourism potential.
3.1.7. Other methods
Mishra et al. (Citation2022) proposed a decision framework combining interval-valued intuitionistic fuzzy sets (IVIFSs) and ARAS to find a solution for an MCDM problem. They developed a similarity measure for IVIFSs to calculate the weights of criteria. Lin and Wang (Citation2017) aggregated individual decision matrices with linguistic terms into a group triangular fuzzy decision matrix, and developed a linear programming model to find an optimal criteria weight vector considering the incomplete criteria weight information. Yang et al. (Citation2017) used neutrosophic fuzzy preference relations (NFPRs) to characterise the preferences of tourists and developed some operators for aggregating the NFPRs, and proposed an MCGDM method to prioritise medical tourism destinations. Wei et al. (Citation2020) developed a MAGDM framework with pythagorean fuzzy sets. They combined a taxonomy method and PFS to compare different alternatives, and used the entropy method to obtain the weights of criteria. Hu (Citation2009) used TFNs to describe the preferences of evaluators, applied a genetic algorithm to determine the weights of criteria, constructed a hierarchical network, and selected the key criteria affecting the quality of electronic service. Stanujkic et al. (Citation2015) used IFSs to express uncertain information and rank websites. Fu and Tzeng (Citation2016) used a five-scale fuzzy linguistic scale to depict the evaluation information of experts, applied geometric mean to determine fuzzy weights, and adopted the COA defuzzification to convert a fuzzy number into a crisp value. Lian et al. (Citation2017) used TFNs to construct a fuzzy evaluation matrix and ranked tourism websites according to the calculated comprehensive expectation values. Liu and Gao (Citation2017) used the 2-tuple linguistic model to characterise fuzzy information and constructed a house of quality to calculate the weights of criteria and rank alternatives. Yang and Wang (Citation2020) used intuitionistic fuzzy preference relations (IFPRs) to describe evaluators’ pairwise comparison results, and proposed a hierarchical MCDM method to determine the comprehensive score of schemes based on intuitionistic fuzzy value. Zhang et al. (Citation2022) used q-ROFSs to express fuzzy information, and employed the thermodynamic feature-based model to rank alternative low-carbon tourism destinations. Wang et al. (Citation2021) used the maximum deviation method to determine the weights of criteria based on online reviews and ranked hotels by closeness coefficients.
One paper was devoted to solving fuzzy MODM problems. Huang et al. (Citation2007) developed a fuzzy MODM framework for assessing enterprise computing solutions in international tourism, in which the fuzzy sets were used to represent the cost data.
3.2. Hybrid fuzzy MCDM methods
The hybrid fuzzy MCDM models include two or more MCDM methods with fuzzy environment. shows the hybrid fuzzy MCDM methods and corresponding references.
Table 8. Hybrid fuzzy MCDM methods applied in hospitality and tourism industries.
3.2.1. Fuzzy AHP with other methods
Nine papers combined fuzzy AHP with other MCDM methods. Mardani, Zavadskas, et al. (Citation2016) suggested a framework of combining the fuzzy Delphi method, fuzzy TOPSIS and fuzzy AHP method to evaluate energy-saving technologies of Iranian hotels, where the fuzzy Delphi method was used to determine energy-saving technology and design questionnaire, the fuzzy AHP method was applied to determine weights of technologies, and the fuzzy TOPSIS method was employed to rank Iranian hotels and select the optimal options. Vahdat et al. (Citation2018) combined the AHP and TOPSIS methods to assess the service quality of hotels. They used the fuzzy logic and membership function to express linguistic variables and applied AHP method to determine the weights of criteria. Then, the fuzzy TOPSIS method was employed to evaluate the performance of one modern and one atmospheric hotel according to the weights of criteria. Rashmi et al. (Citation2019) used the fuzzy AHP method to determine the weights of criteria and applied the TOPSIS method to rank alternatives. Arsić et al. (Citation2017) proposed a hybrid SWOT-AHP-FANP model to identify priority strategies of sustainable development in ecotourism, where the SWOT method was used to determine the criteria and sub-criteria, the AHP and FANP methods were applied to evaluate the weights of criteria and rank strategies, respectively. Horng et al. (Citation2018) assessed the corporate social responsibility (CSR) practice in the tourism industry by the fuzzy Delphi method and DEMATEL-based AHP model. The opinion given by experts are collected by the fuzzy Delphi method and expressed in the form of TFNs, and then the DEMATEL-based AHP model was applied to determine the weights of factors and explore the causal relations between different factors. Roy et al. (Citation2018) proposed an MCDM model to prioritise medical tourism destinations. They used rough numbers to aggregate the judgments of experts, applied the rough AHP method to determine the relative importance of criteria, and adopted the rough MABAC to assess the alternative sites based on criteria weights. Ronizi et al. (Citation2020) combined the fuzzy quantifier algorithm, AHP method and ordered weighted averaging (OWA) operator to identify a suitable travel location. The fuzzy quantifier algorithm was used to define the membership function, the AHP method was applied to calculate the weights of input data and the OWA operator was used to determine the weights of criteria. Zhu et al. (Citation2021) used the fuzzy AHP-PROMETHEE method to rank the risk elements of new-build river cruise ships. They used IFSs to express evaluation information, employed the AHP method to determine the weights of criteria, and adopted the PROMETHEE method to rank risk factors. García Mestanza and Bakhat (2021) developed a fuzzy hybrid MCDM framework based on the AHP and MAIRCA model, where an improved fuzzy AHP method was developed to assess the importance of criteria and a modified fuzzy MAIRCA method was applied to rank alternative municipalities.
3.2.2. Fuzzy ANP with other methods
There were five papers that combined the fuzzy ANP with other MCDM methods. Liao et al. (Citation2019) developed a hybrid MCDM framework combining the fuzzy Delphi method, DEMATEL and ANP method in which the fuzzy Delphi method was used to determine the evaluation criteria of online travel agencies, the DEMATEL method was used to extract the interrelationships of criteria, and the ANP method was used to rank alternatives. Chou et al. (Citation2021) used the fuzzy Delphi method to obtain the opinions of experts, applied the DEMATEL method to analyse the interrelationships of criteria, and employed the ANP method to determine the weights of criteria. Ho et al. (Citation2021) combined fuzzy DEMATEL method and fuzzy DEMATEL-based ANP method to measure green marketing orientations. The DEMATEL method was used to construct an influence relation matrix and explore causal relations between criteria. The DEMATEL-based ANP method was applied to determine the weights of influence for each factor. Chang (Citation2015) proposed a hybrid model combining the fuzzy Delphi method, ANP and TOPSIS method to select the optimal public relation personnel for the tourism industry. They used the Delphi method to determine criteria, the ANP method to obtain the weights of criteria, and the TOPSIS method to rank alternatives. Tian and Peng (Citation2019) integrated the ANP model and TODIM model to solve the problem with unknown weights of criteria, where the ANP method was used to determine the weights of criteria, and the TODIM method was used to select optimal tourism attractions.
3.2.3. Fuzzy VIKOR with other methods
Four papers combined the fuzzy VIKOR with other MCDM methods. Peng and Tzeng (Citation2012) used DEMATEL-based ANP method to construct the influential matrix among criteria, and applied the VIKOR method to evaluate tourism competitiveness to determine the competitiveness gaps and explore development strategy based on the influential relation map. Mi et al. (Citation2019) developed a decision-making framework that combined the score function of q-rung orthopair fuzzy sets, the BWM and VIKOR method. Çalık (Citation2020) used the BWM method to acquire the weights of criteria, and then applied the fuzzy VIKOR method to rank social media platforms. Hosseini et al. (Citation2021) used the fuzzy DEMATEL method to construct the fuzzy influential network diagram and determine the fuzzy influential weights, and then employed a modified fuzzy VIKOR method to assess tourism risks.
3.2.4. Fuzzy SWARA with other methods
Two papers combined the fuzzy SWARA with other MCDM methods. He et al. (Citation2021) developed a decision framework combining the SWARA and MULTIMOORA methods with interval-valued pythagorean fuzzy sets. The SWARA model was used to obtain criteria weights, while the MULTIMOORA method was applied to rank sustainable community-based tourism (CBT) options. Ghasemi et al. (Citation2021) proposed an MCDM tool combining the fuzzy SWARA and PROMETHEE methods to rank tourism destinations. They used the SWARA method to determine the weights of criteria and employed the PROMETHEE method to prioritise medical tourism destinations.
3.2.5. Fuzzy TOPSIS with other methods
Three papers combined the fuzzy TOPSIS with other MCDM methods. Nilashi et al. (Citation2019) combined the DEMATEL and TOPSIS methods to identify priority preparation factors of hotels that influence the adoption of medical tourism services, where the DEMATEL method was used to reveal the correlation between preparation factors and the fuzzy TOPSIS method was applied to determine the importance of factors. Kilic et al. (Citation2021) proposed a hybrid decision-making framework to assess hotel information systems. They used the IVIF-DEMATEL model to determine the weights of criteria, and employed the IVIF-TOPSIS model to rank hotel information systems. Fu et al. (Citation2020) combined the nominal group technique (NGT), fuzzy TOPSIS and MCGP method to select hotels for airlines. They used the NGT to determine criteria, applied the TOPSIS method to calculate the weights of criteria and employed the MCGP method to select the best hotel.
3.2.6. Fuzzy TODIM with other methods
One paper combined the fuzzy TODIM with other MCDM methods. Yu et al. (Citation2018) developed a hybrid MCDM model combining the TODIM method and NLP with intuitionistic linguistic numbers. They defined operations and the generalised distance measure for ILNs. On this basis, an NLP-based TODIM method was applied to rank hotels.
3.2.7. Fuzzy PROMETHEE with other methods
One paper combined the fuzzy PROMETHEE with other MCDM methods. Ostovare and Shahraki (Citation2019) used the fuzzy Delphi method to determine criteria and sub-criteria, Shannon entropy to weight the criteria, and the PROMETHEE and GAIA methods to rank hotel websites.
3.3. Observations
In individual fuzzy MCDM models, 14 papers used the AHP method to determine the weights of criteria, 12 papers used the TOPSIS method to prioritise alternatives, 8 papers proposed aggregation operators, 5 papers used the DEMATEL method to explore the causal relationships between criteria, 2 papers used the VIKOR method to rank alternatives, 2 papers used the MARCOS method to select the optimal alternatives, 2 paper used the ANP method to determine criteria weights, 1 paper used the TODIM method to describe the preferences of decision-makers, 1 paper used the MABAC method to deal with MCDM problems, 1 paper constructed a LP model and the remaining 12 papers used other MCDM models. The frequency distribution of MCDM methods is shown in .
In hybrid fuzzy MCDM methods, the AHP method was the most widely applied method combined with other methods, with a total of 9 papers. The second is the ANP method, with a number of 8. Followed by TOPSIS and DEMATEL methods, with a total of both 7 papers. There are 4 and 3 papers separately on the combination of VIKOR, PROMETHEE with other methods. There are 2 papers on TODIM, 2 papers on BWM, and 2 papers on SWARA combined with other methods.
4. Applications of fuzzy MCDM methods in hospitality and tourism industries
A variety of fuzzy MCDM methods have been applied to solve decision-making problems in hospitality and tourism industries. This section analyses the application areas of fuzzy MCDM methods in the field of hospitality and tourism industries based on the collected data source.
4.1. Data source analysis
By investigating the data source of 85 reviewed papers, we found that the data source in 59 papers came from expert evaluation, among which 27 papers obtained evaluation information by issuing questionnaires to experts. In 32 papers experts directly gave evaluation opinions without questionnaire surveys. A total of 39 papers obtained data through questionnaire surveys and the respondents included not only experts, but also tourists, users, tour guides and hotel managers. The Likert rating scale was a commonly used survey method in these studies. Seven papers studied online travel reviews, and the data were from the website TripAdvisor.com. Five papers acquired data by interviewing with users, tourists and customers. Other data sources mainly included Alexa, Expedia.com and Allmedicaltourism.com.
4.2. Application areas in hospitality and tourism industries by means of fuzzy MCDM methods
The applications of fuzzy MCDM models applied in hospitality and tourism industries can be divided into the following aspects: tourism evaluation, hotel evaluation and selection, tourism destination evaluation and selection, strategic assessment and selection, tourism enterprise evaluation and selection, location selection from the perspective of land distribution, risk assessment, satisfaction evaluation and other problems. lists the application areas in hospitality and tourism industries by means of fuzzy MCDM methods and corresponding references.
Table 9. Application areas in hospitality and tourism industries by means of fuzzy MCDM methods.
4.2.1. Tourism evaluation – current situation, potential, service quality and influencing factors
There were twenty papers dealing with tourism evaluation.
Nine papers were related to the evaluation of tourism status. Peng and Tzeng (Citation2012) used the DEMATEL-based ANP and VIKOR methods to evaluate and identify the critical criteria affecting tourism competitiveness. Lian et al. (Citation2017) applied an MCDM method based on TFNs to evaluate and prioritise tourism websites according to a comprehensive expectation. Liu and Wang (Citation2017) employed an aggregation operator-based method to assess and rank ecotourism environmental carry capacity. Wu et al. (Citation2020) assessed the ecological value of forest ecological tourism demonstration based on an aggregation operator-based method. Mijajlović et al. (Citation2020) combined the FUCOM and fuzzy MARCOS method to assess the advantages and disadvantages of spas for improving spas service, so as to enhance the competitiveness of spas. Martín et al. (Citation2020) applied the fuzzy TOPSIS method to evaluate the comprehensive level of agreement that Islamic finance contributed to the development of halal tourism. He et al. (Citation2021) proposed a framework combining the SWARA and MULTIMOORA methods for evaluating the current status of sustainable CBT in the Indian-Himalayan region. García Mestanza and Bakhat (2021) conducted an over-tourism assessment based on the AHP and MAIRCA methods to help a tourism decision-maker identify over-tourism and formulate an optimal strategy. Erdenejargal et al. (Citation2021) used the fuzzy AHP method to evaluate natural landscape aesthetics in Mongolia.
Six papers evaluated the potential of tourism development, among which four papers were related to ecotourism, one paper was on rural tourism, and one paper measured the natural potential of tourism. Erfani et al. (Citation2015) used the fuzzy AHP method to evaluate the natural potential of regions for tourism. Gigović et al. (Citation2016) identified and evaluated zone suitability of ecotourism based on the fuzzy DEMATEL method. The result provided support for planners in ecotourism management. Kaymaz et al. (Citation2021) employed the fuzzy DEMATEL method to evaluate the potential of Uzundere distinct in terms of ecotourism. Omarzadeh et al. (Citation2022) applied the fuzzy AHP method to assess the potential of ecotourism development and identified the region with the greatest potential of ecotourism development, which helped decision-makers manage the region and provide a better service in sustainable ecotourism. Aliani et al. (Citation2017) evaluated and identified potential regions for the development of ecotourism based on the fuzzy ANP method. Puška et al. (Citation2021) combined the fuzzy FUCOM and MARCOS methods to evaluate and rank rural tourism potential.
There were three papers associated with the evaluation of service quality of tourism. To assess the service quality of tourism websites, Hu (Citation2009) determined a hierarchical network including 12 aspects and 45 criteria after reviewing the literature and collecting expert opinions and used the genetic algorithm to calculate the weights of criteria, then explored the critical criteria that had an impact on consumer satisfaction and were helpful for tourism websites to improve the quality of e-commerce. Stanujkic et al. (Citation2015) measured website quality in the rural tourism industry with IFSs. Saayman et al. (Citation2016) evaluated service quality in national parks based on the fuzzy TOPSIS method. A questionnaire was conducted to measure service quality.
Two papers assessed the influence factors of tourism. Chen and Bau (Citation2016) explored the priority factors for beach management using the AHP method. Through expert interviews and literature reviews, the factors affecting the beach environment were determined, and four dimensions were extracted through factor analysis. The results showed that the cleanliness and safety of beach environment were relatively important factors. MerdİVencİ and KarakaŞ (Citation2020) employed the fuzzy DEMATEL method to assess the factors affecting health tourism performance.
4.2.2. Hotel evaluation and selection
Nineteen papers were associated with hotel evaluation and selection.
Five papers selected appropriate hotels for tourists and airlines. Yu et al. (Citation2017) used the fuzzy MABAC method to select the most suitable hotel on TripAdvisor.com based on existing data in terms of location, service, facilities, price and comfort level. Pahari et al. (Citation2018) applied the fuzzy TOPSIS method to select hotels by analysing online reviews on a travel website. Yu et al. (Citation2018) combined the fuzzy TODIM and NLP methods to select the most desirable hotel from TripAdvisor.com. Fu et al. (Citation2020) combined the fuzzy TOPSIS and MCGP methods to select hotels for airlines to explore potential customers. Wang et al. (Citation2021) employed the maximum deviation method to select suitable hotels according to the preferred characteristics of tourists regarding location, cleanliness, comfort level, sleep quality, value and service.
Ten papers evaluated the management in the hotel industry. Chen et al. (Citation2014) applied the fuzzy AHP method to evaluate and rank the criteria influencing the atmosphere of international hotels. Fu and Tzeng (Citation2016) used the fuzzy COA method to evaluate the safety management for hot spring hotels. Jusoh et al. (Citation2018) applied the fuzzy AHP method to evaluate the critical success factors of total quality management in the hotel industry. Ostovare and Shahraki (Citation2019) combined the fuzzy PROMETHEE and GAIA methods to evaluate the quality of hotel websites and e-services of five-star hotels. Mi et al. (Citation2019) combined the fuzzy BWM and VIKOR methods to evaluate the brands of hospitality and rank the alternative hotels. Ozturkoglu et al. (Citation2021) used the fuzzy DEMATEL method to define the dimensions for sustainability-oriented hospitality service innovation in the food and beverage industry. The result showed that environmental entrepreneurship, interior design, and brand management dimensions should be paid more attention. Nilashi et al. (Citation2019) combined the fuzzy DEMATEL and TOPSIS methods to investigate the preparation factors for the adoption of medical tourism services by hotel firms. The results showed that technical and human factors were more important than organisational and environmental factors. Vatankhah et al. (Citation2021) employed the fuzzy AHP method to evaluate and rank the factors affecting the stress of employees in hospitality and tourism industries. Bueno et al. (Citation2021) evaluated tourism services based on the fuzzy AHP method to rank hotels by considering the customer opinion values obtained by the RFH model. Kilic et al. (Citation2021) assessed and selected appropriate hotel information systems based on DEMATEL and TOPSIS methods.
Three papers evaluated the service quality of hotels. Benítez et al. (Citation2007) applied the fuzzy TOPSIS method to evaluate the service performance of three hotels in a corporation for designing marketing strategies. Martín and Román (Citation2017) evaluated the service quality of hotels in Gran Canaria based on the fuzzy TOPSIS method. Vahdat et al. (Citation2018) combined the fuzzy AHP and TOPSIS methods to evaluate and rank the features influencing the service quality in the hotel industry.
One paper involved the location selection of hotels. Chou et al. (Citation2008) selected international tourist hotel locations in Taiwan based on the fuzzy AHP method.
4.2.3. Tourism destination evaluation and selection
Seventeen papers were associated with tourism destination evaluation and selection.
Four papers regarded the selection of medical tourism destinations. Yang et al. (Citation2017) developed an MCGDM framework to evaluate and prioritise medical tourism destinations. Roy et al. (Citation2018) combined the fuzzy AHP and MABAC methods to assess and select the most appropriate medical tourism destinations in India. Ghasemi et al. (Citation2021) combined the fuzzy SWARA and PROMETHEE methods to prioritise medical tourism destinations. Guru et al. (Citation2022) analysed the related factors of medical tourism and prioritised candidate medical tourism destinations based on the fuzzy AHP method.
Three papers dealt with low-carbon tourism destination selection. Lin and Wang (Citation2017) developed a GDM framework to rank and select low-carbon tourism destinations. Wei et al. (Citation2020) proposed an MAGDM framework to select low-carbon tourism destinations. Zhang et al. (Citation2022) selected a low-carbon tourism destination based on the thermodynamic feature-based model.
There were six papers regarding the selection of the most appropriate tourism destination according to the factors such as sustainable performance value and competitiveness. Huang and Peng (Citation2012) used the fuzzy TOPSIS method to analyse the competitiveness of tourism destinations in nine Asian countries, and the evaluation system involved 6 criteria and 15 indices. The results showed that China, Japan and Hong Kong are the most competitive countries/regions in tourism. Qin (Citation2017) ranked the alternatives of tourism destinations and selected the most desirable one based on the aggregation operator-based method. Andria et al. (Citation2021) applied the fuzzy AHP method to evaluate and rank tourist destinations from the perspective of sustainability. Rashmi et al. (Citation2019) combined the fuzzy AHP and TOPSIS methods to select optimal travel destinations in India. Forouzandeh et al. (Citation2022) employed the fuzzy TOPSIS method to develop an approach to recommend suitable tourist destinations to different types of tourists according to their preferences. Hameed et al. (Citation2022) selected the optimal tourism destination in Baluchistan based on the fuzzy TOPSIS method.
One paper focused on the quality evaluation of tourism destinations. Wu et al. (Citation2018) applied the aggregation operator-based method to evaluate the quality of elderly tourism services in the tourism destination.
Three papers were related to the evaluation of tourist attractions, of which two papers selected tourist attractions. Tian and Peng (Citation2019) selected an optimal tourism attraction using the fuzzy ANP and TODIM methods to provide personalised tourism recommendations for tourists. Peng et al. (Citation2019) selected tourism attractions based on the aggregation operator-based method, which improved the accuracy of personalised travel recommendations. The rest paper evaluated tourist attractions. Yang and Wang (Citation2020) proposed a hierarchical MCDM method to determine the comprehensive scores of schemes, and employed the method to evaluate the performance and efficiency of low-carbon tourist attractions. The obtained weights and sorting results provided references to the managers of scenic spots in formulating decisions.
4.2.4. Strategy assessment and selection
There were nine papers regarding strategic evaluation.
Three papers focused on marketing strategies. Lin et al. (Citation2010) used the fuzzy AHP method to identify the most important marketing resources and capabilities for marketing strategy in private hotels. Sheng et al. (Citation2019) assessed brand marketing of leisure tourism cities based on the aggregation operator-based method. Abrishamchi et al. (Citation2019) applied the fuzzy TODIM method to assess the development of Hendourabi Island (Iran) for tourism and identify the best development plan.
Two papers discussed development strategies for sustainable tourism. Arsić et al. (Citation2017) combined the fuzzy AHP and fuzzy ANP methods to identify the priority strategies of sustainable development in ecotourism according to the criteria and sub-criteria determined by the SWOT method. Ho et al. (Citation2021) combined the fuzzy DEMATEL and DEMATEL-based ANP methods to assess green marketing orientations in terms of sustainability.
Some scholars addressed tourism strategies from the perspective of low carbon and energy saving. For example, Mardani, Zavadskas, et al. (Citation2016) combined the fuzzy AHP and TOPSIS methods to assess and rank the energy-saving technologies and solutions in Iranian hotels, and the criteria were obtained by a literature survey and the fuzzy Delphi method. Mishra et al. (Citation2022) applied the fuzzy ARAS method to assess and select the most desirable low-carbon tourism strategy from the perspective of sustainability.
There were special topics regarding the strategy assessment in the tourism industry. Huang et al. (Citation2007) developed a fuzzy MODM framework to evaluate enterprise computing solutions in international tourism. Chou et al. (Citation2021) employed the fuzzy DEMATEL and ANP methods to assess the hospitality strategies for innovation development and identify the critical criteria influencing innovation performance.
4.2.5. Tourism enterprise selection and evaluation
Five papers were related to the evaluation of tourism enterprises.
Four papers selected partners from the perspective of enterprise cooperation. Chou et al. (Citation2012) assessed and prioritised the medical provider based on the fuzzy TOPSIS method. Liu and Gao (Citation2017) constructed a house of quality to assess suppliers in the tourism supply chain. Büyüközkan et al. (Citation2019) used the fuzzy AHP method to select the best service provider for the digital hospitality industry. He et al. (Citation2020) selected investment partners in the tourism market based on the aggregation operator-based method.
One paper evaluated the practice of CSR. Horng et al. (Citation2018) combined the fuzzy AHP and DEMATEL methods to construct an assessment model of CSR practice in the tourism industry.
4.2.6. Location selection from the perspective of land distribution
Three papers were associated with location selection from the perspective of land distribution.
Two papers selected the location in ecotourism. Ronizi et al. (Citation2020) selected the best location for ecotourism development using the fuzzy AHP and OWA methods. Aliani et al. (Citation2016) evaluated the land capability of Taleghan County and determined suitable areas for ecotourism activity based on ANP method. Besides, Jeong et al. (Citation2016) applied the fuzzy DEMATEL method to determine the suitable location of rural housing in reservoir areas, which can help decision-makers make sustainable planning policies.
4.2.7. Risk assessment
Four papers focused on tourism risk, market risk and financial risk.
Sheng Hshiung et al. (1997) employed the fuzzy AHP method to assess the tourist risk of five most frequently visited destinations by Taiwan tour groups. Wu et al. (Citation2019) used the fuzzy VIKOR method to assess the financial risk of rural tourism projects. Hosseini et al. (Citation2021) combined the fuzzy DEMATEL and VIKOR methods to evaluate the tourism risk of urban heritage. Zhu et al. (Citation2021) assessed the market risk of new-build river cruise ships based on the fuzzy AHP and PROMETHEE methods.
4.2.8. Satisfaction evaluation
Two papers discussed the satisfaction of tourists.
Carlos Martin et al. (2019) evaluated the satisfaction of tourists with tourism destinations with the fuzzy TOPSIS method to develop better strategies for marketing organisations. Martin et al. (Citation2021) applied the fuzzy TOPSIS method to evaluate visitors’ satisfaction with different access transport modes.
4.2.9. Other problems
Six papers talked about other problems in hospitality and tourism industries.
Chang (Citation2015) combined the fuzzy ANP and TOPSIS methods to select the optimal public relations personnel for the tourism industry. Skondras et al. (Citation2018) employed the fuzzy TOPSIS methods to select the most suitable heritage route for the drone. Liao et al. (Citation2019) selected the optimal online travel agency based on the fuzzy DEMATEL and ANP method. Ling (Citation2019) used an aggregation operator-based method to assess government’s administrative power and investigate the service quality of tourism agencies. Çalık (Citation2020) combined the fuzzy BWM and VIKOR methods to select optimal social media platforms for Turkish travel agencies. Krishnan et al. (Citation2021) used the fuzzy VIKOR method to rank the benchmark applications of smart e-tourism data management.
4.3. Observations
The frequency distribution of publications regarding the application areas of fuzzy MCDM methods in hospitality and tourism industries is shown in . Tourism evaluation, hotel evaluation and selection, and tourism destination evaluation and selection are the three most studied directions. From the perspective of tourism types, nine papers involved ecotourism, seven papers were about medical tourism, five papers were related to low-carbon tourism, four papers were associated with rural tourism, three papers dealt with international tourism and two papers were aimed at heritage tourism. As can be seen from , ecological tourism, medical tourism, and low-carbon tourism are more attractive research contents at present, representing the most advanced directions, which is in line with the current purpose of putting the protection of the ecological environment in first place.
5. Discussion
This article summarised 85 publications of fuzzy MCDM models applied in hospitality and tourism industries published from 1997 to 2022. First, a bibliometric analysis was conducted to analyse publication and citation trends, the regions and institutions with the most publications, the categories of reviewed papers, the highly CPs and keywords. In terms of publication and citation trends, the quantity of publications showed an overall upward trend, while the citations of each CP presented a gradual downward trend despite small fluctuations. As for the region and institution distributions of reviewed papers, Asia made a major contribution to this research field. From the categories of the reviewed literature, business & economics, environmental sciences & ecology and computer science are the most frequent categories. Among the top 10 most highly cited publications, 4 papers were from China (Mainland), which indicates the strong influence of Chinese scholars and the leading position in this research field. From the keyword co-occurrence, ‘model’, ‘decision-making’, ‘tourism’ and ‘management’ appeared more frequently. The fuzzy MCDM models applied in hospitality and tourism industries can be divided into individual fuzzy MCDM models and hybrid fuzzy MCDM models. In individual fuzzy MCDM models, AHP and TOPSIS methods were most widely used. In hybrid fuzzy MCDM models, AHP, ANP and TOPSIS were the most three methods combined with other methods. Regarding specific applications in hospitality and tourism industries, tourism evaluation, hotel evaluation and selection, and tourism destination evaluation and selection were the most studied research direction.
Through the bibliometric analysis and the review of methods and applications of fuzzy MCDM models in hospitality and tourism industries, the following research findings can be found. By analysing the growth trend of publications, it is reasonable to think that the number of publications will continue to grow in the future. The publications in recent years are less cited relatively, indicating that the dissemination rate and acceptance of papers are not enough, and researchers need to improve the quality of papers in future research. From the analysis of the regions with the largest publications, we found that China has the most publications among all countries, and Asia is the region with the largest publications, which shows that Asia is at a leading position in this research field. Scholars from other countries can cooperate with researchers from Asia to get academic exchanges and progress. According to the analysis of institutions with the most publications, it is found that the number of papers issued by each institution is relatively average, and each institution should keep up with research hotspots to form unique advantages. Through the table of research categories involved in the research field, business & economics, environmental sciences & ecology and computer science are the most frequent categories. According to the table of highly CPs of reviewed literature, Yu et al. (Citation2017, Citation2018) contributed two highly CPs. We can grasp useful knowledge by paying attention to these authoritative scholars in the field. Tourism management journals are supposed to receive more attention due to their frequency. From the figure of keywords co-occurrence, we can observe that TOPSIS is the most used method of MCDM method applied in hospitality and tourism industries owing to its advantage of simple calculation. Service quality is a common application in the industry by means of fuzzy MCDM methods. Selection problems are more discussed in decision-making. Ecotourism and medical tourism should be paid more attention in the future. As for the fuzzy MCDM methods applied in hospitality and tourism industries, it is found that AHP and TOPSIS methods are the most widely used MCDM methods. The application domains of tourism evaluation, ecological tourism, medical tourism and low-carbon tourism are more attractive research content at present. This finding facilitates researchers in pursuing research hotspots.
Based on the bibliometric analysis and content analysis in terms of fuzzy MCDM methods applied in hospitality and tourism industries, future research directions are proposed from the following perspectives.
5.1. From the perspective of fuzzy MCDM methods applied in hospitality and tourism industries
Four future research directions are proposed as follows.
In the reviewed literature, many papers used TFN (Benítez et al., Citation2007; Chou et al., Citation2012; Saayman et al., Citation2016; Martín & Román, Citation2017; Carlos Martin et al., 2019; Martín et al., Citation2020; Hameed et al., Citation2022), IVIFSs (Wu et al., Citation2019; Mishra et al., Citation2022; Kilic et al., Citation2021) and IFSs (Stanujkic et al., Citation2015; Pahari et al., Citation2018; Zhu et al., Citation2021) to express fuzzy information. Language is the most intuitive way of expression, which can depict complex evaluation information and truly reflect the objective evaluation of decision-makers. Few literatures use intuitive language to describe vague information. Some effective fuzzy language expression tools, such as probability language set (Pang et al., Citation2016) and continuous interval value language term set (Liao et al., Citation2018), have been proposed and widely used. In the existing literature, these information expression tools have not been used to solve decision-making problems in hospitality and tourism industries. Therefore, in future research, these tools can be used to express evaluation information related to the tourism and hotel industry.
AHP is the most used method in hospitality and tourism industries to determine the criteria weights. Due to the complexity and uncertainty of the decision-making environment, decision-makers often provide incomplete weights information, which may lead to the variability and inaccuracy of decision-making results. Therefore, future studies could consider the problem of incomplete criteria weights.
Most studies use fuzzy MCDM methods to deal with problems in hospitality and tourism industries without considering the psychology of decision-makers. In fact, decision-makers are not completely rational and will be affected by their psychological behaviour. Therefore, considering the psychological behaviour of decision-makers is conducive to making reasonable decision-making. In future research, prospect theory and regret theory can be considered in the fuzzy MCDM methods applied in hospitality and tourism industries.
We found that the fuzzy MCDM methods reviewed in this paper are mostly static. But due to the impact of COVID-19 on the hospitality and tourism industries (Estiri et al., Citation2022), a static approach to decision-making cannot adapt to a dynamic and unstable environment. Therefore, the decision-making problem in hospitality and tourism industries should be viewed with a developmental and dynamic perspective. Dynamic decision-making should not only consider past data, but also consider the future as a process of thinking and planning. In future research, the data-driven dynamic MCDM methods applied in hospitality and tourism industries are a topic worthy of research.
5.2. From the perspective of the hospitality and tourism industries
Three research directions are proposed to support the development of the industry.
With the development of social platforms, online reviews play an important role in influencing consumers’ selection of destination country and have a considerable impact on travel intention and destination attractiveness. Therefore, evaluating consumer satisfaction from online comments is conducive to mining consumer demands and guiding the better development of the hospitality and tourism industries. In future research, exploring tourists’ preferences by analysing online reviews is worth studying within the context of the hospitality and tourism industries.
The hospitality and tourism industries involve multiple stakeholders. For example, medical tourism includes medical tourists, healthcare providers, government agencies, facilitators, accreditation and credentialing bodies, healthcare marketers, insurance providers and infrastructure and facility providers (Kamassi et al., Citation2020). Decision-making related to medical tourism usually requires multi-party collaboration with a consensus. In the future, large-scale group decision-making methods can be used to solve the problem of multi-party cooperation problem.
Most papers related to business analysis in hospitality and tourism industries only stay on descriptive analysis and predictive analysis. Prescriptive analytics is often considered as the next step towards increasing data analytics maturity and leading to optimised decision-making ahead of time for business performance improvement. In future research of the hospitality and tourism industries, the prescriptive framework can be used to support the decision-making process.
5.3. From the perspective of application areas in hospitality and tourism industries by means of fuzzy MCDM methods
There are three suggestions that should be noted in future research:
Under the influence of COVID-19, stakeholders in hospitality and tourism industries should conduct risk assessments to deal with the uncertain environment, research in the next few years can focus on risk assessment.
It is particularly important to put environmental protection in the first place when advocating sustainable development nowadays (Hasheminasab et al., Citation2022). Therefore, more attention should be paid to ecotourism, low-carbon tourism, and sustainable tourism in future research.
Many studies are associated with hotel evaluation and selection and tourism destination evaluation and selection. But there are few studies dealing with strategic assessment and tourist satisfaction evaluation, which are also issues that tourists and tourism agencies most concerned about. Therefore, future studies can be carried out more on these aspects.
6. Conclusion
When faced with decision-making problems in hospitality and tourism industries, fuzzy MCDM methods can characterise the complex evaluation information given by decision-makers, and provide decision recommendations (ranking, selection or sorting) for a set of alternatives measured by a set of criteria. This paper investigated the literature on fuzzy MCDM methods applied in hospitality and tourism industries, analysed the result of bibliometric analysis, fuzzy MCDM methods and application domains in hospitality and tourism industries, identified the existing research gaps and outlined directions for future research. Our review has shown that fuzzy MCDM methods are useful approaches to tackle decision-making problems in hospitality and tourism industries, which helps tourism institutions, hotel managers and tourists make reasonable decisions.
The following limitations exist in our study. Since the paper only retrieved the Core Collection database in Web of Science, some impactful publications in other data sets were ignored. The scope of the study can be expanded by including databases such as Scopus, Springer and Science Direct. Besides, there are shortages in the retrieval strategy. Some fuzzy MCDM methods applied to the hospitality and tourism industries may not be included in the collected records. Future research will be devoted to developing more accurate retrieval strategies. Moreover, this article summarised each paper briefly and the specific details were not discussed, which need to be further studied.
Disclosure statement
No potential conflict of interest was reported by the author(s).
Additional information
Funding
References
- Abrishamchi, A., Moonesan, A. A., & Maknoon, R. (2019). A fuzzy multi-criteria approach for sustainable island-based tourism development. Scientia Iranica, 28(2), 682-699. https://doi.org/10.24200/sci.2019.53260.3146
- Aliani, H., BabaieKafaky, S., Saffari, A., & Monavari, S. M. (2017). Land evaluation for ecotourism development—an integrated approach based on FUZZY, WLC, and ANP methods. International Journal of Environmental Science and Technology, 14(9), 1999–2008. https://doi.org/10.1007/s13762-017-1291-5
- Aliani, H., Kafaky, S. B., Saffari, A., & Monavari, S. M. (2016). Determining an appropriate method for the purpose of land allocation for ecotourism development (case study: Taleghan County, Iran). Environmental Monitoring and Assessment, 188(11), 651. https://doi.org/10.1007/s10661-016-5651-2.
- Andria, J., di Tollo, G., & Pesenti, R. (2021). Fuzzy multi-criteria decision-making: An entropy-based approach to assess tourism sustainability. Tourism Economics, 27(1), 168–186. https://doi.org/10.1177/1354816619885207
- Arsić, S., Nikolić, D., & Živković, Ž. (2017). Hybrid SWOT - ANP - FANP model for prioritization strategies of sustainable development of ecotourism in National Park Djerdap, Serbia. Forest Policy and Economics, 80, 11–26. https://doi.org/10.1016/j.forpol.2017.02.003
- Atanassov, K. T. (1986). Intuitionistic fuzzy sets. Fuzzy Sets and Systems, 20(1), 87–96. https://doi.org/10.1016/S0165-0114(86)80034-3
- Atanassov, K. T. (1999). Interval valued intuitionistic fuzzy sets. Intuitionistic fuzzy sets, physica, Heidelberg (pp. 139–177). https://doi.org/10.1007/978-3-7908-1870-3_2
- Baydaş, M., Elma, O. E., & Pamučar, D. (2022). Exploring the specific capacity of different multi criteria decision making approaches under uncertainty using data from financial markets. Expert Systems with Applications, 197, 116755. https://doi.org/10.1016/j.eswa.2022.116755
- Benítez, J. M., Martín, J. C., & Román, C. (2007). Using fuzzy number for measuring quality of service in the hotel industry. Tourism Management, 28(2), 544–555. https://doi.org/10.1016/j.tourman.2006.04.018
- Bueno, I., Carrasco, R. A., Porcel, C., Kou, G., & Herrera-Viedma, E. (2021). A linguistic multi-criteria decision making methodology for the evaluation of tourist services considering customer opinion vayanglue. Applied Soft Computing, 101, 107045. https://doi.org/10.1016/j.asoc.2020.107045
- Büyüközkan, G., Feyzioğlu, O., & Havle, C. A. (2019). Intuitionistic fuzzy AHP based strategic analysis of service quality in digital hospitality industry. IFAC-PapersOnLine, 52(13), 1687–1692. https://doi.org/10.1016/j.ifacol.2019.11.443
- Çalık, A. (2020). Evaluation of social media platforms using best-worst method and fuzzy VIKOR methods: A case study of travel agency. Iranian Journal of Management Studies, 13(4), 645–672. https://doi.org/10.22059/ijms.2020.294545.673893
- Carlos Martin, J., Saayman, M., & Du Plessis, E. (2019). Determining satisfaction of international tourist: A different approach. Journal of Hospitality and Tourism Management, 40, 1–10. https://doi.org/10.1016/j.jhtm.2019.04.005
- Chang, K. L. (2015). The use of a hybrid MCDM model for public relations personnel selection. Informatica, 26(3), 389–406. https://doi.org/10.15388/Informatica.2015.54
- Chen, C. L., & Bau, Y. P. (2016). Establishing a multi-criteria evaluation structure for tourist beaches in Taiwan: A foundation for sustainable beach tourism. Ocean & Coastal Management, 121, 88–96. https://doi.org/10.1016/j.ocecoaman.2015.12.013
- Chen, N., Xu, Z., & Xia, M. (2013). Interval-valued hesitant preference relations and their applications to group decision making. Knowledge-Based Systems, 37, 528–540. https://doi.org/10.1016/j.knosys.2012.09.009
- Chen, S. X., Xu, Z. S., Wang, X. X., & Škare, M. (2022). A bibliometric analysis of natural disasters and business management in tourism. Journal of Business Economics and Management, 23(2), 305–326. https://doi.org/10.3846/jbem.2022.16388
- Chen, Y. C., Yu, T. H., Tsui, P. L., & Lee, C. S. (2014). A fuzzy AHP approach to construct international hotel spa atmosphere evaluation model. Quality & Quantity, 48(2), 645–657. https://doi.org/10.1007/s11135-012-9792-2
- Chou, S. F., Horng, J. S., Liu, C. H., & Lin, J. Y. (2021). Innovation strategy development and facilitation of an integrative process with an MCDM framework. Entrepreneurship Research Journal, 000010151520200001. https://doi.org/10.1515/erj-2020-0001
- Chou, S. Y., Vincent, F. Y., Dewabharata, A., & Dat, L. Q. (2012). A fuzzy TOPSIS approach for medical provider selection and evaluation [Paper presentation]. November In 2012 International conference on Fuzzy Theory and Its Applications (iFUZZY2012) (pp. 322–326). IEEE. https://doi.org/10.1109/iFUZZY.2012.6409724
- Chou, T. Y., Hsu, C. L., & Chen, M. C. (2008). A fuzzy multi-criteria decision model for international tourist hotels location selection. International Journal of Hospitality Management, 27(2), 293–301. https://doi.org/10.1016/j.ijhm.2007.07.029
- Chiao, K. P. (2021). Multi-criteria decision making with interval type 2 fuzzy Bonferroni mean. Expert Systems with Applications, 176, 114789. https://doi.org/10.1016/j.eswa.2021.114789
- Duan, G., Papi, M., & Kosti, M. (2021). Multi-criteria decision making trends in ecotourism and sustainable tourism. Ekonomika Poljoprivrede, 68(2), 321–340. https://doi.org/10.5937/ekoPolj2102321G
- Erdenejargal, N., Dorjsuren, B., Choijinjav, L., Doljin, D., Enkhbold, A., Munkhuu, B., Natsagdorj, B., & Girma, A. (2021). Evaluation of the natural landscape aesthetic: A case study of Uvs province. Polish Journal of Environmental Studies, 30(5), 4497–4509. https://doi.org/10.15244/pjoes/132788
- Erfani, M., Afrougheh, S., Ardakani, T., & Sadeghi, A. (2015). Tourism positioning using decision support system (case study: Chahnime—Zabol, Iran). Environmental Earth Sciences, 74(4), 3135–3144. https://doi.org/10.1007/s12665-015-4365-z
- Estiri, M., Heidary Dahooie, J., & Skare, M. (2022). COVID-19 crisis and resilience of tourism SME's: A focus on policy responses. Economic Research-Ekonomska Istraživanja, 35(1), 5556–5580. https://doi.org/10.1080/1331677X.2022.2032245
- Forouzandeh, S., Rostami, M., & Berahmand, K. (2022). A hybrid method for recommendation systems based on tourism with an evolutionary algorithm and topsis model. Fuzzy Information and Engineering, 14(1), 26–50. https://doi.org/10.1080/16168658.2021.2019430
- Fu, H. H., & Tzeng, S. Y. (2016). Applying fuzzy multiple criteria decision making approach to establish safety-management system for hot spring hotels. Asia Pacific Journal of Tourism Research, 21(12), 1343–1356. https://doi.org/10.1080/10941665.2016.1175487
- Fu, Y. K., Huang, W., & Liao, C. N. (2020). The selection model for horizontal alliances between hotels and airlines: An integrated application of NGT, fuzzy TOPSIS and MCGP methods. Tourism Review, 75(4), 681–698. https://doi.org/10.1108/TR-06-2019-0214
- García Mestanza, J., & Bakhat, R. (2021). A fuzzy AHP-MAIRCA model for overtourism assessment: The case of Malaga province. Sustainability, 13(11), 6394. https://doi.org/10.3390/su13116394
- Ghasemi, P., Mehdiabadi, A., Spulbar, C., & Birau, R. (2021). Ranking of sustainable medical tourism destinations in Iran: An integrated approach using fuzzy SWARA-PROMETHEE. Sustainability, 13(2), 683. https://doi.org/10.3390/su13020683
- Gigović, L., Pamučar, D., Lukić, D., & Marković, S. (2016). GIS-Fuzzy DEMATEL MCDA model for the evaluation of the sites for ecotourism development: A case study of “Dunavski ključ” region, Serbia. Land Use Policy, 58, 348–365. https://doi.org/10.1016/j.landusepol.2016.07.030
- Gou, X. J., Xu, Z. S., Liao, H. C., & Herrera, F. (2021). Probabilistic double hierarchy linguistic term set and its use in designing an improved VIKOR method: The application in smart healthcare. Journal of the Operational Research Society, 72(12), 2611–2630. https://doi.org/10.1080/01605682.2020.1806741
- Guru, S., Sinha, A., & Kautish, P. (2022). Determinants of medical tourism: Application of fuzzy analytical hierarchical process. International Journal of Emerging Markets, 17. https://doi.org/10.1108/IJOEM-08-2021-1173
- Hameed, H. B., Ali, Y., & Khan, A. U. (2022). Regional development through tourism in Balochistan under the China-Pakistan economic corridor. Journal of China Tourism Research, 18(1), 1–19. https://doi.org/10.1080/19388160.2020.1787910
- Hasheminasab, H., Hashemkhani Zolfani, S., Kazimieras Zavadskas, E., Kharrazi, M., & Skare, M. (2022). A circular economy model for fossil fuel sustainable decisions based on MADM techniques. Economic Research-Ekonomska Istraživanja, 35(1), 564–582. https://doi.org/10.1080/1331677X.2021.1926305
- He, J., Huang, Z., Mishra, A. R., & Alrasheedi, M. (2021). Developing a new framework for conceptualizing the emerging sustainable community-based tourism using an extended interval-valued Pythagorean fuzzy SWARA-MULTIMOORA. Technological Forecasting and Social Change, 171, 120955. https://doi.org/10.1016/j.techfore.2021.120955
- He, P., Yang, Z., & Hou, B. (2020). A multi-attribute decision-making algorithm using Q-rung orthopair power Bonferroni mean operator and its application. Mathematics, 8(8), 1240. https://doi.org/10.3390/math8081240
- Ho, C. Y., Tsai, B. H., Chen, C., S., & Lu, M. T. (2021). Exploring green marketing orientations toward sustainability the hospitality industry in the COVID-19 pandemic. Sustainability, 13(8), 4348. https://doi.org/10.3390/su13084348
- Horng, J. S., Hsu, H., & Tsai, C. Y. (2018). An assessment model of corporate social responsibility practice in the tourism industry. Journal of Sustainable Tourism, 26(7), 1085–1104. https://doi.org/10.1080/09669582.2017.1388384
- Hosseini, A., Pourahmad, A., Ayashi, A., Tzeng, G. H., Banaitis, A., & Pourahmad, A. (2021). Improving the urban heritage based on a tourism risk assessment using a hybrid fuzzy MADM method: The case study of Tehran’s central district. Journal of Multi-Criteria Decision Analysis, 28(5–6), 248–268. https://doi.org/10.1002/mcda.1746
- Hu, M., Qiu, R. T. R., Wu, D. C., & Song, H. (2021). Hierarchical pattern recognition for tourism demand forecasting. Tourism Management, 84, 104263. https://doi.org/10.1016/j.tourman.2020.104263
- Hu, Y. C. (2009). Fuzzy multiple-criteria decision making in the determination of critical criteria for assessing service quality of travel websites. Expert Systems with Applications, 36(3), 6439–6445. https://doi.org/10.1016/j.eswa.2008.07.046
- Huang, A., & Baker, M. (2021). Exploring skill-based career transitions for entry-level hospitality and tourism workers. Journal of Hospitality and Tourism Management, 48, 368–373. https://doi.org/10.1016/j.jhtm.2021.07.007
- Huang, C. H., Kao, H. Y., & Li, H. L. (2007). Decision on enterprise computing solutions for an international tourism. International Journal of Information Technology & Decision Making, 06(04), 687–700. https://doi.org/10.1142/S0219622007002666
- Huang, J. H., & Peng, K. H. (2012). Fuzzy Rasch model in TOPSIS: A new approach for generating fuzzy numbers to assess the competitiveness of the tourism industries in Asian countries. Tourism Management, 33(2), 456–465. https://doi.org/10.1016/j.tourman.2011.05.006
- Jeong, J. S., García-Moruno, L., Hernández-Blanco, J., & Sánchez-Ríos, A. (2016). Planning of rural housings in reservoir areas under (mass) tourism based on a fuzzy DEMATEL-GIS/MCDA hybrid and participatory method for Alange, Spain. Habitat International, 57, 143–153. https://doi.org/10.1016/j.habitatint.2016.07.008
- Jusoh, A., Mardani, A., Omar, R., Štreimikienė, D., Khalifah, Z., & Sharifara, A. (2018). Application of MCDM approach to evaluate the critical success factors of total quality management in the hospitality industry. Journal of Business Economics and Management, 19(2), 399–416. https://doi.org/10.3846/jbem.2018.5538
- Kamassi, A., Abd Manaf, N. H., & Omar, A. (2020). The identity and role of stakeholders in the medical tourism industry: State of the art. Tourism Review, 75(3), 559–574. https://doi.org/10.1108/TR-01-2019-0031
- Kaushal, V., & Srivastava, S. (2021). Hospitality and tourism industry amid COVID-19 pandemic: Perspectives on challenges and learnings from India. International Journal of Hospitality Management, 92, 102707. https://doi.org/10.1016/j.ijhm.2020.102707
- Kaymaz, Ç. K., Çakır, Ç., Birinci, S., & Kızılkan, Y. (2021). GIS-Fuzzy DEMATEL MCDA model in the evaluation of the areas for ecotourism development: A case study of “Uzundere”, Erzurum-Turkey. Applied Geography, 136, 102577. https://doi.org/10.1016/j.apgeog.2021.102577
- Kilic, H. S., Kalender, Z. T., Yalcin, A. S., Erkal, G., & Tuzkaya, G. (2021). Information system selection for hospitality industry via integrated use of IVIF-DEMATEL and IVIF-TOPSIS. Journal of Intelligent & Fuzzy Systems, 42(1), 317–335. https://doi.org/10.3233/JIFS-219194
- Krishnan, E., Mohammed, R., Alnoor, A., Albahri, O. S., Zaidan, A. A., Alsattar, H., Albahri, A. S., Zaidan, B. B., Kou, G., Hamid, R. A., Alamoodi, A. H., & Alazab, M. (2021). Interval type 2 trapezoidal-fuzzy weighted with zero inconsistency combined with VIKOR for evaluating smart e‐tourism applications. International Journal of Intelligent Systems, 36(9), 4723–4774. https://doi.org/10.1002/int.22489
- Lian, T., Yu, C., Wang, Z., & Hou, Z. (2017). The evaluation study on tourism websites: From the perspective of triangular intuitionistic fuzzy multiple attribute group decision making. Journal of Applied Statistics, 44(16), 2877–2889. https://doi.org/10.1080/02664763.2016.1266466
- Liao, H. C., Wu, X. L., Liang, X. D., Yang, J. B., Xu, D. L., & Herrera, F. (2018). A continuous interval-valued linguistic ORESTE method for multi-criteria group decision making. Knowledge-Based Systems, 153, 65–77. https://doi.org/10.1016/j.knosys.2018.04.022
- Liao, S. K., Hsu, H. Y., & Chang, K. L. (2019). OTAs selection for hot spring hotels by a hybrid MCDM model. Mathematical Problems in Engineering, 2019, 1–9. https://doi.org/10.1155/2019/4251362
- Lin, C. T., Lee, C., & Wu, C. S. (2010). Fuzzy group decision making in pursuit of a competitive marketing strategy. International Journal of Information Technology & Decision Making, 09(02), 281–300. https://doi.org/10.1142/S0219622010003828
- Lin, H., & Wang, Z. J. (2017). Linguistic multi-attribute group decision making with risk preferences and its use in low-carbon tourism destination selection. International Journal of Environmental Research and Public Health, 14(9), 1078. https://doi.org/10.3390/ijerph14091078
- Ling, C R. (2019). Methods for evaluating the government administrative power under the whole area tourism with hesitant fuzzy linguistic information. Journal of Intelligent & Fuzzy Systems, 37(2), 2019–2025. https://doi.org/10.3233/JIFS-179264
- Litvin, S. W., Goldsmith, R. E., & Pan, B. (2018). A retrospective view of electronic word-of-mouth in hospitality and tourism management. International Journal of Contemporary Hospitality Management, 30(1), 313–325. https://doi.org/10.1108/IJCHM-08-2016-0461
- Liu, M., & Gao, Q. (2017). Supplier evaluation in TSC based on fuzzy linguistic term sets and QFD [Paper presentation].2017 29th Chinese Control and Decision Conference (CCDC), (pp. 4671–4675). IEEE. https://doi.org/10.1109/CCDC.2017.7979322
- Liu, M., & Wang, S. (2017). Method for evaluating the eco-tourism environmental carrying capacity with hesitant fuzzy linguistic information. Journal of Intelligent & Fuzzy Systems, 33(3), 1733–1740. https://doi.org/10.3233/JIFS-161057
- Liu, Y., & Suk, S. (2021). Coupling and coordinating relationship between tourism economy and ecological environment—a case study of Nagasaki Prefecture, Japan. International Journal of Environmental Research and Public Health, 18(23), 12818. https://doi.org/10.3390/ijerph182312818
- Lu, K. Y., Liao, H. C., & Zavadskas, E. K. (2021). An overview of fuzzy techniques in supply chain management: Bibliometrics, methodologies, applications and future directions. Technological and Economic Development of Economy, 27(2), 402–458. https://doi.org/10.3846/tede.2021.14433
- Mardani, A., Jusoh, A., Zavadskas, E. K., Kazemilari, M., & Khalifah, Z. (2016). Application of multiple-criteria decision making techniques in tourism and hospitality industry: A systematic review. Transformations in Business & Economics, 15(1), 192–213. https://doi.org/10.3846/16111699.2015.1095233
- Mardani, A., Zavadskas, E. K., Streimikiene, D., Jusoh, A., Nor, K. M. D., & Khoshnoudi, M. (2016). Using fuzzy multiple criteria decision making approaches for evaluating energy saving technologies and solutions in five star hotels: A new hierarchical framework. Energy, 117, 131–148. https://doi.org/10.1016/j.energy.2016.10.076
- Martín, J. C., Orden Cruz, C., & Zergane, S. (2020). Islamic finance and halal tourism: An unexplored bridge for smart specialization. Sustainability, 12(14), 5736. https://doi.org/10.3390/su12145736
- Martín, J. C., & Román, C. (2017). Measuring service quality in the hotel industry: The value of user generated content. Tourism: An International Interdisciplinary Journal, 65(4), 390–405.https://hrcak.srce.hr/191470
- Martin, J. C., Román, C., Moreira, P., Moreno, R., & Oyarce, F. (2021). Does the access transport mode affect visitors’ satisfaction in a World Heritage City? The case of Valparaiso, Chile. Journal of Transport Geography, 91, 102969. https://doi.org/10.1016/j.jtrangeo.2021.102969
- MerdİVencİ, F., & KarakaŞ, H. (2020). Analysis of factors affecting health tourism performance using fuzzy dematel method. Advances in Hospitality and Tourism Research, 8, 371–392. https://doi.org/10.30519/ahtr.734339
- Merigó, J. M., Gil-Lafuente, A. M., & Yager, R. R. (2015). An overview of fuzzy research with bibliometric indicators. Applied Soft Computing, 27, 420–433. https://doi.org/10.1016/j.asoc.2014.10.035
- Mi, X., Li, J., Liao, H., Zavadskas, E. K., Al-Barakati, A., Barnawi, A., Taylan, O., & Herrera-Viedma, E. (2019). Hospitality brand management by a score-based q-rung orthopair fuzzy V.I.K.O.R. method integrated with the best worst method. Economic Research-Ekonomska Istraživanja, 32(1), 3272–3301. https://doi.org/10.1080/1331677X.2019.1658533
- Mijajlović, M., Puška, A., Stević, Ž., Marinković, D., Doljanica, D., Jovanović, S. V., Stojanović, I., & Beširović, J. (2020). Determining the competitiveness of spa-centers in order to achieve sustainability using a fuzzy multi-criteria decision-making model. Sustainability, 12(20), 8584. https://doi.org/10.3390/su12208584
- Mishra, A. R., Chandel, A., & Saeidi, P. (2022). Low-carbon tourism strategy evaluation and selection using interval-valued intuitionistic fuzzy additive ratio assessment approach based on similarity measures. Environment, Development and Sustainability, 24(5), 7236–7282. https://doi.org/10.1007/s10668-021-01746-w
- Nilashi, M., Samad, S., Manaf, A. A., Ahmadi, H., Rashid, T. A., Munshi, A., Almukadi, W., Ibrahim, O., & Hassan Ahmed, O. (2019). Factors influencing medical tourism adoption in Malaysia: A DEMATEL-Fuzzy TOPSIS approach. Computers & Industrial Engineering, 137, 106005. https://doi.org/10.1016/j.cie.2019.106005
- Omarzadeh, D., Pourmoradian, S., Feizizadeh, B., Khallaghi, H., Sharifi, A., & Kamran, K. V. (2022). A GIS-based multiple ecotourism sustainability assessment of West Azerbaijan province, Iran. Journal of Environmental Planning and Management, 65(3), 490–513. https://doi.org/10.1080/09640568.2021.1887827
- Ostovare, M., & Shahraki, M. R. (2019). Evaluation of hotel websites using the multicriteria analysis of PROMETHEE and GAIA: Evidence from the five-star hotels of Mashhad. Tourism Management Perspectives, 30, 107–116. https://doi.org/10.1016/j.tmp.2019.02.013
- Ozturkoglu, Y., Sari, F. O., & Saygili, E. (2021). A new holistic conceptual framework for sustainability oriented hospitality innovation with triple bottom line perspective. Journal of Hospitality and Tourism Technology, 12(1), 39–57. https://doi.org/10.1108/JHTT-02-2019-0022
- Pahari, S., Ghosh, D., & Pal, A. (2018). An online review-based hotel selection process using intuitionistic fuzzy TOPSIS method. Progress in computing, analytics and networking (pp. 203–214). Springer. https://doi.org/10.1007/978-981-10-7871-2_20
- Pang, Q., Wang, H., & Xu, Z. (2016). Probabilistic linguistic term sets in multi-attribute group decision making. Information Sciences, 369, 128–143. https://doi.org/10.1016/j.ins.2016.06.021
- Peng, K. H., & Tzeng, G. H. (2012). Improvement tourism competitiveness using hybrid fuzzy MCDM [Paper presentation]. November In 2012 International conference on Fuzzy Theory and Its Applications (iFUZZY2012) (pp. 24–29). IEEE. https://doi.org/10.1109/iFUZZY.2012.6409670
- Peng, G. D., Tian, S., Zhang, Y., Song, R., & Wang, Z. (2019). Single-valued neutrosophic power Shapley Choquet average operators and their applications to multi-criteria decision-making. Mathematics, 7(11), 1081. https://doi.org/10.3390/math7111081
- Puška, A., Pamucar, D., Stojanović, I., Cavallaro, F., Kaklauskas, A., & Mardani, A. (2021). Examination of the sustainable rural tourism potential of the Brčko district of Bosnia and Herzegovina using a fuzzy approach based on group decision making. Sustainability, 13(2), 583. https://doi.org/10.3390/su13020583
- Qin, J. (2017). Interval type-2 fuzzy Hamy mean operators and their application in multiple criteria decision making. Granular Computing, 2(4), 249–269. https://doi.org/10.1007/s41066-017-0041-x
- Rasch, G. (1960). Probabilistic models for some intelligence and attainment tests. Danish Institute for Educational Research.
- Rashmi, R., Singh, R., Chand, M., & Avikal, S. (2019). An MCDM-based approach for selecting the best state for tourism in India. Harmony search and nature inspired optimization algorithms (pp. 17–26). Springer. https://doi.org/10.1007/978-981-13-0761-4_2
- Rodriguez, R. M., Martinez, L., & Herrera, F. (2012). Hesitant fuzzy linguistic term sets for decision making. IEEE Transactions on Fuzzy Systems, 20(1), 109–119. https://doi.org/10.1109/TFUZZ.2011.2170076
- Ronizi, S. R. A., Mokarram, M., & Negahban, S. (2020). Utilizing multi-criteria decision to determine the best location for the ecotourism in the east and central of Fars province, Iran. Land Use Policy, 99, 105095. https://doi.org/10.1016/j.landusepol.2020.105095
- Roy, J., Chatterjee, K., Bandyopadhyay, A., & Kar, S. (2018). Evaluation and selection of medical tourism sites: A rough analytic hierarchy process based multi-attributive border approximation area comparison approach. Expert Systems, 35(1), e12232. https://doi.org/10.1111/exsy.12232
- Saayman, M., Martín, J. C., & Román, C. (2016). There is no fuzziness when it comes to measuring service quality in national parks. Tourism Economics, 22(6), 1207–1224. https://doi.org/10.1177/1354816616669036
- Scheyvens, R., & Hughes, E. (2019). Can tourism help to “end poverty in all its forms everywhere”? The challenge of tourism addressing SDG1. Journal of Sustainable Tourism, 27(7), 1061–1079. https://doi.org/10.1080/09669582.2018.1551404
- Sheng Hshiung, T., Gwo Hshiung, T., & Kuo Ching, W. (1997). Evaluating tourist risks from fuzzy perspectives. Annals of Tourism Research, 24(4), 796–812. https://doi.org/10.1016/S0160-7383(97)00059-5
- Sheng, Y., Xu, M., Jin, Z., & Zhang, J. (2019). Research on evaluating brand marketing of leisure tourism city with fuzzy information. Journal of Intelligent & Fuzzy Systems, 37(2), 1679–1686. https://doi.org/10.3233/JIFS-179232
- Skondras, E., Siountri, K., Michalas, A., & Vergados, D. D. (2018). A route selection scheme for supporting virtual tours in sites with cultural interest using drones [Paper presentation].2018 9th International Conference on Information, Intelligence, Systems and Applications (IISA), (pp. 1–6). IEEE. https://doi.org/10.1109/IISA.2018.8633594
- Stanujkic, D., Zavadskas, E. K., & Tamošaitienė, J. (2015). An approach to measuring website quality in the rural tourism industry based on atanassov intuitionistic fuzzy sets. E + M Ekonomie a Management, 18(4), 184–199. https://doi.org/10.15240/tul/001/2015-4-013
- Tang, M., Liao, H., Yepes, V., Laurinavičius, A., & Tupėnaitė, L. (2021). Quantifying and mapping the evolution of a leader journal in the field of civil engineering. Journal of Civil Engineering and Management, 27(2), 100–116. https://doi.org/10.3846/jcem.2021.14365
- Tian, C., & Peng, J. J. (2019). An integrated picture fuzzy anp-todim multi-criteria decision-making approach for tourism attraction recommendation. Technological and Economic Development of Economy, 26(2), 331–354. https://doi.org/10.3846/tede.2019.11412
- Torkayesh, A. E., Malmir, B., & Asadabadi, M. R. (2021). Sustainable waste disposal technology selection: The stratified best-worst multi-criteria decision-making method. Waste Management (New York, NY), 122, 100–112. https://doi.org/10.1016/j.wasman.2020.12.040
- Torra, V. (2010). Hesitant fuzzy sets. International Journal of Intelligent Systems, 25(6), n/a–n/a. https://doi.org/10.1002/int.20418
- Vahdat, V., Salehi, S., & Ahmadi, N. (2018). November Service quality assessment via enhanced data-driven MCDM model. INFORMS international conference on service science (pp. 217–230). Springer. https://doi.org/10.1007/978-3-030-04726-9_22
- Van Eck, N., & Waltman, L. (2010). Software survey: VOSviewer, a computer program for bibliometric mapping. Scientometrics, 84(2), 523–538. https://doi.org/10.1007/s11192-009-0146-3
- Vatankhah, S., Bouzari, M., & Safavi, H. P. (2021). Unraveling the fuzzy predictors of stress at work. International Journal of Organizational Analysis, 29(2), 277–300. https://doi.org/10.1108/IJOA-01-2020-2005
- Wang, X. k., Wang, S. h., Zhang, H. y., Wang, J. q., & Li, L. (2021). The recommendation method for hotel selection under traveller preference characteristics: A cloud-based multi-criteria group decision support model. Group Decision and Negotiation, 30(6), 1433–1469. https://doi.org/10.1007/s10726-021-09735-0
- Wei, G., Tang, Y., Zhao, M., Lin, R., & Wu, J. (2020). Selecting the low-carbon tourism destination: Based on Pythagorean fuzzy taxonomy method. Mathematics, 8(5), 832. https://doi.org/10.3390/math8050832
- Wu, L., Gao, H., Wei, C., & Zhang, J. (2019). VIKOR method for financing risk assessment of rural tourism projects under interval-valued intuitionistic fuzzy environment. Journal of Intelligent & Fuzzy Systems, 37(2), 2001–2008. https://doi.org/10.3233/JIFS-179262
- Wu, L., Wei, G., Gao, H., & Wei, Y. (2018). Some interval-valued intuitionistic fuzzy dombi hamy mean operators and their application for evaluating the elderly tourism service quality in tourism destination. Mathematics, 6(12), 294. https://doi.org/10.3390/math6120294
- Wu, L., Wei, G., Wu, J., & Wei, C. (2020). Some interval-valued intuitionistic fuzzy dombi heronian mean operators and their application for evaluating the ecological value of forest ecological tourism demonstration areas. International Journal of Environmental Research and Public Health, 17(3), 829. https://doi.org/10.3390/ijerph17030829
- Wut, T. M., Xu, J. B., & Wong, S. M. (2021). Crisis management research (1985–2020) in the hospitality and tourism industry: A review and research agenda. Tourism Management, 85, 104307. https://doi.org/10.1016/j.tourman.2021.104307
- Yang, Y., Hu, J., Sun, R., & Chen, X. (2017). Medical tourism destinations prioritization using group decision making method with neutrosophic fuzzy preference relations. Scientia Iranica, 25(6), 3744–3764. https://doi.org/10.24200/sci.2017.4514
- Yang, X., & Wang, Z. J. (2020). Intuitionistic fuzzy hierarchical multi-criteria decision making for evaluating performances of low-carbon tourism scenic spots. International Journal of Environmental Research and Public Health, 17(17), 6259. https://doi.org/10.3390/ijerph17176259
- Yu, S. M., Wang, J., & Wang, J. Q. (2017). An interval type-2 fuzzy likelihood-based MABAC approach and its application in selecting hotels on a tourism website. International Journal of Fuzzy Systems, 19(1), 47–61. https://doi.org/10.1007/s40815-016-0217-6
- Yu, S. M., Wang, J., & Wang, J. Q. (2018). An extended TODIM approach with intuitionistic linguistic numbers. International Transactions in Operational Research, 25(3), 781–805. https://doi.org/10.1111/itor.12363
- Zadeh, L. A. (1965). Fuzzy sets. Information and Control, 8(3), 338–353. https://doi.org/10.1016/S0019-9958(65)90241-X
- Zadeh, L. A. (1975). The concept of a linguistic variable and its application to approximate reasoning—I. Information Sciences, 8(3), 199–249. https://doi.org/10.1016/0020-0255(75)90036-5
- Zhang, C., Liao, H., Luo, L., & Xu, Z. (2022). Low-carbon tourism destination selection by a thermodynamic feature-based method. Journal of the Operational Research Society, 73(8), 1692–1707. https://doi.org/10.1080/01605682.2021.1908862
- Zhu, J., Wang, H., & Xu, B. (2021). Using fuzzy AHP-PROMETHEE for market risk assessment of new-build river cruises on the Yangtze river. Sustainability, 13(22), 12932. https://doi.org/10.3390/su132212932
- Zolfani, S. H., Sedaghat, M., Maknoon, R., & Zavadskas, E. K. (2015). Sustainable tourism: A comprehensive literature review on frameworks and applications. Economic Research-Ekonomska Istraživanja, 28(1), 1–30. https://doi.org/10.1080/1331677X.2014.995895