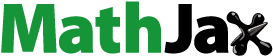
ABSTRACT
Industrial dwelling construction (IDC) combines offsite and onsite construction methods. The current work proposes a multiple-criteria decision-making assistant system (DMAS) approach for evaluating home buildings, selecting decision agents, and determining acceptable IDC types and characteristics for large-scale housing enhancement. Using the analytical hierarchy process (AHP), a multicriteria DMAS was planned. Literature reviews and expert interviews were used to assess thirty decision agents. To validate the procedure, five IDCs were analyzed as case studies. Thirty professionals analyzed decision agents' and housing systems' priority vectors using questionnaires. Three decision agents were identified: (1) user needs, (2) the building industry, and (3) the supply chain. Consequently, Prefabricated Reinforced Concrete Slab, Beam, and Column Blocks (PRCSBCB) and Light Steel Frame Wall Panels (LSFWP) can be used to construct suitable dwellings. These construction options are low-cost, lightweight, and easy to install. They also use inexperienced local workers. Results showed the method's potential and usefulness with so many choices and decision agents. This research examines knowledge and skill gaps in Iran's IDC and encounters a shortage of IDC specialists and mass-construction variants. The study identifies key decision-agents and housing options for quick, appropriate, and cost-efficient mass housing and helps professionals, entrepreneurs, and decision-makers make better decisions.
1. Introduction
Suitable IDC and decision-making systems are available for large-scale and rapid construction, which makes the project more efficient. To promote IDC application, experts must acquire effective IDC decision criteria from local building components. As long as alternatives to IDCs exist, developers must choose the best IDC for mass housing progress. Shen estimates rapid house industrialization when GNP per capita surpasses 600 dollars (Jianzhong Citation2002). Iran’s GNP indicates it has passed rapid industrialization point. Due to high housing demand, developing countries like Iran have struggled to provide adequate and affordable housing (Keivani and Werna Citation2001). In Tehran, where supply and demand are deeply polarized, low-cost housing is in high demand. In recent decades, neither private nor public housing constructions have met demand. One of the key challenges was selecting the right housing system (Burt, Aron, and Lee Citation2001). The government planned to address this by creating low-cost IDCs for dwellings. Two variables prevent appropriate IDC justification.: Firstly, IDC evaluations lack explicit decision factors. Many existing methodologies have been criticized for being quantitatively and financially exaggerated (Pan, Gibb, and Dainty Citation2007). These criticisms have led to inadequate IDC attention. High shipping and import costs, a lack of skills, and local resources have led to IDC failure in many developing nations (MR Abdullah and CEgbu Citation2010). Secondly, Since multicriteria DMASs use different approaches, many areas for decision-making enhancement remain unaddressed, including weighing, scoring, validation, and outcome testing, notably in IDC assessment (Blismas, Pasquire, and Gibb Citation2006). To build rapid and affordable housing in underdeveloped nations, particularly Central Asia, it is necessary to select appropriate IDCs in a systematic and effective manner at the appropriate time and location. This study assesses housing systems using an AHP-based multicriteria DMAS. The AHP analyzes the analogical relevance of linked decision agent variables and selects relevant IDCs for housing expansions (Kokaraki et al. Citation2019; Wong and Li Citation2008).
2. Literature review
2.1. Theoretic context
Three main theoretical perspectives help to establish a framework for decision-making analysis (Edwards Citation1954; Chankong and Haimes Citation2008). The aim of the framework is to better understand the decision-making process with regard to the evaluation and selection of suitable IDCs (Engström and Hedgren Citation2012). Multi-methodology in decision making requires the application of appropriate multi-criteria DMASs (Hedgren and Stehn Citation2014; Dainty Citation2008).
2.2. Multi-criteria DMAS
A multi-criteria decision-making assistant system (DMAS) can organize the necessary information and procedures to allow decision makers to make an informed and effective decision (Saaty Citation2005). The implementation of a DMAS tool in housing system selection includes the participation of stakeholders and professional advisors (Medineckiene et al. Citation2015). It needs to meaningfully combine decision factors that influence the decision regarding which type of IDC to use while considering the local context (Zeleny Citation2012; Chen, Okudan, and Riley Citation2010; Karamoozian et al. Citation2019).
2.3. Decision agents
Although many decision agents have been employed to study housing systems, criteria integration has been neglected (W Pan, AR Dainty, and AG. Gibb Citation2012). Pan et al (Wang et al. Citation2020) presented a conceptual model for synthesizing decision elements using a systemic value-based methodology and determining their importance in making judgments. The decision elements’ concepts for analyzing imported dwelling systems are still lacking (Akmam Syed Zakaria et al. Citation2018). As a consequence of extensive literature study and expert judgment participation, this research addresses a gap in the literature with precise and integrated decision agents (Yu Citation2013; Shahpari et al. Citation2020; Pan, Wong, and Hui Citation2011) As shown in , the majority of the assessment criteria are composed of well-known concepts such as: The Building industry, User requirements, the Supply chain, Sub-structures, and the Environment and Surroundings, as well as increasingly relevant Civic-political aspects. Effective decision-making requires the integration of theoretical viewpoints with linked data and decision-makers’ behavior. As like classical dominant variables, customer demand, affordability, and construction speed are indicated as major decision agents (Buchman Citation2020).
Table 1. References to proposed IDC decision factors.
2.3.1. User requirements
User needs rely on the building construction industry’s ability to create affordable home alternatives (Pan Citation2007; Luo et al. Citation2020; Wheeler and Beatley Citation2014).
2.3.2. Supply chain
Another critical decision agent for effective housing advancement is the supply chain, which should be considered while picking an appropriate housing system(Vrijhoef and Koskela Citation2000; Kanda and Deshmukh Citation2008; Cachon and Fisher Citation2000). For the supply chain to meet the demands of building construction, new investment methods and efficiency are absolutely vital (RezaHoseini, Noori, and Ghannadpour Citation2021; Benton and McHenry Citation2010; Mashayekhi Citation2019; Karamoozian, Karamoozian, and Ashrafi Citation2013).
2.3.3. Building industry
Because labor costs account for 30 to 50% of total construction costs, professional workforce accessibility determines overall building construction efficiency and sophisticated housing system applications (Jato-Espino et al. Citation2014; Karimi et al. Citation2018; Hussain, Xuetong, and Hussain Citation2020). To meet the huge demand for housing, the local building construction industry’s general capacity must be improved (Madanipour Citation2006).
2.3.4. Sub-structures
Any discussion of construction development must take into account both existing structures and sub-structures (Schiller Citation2007). The lower the density of buildings in a given region, the greater the amount of material absorbed by infrastructure (Yang and Kohler Citation2008).
2.3.5. Environment and surroundings
The environmental role is an un deniable factor in the construction industry (Pries and Janszen Citation1995). There are more changes in the environment, which will have a big effect on construction management. One of the most critical strategic decisions is whether or not to specialize or diversify. Energy efficiency, Pollution and Contamination control, Vulnerability conditions such as exposure to the side forces like earthquakes, floods, storms, etc (Oven and Rigg Citation2015). Recyclability/ losses, Healthiness and safety are the key sub-factors affecting the construction process. New construction processes and materials have addressed several problems raised by building waste, including resource conservation and pollution reduction (Afzali and Hamzehloo Citation2018). There are many varying conceptions of vulnerability, and many different viewpoints on what might or should not be used in the comprehensive analysis of vulnerability(Rashed and Weeks Citation2003). Since Iran is located on the earthquakes zone, and especially Tehran is near two major seismic faults, this paper also quantified the primary influencing environmental factors in minimizing susceptibility, and boosting construction ability to respond to environmental changes such as earthquakes, storms, floods, etc (Sadeghi, Ghafory-Ashtiany, and Pakdel-Lahiji Citation2015).
2.3.6. Civic-political
The process of constructing is a social procedure (Schriewer Citation2004). Therefore, in building construction systems, the civic-political dimension must be clearly considered (Higgins-D’Alessandro Citation2010).
3. Methodology
To fulfill the study’s goals, literature studies, question reviews, knowledge extraction interviews, and quantitative and qualitative research were considered (Groat and Wang Citation2013; Patton Citation1990). A decision-analysis framework for the research topic requires both qualitative and quantitative work. The research revealed precise decision factors. Interviews with IDC specialists aided in defining key criteria. A questionnaire was used to collect quantitative and qualitative data for the assessment model. An online EOC (Expert Option Comparison) trading software platform based on AHP was used to provide a realistic assessment.
The AHP comparison in pairs limits the experts’ judgment consistency by status. The EOC allows responders to immediately assess their consistency ratio and weight grade. Additionally, this illustrates that decision-makers are capable of making discerning errors, as long as the errors are within defined limits, such as a constancy ratio of 0.1 or fewer (Saaty Citation1988).
3.1. Expanding the decision-making pattern
Expanding the pattern in three stages: The framework begins by identifying the critical decision elements that drive IDC selection; 2; it then defines the system selection procedure; and 3; it finally discusses the system selection methodology and analysis tools for the given model. depicts the general selection model.
3.1.1. Classifying the related decision elements
To expand the data set units of the decision-making model, a complete set of decision agents must be studied. This study used a multi-method approach to provide a perceptual decision-making framework for selecting IDCs. Professionals were interviewed to discuss IDCs. These interviews centered on the key problems and decision-making agents that affect IDC utilization and the duty of stakeholders in developing its implementation for local building construction. Ten specialists who affect the selection of building materials were interviewed.
Even though the local building sector has minimal experience utilizing IDCs the study picked ten accessible specialists from diverse parts of the local building industry. The experts were picked for their significant involvement in relevant IDC topics. Their experience ranged from university professors to construction executives, governmental politicians, manufacturing CEOs, and a supply department head. The experts were familiarized with the study’s goals and methods of the suggested multicriteria decision-making (MCDM) system () and the listing of defined criteria of relevant work before the interview ().
The primary interviews with each specialist took an average of 45 minutes. They next completed the questionnaire to categorize the linked decision aspects according to the 40 proposed decision criteria gathered from the related surveys. Considering that the interviews were explanatory/expressive, the answers were analyzed for discussion evaluation by arrangement, interaction, and clarifying information. The questions were categorized and coded. Ten experts rated the value of the influencing decision agents mentioned in based on their relationship to IDC application in the local building sector. Among the forty specified decision elements, professionals identified thirty as key influencing factors for employing IDC in local building construction. shows the experts’ chosen thirty choice agents. As a result, the suggested multicriteria decision-making assistant system is evaluated as a tool and relevant decision-making elements are identified. As a consequence of expert interviews and questionnaire research, shows the proposed decision agents as primary and sub-criteria.
Table 2. A summary of the important decision-making factors.
3.1.2. The multi-criteria decision-making model’s framework and elements
In order to build the model, the data generated in multiple modules must be logically linked together. These modules contain information to help with the assessment process. The multi-criteria decision-making assistance technique has interconnected parts. The extended model’s analytical framework is shown in .
3.1.3. The model for multi-criteria decision making
The basic MCDM model runs the IDC evaluation. Initially, experts were given a list of IDCs to choose from. The algorithm then consults experts to determine priority weight scores for the primary and sub-criteria decision aspects. Professionals then decide on the preferred weighting of the IDC variables. Each decision agent’s value is assigned to an IDC based on the precedence score. IDCs are given priority by the model. The expert evaluates each IDC-recommended model and proposes either the highest-scoring living system or an alternate technique in the “outcome” model. The next class of options is then evaluated. A sample IDC evaluation is also provided to demonstrate the MCDM method’s selection mechanism.
4. Using the AHP-MCDM model
The next stage is the evaluation and selection of alternative IDCs. Five Iranian IDCs were chosen among the most common industrialized building constructions to be investigated and evaluated as alternative systems. The most appropriate IDC was determined using AHP in the expanded MCDM model. Professionals with extensive experience in IDC usage and AHP assessment were asked to review the MCDM model to achieve the research’s principal purpose.
Raw material utilization, product input, manufacturing technique, shipment and implementation, component load and type sorting, and labor involvement in the producing and implementation procedures vary between dwelling systems. The variations in defining characteristics over these five IDCs, as seen in , made it possible to conduct an in-depth assessment, which both improved the validity of the research findings and provided concepts for conducting additional research. The attributes of the dwellings were the subject of a comprehensive field study analysis, which was carried out in order to collect vital information regarding these characteristics.
Table 3. Specifications of the five IDCs.
4.1. Justifications for the use of AHP
Earlier studies have established decision-assistant approaches and procedures. The choice hypothesis and its application analysis (Keeney, Raiffa, and Meyer Citation1993), ranking by surrogate weights (Kunsch and Ishizaka Citation2019), weighted summation model (Marler and Arora Citation2010), and the Theorem of multiple-attribute value (Von Winterfeldt and Edwards Citation1993; Dyer Citation2005) are a few examples. The AHP aided experts’ judgments in this study as an assessment tool. The AHP uses quantitative and qualitative methods to identify and prioritize essential success factors (Armacost et al. Citation1994; Banihashemi et al. Citation2017). AHP organizes a multi-dimensional complication into components with aims, entities, sub-agents, and alternatives to the qualitative method (Zanakis et al. Citation1998). The AHP uses pairwise comparison as a quantitative technique to rank the agents in order of importance. The consistency ratio must be less than 0.1 to be considered dependable. Using the technique to assess the consistency helps provide correct priority measures based on decision agents and alternatives to housing (Saaty Citation2008). This research uses AHP as it is mostly a simple conceptual approach to real-world situations (Saaty Citation2003). The work also uses a standard formula to determine an individual’s or group’s inevitable personal priorities (Saaty Citation2000). The AHP significance level obtained in the MCDM model under the uncertainty problem meets an additive value function (Lin, Wang, and W-D Citation2008). AHP elicitations are used to measure multi-attribute value (Dyer Citation1990). Also, normative concerns are range-sensitive in attribute weight (Yeh Citation2002). AHP selection and evaluation is depicted in .
4.2. The deciding hierarchy’s problem modeling
The standard AHP enables quick, straightforward, and classified problem segmentation (Sangiorgio, Uva, and Fatiguso Citation2018). shows the problem rating expression, primary and sub-criteria determination, and various possibilities to meet the primary aims. The primary purpose is to choose the IDC that is best suited for application in the mass housing development being carried out in the city of Tehran. After that, a group of possible architectural frameworks for dwellings that were accessible for selection was arranged (). The approaches were selected on the basis of the knowledge-based regulations and their widespread application in project cases in Iran. Among the most prevalent industrialized building structures in Iran, five IDCs were selected for investigation and evaluation and constructing the MCDM assistant system as alternative solutions. The unique characteristics of these five IDCs enabled a comprehensive examination, which enhanced the validity of the study’s findings and gave lessons for future research. The housing systems that are going to be evaluated are going to be chosen from the realistic case studies that can be found in the present research. In addition, the aspects of the suitable IDCs that have been identified are outlined in this article in order to promote the efficient application of IDC for the development of cost – effective accommodation.
Figure 4. AHP structure and ranking.
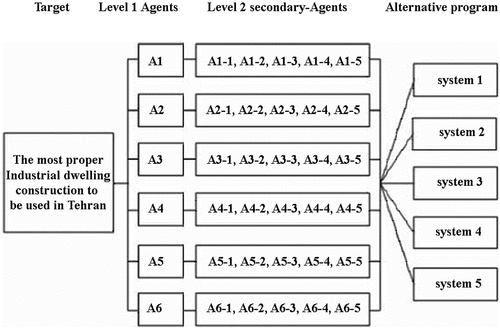
4.3. Weight assessment of AHP
Assessment of intended decision agent value and choice weights follows the structural decision hierarchy arrangement. According to n arranged agents of comparison (for example, primary agents, secondary decision agents, or options concerning the primary and secondary decision agents), an (n × n) decision Matrix B is determined, Where the peak diagonal element > 0 is generated via the Saaty ratio scales comparison of the ith element with jth (See ). The demonstration for the semantic coefficient scale is in , which contains numeric amounts (Jianzhong Citation2002; Burt, Aron, and Lee Citation2001; MR Abdullah and C Egbu Citation2010; Kokaraki et al. Citation2019; Edwards Citation1954) then comes to terms (Keivani and Werna Citation2001; Pan, Gibb, and Dainty Citation2007; Blismas, Pasquire, and Gibb Citation2006; Wong and Li Citation2008) among these amounts. The reciprocating values of the upper moiety complete the lower moiety of Matrix B; via achieving reciprocating parts, Matrix B is as below:
Table 4. The absolute number of Saaty’s substantial scale.
Moreover, Matrix B is called reliable if: besides, its principal eigenvalue is
. Solving the following eigenvector equation provides the weights:
while is a particular eigenvector regarding the principal eigenvalue
. If slight changes are represented, then it accepts
For one of the EOC objectives, the right-side normalized main eigenvector is computed as follows:
where S is SumTotal; n stands for the objectives integer; is the ratio result for the option of the ith target, and
is the global priority grade of the ith target.
Eventually, the approach is validated by doing a sensitivity evaluation to assess the solution’s stability.
4.4. Decision agents’ weight assessment
The priority weight of primary and secondary decision agents is determined by pairwise comparisons of their respective importance rates. The AHP survey included ten experts with AHP knowledge and IDC program experience. Here’s how experts are chosen: Primarily, the experts’ associations provided a list of available experts. Outstanding professionals in each group also offered suggestions. On the basis of their recommendations, candidates with more IDC experience were chosen. Finally, a balanced view was achieved by selecting a similar number of participants from each group.
With the EOC, you can quickly conduct questionnaires and make assessments online. The EOC spontaneously supports the respondents in reviewing their best decisions’ consistency ratio and weight. The consistency ratio should be smaller than 0.1 with this system. In order to determine the decision factors’ relative importance, each pair of comparison matrices was treated as an equivalent eigenvalue problem (note ).
Table 5. Universal precedence and local precedence of decision agents.
4.5. Alternative IDCs’ preferential weight assessment
The next stage was to obtain the overall normalized score and values for each dwelling system. This was followed by the weight value of each main and minor agent. According to AHP, a pairwise comparison was also performed by some selected experienced specialists from the Tehran building construction. The dearth of experienced professionals in IDC is due to the absence of practice in developing and performing industrial construction methods for local building construction. To address the constraints, an ordered questionnaire and redone field tests on IDC reliability options bound by visiting and conversing with the same people in multiple cases were utilized. Also, alternative IDCs were documented.
Thirty experts were chosen using the methods described in Section 4.4. Architects, executive directors, chairmen of production bureaus of prefab manufacturers, bosses of construction departments of producer companies, and professors of civil engineering, construction, and architecture departments of universities were major contributors. The specialists had 18 years of expertise on average. To ensure a balanced analysis, each category had an equal number of participants. Participants as individuals were also selected based on the suggestion of each group’s expert leader, as well as their IDC skills and expertise. The Saaty Ratio Scale assigned to each secondary agent determined the relevance of five IDC options (). Finally, IDC’s five normalized favorite importance levels were rated. Then the top two IDCs were chosen for Tehran’s home expansions.
5. Results and discussions
The study identifies a gap in the creation of an effective decision-making model for accommodation in developing and underdeveloped countries, notably in the Middle East. The data show that the MCDM framework can be used to assess the decision variables and alternative IDCs that affect the selection of the most appropriate IDCS for residential development. The decision-making method utilized in this research enables clear and specific and understandable verification of the derived values of decision agents and acceptable solution IDCs based on the local background. Furthermore, the use of theoretical pluralism enhances understanding of the fundamental assessment in decision making.
5.1. Substantial decision agents
As shown in , the total scores on every main component indicate that the top three elements are the most influential in Tehran when it comes to dwelling system selection. Surroundings and Environment, Sub-structure, and Civic-political issues, on the other hand, are determined to be less significant. The results also indicated that the most important elements to affect the selection of IDC’s application include factors such as affordability, new investment methods, raw material and substances, vulnerability conditions and the availability of trained labors. The following is a discussion of the top three main decision-making agents.
5.1.1. User requirements
Customer needs are contingent upon the construction industry’s capacity to meet the challenge of providing adequate homes at a reasonable price. According to , under user requirements, Reasonable price and Standards and quality, are the most essential criteria influencing the decision to pick a suitable IDC. IDCs technology advancements in Iran are also geared toward resolving challenges of price and quality. Making good housing more widely available is a difficult challenge in Iran. The lack of housing is due to the excessive concentration of the population in big cities, especially the capital of the country, Tehran. And also, the country’s economic policy has made housing a commercial commodity, and there are even a large number of empty apartments in Tehran. Another obstacle to promoting the industrialization of construction and housing in Iran is the risk of increasing the unemployment of many workers who work in the traditional housing construction industry.
In Tehran, this problem is more serious than in other cities, where slum dwellers are steadily increasing both outside and inside the capital. It is largely due to a lack of suitable materials for quality affordable housing. So, implementing affordable IDC initiatives helps establish and accelerate high-quality housing construction.
5.1.2. Supply chain
Another critical component to address when selecting the proper housing system for effective dwelling development is the supply chain. Within the supply chain, new investment methods and Production capacity are critical sub-factors. The government’s efforts to expand manufacturing capacity and build houses are dependent on imported resources. This issue exacerbates the difficulty of implementing IDCs on a wide scale in home construction in Iran.
5.1.3. Building industry
The building industry is shown in as the third significant decision agent. Many sub-agents in the construction sector support this status. The two most important factors in the building construction sector are the number of skilled workers and the ability of the dwelling system to produce fast and easy buildings. These agents have a substantial impact on increasing building output and productivity. Typical of Tehran’s construction industry is the use of conventional methods. Workers from rural areas and neighboring countries make up the majority of the construction crews, and many of them lack formal training. This has had a negative impact on the housing systems’ production and advancement. A significant amount of focus should be placed on increasing the entire capacity of the local building industry in order to accomplish rapid dwelling development.
5.2. The five IDC options prioritization
show that of the five IDC alternatives, precast reinforced concrete slab, beam, and column blocks PRCSBCB and light steel frame wall panels LSFWP are the most suitable for use in Tehran. These practical solutions connect to the local environment’s economic and civic gains. reveals that the PRCSBCB and LSFWP outperform other IDC solutions in all major decision agents. These housing solutions are lightweight, easy to assemble, and cost-effective for mass production. Compared to the typical concrete beam and slab method, PRCSBCB reduces expenses by 40% and material waste by 30% (Lizarralde Citation2014; Noorzai, Gharouni Jafari, and Moslemi Naeni Citation2020). LSFWP halves the cost of standard hollow concrete walls while having higher physical and mechanical attributes. Because these panels eliminate the need for cement plastering, less water is used during construction. PRCSBCB and LSFWP are semi-IDC groups that require low-to-medium funding. They necessitate only rudimentary skills, rely on locally sourced materials, and employ small to medium-sized machines and tools. These mass-produced components follow a modular pattern, size, and shape. In order to suit the needs of a varied spectrum of end users with varying budgetary situations, prefabricated construction components are an ideal and cost-effective choice. These IDCs are flexible systems compatible with various conventional and newer approaches. As shown in )–(c) a high score of substantial decision agents distinguishes PRCSBCB and LSFWP from other advanced systems (see ). However, these systems are constrained by material shortages, component fragility in transit, joint fractures, and usage rigidity.
Figure 7. (a) Priority range for IDC options according to user requirements (b). Priority range for IDC options according to supply chain (3). Priority range for IDC options according to the building industry.
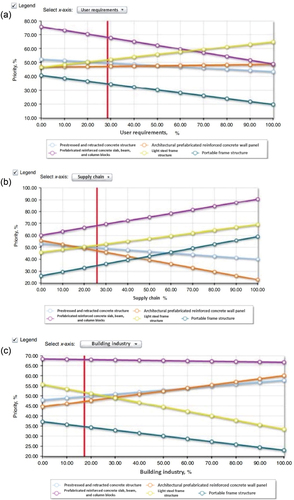
Table 6. The normalized priority scale for IDC options.
Table 7. Options rankings for the dwelling systems.
The PRCSBCB’s maximum span is five meters, restricting its potential to create huge expanses. Building multi-story buildings is equally difficult for PRCSBCB. Joint fractures that require prompt and continuous rehabilitation are common. LSFWP has the same fracture problem unless the joints are completely sealed after construction. Since it collects water from the environment, it requires a special treatment in humid places. Inadequate supply chains and production volume are among the problems that prevent installation on a huge scale of useful residential systems.
5.3. In brief, the features of suitable IDCs
In , the advantages and disadvantages of the two most significant IDCs for implementation in Tehran are outlined. In addition, displays the fundamental values, which include structures, architectural details, industrialization procedures, shipping and installation issues, as well as political and social perspectives. In determining whether residential systems are suitable for use in developing nations like Iran, these characteristics are also crucial factors.
Table 8. The top two selected industrial dwelling systems comparison review.
Table 9. The most proper applicable dwelling system’s general specifications.
6. Conclusion
This study examines the AHP decision-making process to help Iran’s IDC select large-scale affordable housing expansions. The investigation indicates the absence of a decision-making assistant system in Iran. Thirty decision agents are identified in the literature analysis under six key decision agents: the building industry, user requirements, supply chain, sub-structures, surrounds, and increasingly important civic-political agents.
The MCDM assistance system model alternatives chosen were the five existing IDCs. Using AHP, housing experts and developers can select appropriate IDCs and compare possibilities among decision agents. The expert’s judgment was tested using EOC trading software. The most important decision agents are users’ needs, the supplier chain, and the construction industry. The best programs for home expansions are PRCSBCB and LSFWP. The study’s findings acknowledge the model’s viability and usefulness. The proposed method for developing the MCDM prototype demonstrated that the Decision-Making Assistant System DMAS could be efficiently used to measure the significance of materials. There were few IDC possibilities and limited IDC installation practice in large-scale housing developments. These obstacles hampered information collection. All accessible building construction professionals with IDC capabilities were asked to participate. Alternative IDCs from the marketplace are picked for assessment. Additionally, the analytical evaluation system utilized to identify key decision agents and typical requirements for affordable house building is intended to benefit home builders and policymakers in developing nations.
6.1. Possible future research areas
The model constituent elements can be designed or modified to suit the needs of each specific country. Although this research summarizes a subset of IDC options for dwelling expansion, the study’s technique can be authorized in different contexts to prove its validity.
Data availability
Some or all data, models, or codes that support the findings of this study are available from the corresponding author upon reasonable request.
Nomenclature
IDC | = | Industrial dwelling construction |
DMAS | = | decision-making assistant system |
AHP | = | Analytical Hierarchy Process |
PRCSBCB | = | Prefabricated Reinforced Concrete Slab, Beam, and Column blocks |
LSFWP | = | Light Steel Frame Wall Panels |
EOC | = | Expert Option Comparison |
MCDM | = | Multi-Criteria Decision Making |
GNP | = | Gross National Income |
CEO | = | Chief Executive Officer |
PRCS | = | Prestressed and retracted Concrete structures |
PFS | = | Portable frame structure |
APRCWP | = | Architectural prefabricated reinforced concrete wall panel |
Acknowledgments
This work is supported by: 高品质色建筑设计方法与智慧协同平台,项目编号:2022YFC3803800 High-quality green building design methods and intelligent collaboration platform,NO.2022YFC3803800, Architects & Engineers Co., Ltd. of Southeast University, Sipailou Road, Nanjing 210096, China.
Disclosure statement
No potential conflict of interest was reported by the author(s).
Additional information
Funding
References
- Abdullah, M., and C. Egbu, editors. 2010. “The Role of Knowledge Management in Improving the Adoption and Implementation Practices of Industrialised Building System (IBS) in Malaysia.” CIB World Conference 2010, Manchester, UK, School of Built Environment, The University of Salford.
- Abdullah, M. R., and C. Egbu, editors. 2010. “Selection Criteria Frameword for Choosing Industrialized Building Systems for Housing Projects.” Procs 26th Annual ARCOM Conference, Manchester, UK, Association of Researchers in Construction Management.
- Abey, S. T., and K. Anand. 2019. “Embodied Energy Comparison of Prefabricated and Conventional Building Construction.” Journal of the Institution of Engineers (India): Series A 100 (4): 777–790. doi:10.1007/s40030-019-00394-8.
- Afzali, N., and S. Hamzehloo. 2018. “Evaluating the Role of Recycling Materials in Construction Industry (Case Study: City of Tehran).” Journal of Environmental Friendly Materials 2 (2): 49–58.
- Akhnoukh, A. K., and H. Elia. 2019. “Developing High Performance Concrete for precast/prestressed Concrete Industry.” Case Studies in Construction Materials 11: e00290. doi:10.1016/j.cscm.2019.e00290.
- Akmam Syed Zakaria, S., T. Gajendran, T. Rose, and G. Brewer. 2018. “Contextual, Structural and Behavioural Factors Influencing the Adoption of Industrialised Building Systems: A Review.” Architectural Engineering and Design Management 14 (1–2): 3–26. doi:10.1080/17452007.2017.1291410.
- Arif, M., and C. Egbu. 2010. “Making a Case for Offsite Construction in China.” Engineering, Construction and Architectural Management 17 (6): 536–548. doi:10.1108/09699981011090170.
- Armacost, R. L., P. J. Componation, M. A. Mullens, and W. W. Swart. 1994. “An AHP Framework for Prioritizing Customer Requirements in QFD: An Industrialized Housing Application.” IIE transactions 26 (4): 72–79. doi:10.1080/07408179408966620.
- Attia, S. 2018. “Net Zero Energy Buildings (Nzeb). Concepts Frameworks and Roadmap for Project Analysis and 366 Implementation.”
- Azman, M. N. A., M. S. S. Ahamad, T. A. Majid, A. S. Yahaya, and M. H. Hanafi. 2013. “Statistical Evaluation of pre-selection Criteria for Industrialized Building System (IBS).” Journal of Civil Engineering and Management 19 (Supplement_1): S131–S40. doi:10.3846/13923730.2013.801921.
- Baghchesaraei, A., M. V. Kaptan, and O. R. Baghchesaraei. 2015. “Using Prefabrication Systems in Building Construction.” International Journal of Applied Engineering Research 10 (24): 44258–44262.
- Banai-Kashani, A. 1986. “Building Systems Innovations in Urban Housing Production: A Planning Application of the Analytic Hierarchy Process.” Architectural Science Review 29 (4): 99–109. doi:10.1080/00038628.1986.9696599.
- Banihashemi, S., M. R. Hosseini, H. Golizadeh, and S. Sankaran. 2017. “Critical Success Factors (Csfs) for Integration of Sustainability into Construction Project Management Practices in Developing Countries.” International Journal of Project Management 35 (6): 1103–1119. doi:10.1016/j.ijproman.2017.01.014.
- Bardhan, S. 2011. “Assessment of Water Resource Consumption in Building Construction in India.” WIT Trans Ecol Environ 144: 93–101.
- Bari, N. A. A., R. Yusuff, N. Ismail, A. Jaapar, and R. Ahmad. 2012. “Factors Influencing the Construction Cost of Industrialised Building System (IBS) Projects.” Procedia-Social and Behavioral Sciences 35: 689–696. doi:10.1016/j.sbspro.2012.02.138.
- Barlow, J., and D. Gann. 1995. “Flexible Planning and Flexible Buildings: Reusing Redundant Office Space.” Journal of Urban Affairs 17 (3): 263–276. doi:10.1111/j.1467-9906.1995.tb00347.x.
- Benton, W., and L. F. McHenry. 2010. Construction Purchasing & Supply Chain Management. New York , USA: McGraw-Hill New York.
- Berge, B. 2007. Ecology of Building Materials. London, UK: Routledge doi:10.4324/9780080504988.
- Bildsten, L. 2014. “Buyer-supplier Relationships in Industrialized Building.” Construction Management and Economics 32 (1–2): 146–159. doi:10.1080/01446193.2013.812228.
- Blismas, N., C. Pasquire, and A. Gibb. 2006. “Benefit Evaluation for Off‐site Production in Construction.” Construction Management and Economics 24 (2): 121–130. doi:10.1080/01446190500184444.
- Bortolini, R., C. T. Formoso, and D. D. Viana. 2019. “Site Logistics Planning and Control for engineer-to-order Prefabricated Building Systems Using BIM 4D Modeling.” Automation in Construction 98: 248–264. doi:10.1016/j.autcon.2018.11.031.
- Brandenburg, S. G., C. T. Haas, and K. Byrom. 2006. “Strategic Management of Human Resources in Construction.” Journal of Management in Engineering 22 (2): 89–96. doi:10.1061/(ASCE)0742-597X(2006)22:2(89).
- Buchman, A. 2020. “Housing Solutions for a Mass Population of Immigrants: The Israeli Experience.“ In Ethnicity and Housing, edited by W. Boal Frederick, 1st ed, 152–160. London, UK: Routledge.
- Burt, M. R., L. Y. Aron, and E. Lee. 2001. Helping America’s Homeless: Emergency Shelter or Affordable Housing?. Washington, DC, USA: Urban Insitute press.
- Buswell, R. A., R. C. Soar, A. G. Gibb, and A. Thorpe. 2007. “Freeform Construction: Mega-scale Rapid Manufacturing for Construction.” Automation in Construction 16 (2): 224–231. doi:10.1016/j.autcon.2006.05.002.
- Cachon, G. P., and M. Fisher. 2000. “Supply Chain Inventory Management and the Value of Shared Information.” Management Science 46 (8): 1032–1048. doi:10.1287/mnsc.46.8.1032.12029.
- Celadyn, W. 2014. “Durability of Buildings and Sustainable Architecture.” Technical Transactions/Czasopismo Techniczne, no. 14.
- Chankong, V., and Y. Y. Haimes. 2008. Multiobjective Decision Making: Theory and Methodology: Courier. Mineola, New York, USA: Dover Publications.
- Chen, Y., G. E. Okudan, and D. R. Riley. 2010. “Sustainable Performance Criteria for Construction Method Selection in Concrete Buildings.” Automation in Construction 19 (2): 235–244. doi:10.1016/j.autcon.2009.10.004.
- Ching, F. D. 2020. Building Construction Illustrated. Hoboken, New Jersey, USA: John Wiley & Sons.
- Cole, R. J. 2000. “Building Environmental Assessment Methods: Assessing Construction Practices.” Construction Management & Economics 18 (8): 949–957. doi:10.1080/014461900446902.
- Dainty, A. 2008. “Methodological Pluralism in Construction Management Research.” Advanced Research Methods in the Built Environment 1: 1–13.
- Detsimas, N., V. Coffey, Z. Sadiqi, and M. Li. 2016. “Workplace Training and Generic and Technical Skill Development in the Australian Construction Industry.” Journal of Management Development 35 (4): 486–504. doi:10.1108/JMD-05-2015-0073.
- Dyer, J. S. 1990. “Remarks on the Analytic Hierarchy Process.” Management Science 36 (3): 249–258. doi:10.1287/mnsc.36.3.249.
- Dyer, J. S. 2005. “MAUT—multiattribute Utility Theory.” In Multiple Criteria Decision Analysis: State of the Art Surveys, 265–292. New York, USA: Springer.
- Ebadolazadehmaleki, B., Z. S. S. Zarabadi, S. Piri, and M. Farzad Behtash. 2021. “Interpretive Structural Model of the Resilience Threshold of Urban Spaces against Earthquakes with a Socio-Ecological Approach, Case Study: Zanjan City.” Journal of Urban Ecology Researches 12 (24): 101–118.
- Ebrahimzadeh, F., F. Mehdizadeh Saradj, S. Norouzian-Maleki, and S. Piri. 2021. “Mapping of Co-occurrence of Scientific Products in the Field of Children’s Physical Activity in the Open Space of Primary Schools.” Motor Behavior 13 (46): 201–230.
- Edwards, W. 1954. “The Theory of Decision Making.” Psychological Bulletin 51 (4): 380. doi:10.1037/h0053870.
- Engström, S., and E. Hedgren. 2012. “Sustaining Inertia? Construction Clients’ Decision‐making and Information‐processing Approach to Industrialized Building Innovations.” Construction Innovation 12 (4): 393–413. doi:10.1108/14714171211272180.
- Faludi, J., M. D. Lepech, and G. Loisos. 2012. “Using Life Cycle Assessment Methods to Guide Architectural decision-making for Sustainable Prefabricated Modular Buildings.” Journal of Green Building 7 (3): 151–170. doi:10.3992/jgb.7.3.151.
- Forbes, L. H., and S. M. Ahmed. 2010. Modern Construction: Lean Project Delivery and Integrated Practices. Philadelphia, USA: CRC press.
- Freedman, S. 1999. “Loadbearing Architectural Precast Concrete Wall Panels.” PCI Journal 44 (5): 92–115. doi:10.15554/pcij.09011999.92.115.
- Girmscheid, G, editor. 2005. “Industrialization in Building Construction: Production Technology or Management Concept? Understanding the Construction Business and Companies in the New Millennium.” Proceedings of the 11th Joint Cib International Symposium: Combining Forces-Advancing Facilities Management and Construction through Innovation, 2005 June 13–16, Helsinki, Finland, Vol. 1, 427–441. Espoo, Finland: VTT Technical Research Centre of Finland and RILUniversity of West Indies.
- Groat, L. N., and D. Wang. 2013. Architectural Research Methods. 2nd ed. Hoboken, New Jersey, USA: John Wiley & Sons.
- Gurung, N., and M. Mahendran. 2002. “Comparative Life Cycle Costs for New Steel Portal Frame Building Systems.” Building Research & Information 30 (1): 35–46. doi:10.1080/09613210110054999.
- Hamza, S. A., S. Rasheed, and A. Hussein. 2022. “Procurement Challenges Analysis of Iraqi Construction Projects.” Journal of the Mechanical Behavior of Materials 31 (1): 112–117. doi:10.1515/jmbm-2022-0012.
- Harris, D. J. 1999. “A Quantitative Approach to the Assessment of the Environmental Impact of Building Materials.” Building and Environment 34 (6): 751–758. doi:10.1016/S0360-1323(98)00058-4.
- Harris, F., R. McCaffer, A. Baldwin, and F. Edum-Fotwe. 2021. Modern Construction Management. 8th ed. Hoboken, New Jersey, USA: John Wiley & Sons.
- Hedgren, E., and L. Stehn. 2014. “The Impact of Clients’ decision-making on Their Adoption of Industrialized Building.” Construction Management and Economics 32 (1–2): 126–145. doi:10.1080/01446193.2013.833340.
- Higgins-D’Alessandro, A. 2010. “The Transdisciplinary Nature of Citizenship and civic/political Engagement Evaluation.” Handbook of Research on Civic Engagement in Youth 559–592.
- Hitt, M. A., B. W. Keats, and S. M. DeMarie. 1998. “Navigating in the New Competitive Landscape: Building Strategic Flexibility and Competitive Advantage in the 21st Century.” Academy of Management Perspectives 12 (4): 22–42. doi:10.5465/ame.1998.1333922.
- Horvath, A. 2004. “Construction Materials and the Environment.” Annual Review of Environment and Resources 29 (1): 2. doi:10.1146/annurev.energy.29.062403.102215.
- Hussain, S., W. Xuetong, and T. Hussain. 2020. “Impact of Skilled and Unskilled Labor on Project Performance Using Structural Equation Modeling Approach.” SAGE Open 10 (1): 2158244020914590. doi:10.1177/2158244020914590.
- Iranpour, A., H. Ebrahimpour, and R. Rahgozar. 2019. “Evaluation of bond-slip Behavior in Precast Reinforced Concrete beam-to-column Connection Using Finite Element Modeling.” Journal of Structural and Construction Engineering 6 (3): 159–174.
- Jaillon, L., C.-S. Poon, and Y. H. Chiang. 2009. “Quantifying the Waste Reduction Potential of Using Prefabrication in Building Construction in Hong Kong.” Waste Management 29 (1): 309–320. doi:10.1016/j.wasman.2008.02.015.
- Jato-Espino, D., E. Castillo-Lopez, J. Rodriguez-Hernandez, and J. C. Canteras-Jordana. 2014. “A Review of Application of multi-criteria Decision Making Methods in Construction.” Automation in Construction 45: 151–162. doi:10.1016/j.autcon.2014.05.013.
- Jianzhong, S. 2002. “Present State and Prospects of China Housing Industry Development.” Housing Science 11.
- Joshi, S. M. 2008. “The Sick Building Syndrome.” Indian Journal of Occupational and Environmental Medicine 12 (2): 61. doi:10.4103/0019-5278.43262.
- Kanda, A., and S. Deshmukh. 2008. “Supply Chain Coordination: Perspectives, Empirical Studies and Research Directions.” International Journal of Production Economics 115 (2): 316–335. doi:10.1016/j.ijpe.2008.05.011.
- Karamoozian, A., M. Karamoozian, and H. Ashrafi. 2013. “Effect of Nano Particles on Self Compacting Concrete: An Experimental Study.” Life Science Journal 10 (2): 95–101.
- Karamoozian, A., D. Wu, C. P. Chen, and C. Luo. 2019. “An Approach for Risk Prioritization in Construction Projects Using Analytic Network Process and Decision Making Trial and Evaluation Laboratory.” IEEE Access 7: 159842–159854. doi:10.1109/ACCESS.2019.2939067.
- Karimi, H., T. R. Taylor, G. B. Dadi, P. M. Goodrum, and C. Srinivasan. 2018. “Impact of Skilled Labor Availability on Construction Project Cost Performance.” Journal of Construction Engineering and Management 144 (7): 04018057.
- Keeney, R. L., H. Raiffa, and R. F. Meyer. 1993. Decisions with Multiple Objectives: Preferences and Value trade-offs. Cambridge, United Kingdom: Cambridge university press.
- Keivani, R., and E. Werna. 2001. “Modes of Housing Provision in Developing Countries.” Progress in Planning 55 (2): 65–118. doi:10.1016/S0305-9006(00)00022-2.
- Kellenberger, D., and H.-J. Althaus. 2009. “Relevance of Simplifications in LCA of Building Components.” Building and Environment 44 (4): 818–825. doi:10.1016/j.buildenv.2008.06.002.
- Kokaraki, N., C. J. Hopfe, E. Robinson, and E. Nikolaidou. 2019. “Testing the Reliability of Deterministic multi-criteria decision-making Methods Using Building Performance Simulation.” Renewable and Sustainable Energy Reviews 112: 991–1007. doi:10.1016/j.rser.2019.06.018.
- Koskela, L, editor. 1999. “Management of Production in Construction: A Theoretical View.” Proceedings of the 7th Annual Conference of the International Group for Lean Construction, Berkeley, California, USA.
- Kunsch, P. L., and A. Ishizaka. 2019. “A Note on Using Centroid Weights in Additive multi-criteria Decision Analysis.” European Journal of Operational Research 277 (1): 391–393. doi:10.1016/j.ejor.2019.02.021.
- Landolfo, R. 2019. “Lightweight Steel Framed Systems in Seismic Areas: Current Achievements and Future Challenges.” Thin-Walled Structures 140: 114–131. doi:10.1016/j.tws.2019.03.039.
- Lawson, R., and R. Ogden. 2008. “‘Hybrid’light Steel Panel and Modular Systems.” Thin-Walled Structures 46 (7–9): 720–730. doi:10.1016/j.tws.2008.01.042.
- Lin, -C.-C., W.-C. Wang, and Y. W-D. 2008. “Improving AHP for Construction with an Adaptive AHP Approach (A3).” Automation in Construction 17 (2): 180–187. doi:10.1016/j.autcon.2007.03.004.
- Li, X., G. Q. Shen, P. Wu, and T. Yue. 2019. “Integrating Building Information Modeling and Prefabrication Housing Production.” Automation in Construction 100: 46–60. doi:10.1016/j.autcon.2018.12.024.
- Liu, J., Y. Liu, and X. Wang. 2020. “An Environmental Assessment Model of Construction and Demolition Waste Based on System Dynamics: A Case Study in Guangzhou.” Environmental Science and Pollution Research 27 (30): 37237–37259. doi:10.1007/s11356-019-07107-5.
- Lizarralde, G. 2014. The Invisible Houses: Rethinking and Designing low-cost Housing in Developing Countries. 1st ed, 250. New York, USA: Routledge.
- Loonen, R. C., M. Trčka, D. Cóstola, and J. L. Hensen. 2013. “Climate Adaptive Building Shells: State-of-the-art and Future Challenges.” Renewable and Sustainable Energy Reviews 25: 483–493. doi:10.1016/j.rser.2013.04.016.
- Luo, T., X. Xue, Y. Wang, W. Xue, and Y. Tan. 2020. “A Systematic Overview of Prefabricated Construction Policies in China.” Journal of Cleaner Production 124371.
- Lützkendorf, T., and D. Lorenz. 2005. “Sustainable Property Investment: Valuing Sustainable Buildings through Property Performance Assessment.” Building Research & Information 33 (3): 212–234. doi:10.1080/09613210500070359.
- L-z, L., C. Mao, S. L-y, and L. Z-d. 2015. “Risk Factors Affecting Practitioners’ Attitudes toward the Implementation of an Industrialized Building System: A Case Study from China.” Engineering, Construction and Architectural Management 22 (6): 622–643. doi:10.1108/ECAM-04-2014-0048.
- Madanipour, A. 2006. “Urban Planning and Development in Tehran.” Cities 23 (6): 433–438. doi:10.1016/j.cities.2006.08.002.
- Maes, R. 2013. Physically Unclonable Functions: Constructions, Properties and Applications. Berlin, Heidelberg, New York, Dorderecht, London: Springer Science & Business Media. doi:10.1007/978-3-642-41395-7.
- Mahendran, M., and C. Moor. 1999. “Three-dimensional Modeling of Steel Portal Frame Buildings.” Journal of Structural Engineering 125 (8): 870–878. doi:10.1061/(ASCE)0733-9445(1999)125:8(870).
- Marler, R. T., and J. S. Arora. 2010. “The Weighted Sum Method for multi-objective Optimization: New Insights.” Structural and Multidisciplinary Optimization 41 (6): 853–862. doi:10.1007/s00158-009-0460-7.
- Mashayekhi, A. 2019. “The 1968 Tehran Master Plan and the Politics of Planning Development in Iran (1945–1979).” Planning Perspectives 34 (5): 849–876. doi:10.1080/02665433.2018.1468805.
- Medineckiene, M., E. Zavadskas, F. Björk, and Z. Turskis. 2015. “Multi-criteria decision-making System for Sustainable Building assessment/certification.” Archives of Civil and Mechanical Engineering 15 (1): 11–18. doi:10.1016/j.acme.2014.09.001.
- Meloy, G. 2016. “Architectural Precast Concrete Wall Panels: Their Technological Evolution, Significance, and Preservation.”
- Mohammed, H. A. 2018. “Variety of Precast Concrete.” Presentation presented to Al-Furat Al-Awsat Technical University-Technical Collage of Al-Mussaib-Session Presentation, Iraq.
- Nassar, K., W. Thabet, and Y. Beliveau. 2003. “A Procedure for multi-criteria Selection of Building Assemblies.” Automation in Construction 12 (5): 543–560. doi:10.1016/S0926-5805(03)00007-4.
- Navaratnam, S., T. Ngo, T. Gunawardena, and D. Henderson. 2019. “Performance Review of Prefabricated Building Systems and Future Research in Australia.” Buildings 9 (2): 38. doi:10.3390/buildings9020038.
- Nejad, M. M., M. J. T. Amiri, M. Mirdoost, H. Darvishi, and M. J. Barforoosh. 2016. “Investigating and Comparing the Economic Use of Normal Concrete and Lightweight Concrete in Construction Projects in Iran.” Journal of Current Research in Science, no. 1: 560.
- Ngowi, A., E. Pienaar, A. Talukhaba, and J. Mbachu. 2005. “The Globalisation of the Construction industry—a Review.” Building and Environment 40 (1): 14–19. doi:10.1016/j.buildenv.2004.05.008.
- Noorzai, E., K. Gharouni Jafari, and L. Moslemi Naeni. 2020. “Lessons Learned on Selecting the Best Mass Housing Method Based on Performance Evaluation Criteria in Iran.” International Journal of Construction Education and Research 1–19.
- Omer, A. M. 2008. “Energy, Environment and Sustainable Development.” Renewable and Sustainable Energy Reviews 12 (9): 2265–2300. doi:10.1016/j.rser.2007.05.001.
- Oven, K. J., and J. D. Rigg. 2015. “The Best of Intentions?: Managing Disasters and Constructions of Risk and Vulnerability in Asia.” Asian Journal of Social Science 43 (6): 685–712. doi:10.1163/15685314-04306003.
- Pan, Y-h. 2007. “Application of Industrialized Housing System in Major Cities in China: A Case Study of Chongqing.”
- Pan, W., A. Dainty, and A. Gibb. 2012. “Establishing and Weighting Decision Criteria for Building System Selection in Housing Construction.”
- Pan, W., A. R. Dainty, and A. G. Gibb. 2012. “Establishing and Weighting Decision Criteria for Building System Selection in Housing Construction.” Journal of Construction Engineering and Management 138 (11): 1239–1250. doi:10.1061/(ASCE)CO.1943-7862.0000543.
- Pan, W., A. G. Gibb, and A. R. Dainty. 2007. “Perspectives of UK Housebuilders on the Use of Offsite Modern Methods of Construction.” Construction Management and Economics 25 (2): 183–194. doi:10.1080/01446190600827058.
- Pan, W., A. G. Gibb, and A. R. Dainty. 2008. “A Decision Support Matrix for Build System Selection in Housing Construction.” International Journal for Housing Science and Its Applications 32 (1): 61.
- Pan, Y., F. K. Wong, and E. C. Hui. 2011. “Application of Industrialized Housing System in China: A Chongqing Study.” In Modeling Risk Management in Sustainable Construction, 161–168. Berlin, Heidelberg, Germany: Springer. doi:10.1007/978-3-642-15243-6_19.
- Parastesh, H., I. Hajirasouliha, and R. Ramezani. 2014. “A New Ductile moment-resisting Connection for Precast Concrete Frames in Seismic Regions: An Experimental Investigation.” Engineering Structures 70: 144–157. doi:10.1016/j.engstruct.2014.04.001.
- Passer, A., J. Wall, H. Kreiner, P. Maydl, and K. Höfler. 2015. “Sustainable Buildings, Construction Products and Technologies: Linking Research and Construction Practice.” The International Journal of Life Cycle Assessment 20 (1): 1–8. doi:10.1007/s11367-014-0820-4.
- Patton, M. Q. 1990. Qualitative Evaluation and Research Methods. Thousand Oaks, California, USA: SAGE Publications.
- Phan, D. T., J. B. Lim, T. T. Tanyimboh, and W. Sha. 2012. “An Efficient Genetic Algorithm for the Design Optimization of cold-formed Steel Portal Frame Buildings.”
- Pishvaee, M. S., M. Rabbani, and S. A. Torabi. 2011. “A Robust Optimization Approach to closed-loop Supply Chain Network Design under Uncertainty.” Applied Mathematical Modelling 35 (2): 637–649. doi:10.1016/j.apm.2010.07.013.
- Pishvaee, M. S., and J. Razmi. 2012. “Environmental Supply Chain Network Design Using multi-objective Fuzzy Mathematical Programming.” Applied Mathematical Modelling 36 (8): 3433–3446. doi:10.1016/j.apm.2011.10.007.
- Pishvaee, M. S., and S. A. Torabi. 2010. “A Possibilistic Programming Approach for closed-loop Supply Chain Network Design under Uncertainty.” Fuzzy Sets and Systems 161 (20): 2668–2683. doi:10.1016/j.fss.2010.04.010.
- Polat, G. 2008. “Factors Affecting the Use of Precast Concrete Systems in the United States.” Journal of Construction Engineering and Management 134 (3): 169–178. doi:10.1061/(ASCE)0733-9364(2008)134:3(169).
- Pries, F., and F. Janszen. 1995. “*innovation in the Construction Industry: The Dominant Role of the Environment.” Construction Management and Economics 13 (1): 43–51. doi:10.1080/01446199500000006.
- Pulselli, R., E. Simoncini, R. Ridolfi, and S. Bastianoni. 2008. “Specific Emergy of Cement and Concrete: An energy-based Appraisal of Building Materials and Their Transport.” Ecological Indicators 8 (5): 647–656. doi:10.1016/j.ecolind.2007.10.001.
- Rashed, T., and J. Weeks. 2003. “Assessing Vulnerability to Earthquake Hazards through Spatial Multicriteria Analysis of Urban Areas.” International Journal of Geographical Information Science 17 (6): 547–576. doi:10.1080/1365881031000114071.
- Reisinger, J., P. Hollinsky, and I. Kovacic. 2021. “Design Guideline for Flexible Industrial Buildings Integrating Industry 4.0 Parameters.” Sustainability 13 (19): 10627. doi:10.3390/su131910627.
- RezaHoseini, A., S. Noori, and S. F. Ghannadpour. 2021. “Integrated Scheduling of Suppliers and multi-project Activities for Green Construction Supply Chains under Uncertainty.” Automation in Construction 122: 103485. doi:10.1016/j.autcon.2020.103485.
- Roque, E., and P. Santos. 2017. “The Effectiveness of Thermal Insulation in Lightweight steel-framed Walls with respect to Its Position.” Buildings 7 (1): 13. doi:10.3390/buildings7010013.
- Ruuska, A., and T. Häkkinen. 2014. “Material Efficiency of Building Construction.” Buildings 4 (3): 266–294. doi:10.3390/buildings4030266.
- Saaty, T. L. 1988. “What Is the Analytic Hierarchy Process?.“ In Mathematical Models for Decision Support, edited by G. Mitra, H. J. Greenberg, F. A. Lootsma, M. J. Rijkaert, and H. J. Zimmermann, 109–121. Berlin, Heidelberg, Germany: Springer-Verlag. doi:10.1007/978-3-642-83555-1.
- Saaty, T. L. 2000. Fundamentals of Decision Making and Priority Theory with the Analytic Hierarchy Process, Vol. 6. Pittsburgh, Pennsylvania, USA: RWS publications.
- Saaty, T. L. 2003. “Decision-making with the AHP: Why Is the Principal Eigenvector Necessary.” European Journal of Operational Research 145 (1): 85–91. doi:10.1016/S0377-2217(02)00227-8.
- Saaty, T. L. 2005. “Making and Validating Complex Decisions with the AHP/ANP.” Journal of Systems Science and Systems Engineering 14 (1): 1–36. doi:10.1007/s11518-006-0179-6.
- Saaty, T. L. 2008. “Decision Making with the Analytic Hierarchy Process.” International Journal of Services Sciences 1 (1): 83–98. doi:10.1504/IJSSCI.2008.017590.
- Sadeghi, M., M. Ghafory-Ashtiany, and N. Pakdel-Lahiji. 2015. “Developing Seismic Vulnerability Curves for Typical Iranian Buildings.” Proceedings of the Institution of Mechanical Engineers, Part O: Journal of Risk and Reliability 229 (6): 627–640.
- Saez, P. V., M. Del Río Merino, -A. S.-A. González, and C. Porras-Amores. 2013. “Best Practice Measures Assessment for Construction and Demolition Waste Management in Building Constructions.” Resources, Conservation and Recycling 75: 52–62. doi:10.1016/j.resconrec.2013.03.009.
- Sangiorgio, V., G. Uva, and F. Fatiguso. 2018. “Optimized AHP to Overcome Limits in Weight Calculation: Building Performance Application.” Journal of Construction Engineering and Management 144 (2): 04017101. doi:10.1061/(ASCE)CO.1943-7862.0001418.
- Santos, P., and L. S. da Silva. 2017. “Energy Efficiency of Lightweight steel-framed Buildings.” Energy Effic Build 35: 180–191.
- Saradj, F. M. 2007. “Earthquake Intensity, Damage, and Conservation of Unreinforced Masonry Buildings.” Architectural Science Review 50 (2): 130–140. doi:10.3763/asre.2007.5019.
- Schiller, G. 2007. “Urban Infrastructure: Challenges for Resource Efficiency in the Building Stock.” Building Research & Information 35 (4): 399–411. doi:10.1080/09613210701217171.
- Schriewer, J. 2004. “Constructions of Internationality. The Global Politics of Educational Borrowing and Lending.” 29.
- Seguirant, S. J., R. Brice, and B. Khaleghi. 2005. “Flexural Strength of Reinforced and Prestressed Concrete T-beams.” PCI Journal 50 (1): 44–73. doi:10.15554/pcij.01012005.44.73.
- Shahpari, M., F. M. Saradj, M. S. Pishvaee, and S. Piri. 2020. “Assessing the Productivity of Prefabricated and in-situ Construction Systems Using Hybrid multi-criteria Decision Making Method.” Journal of Building Engineering 27: 100979. doi:10.1016/j.jobe.2019.100979.
- Sharma, A., A. Saxena, M. Sethi, and V. Shree. 2011. “Life Cycle Assessment of Buildings: A Review.” Renewable and Sustainable Energy Reviews 15 (1): 871–875. doi:10.1016/j.rser.2010.09.008.
- Soares, N., P. Santos, H. Gervásio, J. Costa, and L. S. Da Silva. 2017. “Energy Efficiency and Thermal Performance of Lightweight steel-framed (LSF) Construction: A Review.” Renewable and Sustainable Energy Reviews 78: 194–209. doi:10.1016/j.rser.2017.04.066.
- Solís-Guzmán, J., M. Marrero, M. V. Montes-Delgado, and A. Ramírez-de-arellano. 2009. “A Spanish Model for Quantification and Management of Construction Waste.” Waste Management 29 (9): 2542–2548. doi:10.1016/j.wasman.2009.05.009.
- Song, J., W. R. Fagerlund, C. T. Haas, C. B. Tatum, and J. A. Vanegas. 2005. “Considering Prework on Industrial Projects.” Journal of Construction Engineering and Management 131 (6): 723–733. doi:10.1061/(ASCE)0733-9364(2005)131:6(723).
- Steiger, T. L. 1993. “Construction Skill and Skill Construction.” Work, Employment and Society 7 (4): 535–560. doi:10.1177/095001709374002.
- Vatan, M. 2017. “Evolution of Construction Systems: Cultural Effects on Traditional Structures and Their Reflection on Modern Building Construction.” Cultural Influences on Architecture: IGI Global 35–57.
- Von Winterfeldt, D., and W. Edwards. 1993. “Decision Analysis and Behavioral Research.”
- Vrijhoef, R., and L. Koskela. 2000. “The Four Roles of Supply Chain Management in Construction.” European Journal of Purchasing & Supply Management 6 (3–4): 169–178. doi:10.1016/S0969-7012(00)00013-7.
- Vyas, V. S. 2015. “Survey of Precast Concrete Method and Cast-in-Situ Concrete Method.” Int J Eng Tech Res 3 (11): 70–73.
- Wang, Q., Y. Deng, G. Li, C. Meng, L. Xie, M. Liu, L. Zeng, et al. 2020. “The Current Situation and Trends of Healthy Building Development in China.” Chinese Science Bulletin 65 (4): 246–255. doi:10.1360/TB-2019-0629.
- Wang, H., E. M. Marino, P. Pan, H. Liu, and X. Nie. 2018. “Experimental Study of a Novel Precast Prestressed Reinforced Concrete beam-to-column Joint.” Engineering Structures 156: 68–81. doi:10.1016/j.engstruct.2017.11.011.
- Waterson, R. 2012. Living House: An Anthropology of Architecture in South-East. Asia: Tuttle publishing.
- Wheeler, S. M., and T. Beatley. 2014. Sustainable Urban Development Reader. London and New York: Routledge.
- Wolsink, M. 2010. “Contested Environmental Policy Infrastructure: Socio-political Acceptance of Renewable Energy, Water, and Waste Facilities.” Environmental Impact Assessment Review 30 (5): 302–311. doi:10.1016/j.eiar.2010.01.001.
- Wong, J. K., and H. Li. 2008. “Application of the Analytic Hierarchy Process (AHP) in multi-criteria Analysis of the Selection of Intelligent Building Systems.” Building and Environment 43 (1): 108–125. doi:10.1016/j.buildenv.2006.11.019.
- Yang, W., and N. Kohler. 2008. “Simulation of the Evolution of the Chinese Building and Infrastructure Stock.” Building Research & Information 36 (1): 1–19. doi:10.1080/09613210701702883.
- Yee, A. A., and P. H. D. Eng. 2001. “Social and Environmental Benefits of Precast Concrete Technology.” PCI Journal 46 (3): 14–19. doi:10.15554/pcij.05012001.14.19.
- Yeh, C. H. 2002. “A Problem‐based Selection of Multi‐attribute Decision‐making Methods.” International Transactions in Operational Research 9 (2): 169–181. doi:10.1111/1475-3995.00348.
- Younger, M., H. R. Morrow-Almeida, S. M. Vindigni, and A. L. Dannenberg. 2008. “The Built Environment, Climate Change, and Health: Opportunities for co-benefits.” American Journal of Preventive Medicine 35 (5): 517–526. doi:10.1016/j.amepre.2008.08.017.
- Yu, P-L. 2013. Multiple-criteria Decision Making: Concepts, Techniques, and Extensions. New York, London: Springer Science & Business Media. doi:10.1007/978-1-4684-8395-6.
- Yuan, Z., C. Sun, and Y. Wang. 2018. “Design for Manufacture and Assembly-oriented Parametric Design of Prefabricated Buildings.” Automation in Construction 88: 13–22. doi:10.1016/j.autcon.2017.12.021.
- Yunus, R., and J. Yang. 2011. “Sustainability Criteria for Industrialised Building Systems (IBS) in Malaysia.” Procedia Engineering 14: 1590–1598. doi:10.1016/j.proeng.2011.07.200.
- Zanakis, S. H., A. Solomon, N. Wishart, and S. Dublish. 1998. “Multi-attribute Decision Making: A Simulation Comparison of Select Methods.” European Journal of Operational Research 107 (3): 507–529. doi:10.1016/S0377-2217(97)00147-1.
- Zeleny, M. 2012. Multiple Criteria Decision Making Kyoto 1975. Berlin, Heidelberg, New York: Springer Science & Business Media. doi:10.1007/978-3-642-45486-8.
- Zhang, X., M. Skitmore, and Y. Peng. 2014. “Exploring the Challenges to Industrialized Residential Building in China.” Habitat International 41: 176–184. doi:10.1016/j.habitatint.2013.08.005.
- Zhi, H. 1995. “Risk Management for Overseas Construction Projects.” International Journal of Project Management 13 (4): 231–237. doi:10.1016/0263-7863(95)00015-I.
- Zukin, S. 1989. Loft Living: Culture and Capital in Urban Change. New Brunswick, New Jersey, USA: Rutgers University Press.