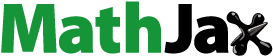
Abstract
In the context of carbon peaking and neutrality, it would be interesting to study the policy conditions for achieving carbon targets. From a technical perspective, this study constructed a theoretical model of the relationship between energy tax policy, R&D subsidy policy, and energy intensity within the framework of biassed technical change. Subsequently, through the logical relationship between energy and carbon intensities, three combination policy schemes for achieving the carbon intensity target were obtained. The findings are as follows: First, a higher rate of energy tax and R&D subsidy is destined to lead to a lower energy intensity, and energy tax policy can better boost energy intensity than R&D subsidy policy. Second, in terms of pulling the carbon intensity, the effect of the energy tax policy is better than that of the R&D subsidy policy, but in terms of its impact on the future policy effect, the R&D subsidy policy is better than the energy tax policy. Third, China should adopt a combination of policies to reduce carbon intensity, with a certain increase in the energy tax policy and a certain increase in the R&D subsidy rate policy.
JEL CODES:
1. Introduction
China has issued a series of polices titled ‘1 + N’ to achieve carbon peak and carbon neutrality. The goal of reducing carbon dioxide emissions per unit of GDP by 18% by 2025 than that of 2020 is introduced in the ‘Carbon Peak 2030 Action Plan’, which will lay a solid foundation for achieving carbon peak. Carbon dioxide emissions per unit of GDP will decrease by more than 65% compared with that of 2005, and the carbon peak will be achieved smoothly by 2030.
Experts and scholars in various institutions at home and abroad have conducted a large amount of research on finding ways to reduce carbon intensity in China, and their views are basically about ‘reducing energy consumption and vigorously developing non-fossil energy’ (Hu, Citation2021; Zhang et al., Citation2022; Zhao, Citation2022). However, here the question is whether this ‘consensus’ is suitable for China’s domestic condition: First, China’s high-quality economic development in the future needs to be supported by energy (Lin, Citation2021); Second, coal is China’s dominant and basic energy, thus blind ‘de-coal’ will lead to a hard landing of the energy structure adjustment (Lin, Citation2018). Therefore, can carbon intensity targets be met without disrupting the economy or limiting energy consumption, including fossil fuels? The biased technical change theory provides a possible plan.
The theory of biased technical change indicates that there is an invisible technological force in the market that leads to the transformation of the proportion of demand when the supply is being changed. We can optimise the energy structure and reduce carbon intensity by adjusting the supply of the energy system in a mild manner, while trying to leave the economy alone. However, the invisible force market needs an intervention by the visible hand of the government, because technological progress tends to favour relatively affluent factors. Without the government’s intervention, the market will allocate resources to innovate the fossil energy sector because of the scale effect, and a laissez-faire market will eventually cause a disaster to the environment (Acemoglu et al., Citation2012). High-carbon fossil fuels are the primary energy sources in China. As market-oriented energy reform is carried out and the government lifts most regulations, China’s relatively rich fossil energy will win the favour of technological progress. Therefore, the demand for fossil fuels will continue to increase amid biased technical changes, which will hinder the realization of carbon intensity targets. The theory of biased technical change guides policy formulation and indicates that the direction of technological progress can be manipulated. By taking advantage of this theory, governments can formulate targeted policies to encourage enterprises to create low-carbon technologies to reduce carbon emissions.
Therefore, how policies affect the direction of energy technology to reduce carbon emissions, and how much energy tax rate and R&D subsidy rate can help China achieve carbon intensity targets in 2025 and 2030 are the research topics of this paper. We discuss carbon targets from a technical perspective under the framework of biased technical change to provide the academic community with a theoretical model of carbon intensity from the perspective of energy technology. Therefore, for policy research in the fields of energy and environment, we will no longer be limited to qualitative analysis, and quantitative measurement will open a new perspective for this field of research.
The remainder of this paper is organised as follows. The next section reviews the research status at home and abroad in the field of carbon. In Section 3, we construct a theoretical model of the relationship among energy tax policy, R&D subsidy policy, and energy intensity. Section 4 deduces the logical relationship between energy intensity and carbon intensity. In Section 5, we conclude with policy conditions for achieving the carbon intensity target based on Sections 3 and Section 4. The final section provides the conclusions and limitations of this study.
2. Review of domestic and foreign research
Since the ‘United Nations Framework Convention on Climate Change’ was signed in 1992, domestic and foreign academic circles have focused on carbon issues and have formed three main types of literature on carbon emissions and carbon intensity.
First, there are studies on the factors influencing carbon emissions and intensity. Most literature refers to the study of energy consumption variation decomposition and adopts index decomposition methods, such as the Laspeyres, Divisia, and Logarithmic Mean Divisia Index (LMDI), to decompose carbon emissions or carbon intensity (Wang et al., Citation2010; Yang et al., Citation2019). As more in-depth research has been conducted, more scholars have begun to study the driving factors using the IPAT model, KAYA, and STIRPAT models (Gani, Citation2021; Khoshnevis Yazdi & Dariani, Citation2019; Wang & Xia, Citation2020). Combined with the LMDI decomposition method, these models provide determinants affecting carbon dioxide emissions, including population, economic income, energy intensity, industrial structure, and energy consumption structure. By adding dimensionless variables to the STIRPAT model, scholars have recently examined factors such as urbanization and R&D investment (Lin & Xu, Citation2020).
Second, research has been conducted on the prediction of carbon emissions and intensity. Requirements for carbon emission reduction and carbon intensity of all countries around the world have been presented since the ‘Kyoto Protocol’ was passed in 1997 and the 2009 Copenhagen World Climate Conference was held. Researchers are also studying the feasibility of reducing carbon emissions. The main tools for carbon emission prediction are model and scenario analyses, although some scholars also use model and Monte Carlo dynamic analyses. Currently, there are three main carbon emission models in academic circles: 1) the carbon factor analysis model, which includes the IPAT model, STIRPAT model, and extended STIRPAT model (Yuan et al., Citation2020); 2) the energy consumption and energy structure model. According to this model, carbon dioxide emissions are the sum of carbon produced by different types of energy consumption, and carbon emissions can be predicted based on the total energy consumption and energy structure predicted (Lin & Li, Citation2015); and 3) the energy system optimization model, which predicts energy and carbon from the perspective of system dynamics (Sun et al., Citation2022; Martin & Chen, Citation2016).
Third, research has been conducted on the carbon peaks. Lin and Jiang (Citation2009) first began to research carbon peaks in China; however, it was not until the Paris Agreement was signed in 2014 that much research was conducted. This type of research was carried out from three perspectives: 1) the Kuznets curve of our environment was calculated to measure the per capita GDP at the peak to calculate the time when the per capita GDP reached this (Xu & Song, Citation2010; Yue et al., Citation2022); 2) to estimate carbon emissions through economic accounting methods (Guo, Citation2014); and 3) to make predictions based on carbon emissions (Pan et al., Citation2021; Yang & Zhengwei, Citation2023).
Although research in the carbon field has harvested a lot and the research methods have become relatively mature, there is still room for improvement. (1) Very few studies have been conducted from a technical perspective, which could be attributed to the fact that energy-related technologies are not measured and technical research in the carbon field is limited. (2) Supportive economic theory is lacking and scholars have directly studied carbon intensity using existing carbon emission models, most of which are not supported by relevant economic theories. (3) Most extant research on environmental policies is based only on qualitative analysis. The emission reduction policies proposed by scholars are based on a qualitative analysis of the conclusions of their articles.
3. Research on the impact of energy policy on energy intensity amid biased technical change
The energy tax and R&D subsidy policy of the energy department affect energy demand and energy intensity in two ways, with one being the alternative way, which means that the energy tax will increase the price of energy to a degree higher than that of other factors; therefore, other factors will be replaced. The other is the technological approach, which means that policies affect the direction of technological progress by influencing the demand and supply of technology and changing the amount of energy demand. On the one hand, energy tax changes the market’s demand for technology and affects the marginal benefit of technology production in the R&D department; on the other hand, R&D subsidies change the marginal cost of technology production of the R&D department.
3.1. Production environment
Based on ideas drawn from Acemoglu’s research (Citation2002), this study divides the production field into the final product production department, intermediate product production department, and R&D department. The intermediate product production department is further divided into labour-intensive, capital-intensive, and energy-intensive sectors. This study describes the input-output relationship in China’s production field with the non-nested CES production function:
(1)
(1)
In the above formula, L, K, and E respectively represent the input of labour, capital, and energy; measures the factor-augmenting technical change;
is the substitution parameter reflecting the substitution between factors.
、
、
are distribution parameters that determine the importance of each input factors.
This paper adopts the laboratory equipment model to form the technical production function,
(2)
(2)
In the above formula, is the number of operating tools or software newly added by i department;
is the total amount of research and development invested by i research and development department; and
is the technical production coefficient of i research and development department. The technology production function demonstrates that the marginal benefit of R&D investment is constant.
3.2. The impact of energy tax policy on energy intensity
The use of energy taxes raises the cost of energy for enterprises, indicating that energy taxes raise energy intensity by upgrading the price of energy. Liu and Xu (Citation2020) clarify the theoretical relationship between energy prices and energy intensity amid biased technical changes.
(3)
(3)
In this formula, EI is energy intensity, q is energy price, the variable
w represents the labour price, r the capital price, and
the elasticity of substitution among factors.
Supposing the energy tax rate is then the energy price after tax is added is
By substituting these into the formula, we can determine the influence of the energy tax rate on energy intensity. Furthermore, the model considers the impact of energy prices on energy intensity in the lag period. However, in the long run, we can combine these lagged variables, and this study deduces the impact model of the energy tax on energy intensity:
(4)
(4)
In the formula, From the above formula, we can identify that when the energy tax rate increases by 1 percentage point, such as increases from 1% to 2%, the decrease of energy intensity will be within the rate of
Putting into the value of the elasticity of substitutionFootnote1, we can gauge that the reduction in energy intensity will be within the range of
Furthermore, the decrease in energy intensity after the energy tax policy is applied is inversely proportional to the energy tax rate. A higher energy tax rate leads to a smaller decrease in energy intensity; that is, the effect of the energy tax policy becomes less apparent. In other words, the energy intensity reduction effect of the energy tax policy is stronger when the energy tax rate of a country or of a certain period is lower than when the energy tax rate is high.
3.3. Impact of R&D subsidy policies on energy intensity
As the R&D allowances for the energy technologies of the R&D production department increase, the total cost of the R&D sector decreases and thus the R&D marginal cost of each energy-augmenting technology decreases. Consequently, it influences the balanced number of energy-augmenting technologies, and ultimately influences the energy intensity. Supposing the total R&D cost of the energy R&D sector is
if the R&D subsidy given by the government is
then the total cost of technology R&D investment in the energy sector will be reduced to
As this study adopts a production function with constant marginal returns, the marginal cost MC of each technology produced by the R&D department is equal to its average cost AC. Therefore, under the R&D subsidy rate the marginal cost of each R&D technology will decrease to
which can be deduced from the clearing conditions of the technical market:
(5)
(5)
Liu and Liu (Citation2022) derived the relative price ratio of intermediate goods and the relative demand curve of the following factors:
(6)
(6)
(7)
(7)
This study replaces formula (6) for formula (5) and obtains:
(8)
(8)
After substituting the above formula into formula (7), we obtained the following formula:
(9)
(9)
Thus, we obtain the product price ratio of the intermediate products:
(10)
(10)
Substituting this into formula (4), we obtain the following model for the effect of R&D allowances on energy intensity:
(11)
(11)
In this formula, and
、
respectively represent:
(12)
(12)
Therefore, when the R&D subsidy rate increases by 1 percentage point, such as from 1% to 2%,
increases
and
increases
so the energy intensity will decrease to a degree between
Entering the elasticity of the substitution value, we can identify that once R&D subsidy rate
increases by 1, the energy intensity will decrease to the degree between (0,
). We can observe that the decrease in energy intensity caused by the R&D subsidy policy is proportional to the subsidy rate. A higher subsidy rate will decrease energy intensity to a greater extent; that is, it strengthens the effect of the R&D subsidy policy. In other words, when R&D subsidies account for a relatively high proportion of R&D inputs in a country or in a period, the effect of reducing energy intensity caused by the implementation of the subsidy policy is lower than that of a higher subsidy rate.
4. Derivation of the relationship between carbon intensity and energy intensity
4.1. The measurement methods of CO2 emission
The measurement methods of carbon dioxide emissions include the inventory method, material balance algorithm, model estimation method, life cycle method, and actual measurement method. The inventory method is currently recognised internationally, and it measures carbon dioxide emissions by multiplying the carbon emission coefficient provided by the United Nations Intergovernmental Panel on Climate Change (IPCC) by the consumption of the corresponding energy species. This method is practical, easy to perform and does not require strict statistical analyses. Therefore, the corresponding data from the Statistical Yearbook and Energy Statistical Yearbook can be used for this calculation. Therefore, most countries have adopted this method to compile the national GHG emission inventories. Some scholars measure carbon emissions by dividing energy into coal, oil, and natural gas, whereas others subdivide this category into nine subcategories or even sub-15 categories. This study adopts the former classification.
However, the academician Ding (Citation2010) pointed out that the carbon dioxide emissions promised by the Chinese government refer to emissions from fossil energy utilization and cement production. Therefore, this study also refers to the practice of academician Ding to calculate China’s carbon emissions, which include not only CO2 emissions from energy consumption but also those from cement production. The following formula clarifies the calculation of CO2 emissions in tons:
(13)
(13)
represents the consumption of the ith species of fossil energy, and
represents the carbon emission coefficient of the ith species of fossil energy. Noticeably, four indicators need to be provided to calculate carbon dioxide emissions: (1) the proportion of fossil energy consumption in total energy consumption; (2) the energy variety structure in fossil energy, which include the proportion of coal, oil, and gas; (3) CO2 emission coefficients of various energies; (4) CO2 emissions from cement production.
4.2. The proportion of fossil energy and its structure
The ‘China Statistical Yearbook 2022’ elaborates on China’s total fossil energy consumption and fossil energy structure from 1978 to 2020. The ‘Carbon Peak Action Plan before 2030’ clarifies that the proportion of non-fossil energy in China’s primary energy consumption in 2025 will be about 20% and 25% in 2030. This study assumes that the mean proportion of fossil fuel energy consumption in China from 2025 to 2030 will be 77.5%. The ‘China Statistical Yearbook 2022’ indicates that China’s coal, oil, and natural gas consumption in 2020 will account for 67.15%, 22.18%, and 10.67% of total fossil energy consumption, respectively. This study assumed that this proportion is constant.
4.3. The coefficient of carbon emission
The ‘2006 IPCC Guidelines for National Greenhouse Gas Inventories’ provides carbon dioxide emission coefficients for various energies. However, the proportion of oxidised carbon is assumed to be 1, which is inconsistent with the reality. Moreover, because new equipment at different levels is introduced, adopting an internationally unified carbon emission coefficient will prove that the carbon dioxide emissions of developing countries are higher than the actual amount (Ding, Citation2010). Li (Citation2014) used the oxidation rates of different fuels recommended by the ‘Guidelines for Compilation of Provincial Greenhouse Gas Inventories’ and ‘General Principles of Calculating Comprehensive Energy Consumption’ to calculate the carbon dioxide emission factors, and calculates the carbon emission factors basing on the carbon conversion coefficient (44/12). The calculation formula is as follows:
(14)
(14)
The following table lists the average Qnet, carbon content per unit calorific value, carbon oxidation rate, and standard coal coefficients for coal, crude oil, and natural gas. By substituting these data into the above formula, we obtained the carbon emission coefficients of the corresponding energies (the results are presented in ).
Table 1. Calculation results of carbon emission coefficients of three energies.
4.4. Measurement of carbon dioxide emissions from cement production
The academician Ding (Citation2010) noted that carbon dioxide emissions from cement production accounted for a little more than 10% of the total carbon dioxide emissions in China. However, because China’s cement production accounts for half of the world’s total production, relevant research indicates that there will be no apparent increase in the future. This study predicts the carbon dioxide emissions from energy in China from 1978 to 2021 in light of various energy consumption and carbon emission coefficients (see ). By referring to the method proposed by the academician Ding, this paper calculates the carbon dioxide emissions from cement production over the past years; and finally, this paper assumes that the CO2 emissions from cement production in 2025 and 2030 will not change significantly from that of 2021, which totals 1.179 billion tons.
Table 2. China’s carbon dioxide emissions; unit: billion tons of CO2.
4.5. The relationship between carbon intensity and energy intensity
The formula for carbon dioxide emissions in 2025 and 2030 can be calculated according to the above four indicators, where E is the total energy consumption and the unit is 10,000 tons of standard coal. This study used gigatons as the unit to calculate carbon dioxide emissions:
(15)
(15)
After dividing both sides of the equation by Y (GDP, unit is 100 million yuan), the relationship between carbon intensity () and energy intensity (E/Y) can be deduced. This paper uses ton/10,000 yuan as the unit of carbon intensity:
(16)
(16)
5. Policies to achieve the carbon intensity targets
5.1. Carbon intensity estimates in 2025 and 2030
By 2025, China’s carbon intensity will decrease by 18% from that in 2020, and by 2030, China’s carbon intensity will decrease by more than 65% from that in 2005. Based on the formula (15) and formula (16), this study calculates the carbon intensity of China from 1978 to 2021 (see )Footnote2. As per calculations, to achieve the targeted carbon intensity, China’s carbon intensity needs to drop to 1.76 tons/10,000 yuan in 2025 and below 1.49 tons/10,000 yuan in 2030.
Table 3. China’s carbon intensity from 2005 to 2021; unit: ton/10,000 yuan.
5.2. Energy intensity estimates for 2025 and 2030
Considering the relationship between energy intensity and carbon intensity, this study estimates the energy intensity that China needs to achieve by 2025 and 2030 to realise the carbon intensity targets. First, it is necessary to predict China’s future GDP. Since a new economic normal began in China in 2014, the GDP growth rate has dropped below single digits, and the GDP growth rate in recent years has been approximately 6%. The scholar Lin Yifu also predicts China’s future GDP growth and points out that before 2035, China’s economy will increase at an annual rate of 8%, which may turn out to be an average rate of 6%. Therefore, this study makes three assumptions regarding China’s GDP growth rates in 2025 and 2030, which are 5%, 6%, and 7% respectively. We then deduce the energy intensity values in the three GDP growth scenarios according to formula (16) and present the results in .
Table 4. Energy intensities in 2025 and 2030; unit: 10,000 tons of standard coal/10,000 yuan.
5.3. Energy taxes to achieve the carbon intensity targets
From the previous analysis, we can infer that to achieve the carbon intensity targets for 2025 and 2030, it is necessary to decrease the energy intensity to 0.8292–0.8306 in 2025 and 0.7534–0.7545 in 2030, which means that the energy intensity in 2025 will decrease by 11.94%–12.08% than that of 2020, and we take the mean value of 12%; in 2030, the energy intensity will decrease by 20%–20.12% than that of 2020 2020, and we take the mean value of 20%.
According to formula (4), the energy intensity will decrease to within the range of if the energy tax rate
increases by one percentage point. China implemented a new resource tax rate on September 1, 2020, which stipulates that the tax rate on crude oil and natural gas is 6%, and the tax rate on coal is 2%–10%. Therefore, this study considers 6% as China’s energy tax in 2020, which means that
Therefore, if the energy tax increases from 6% to 7%, the energy intensity will decrease by 0.57%–0.94%.
Therefore, if we need to decrease the energy intensity in 2025 by 12% from that of 2020, it is necessary to increase the energy tax rate by 21.02 percentage point, which means that the energy tax rate will increase to 27.02% from the current rate of 6% or between 12.72 percentage point and 21.02 percentage point, and the energy tax rate will increase to between 18.72%–27.02%. It is worth highlighting that increasing the energy tax rate by 21.02 percentage point is a conservative plan, which means that the energy intensity will decrease by at least 12% to reach the target; however, increasing the energy tax rate by more than 12.72 percentage point and less than 21.02 percentage point is a probability plan. In other words, under this growth rate, energy intensity will decrease between 7.26%–19.83%, and the energy intensity has a certain probability of achieving the target. The greater the increase in the energy tax rate, the higher is the probability of achieving the energy intensity target.
If the energy intensity in 2030 is reduced by 20% from that in 2020, the energy tax rate needs to increase by 35.04 percentage points, which means that the energy tax rate will increase from the current 6% to 41.04%, or more than 21.2 percentage points and less than 35.04 percentage points, which means that the energy tax rate will increase to 27.2%–41.04%. Among them, increasing the energy tax rate by 35.04 percentage points is a conservative plan, which means that the energy intensity will decrease by at least 20% to reach the target value; the energy tax increase by more than 21.2 percentage points and less than 35.04 percentage points is a probability plan, which means that the decline in energy intensity is between 12.1% and 33.06%, and the targeted energy intensity might be achieved (please refer to for details).
Table 5. The decrease of energy intensity under different growth rates of energy tax.
Therefore, we can understand that four energy tax plans can be implemented to achieve the targets in 2025 and 2030: first, a conservative plan, which means increasing the energy tax rate in 2025 and 2030 to 27.02% and 41.04%, respectively; thus, the carbon intensity targets in 2025 and 2030 will be met. All energy prices in China include taxes; for example, the price of natural gas in one province of China is 2 yuan per cubic metre, which includes a tax rate of 6%. Therefore, raising the tax rate to 27% and 41% would be equivalent to raising the price of natural gas to 2.39 yuan per cubic meter and 2.66 yuan per cubic meter. The carbon intensity targets for 2025 and 2030 could be achieved if the tax rates included in other energy prices were increased by the same amount on average; second, the probability plan 1, which means the energy tax rate will reach 27.02% in 2025, and the energy tax rate will be greater than 27.2% and less than 41.04% in 2030, the target value in 2025 is sure to be achieved, but the target in 2030 cannot be guaranteed to be met; third, the probability plan 2, which means the energy tax rate in 2025 is greater than 18.72% and less than 27.02%, and the energy tax rate in 2030 will increase to 41.04%, then the target in 2025 might be achieved, but the target in 2030 is sure to be achieved; fourth, the probability plan 3, which means the energy tax rate in 2025 is greater than 18.72% and less than 27.02%, and the energy tax rate in 2030 is greater than 27.02% less than 41.04% (see for details).
Table 6. Energy tax plans to achieve carbon intensity targets in 2025 and 2030.
5.4. R&D Subsidy to achieve carbon intensity targets
According to formula (11), the energy intensity decreases to the range from (0, ) if the R&D allowance rate
increases up by one percentage point. This study considers government funds in R&D expenditures as government subsidies, and the ratio of government funds to R&D expenditures as the R&D subsidy rate. As China’s R&D expenditure in 2020 was 2,439.31 billion yuan, of which government funds cover 482.56 billion yuanFootnote3, the R&D subsidy rate in China in 2020 was 19.8%. Therefore, to increase the R&D subsidy rate by one percentage point, the energy intensity must decrease between 0 and 0.49%. This means that to achieve the 2025 target value, the R&D subsidy rate needs to increase by at least 24.37 percentage points in five years to 44.17%; however, to achieve the 2030 target, the R&D subsidy rate needs to increase by at least 40.62 percentage points in ten years to 60.42%. For example, 19.8% of the R&D funds of an energy company in China was supported by the government in 2020. If we can increase the proportion of all enterprises in China to 44.17% and 60.42% by 2025 and 2030, respectively, we can achieve the carbon intensity targets in 2025 and 2030.
5.5. Policy mixes to achieve carbon intensity targets
The above analyses indicate that if an appropriate energy tax policy or R&D subsidy policy is adopted, China’s carbon intensity targets for 2025 and 2030 can be achieved. However, this study holds the opinion that each policy has its disadvantages: the implementation of energy tax policies can decrease energy intensity; however, the model (EquationEquation 4(4)
(4) ) indicates that the reduction in energy intensity caused by energy tax policies decreases as the tax increases. Therefore, if we apply a very high energy tax at the beginning, such as in a conservative plan, we can ensure that the carbon intensity targets of 2025 and 2030 will be met. However, this will make it more difficult to adopt this policy in the future.
The R&D subsidy policy can be applied to decrease energy intensity; the higher the subsidy rate, the greater the decrease in energy intensity. Therefore, it is not difficult to adopt this policy again. From this perspective, an R&D subsidy policy is better than an energy tax policy. However, the R&D subsidy policy puts pressure on the government’s finances on the one hand, and it is not as effective in decreasing energy intensity as an energy tax policy.
As both policies have advantages and disadvantages, we recommend that the Chinese government combine them. The Chinese government has not suggested adopting an aggressive energy tax policy nor is it considering the probability plan 3. Although it cannot guarantee that the carbon intensity targets in 2025 and 2030 will be met, the combination of the R&D subsidy policy and tax policy can be used to decrease energy intensity while avoiding its premature overdraft on the one hand; on the other hand, it can use the sustainable advantages of the R&D subsidy policy to ease the fiscal pressure simultaneously.
First, we need to determine the appropriate energy tax growth in Probability Plan 3, calculate the difference from the target value by its pulling effect, and then calculate the required increase in R&D subsidies necessary to complete this difference according to the pulling effect of the subsidy policy. For example, to achieve the carbon intensity target in 2025, we need to increase the energy tax rate to 19% and maintain the energy intensity reduction rate between (7.42% and 12.26%). However, it cannot guarantee that the target value will be met and continues to lag behind the target by 4.58 percentage points. Therefore, we can adopt an R&D subsidy policy to ensure that this goal is met. In light of the pulling rate of the R&D subsidy rate on energy intensity, we can calculate that it will take 9.3 percentage points to achieve this goal, which means that we need to increase the R&D subsidy rate to 29.1%. We proposed several combinations, which are presented in the table below.
Therefore, this study proposes three policy options for achieving China’s carbon intensity targets by 2025 and 2030. In the first scenario, China increases its energy tax rate on fossil fuels to 19% by 2025 and 28% by 2030. Simultaneously, China will increase its R&D subsidy rate for energy technology R&D enterprises by 29.1% and 34.92%, respectively. The second is a higher energy tax rate of 21% in 2025 and 31% in 2030, as well as an increase in the R&D subsidy rate to 26.78% and 31.44%, respectively, a policy mix that could also meet China’s carbon targets. The third option is to continue raising the energy tax rate to 23% in 2025 and 33% in 2030 and the R&D subsidy rate to 24.47% and 29.12%, respectively ().
Table 7. Proposed policy mixes to achieve carbon intensity targets.
6. Main conclusions and limitations
6.1. Conclusions
From a technical perspective, this study examines China’s carbon target under the framework of biassed technical change to provide a theoretical model of carbon intensity from the perspective of energy technology for the academic community. Therefore, for policy research in the fields of energy and the environment, we will no longer be limited to qualitative analysis; quantitative measurement will open a new perspective for this field of research. Linhui, who conducted a similar study, also simulated the impact of environmental policies (environmental tax or emission trading regulatory policies) and R&D subsidies on environmental quality (carbon emissions) from a quantitative perspective and came up with a view relatively consistent with our study, that is, they also concluded that both environmental policies and R&D subsidy policies have advantages and disadvantages. However, the difference in this study is that we adopt China’s actual data for our research. Instead of running numerical simulations. The following three conclusions were drawn from this study:
First, as the energy tax and R&D subsidy rates increase, energy intensity will decrease, and the energy tax policy has a greater pulling effect on energy intensity than does the R&D subsidy policy. If the energy tax rate increases by one percentage point, the energy intensity will decrease by 0.57%–0.94%; if the R&D subsidy rate increases by one percentage point, the energy intensity will decrease by 0–0.49%, indicating that the maximum pulling effect of the R&D subsidy policy is smaller than the minimum pulling effect of the energy tax policy.
Second, both energy tax and R&D subsidy policies have advantages and disadvantages in reducing carbon intensity. Energy tax policy is more effective than R&D subsidy policy in decreasing energy intensity. However, an overly high energy tax will make it more difficult to implement the energy tax policy in the future, and the higher the R&D subsidy rate, the more the energy intensity will decrease. Therefore, from the perspective of its impact on future policies, an R&D subsidy policy is more effective than an energy tax policy. Therefore, the government should adopt moderate energy taxes and R&D subsidy policies.
Third, we recommend that the Chinese government adopt a policy mix to reduce carbon intensity. As each policy is imperfect, the Chinese government is recommended to consider combining policies, which means to match the energy tax rate policy of a certain rate of increase with the R&D subsidy rate policy of a certain rate of increase. We also recommend to gradually raise the tax on fossil fuels such as oil, coal, and natural gas to increase the cost of fossil fuels and stimulate cost-saving technological innovation for businesses. For example, the government can raise the energy tax rate from 6% to 19% by 2025, and 28% by 2030. Simultaneously, the government could increase funding subsidies for energy technology R&D, especially for new energy and carbon capture technology R&D enterprises. For example, the rate of R&D subsidies is expected to increase to 29.1% in 2025 and to 34.92% in 2030.
6.2. Limitations
In this study, some of the content of the article has been simplified. First, for the value of elasticity of substitution, this paper directly adopts the calculated value of the author (2020), and the data range involved in the calculation process is 1980–2016. Although this will not have a significant impact on the results, expanding the sample to 2020 will undoubtedly improve the accuracy of the results. Second, in terms of the calculation of the carbon emission coefficient, this study fixed the proportion of coal, oil, and natural gas in fossil energy and the proportion of fossil energy in a certain year; however, these proportions differ from year to year, therefore, the calculated carbon emission coefficient should have a small difference every year. This study simplifies this by assuming that the carbon emissions coefficient is the same every year; thus, the relationship between carbon and energy intensities does not change over time.
Disclosure statement
No potential conflict of interest was reported by the authors.
Additional information
Funding
Notes
1 The author measured the elasticity of substitution of factors in China in 2020 (Liu & Xu, Citation2020), which is directly quoted here.
2 Due to lack of space, only partial data are listed here.
3 The data are from ‘China Statistical Yearbook of Science and Technology 2021’.
References
- Acemoglu, D. (2002). Directed technical change. Review of Economic Studies, 69(4), 781–809. https://doi.org/10.1111/1467-937X.00226
- Acemoglu, D., Aghion, P., Bursztyn, L., & Hemous, D. (2012). The environment and directed technical change. The American Economic Review, 102(1), 131–166. https://doi.org/10.1257/aer.102.1.131
- Ding, Z. (2010). A rough analysis of China’s CO2 emission reduction targets in 2020. Energy and Energy Conservation, 3, 1–5.
- Gani, A. (2021). Fossil fuel energy and environmental performance in an extended STIRPAT model. Journal of Cleaner Production, 297, 126526. https://doi.org/10.1016/j.jclepro.2021.126526
- Guo, C. (2014). Estimation of China’s industrial carbon emission reduction potential. China Population, Resources and Environment, 24(9), 13–20.
- Hu, A. G. (2021). Main approaches to China’s carbon peak by 2030. Journal of Beijing University of Technology (Social Sciences Edition), 21(3), 1–15.
- Khoshnevis Yazdi, S. K., & Dariani, A. G. (2019). CO2 emissions, urbanisation and economic growth: Evidence from Asian countries. Economic Research-Ekonomska Istraživanja, 32(1), 510–530. https://doi.org/10.1080/1331677X.2018.1556107
- Li, H. Y. (2014). Study on carbon dioxide intensity in China based on comparable price IO-SDA model. Southwest University of Finance and Economics.
- Lin, B. Q., & Jiang, Z. J. (2009). Environmental Kuznets curve prediction and influencing factors analysis of carbon dioxide in China. Management World, 4, 27–36.
- Lin, B. Q. (2018). The energy revolution promotes China’s clean and low-carbon development of the “tackling period” and “window period. China Industrial Economy, 6, 15–23.
- Lin, B. Q., & Xu, B. (2020). Research and development investment, carbon intensity and regional CO2 emissions. Journal of Xiamen University: Philosophy and Social Sciences, 4, 15.
- Lin, B. Q. (2021). Difficulties and solutions for China to become carbon neutral. New Finance, 7, 26–29.
- Lin, B. Q., & Li, J. L. (2015). Transformation of China’s energy structure under the constraints of environmental governance—Analysis based on coal and carbon dioxide peaks. Chinese Social Sciences, 9, 84–107. + 205.
- Liu, H. H., & Liu, H. (2022). An empirical study on the endogeneity of directed technical change in China. Economic Research-Ekonomska Istraživanja, 35(1), 2693–2711. https://doi.org/10.1080/1331677X.2021.1975148
- Liu, H. H., & Xu, C. (2020). Research on China’s energy intensity change under biased technical change. Science and Technology Management Research, 40(8), 251–259.
- Martin, & Chen, W. Y. (2016). Study on the peak level and path of China’s carbon emissions in 2030. China Population, Resources and Environment, 1, 4.
- Pan, D., Li, N., Li, F., Feng, K. S., Peng, L. L., & Wang, Z. (2021). Development of peaking strategy for eastern China based on energy carbon emission prediction. Journal of Environmental Sciences. https://doi.org/10.21203/rs.3.rs-2175268/v1
- Sun, B. D., Zhang, J., Han, Y. J., Chun, Y. T., Zhang, J. B., & Wu, L. (2022). Evolution trend and path of China’s energy system under the dual carbon goal of energy security and low-carbon transition. China Coal, 48(10), 1–15.
- Wang, F., Wu, L. H., & Yang, C. (2010). Research on driving factors of carbon emission growth in China’s economic development. Economic Research Journal, 45(02), 123–136.
- Wang, X. Q., & Xia, D. (2020). Study on carbon emission and influencing factors of industrial coal production and consumption: A comparison between Anhui and Henan Provinces based on STIRPAT-EKC. Soft Science, 34(8), 6.
- Xu, G. Y., & Song, D. Y. (2010). Empirical study on Kuznets Curve of carbon emission environment in China: Based on provincial panel data. China Industrial Economy, 5, 11.
- Yang, L. S., Zhu, J. P., & Jia, Z. J. (2019). The impact factors and current challenges of carbon emission reduction in China: From the perspective of technological progress. Economic Research Journal, 54(11), 118–132.
- Yang, W., & Zhengwei, T. (2023). Prediction of “double carbon” target value in L City based on Grey Markov Model. China-Arab States Science and Technology Forum, 1, 103–107.
- Yuan, X. L., Xi, J. H., Li, C. P., & Zhang, W. L. (2020). Prediction of peak carbon emissions and research on emission reduction potential in China’s industrial sector. Forum on Statistics and Information, 35(9), 11.
- Yue, Q., Qin, X. Y., Huang, H. G., & Wang, H. Q. (2022). Regional coordination of peaking carbon neutrality: Evidence and approaches. Science of Finance and Economics, 1, 16.
- Zhang, X. L., Huang, X. D., Zhang, D., Geng, Y., Tian, L. X., Fan, Y., & Chen, W. Y. (2022). Research on the path and policy of energy economic transformation under the goal of carbon neutrality. Management World, 38(1), 35–66.
- Zhao, W. (2022). China’s goal of achieving carbon neutrality before 2060: Experts explain how. National Science Review, 9(8), nwac115. https://doi.org/10.1093/nsr/nwac115