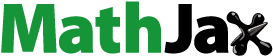
ABSTRACT
The renewable energy transformation will impact the entire economy. We investigate the impact and interlinkages in employment and non-renewable energy with the renewable energy transition in Europe. We further assess the potential contributions of renewable energy and non-renewable energy to the variability (changes) of future employment, output, and carbon emissions within the European Union (EU). Analyzing recent data from 28 EU countries and Norway, we employ a panel vector autoregressive regression model to estimate the potential interlinkages. Our results suggest that the transition to renewable energy sources has a positive but small and significant net impact on average employment in EU. We further find that renewable energy consumption contributes substantially to the future changes in employment in the short and the medium term. The potential effect of employment on non-renewable fossil-fuel-based energy consumption is relatively lower. Moreover, future renewable energy consumption contributes significantly to variations in non-renewable energy, per capita carbon emissions and GDP per capita in the short and the medium-term. The contribution of non-renewable energy to the future variability in renewable energy consumption is low, reflecting the diminishing impact of fossil-fuel-based energy on renewable energy consumption.
1. Introduction
Energy accounts for two-thirds of the global greenhouse emissions (IEA, Citation2021). For this to change, renewable energy will be a critical element for any energy transformation. This transition to renewable energy systems has the potential to affect the entire economy – from food and agriculture, ecosystems and climate, poverty, and health, to employment and economic growth, etc. The level of impact depends on the available sources of energy, their demand in the various sectors of the economy and the substitution possibilities between the various energy sources. A growing body of research literature is examining the interlinkages of the sustainable and clean energy goal (Sustainable Development Goal (SDG) 7) that aims to increase the share of renewables to ensure affordable, reliable, and sustainable sources of energy, with other SDG indicators (Hillebrand et al. Citation2006; Moreno and López Citation2008; Neuwahl et al. Citation2008; Riekkola et al. Citation2011; McCollum et al. Citation2018; Bali Swain and Karimu Citation2020). However, only a few studies have examined the interlinkages between employment and renewable energy within the European Union (Manne and Wene Citation1992; Neuwahl et al. Citation2008; Lehr et al. Citation2012; Ortega et al. Citation2015; Mu et al. Citation2018).
Policymakers see renewable energy source (RES) transformation as a panacea to multiple socio-economic-environmental challenges. It is promoted as a win-win solution that at once addresses the climate change, sustainable development, and unemployment-related problems. However, is this true? This study investigates the potential interlinkages in employment, renewable energy, and non-renewable energy amongst the European Union (EU) countries. Second, it further assesses the potential contributions of renewable energy and non-renewable energy to the variability (changes) of future employment, output, and carbon emissions in the EU.
The share of renewable sources in gross energy use in EU nearly doubled in 2018 as compared to 2005 (EEA Citation2019a, Citation2019b). This has been possible due to the 2020 targets for renewable energy under the 2009 Renewable Energy Directive (EU Citation2009) and the increased competitiveness, following fast technological progress and significant cost reductions (IRENA Citation2019). The energy transformation towards renewable resources and technologies is expected to bring about relative improvements of 2.5% in GDP and 0.2% in the whole-economy employment, by 2050 (IRENA Citation2019).
According to IRENA (Citation2020), the current global employment in renewable energy is estimated at 11.5 million in 2019, as compared to 11 million in 2018 (32% of these jobs are held by women; 38% are in China; and about 3.8 million jobs are in the photo-voltaic (PV) industry). Within the EU, 1.3 million renewable energy jobs are estimated in 2019 (EurObserv’ER Citation2020). Germany, the United Kingdom, France, Italy, Spain, and Poland are the leading renewable energy employers. The bioenergy sector is the largest EU renewables employer with 392400 jobs estimated in the solid biomass (heat and electricity), followed by biofuels (239 000), and biogas (74 900). The International Renewable Energy Agency (IRENA) estimates EU wind power employment at about 292300 jobs (Wind Europe Citation2020) and the employment in the solar PV industry at 127300 jobs, indicating a significant increase in the European markets (IRENA Citation2020).
Investigating the potential interlinkages in employment, renewable and non-renewable energy, we evaluate the impact of RES on future employment changes within the EU. Employing a panel vector autoregressive (PVAR) regression model, which utilizes a system of equations to estimate the potential interlinkages between various variables and their lags for the EU. The analysis is performed using Eurostat database that covers EU-28 countries and Norway, for the period 2000 to 2018. Additional variables are merged from the World Development Indicators (WDI). In this paper, we focus on the average employment effect of renewable energy in the EU (plus Norway) and not the individual countries’ employment effect. However, we control for the countries’ specific effects in order to estimate a reliable average employment effect.
Our results suggest that the transition from the non-renewable energy sources to renewable energy sources has a positive but small and significant net impact on average employment in EU. According to our estimates, the renewable energy consumption contributes 17.6% (15.1%) to the future variability or changes in employment at 5-years (10-years) horizon. The potential effect of employment on non-renewable fossil-fuel-based energy consumption is relatively lower. Moreover, future renewable energy consumption contributes significantly to variations in non-renewable energy, per capita carbon emissions and GDP per capita in both the short (5-years) and the medium-term (10-years horizon). The contribution of non-renewable energy to the future variability in renewable energy consumption was found to be low, showing a diminishing impact of fossil-fuel-based energy on renewable energy consumption.
This study makes some important novel contributions to the existing literature. First, acknowledging the interlinkages between the energy sector and various other sustainable development goals, we employ a system of equations via PVAR approach to estimate the impact of both renewable and non-renewable energy on employment, while accommodating the likely interlinkages between the energy sector, economy, and the environment. Whilst some of the studies focus on energy nexus with a few SDGs, they seldom use it in assessing the contributions to the variability of future employment outcomes (McCollum et al. Citation2018; Bali Swain and Karimu Citation2020). Some of the literature that considers the various sectors within the economy and their interlinkages, remain limited to a single country (for example, Bulavskaya and Reynes, Citation2018 on Netherlands; Pereira da Silva et al. Citation2013 on Portugal; Haerer and Pratson Citation2015; Garett-Peltier, Citation2017 on USA).
Second, only a few studies have investigated the employment impact of renewable energy in the EU (Moreno and López Citation2008; Lehr et al. Citation2012) while studies that explicitly estimate the net energy employment effect in EU are also limited (OECD Citation2018; IRENA Citation2020, Citation2019 etc.). In addition to estimating the net RES employment effect, we further analyze how the unexpected changes in RES may impact the employment in the short and mid-term, while accommodating the potential substitution possibilities of the employment effect of the two energy sources (renewable and non-renewable).
Third, according to IRENA estimate a transition to renewable energy is expected to lead to 24 million jobs by 2030 and create about 25 million jobs globally by 2050. This new job creation in renewables and energy efficiency is expected to be greater than the job losses in the traditional energy sector, thus leading to a positive net employment (IRENA Citation2017b). Furthermore, the net positive employment impact of renewable energy uptake will be enhanced from the additional job creation from climate stabilization impact on ecosystem services. This is possible as sectors that rely significantly on the environment and its natural processes to create goods and services, such as forestry, fishing industry, agriculture, eco-tourism, and the pharmaceutical industry, will be able to scale-up as the environment improves. This channel of renewable energy impact on employment is either ignored or aggregated into total employment impact of renewables. This study provided such an analysis to highlight the potential employment possibilities from ecosystem services channel for the EU.
Finally, the PVAR approach adopted in this paper provides us with an alternative method to address endogeneity and capture the simultaneous interactive effects of the transition towards renewable energy through the economic mechanisms of price (cost) on the demand of industry and households.
The structure of the paper is as follows. The following sections present a brief overview of the existing literature and methods used to investigate the relationship between SDGs, energy sources and employment. Sections 3 and 4 describe the data and methods used in this paper. This is followed by the discussion of results in section 5. The final section presents the discussion and conclusions.
2. Energy sector and employment
2.1 Theory, mechanisms, and components of renewable energy effect on employment
Recent literature has focused on the consequential effects of clean energy production on economic growth, employment generation, industrial innovation, and infrastructural developments (Manne and Wene Citation1992; Messner and Schrattenholzer Citation2000; Duscha et al. Citation2014; Meyer and Sommer Citation2014; Henriques et al. Citation2016). Most studies are concerned with the different sources of renewable energy and their contributions in reducing the GHG (greenhouse gas) emissions (Ryan et al. Citation2006) or increasing economic growth and employment generation (Hillebrand et al. Citation2006; Moreno and López Citation2008; Neuwahl et al. Citation2008; Lambert and Pereira da Silva Citation2012; Böhringer et al. Citation2013; McCollum et al. Citation2018).
The underlying theoretical explanation of the impact of renewable energy transition on employment is through the economic mechanisms of price and cost effects or the impact of energy prices (costs) on the industry and the households. It is also explained in terms of the structural demand effects or the impact of demand on the industry, the households and trade. Furthermore, the multiplier and accelerator effects of the households and industry behavior on other economic sectors; and the impact of innovation or productivity on industry and households are other causal factors that lead to changes in employment with transition in the renewable energy sector (Walz et al. Citation2008; Walz and Schleich Citation2008; OECD Citation2018).
The export orientation, technological competitiveness, regulation, and market context factors, such as demand, prices, market structure, etc., leads to quality competition that determines foreign trade successes and creates lead markets or first movers’ advantage. The supporting policies for renewable energy and the resulting first mover advantage, impacts the households, firms, and trade as they react to the price, quality, and quantity changes. This response is amplified further by the multiplier, accelerator, and innovation effects. It leads to an impact on the demand and the prices in the economic sub-sectors, such as investment, operation and maintenance and consumption across all sectors in the economy. For a detailed theoretical discussion and conceptual framework, refer to Ragwitz et al. (Citation2009).
The resulting net employment impact of renewable energy policy is disaggregated in the literature into these three components – direct, indirect (substitution and income effect) and trade effect. The direct (gross) effect measures a change in job creation in renewable sectors. However, this does not take into account the substitution and income effects that may be negative for job creation in the non-renewable energy sector. For example, budget re-allocation to renewable sector and increasing extraction cost of exhaustible resources (e.g., crude oil) from a deep well may increase the price of non-renewable energy and may lead to a reduction in the employment opportunities. This type of substitution effect is primarily driven by the re-allocation of investment budget and the changes in energy prices. In the literature (e.g. Lehr et al. Citation2012; Ortega et al. Citation2015), this is termed as the indirect effect. The direct effect provides only a positive side of investment in renewables, but the size of indirect effect is a key determinant for estimating the net employment effect. For example, Lehr et al. (Citation2011) suggest that the number of indirect jobs is usually larger than the direct jobs for all renewable energy technologies.
The scope for exports and imports of renewable and non-renewable energy may also influence the size of the net employment effect. The size of trade effect may vary with the gravity of origin and destination countries. For instance, a sharp reduction in consumption of fossil fuels in oil importing countries may reduce the employment opportunities in the Organization of the Petroleum Exporting Countries (OPEC) countries, whereas the investment in renewables (e.g., solar or wind energy) by these oil importing countries may create more jobs in their domestic markets.
A part of the literature (Mu et al. Citation2018; Stavropolous and Burger, Citation2020) have a slightly different interpretation of these effects. According to them the direct employment effects are the jobs created as a result of increased RES capacity. Indirect employment effect is generated by industries that support the RES sector expansion. These effects are considered positive on net employment. The induced effects, such as the decline in fossil fuel investment, changes in energy prices to competition for capital, changes in labor wages and household income, etc., may produce either a positive or a negative net impact on employment.
2.2 Methods for estimating employment impact of renewable energy
An additional challenge in collecting data on green jobs is on how to define and measure them. UNEP et al. (Citation2008) suggest finding universally applicable measures of green employment such as: employment in new technologies, business practices, or shifts in employment where it is hard to identify differences between efficiency improvement from regular employment; employment in environment-related technologies or ‘green tech’; and employment in newly emerging sectors of the economy such as renewable energy production etc.
The literature on the methodologies estimating the employment generation in the renewable energy literature can be broadly classified into three broad approaches: employment factor approach; supply chain analysis or input-output (I-O) method; and computable general equilibrium (CGE) models. For an in-depth discussion on the methods refer to Lambert and Silva (Citation2012). These three methods differ in terms of the induced effects in their estimation, for example, I-O methods are limited to accounting for investment decreases in traditional energy sources and household income (Stavropoulos and Burger Citation2020). Although the CGE models can introduce multiple induced effects they are computation and data intensive, requiring data for all sectors of the economy that may not be available (Mu et al. Citation2018)
The employment factor approach is the easiest and quickest approach to estimate direct effects, multiplying certain renewable energy capacity by employment factors. The employment factor denotes the number of jobs (in full-time equivalents) created per physical unit (e.g. megawatts energy produced or megawatt-hours for electricity generation, etc.) (IRENA Citation2013). Different employment factors are applied for different phases of the life cycle. Furthermore, the employment factors also depend on the technology generation, labor productivity and regional characteristics.
Supply chain analysis, or the input-output method, is more suited to a project-specific analysis than a macro-economic modeling and employment assessment. Supply chain analysis is a microeconomic approach based on business surveys to map the specific supply hierarchy and relationships among companies of a specific renewable technology. The stages of production and services are identified and based on the data on capacity, project costs, labor and other inputs, turnover, and production values at each tier of the supply chain – the labor inputs are related to the output capacity (IRENA Citation2013; Llera et al. Citation2013)
Several studies use a fixed coefficient input-output framework to explore the impact of renewable energy policies on employment and the labor market (Hillebrand et al. Citation2006; Lehr et al. Citation2008; Ragwitz et al. Citation2009). Based in economic theory, the input-output analysis provides in-depth information on the flows of intermediary goods and services among all sectors of the economy and the rest of the world. Thus, the total production of an industry is the sum of all its inputs to the other industries plus final demand and the exports minus imports (Breitschopf et al. Citation2011; IRENA Citation2013). For example, analyzing the German renewable support policy, Hillebrand et al. (Citation2006) conclude that the policy is likely to result in positive level of net employment in the short term but a negative level impact in the medium to long-term. Lehr et al. (Citation2008) find a net positive effect of the German renewable support policy, with a lower long-run rate of unemployment. Investigating this for the EU renewable support policies, Ragwitz et al. (Citation2009) confirm a similar net positive impact on employment.
The employment factor approach and the input-output modeling do not capture the economy-wide employment effects in terms of net employment. To estimate these net employment impact, comprehensive economic models (e.g. CGE or macro-econometric models) are estimated, taking the economy-wide price, income and substitution effects into account. As Breitschopf et al. (Citation2011) argue, these affect the households’ consumption and production of intermediate products and services, in addition to impacting the competitiveness of the entire industries due to changed energy prices. CGE models are thus analytical approaches for assessing direct, indirect and induced employment effects (Meyer and Sommer Citation2014). For instance, assessing a CGE model in Canada, Böhringer et al. (Citation2012) suggest that the tariff policy is likely to result in a decrease in employment and a rise in unemployment. Implementing a multi-sector, multi-region, recursive dynamic computable general equilibrium model, Kuster et al. (Citation2007) conclude that the impact of renewable energy investment subsidies in EU increase unemployment rates in each of the EU countries.
A body of theoretical literature employs stylized general equilibrium models to investigate the relation between environmental taxation and employment (Bovenberg and de Mooij Citation1994; Bovenberg Citation1995; Bovenberg and van der Ploeg Citation1996; Schneider Citation1997; Scholz Citation1998, Citation1998a, Citation1998b). Employing a three-sector general equilibrium model, Rivers (Citation2013) investigates the impact of renewable electricity support policies on the rate of equilibrium unemployment. Parameterizing the model to represent the US economy, Rivers concludes that reducing the electricity sector emissions by 10% by switching to renewable electricity support policies would increase the equilibrium unemployment rate by 0.1–0.3%.
Previous studies have utilized both a bottom-up (BU) and top-down (TD) models in an interactive fashion to estimate the economic impacts of sustainable energy policy (Böhringer Citation1998; Messner and Schrattenholzer Citation2000; Chen Citation2005; Böhringer and Rutherford Citation2008; Neuwahl et al. Citation2008). The BU models such as MARKAL, APOLLO, MESSAGE, etc., provide a technology-detailed description of the energy system, while the TD models, such as MACRO or CGE models describe the broader macroeconomic activities of the country. A synthesis of these BU and TD models allow for interactions between the energy system and the rest of the economy, and are referred to as a soft or hard linked model, depending on the nature of the linkage (Manne and Wene Citation1992; Böhringer Citation1998; Riekkola et al. Citation2013).
In general, the hybridization of these BU and TD models is considered as a hard-link (Böhringer and Rutherford Citation2008), which can ensure the simultaneous equilibrium between the BU energy system models and the TD macroeconomic growth models. The soft-link approach defines a functional form to transmit the estimation outcomes from one to the other model (see Riekkola et al. Citation2013 for details). In the soft-link approach, every single model is treated as an individual entity and allows for explaining the technical details. In doing so, the BU model have a wider space to represent the technical details of energy system, and the TD model can describe macroeconomic activities with a wider scope. In the literature, the hard-link approach has been used extensively in energy policy analysis (Böhringer Citation1998; Messner and Schrattenholzer Citation2000). With the hard link it is easier to produce the impact output, as it is embedded in the model system and computes the impact directly. The soft-link approach is more tractable and has been used by the researchers to analyze the impulse response of energy policy on the job market.
These methodologies differ in their capability to estimate direct, indirect and induced effects of RES and thus result in different reported net employment effects (Mu et al. Citation2018; Stavropoulos and Burger Citation2020).
2.3 Renewable energy transition and the employment impact
The employment impact of renewable energy sources has largely been found to have a net positive effect (Meyer and Sommer Citation2014; Kabel and Bassim Citation2019). In a meta-analysis of 23 peer-reviewed impact studies, Meyer and Sommer (Citation2014) analyze the employment impact of renewable technologies and model-based scenario assessments from specific renewable policies. They find an overall positive net employment effect, although the results crucially depend on the financing of the renewable energy source support scheme and the global competitiveness of a specific technology.
Several studies also find a positive net employment effect because of new investments in the supply-chains of renewable energy system. Moreno and López (Citation2008) report a large effect on job creation in the construction and installation phase of renewable energy sources, which can possibly drop in the later phase. Lehr et al. (Citation2012) find positive effects on labor market with an increased amount of investment in the renewable energy sector. However, the technology effect may lower the employment opportunities. IRENA (Citation2017a) reports that the technological revolution can increase automation in operation and maintenance, e.g., of solar photovoltaics and wind plants, which can lower investment and job creations. IRENA (Citation2017b) finds that low oil prices and oversupply resulted in a loss of about 440000 jobs in the oil and gas sector.
Analyzing an input-output model for US between 2008 and 2012, Haerer and Pratson (Citation2015) find that renewable energy sources (natural gas, solar and wind industries) create greater employment than the fossil-fuel industry. In fact, Garrett-Peltier (Citation2017) argue that energy efficiency and renewable energy industries in the US created three times as many jobs as the fossil-fuel industry, at the same level of spending. In the US, the solar industry alone employs more than the coal industry in Electric Power generation (DOE Citation2017).
Overall, in the EU, the renewable energy employment has increased, with Germany, UK, France, Spain in the lead (IRENA Citation2020). However, other countries are also making successful gains in employment with the transition towards renewable energy. For Netherlands, Bulavskaya and Reynès (Citation2018) find that transition to renewable energy will create about 50000 new jobs by 2030 and add about 1% to the GDP due to higher labor and capital intensity of renewable energy technology, as compared to gas and coal plants. Pereira da Silva et al. (Citation2013) also finds significant employment benefit of renewable energy deployment in Portugal. In Ukraine, solar PV deployment capacity tripled in 2019 and created24800jobs (one-third of which are in the labor-intensive rooftop solar segment) (IRENA Citation2020).
3. Methods
The focus of this study is to examine the impact of renewable and non-renewable energy on employment, considering the interlinkages between energy, economy, and the environment. Furthermore, we quantify potential contributions of renewable energy and non-renewable energy to the future variation in employment, output and carbon emissions within the EU. To address the various interlinkages between the energy sector, economy and the environment, we use a system of equations modelling approach that underpins the PVAR econometric model. An additional reason for the choice of PVAR is the fact that by design it makes it easy to quantify the potential changes in renewable and non-renewable energy on future changes in employment, output (GDP) and carbon emissions.
The PVAR model for this study is presented compactly as
where yit is z*1 vector of z variables for country i in time t, yit-1 is a vector of lag variables of the vector yit, is a z*z vector of parameters to be estimated, and
it is a composite term that is made up of time fixed effects (vt) to account for time variant unobserved factors, such as economy policy, unobserved individual effects (
i) to capture unobserved country heterogeneity, and a random error term (
it). The variables composed in yit are the logarithmic values of renewable energy, non-renewable energy sources, employment rates, per capita GDP and greenhouse gas emissions. The logarithmic transformation of the employment is used, having the benefit of reducing the effects of outliers.
All the equations stacked in Equationequation (1)(1)
(1) are estimated jointly as a system, which makes it possible to trace the feedback effect from each variable on to the other. Thus, we can assess the potential trade-offs or complementarity, for instance, of renewable energy directly on each of the other variables in the model and vice-versa.
Given the lag dependent structure in Equationequation (1)(1)
(1) , a fixed effect model will suffer from nickel bias if the time dimension is less than 30 years (Bali Swain and Karimu Citation2020). The standard procedure to address such a bias is to use instrumental variable (IV) approach if there are valid external instruments or use the generalized method of moment procedure (GMM) when there are no valid external instruments (Arellano and Bover Citation1995). In the GMM approach lagged internal variables are used as instruments. We opted for the GMM instrumentation approach due to lack of valid external instruments and applied it to the PVAR model.
The empirical strategy follows two steps. In the first step, the PVAR model is estimated. This step provides estimates for each of the variables in the model for each of the equations that constitute the system. Such a system approach makes it possible to assess the interlinkages and causal impacts of the variables in the model. In the second step, the estimated model results are utilized to quantify the changes in both renewable and non-renewable energy and how these changes contribute to the variation of each of the other variables in the model, particularly employment, output and carbon emissions for a short term (5 years ahead) and medium term (10 years ahead).
It should be noted that our methods have a few limitations. Due to the differences in energy resource endowment across EU countries, trade in these resources is an important issue to consider. Our main result does not explicitly include trade in the analysis but the likely bias that would be created by not explicitly including trade is reduced by the inclusion of the time fixed effects in the model. The time fixed effects capture the trade effect in addition to other factors, such as economic policy, which are not explicitly included in the model. Moreover, the development of renewable energy in different countries within the EU is different. A heterogeneous study may have been considered rather than estimating the average effects within the EU, but the available data that we have access to, has a short-time dimension and therefore makes it unreasonable to use heterogeneous panel estimators or individual country time series analysis. It is important to note that we included country fixed effects to account for country heterogeneity and therefore limits the likely bias due to country differences.
4. Data
The analysis is based on the Eurostat database for EU-28 countries plus Norway for the period 2000 to 2018. Renewable and non-renewable energy sources are final consumption measured in 1000 tons of oil equivalents (TOE). Employment measures the total employment rate. Greenhouse gas emissions (GHG) are CO2 equivalents measured in tons per capita and gross domestic product (GDP) is also measured in per capita terms.
Consumption data is used instead of generation data since consumption or demand is the main signal for producing any good or service. In the case of energy production, demand profile determines production profile to a large extent plus renewable energy services. This suggest that relying on production data instead, we will likely lose the potential services aspect of renewable energy technologies (RET) on employment as it only captures the production aspect. In such a case, we will not be able to account for RET impact on employment from the services related employment. This is consistent with previous studies, such as Apergis and Payne (Citation2014), who used consumption data.
outlines the distribution of the key variables in our study (renewable and non-renewable energy, employment rate and carbon emission). The figure shows significant variability within countries depicted by the size of the boxes (inter-quartile range) and across countries depicted by variation in the median value of the variables of interest (this is depicted by the white inline in the boxes). The top five countries in renewable energy consumption (in volume) over the period under study are Germany, France, Italy, Sweden and Finland, whereas countries, such as Malta, Luxemburg and Cyprus, have the least renewable energy consumption. Note that these rankings are in absolute values, so naturally the countries with larger economies also have more energy consumption, both from renewable and non-renewable sources. In per-capita terms, the rankings would be somewhat different.
Figure 1. Distribution of key variables: Renewable energy sources, non-renewable energy sources, total employment rate and GHG emissions.
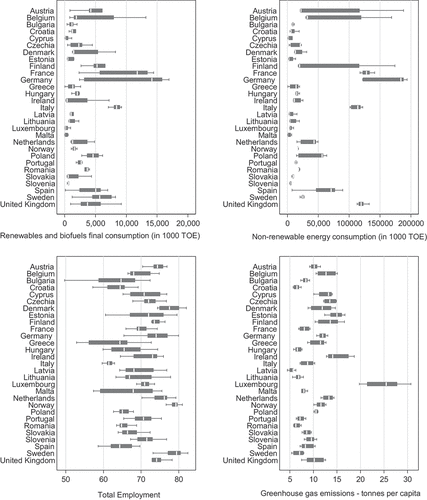
In the case of non-renewable energy, the five top consuming countries in the period are Germany, France, United Kingdom, Italy and Spain. Furthermore, the figure also reveals significant variability both within and between countries. Similarly, we see significant variation between and within countries in the case of employment variable. It appears, however, that the variation is more apparent between countries as the median value of employment between countries range from 63% in Italy (the lowest) to 78% in Sweden (the highest). The variations within and between countries is used in the empirical estimation process to answer the key research questions of our study.
5. Results
Before proceeding with the results of the model, we need to verify the model fit and stability. This is required in order to meaningfully interpret the results of the model and asses the causal relationships. We use Hansen’s J-test to verify the model fit and assess the stability of the model by inspecting the modulus of each eigenvalue of the estimated PVAR model.
Hansen’s J-test tests whether the model specification is overidentified with the length of lags used for the instruments and can be classified as a model specification test. The model fit is accepted if there is no overidentification. The test statistic is reported in and shows that there is no overidentification, justifying the model fit.
Table 1. PVAR results
The model stability is determined by the modulus of each eigenvalue of the estimated PVAR model. The model is stable only if each modulus is strictly less than 1 (Hamilton Citation1994; Lütkepohl Citation2005). Results for the stability test are presented in the Appendix (). All moduli are strictly less than one. The model therefore satisfies both the Hansen’s J-test for model fit and the stability test.
Furthermore, in choosing the appropriate lags to use in the PVAR model, Andrews and Lu (Citation2001) Model Moment Selection Criteria (MMSC) was adopted, where the appropriate lag length is based on the minimum value for each of the following lag section criteria MBIC, MAIC, and MQIC, which are the MMSC versions of the standard BIC, AIC and QIC. The results based on this procedure is reported in the Appendix () and all three criteria indicate that the lag length of 1 is appropriate, therefore a lag length of 1 is used for the PVAR model estimation.
Additionally, we check the time series properties of the data, though the time period is short and some of the tests (unit root test) may not be appropriate due to low power in such an instance. In the interest of completeness, we went ahead to perform unit root test on the data. Using the Pesaran’s (Citation2007) unit root test, the result suggests all the variables are stationary except GDP and CO2, which become stationary after first difference. These results are reported in Appendix . Given the mixed integration, we tested for cointegration based on Pedroni’s (Citation1999) panel cointegration approach. The results from the test reported in Appendix also suggest that the null hypothesis of no cointegration relationship is rejected at the 5% significance level, implying evidence of long-run relationship among the variables. raph: use this for the first paragraph in a section, or to continue after an extract.
5.1 PVAR results
Results from the PVAR are presented in . The estimated coefficients show that all variables have statistically significant impacts on employment rates at least at the 5% level except in the case of per capita carbon emissions, which is only significant at the 10% level. While the elasticity value of the effect of renewable energy sources on employment is 0.036, that of non-renewable energy sources show a negative effect (−0.031).
Combining the two estimates (0.036 and −0.031), the total net energy impact on employment is positive (0.005) but the magnitude is small. The total net energy impact on employment may be explained in terms of the employment substitution effect from non-renewables via one or both of the mechanism presented in the next paragraph. Relocation of the employment segment of the renewable energy value chain in cost advantage countries, such as China, can be another possible factor.
There are two potential mechanisms through which energy impacts employment, and which explain the above findings. These are the investment impulse mechanism and the price-induced mechanism. An increase in investment that favors Renewable Energy Technology (RET) instead of Conventional Energy Technologies (CET) and services, would trigger production in the renewable energy industry and create positive employment outcomes for green jobs. As resources are limited, increased investment in the renewable energy industry will result in a displacement of investment in conventional energy technologies, services and ultimately reduce production in that sector. This will have a negative effect on employment. Furthermore, trade would enhance the employment effect of investing in RET through creating market and increasing demand for renewable energy products and services across the globe (Duscha et al. Citation2014).
The second mechanism works through prices and how the economy reacts to price changes induced by taxes, levies or direct price changes. Unlike the stimulating effect of the increase in investment, price increases on the contrary have a damping effect on economic activity as it reduces households’ budget and hence consumption expenditure. The reduced households’ demand will reduce production and incomes in the affected industries, which has a multiplier effect and ultimately impact job creation. This suggests that if RET is favored in terms of lower taxes and levies, it will increase their profit margins and generate more green jobs via the multiplier effect. Conversely, an increase in taxes on CET due to environmental and other concerns would likely affect their profit margins and consequently reduce job creation via the multiplier effect.
The positive net effect of total energy on employment is found to be consistent with previous studies, such as Moreno and López (Citation2008) and Lehr et al. (Citation2012). Moreover, these results provide partial evidence in support of a substitution effect between renewable and non-renewable energy impact on employment.
Consistent with our intuition, the results reveal a positive GDP per capita effect on employment. On the other hand, the effect of per capita carbon emissions is negative, but only significant at the 10% significance level. The negative impact of carbon emission is consistent with the finding from ILO (Citation2018), which indicated a net negative effect of CO2 and broadly climate change on employment. This is driven by the influence of CO2 on climate change impact on loss of ecosystem services and jobs that rely on such natural processes, such as farming; fishing; forestry; food, drink and tobacco; wood and paper; renewable energy; water; textile; chemical and environment-related tourism. A study by Montt et al. (Citation2018) provide further support on the impact of rising levels of CO2 and climate change on employment. Their study revealed that 16% of the total employment in Europe depend directly on ecosystem services, suggesting a significant loss of ecosystem services could potentially result in job losses to a maximum of 16% in Europe.
Renewable energy sources are positively impacted by the consumption of renewable energy sources (6.202), and negatively impacted by GDP (−1.934) and carbon emissions (−1.066). The non-renewable energy sources have no significant impact on renewable energy. The reverse causality, i.e., how renewable energy affects non-renewable energy, is also positive and non-significant, as shown by the third equation (non-RES equation).
The consumption of both renewable and non-renewable energy sources is negatively affected by increases in GDP per capita, with elasticity values of −1.934 and −1.876, respectively. This may be explained by the decoupling of GDP from energy in general, driven in part by energy efficiency achievements within the EU. Such a decoupling was also found for Swedish industrial sectors regarding carbon emissions and output for Swedish energy-intensive industries by Brännlund et al. (Citation2015).
Furthermore, from the GDP equation, the results indicate that the consumption of renewable energy and previous level of GDP per capita are positively significant causal factors for GDP per capita, whereas carbon emission is a negative significant causal factor. The consumption of non-renewable energy, on the other hand, has a non-significant estimate.
The last equation (carbon emission equation) suggest that greenhouse gas emissions are significantly impacted by non-renewable energy, with an elasticity value of 0.062. The coefficient for renewable energy is negative (−0.001) but insignificant at any of the conventional significance level.
5.2 Forecast Error Variance Decomposition (FEVD)
Forecast Error Variance Decomposition (FEVD) is shown in . The focus is to assess the response of each of the variables to the future variability in renewable () and non-renewable energy consumption () in the region. The results in indicate that 17.6 and 15.1% of the future variation in employment is explained by renewable energy resources at 5-year and 10-year horizons, respectively. In contrast, non-renewable energy consumption for the 5-year and 10-year horizon explains about 9.8% and 14.3% of the future variations in employment.
Figure 2. Forecast Error Variance Decomposition (FEVD), shown as 5 and 10 years ahead responses to one standard deviation shock to non-renewables (left panel) and renewables (right panel). The measurements show how much shocks in renewables and non-renewables contribute to the future variability of the variables.
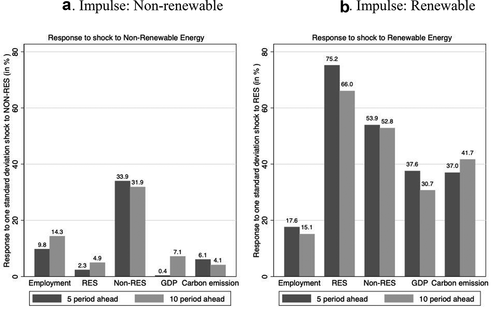
Short-term and medium-term renewable energy consumption contributes significantly to both the future variation in non-renewable energy and per capita carbon emissions (). Whereas the short-term response (53.9%) is slightly larger relative to the medium-term (52.8%) in the case of non-renewable energy, the reverse is true in the case of per capita carbon emissions (37% in the short-term against 41.7% for the medium-term). Renewable energy also contributed significantly to the future GDP per capita variability, about 37.6% in the short term and 30.7% in the medium term. The future variability in renewable energy is self-contributed by renewable energy (75.2% in the short run and 66% in the medium horizon).
presents the future variability of the other variables in the model in response to non-renewable energy shock. 9.8% (14.3%) of the future variability in employment is in response to non-renewable energy shock in the short (medium) horizon. Non-renewable energy has a low impact on the future variation of GDP per capita and per capita carbon emissions in the short and medium term. Only 0.4% of the future GDP per capita variations in the short term and 7.1% in the medium term is explained by non-renewable energy shock. Whereas in the case of per capita carbon emission, 6.1% in the short term and 4.1% in the medium term of the future variability is explained by non-renewable energy. Interestingly, non-renewable energy’s contribution to the future variability in renewable energy consumption is low, about 2.3% in the short term and 4.9% in the medium term.
6. Discussion
Renewable energy is the key component of the EU energy system transformation with a thrust from the EU Directive 2009 and the energy union strategy of the EU (European Commission Citation2015) advocating a move towards using more renewable energy – and supporting research, innovation and competitiveness. The 2030 framework for climate and energy sets the target for achieving a 32% share of renewable energy consumption. It is widely accepted that this transformation will have several socio-economic impacts, especially an impact on GDP, greenhouse gas emissions and employment.
Analyzing country-level Eurostat data and data from the World Bank’s World Development Indicators (WDI) for the period 2000–2018, we estimate the average impact of renewable energy on employment for the European Union (EU) countries. We also analyze the impact of carbon emission on employment in the EU. Furthermore, we examine the contributions of renewable energy and non-renewable energy to the future variation in employment, output and carbon emissions within the EU.
Several interesting results emerge. We find that renewable energy has a positive and significant employment impact for the EU and renewable energy consumption contributes substantially to the future variability in employment both for the short and medium term. We also find that the average total net effect of carbon emission on employment is negative. Furthermore, consistent with prior studies such as (Lehr et al. Citation2012), we find a positive effect of RES on GDP. These results support and extend the earlier research on employment impact of renewable energy (Moreno and López Citation2008; Lehr et al. Citation2012).
The positive net employment effect of renewable energy in the EU suggests that the policies promoting the low carbon energy transition in the EU appear to be in the correct direction and likely to encourage public support from the employment perspective. This together with the positive impact on GDP implies RES promotion could be a panacea to some socio-economic-environmental challenges in the EU.
The current Russia–Ukraine war highlights the vulnerability of EU to energy security due to the high dependence on Russia for its energy needs, particularly that of gas. For instance, in the year 2020, Europe imported about 78.9% of its total oil needs, out of this, 29% is from Russia (British Petroleum Citation2021), suggesting a significant oil import dependence. Given the positive impact of RES on both GDP and employment, it makes it easy and prudent to promote RES even if it is based on energy security as it does not compromise employment and GDP needs.
Furthermore, the contribution of RES to changes in the future employment levels are quite significant for the short to medium term, while those coming from non-renewables are significantly smaller in comparison. This among other things, is driven by the labor-intensive nature of renewable energy technologies relative to non-renewable energy technologies; and partly because most of the jobs from non-renewable energy deployment are generated in countries outside the EU, particularly Asian countries. The domestic employment effect of RES in the EU is higher than that from non-renewable energy as most of the jobs in the non-renewable energy sector are related to the supply of the fuels, which for the EU is very minimal.
These findings, especially the findings on the RES employment effects and the effect on GDP are in line with theoretical predictions on the mechanism through which RES will impact employment. Though the study does not explicitly distinguish between the direct and indirect effects in the empirical analysis, the fact that a net employment effect is determined suggests that these effects are captured. Both the investment mechanism and the price-induced mechanism are in play, producing the positive net employment effect of RES in the EU. These mechanisms are working through the EU renewable energy policy (Renewable Energy Directive) that started in 2009, where a 20% target of all energy consumption in the EU should be from renewable sources and 10% of all transport fuels should be from renewable sources. These targets were revised in 2018 and more recently in 2021, where 40% of EU total energy consumption should be from RES by 2030. Beyond these two mechanisms as prescribed by theory; our study identifies a third mechanism that works through climate stabilization effect of RES on ecosystem services. This employment impact within EU can be further amplified by designing policies that continue to support this transformation and policies that attempt to relocate renewable resource value chain within the European Union.
Acknowledgments
The authors express their gratitude to Christina Gravert, Xiang Lin, Linus Palmblad, Carl-Martin Johborg, Svetlana Gross, Shyam Basnet, Shyam Rangnathan and the participants of the Sustainable Development and Energy Use workshop at Misum, Stockholm School of Economics and the seminar participants at Södertörn University. Thanks are due to Hjalmar Skog for excellent research assistance. This work was supported by the Swedish Energy Agency. The usual disclaimer applies.
Disclosure statement
No potential conflict of interest was reported by the author(s).
Additional information
Funding
References
- Andrews DWK, Lu B. 2001. Consistent model and moment selection procedures for GMM estimation with application to dynamic panel data models. Journal of Econometrics. 101(1):123–164. doi:10.1016/S0304-4076(00)00077-4.
- Apergis N, Payne JE. 2014. The causal dynamics between renewable energy, real GDP, emissions and oil prices: evidence from OECD countries. Applied Economics. 46(36):4519–4525. doi:10.1080/00036846.2014.964834.
- Arellano M, Bover O. 1995. Another look at the instrumental variable estimation of error-components models. Journal of Econometrics. 68(1):29–51. doi:10.1016/0304-4076(94)01642-D.
- Bali Swain R, Karimu A. 2020. Renewable electricity and sustainable development goals in the EU. World Development. 125(1), 1-11.
- Böhringer C 1998. The synthesis of bottom-up and top-down in energy policy modeling.
- Böhringer C, Rutherford TF. 2008. Combining bottom-up and top-down. Energy Economics. 30(2):574–596. doi:10.1016/j.eneco.2007.03.004.
- Böhringer C, Rivers N, Rutherford T, Wigle R. 2012. Green jobs and renewable electricity policies: employment impacts of Ontario’s feed-in tariff. The BE Journal of Economic Analysis & Policy. 12(1):1–40.
- Böhringer C, Keller A, van der Werf E. 2013. Are green hopes too rosy? Employment and welfare impacts of renewable energy promotion. Energy Economics. 36:277–285. doi:10.1016/j.eneco.2012.08.029.
- Bovenberg A, de Mooij R. 1994. Environmental levies and distortionary taxation. The American Economic Review. 84(4):1085–1089.
- Bovenberg A. 1995. Environmental taxation and employment. De Economist. 143(2):111–140. doi:10.1007/BF01384532.
- Bovenberg A, van der Ploeg F. 1996. Optimal taxation, public goods and environmental policy with involuntary unemployment. Journal of Public Economics. 62(1–2):59–83. doi:10.1016/0047-2727(96)01574-5.
- Bovenberg A, van der Ploeg F. 1998a. Consequences of environmental tax reform for unemployment and welfare. Environmental and Resource Economics. 12(2):137–150. doi:10.1023/A:1016040327622.
- Bovenberg A, van der Ploeg F. 1998b. Tax reform, structural unemployment and the environment. Scandinavian Journal of Economics. 100(3):593–610. doi:10.1111/1467-9442.00124.
- Brännlund R, Lundgren T, Söderholm P. 2015. Convergence of carbon dioxide performance across Swedish industrial sectors: an environmental index approach. Energy Economics. 51:227–235. doi:10.1016/j.eneco.2015.07.004.
- Breitschopf B, Nathani C, Resch G 2011. Review of approaches for employment impact assessment of renewable energy deployment, Franhofer ISI, rütter + partner, Energy Economics Group, Study commissioned by IEA-RETD, November 2011.
- British Petroleum. 2021. Statistical review, July. https://www.bp.com/en/global/corporate/energy-economics/statistical-review-of-world-energy.html
- Bulavskaya T, Reynès F. 2018. Job creation and economic impact of renewable energy in the Netherlands. Renewable Energy. 119:528–538. doi:10.1016/j.renene.2017.09.039.
- Chen W. 2005. The costs of mitigating carbon emissions in China: findings from China MARKAL-MACRO modelling. Energy Policy. 33(7):885–896. doi:10.1016/j.enpol.2003.10.012.
- DOE, The US department of energy. 2017. US Energy and Employment Report.
- Duscha V, Ragwitz M, Breitschopf B, Schade W, Walz R, Pfaff M. 2014. Employment and growth effects of sustainable energies in the European Union. (Accessed on 24 May 2022) https://ec.europa.eu/energy/sites/ener/files/documents/EmployRES-II%20final%20report_0.pdf.
- EEA. 2019a. Renewable energy in Europe: key for climate objectives, but air pollution needs attentions. Briefing No. 13/2019.
- EEA. 2019b. Renewable energy in Europe-2019, EEA, Eionet Report – ETC/CME/2019/8.
- EU. 2009. Promotion of the use of energy from renewable sources. EU Directive 2009/28/EC.
- EurObserv’ER. 2020. The state of renewable energies in Europe, 2019 edition. Paris. (Accessed on 24 May 2022) https://www.isi.fraunhofer.de/content/dam/isi/dokumente/ccx/2020/The-state-of-renewableenergies-in-Europe-2019.pdf.
- European Commission. 2015. A framework strategy for a resilient energy union with a forward-looking climate change policy. Brussels: European Commission.
- Garrett-Peltier H. 2017. Green versus brown: comparing the employment impacts of energy efficiency, renewable energy, and fossil fuels using an input-output model. Economic Modelling. 61:439–447. doi:10.1016/j.econmod.2016.11.012.
- Haerer D, Pratson L. 2015. Employment trends in the U.S. Electricity Sector, 2008 – 2012. Energy Policy. 82:85–98. doi:10.1016/j.enpol.2015.03.006.
- Hamilton JD. 1994. Time series analysis. New Jersey, USA: Princeton university press.
- Henriques CO, Coelho DH, Cassidy NL. 2016. Employment impact assessment of renewable energy targets for electricity generation by 2020—An IO LCA approach. Sustainable Cities and Society. 26:519–530. doi:10.1016/j.scs.2016.05.013.
- Hillebrand B, Buttermann HG, Behringer JM, Bleuel M. 2006. The expansion of renewable energies and employment effects in Germany. Energy Policy. 34(18):3484–3494. doi:10.1016/j.enpol.2005.06.017.
- IEA. 2021. Net Zero by 2050. Paris: IEA https://www.iea.org/reports/net-zero-by-2050
- ILO. 2018. The employment impact of climate change adaptation. input document for the G20 climate sustainability working group international labour office. Geneva (ILO): 2018.
- IRENA. 2013. Renewable energy and jobs, international renewable energy agency. Abu Dhabi, UAE.
- IRENA. 2017a. Renewable energy and jobs. international renewable energy agency (IRENA). Abu Dhabi, UAE.
- IRENA. 2017b. Renewable energy benefits: understanding the socio-economics. International Renewable Energy Agency (IRENA), Abu Dhabi, UAE.
- IRENA. 2019. Global energy transformation. International Renewable Energy Agency, Abu Dhabi, UAE.
- IRENA. 2020. Renewable Energy and jobs, Annual Review 2020, IRENA.
- Kabel TS, Bassim M. 2019. Literature Review of Renewable Energy Policies and Impacts. European Journal of Marketing and Economics. 2(2):28–41. doi:10.26417/ejme-2019.v2i2-68.
- Kuster R, Ellersdorfer I, Fahl U 2007. A CGE analysis of energy policies considering labor market imperfections and technology specifications. Working Paper 73, Fondazione Eni Enrico Mattei.
- Lambert RJ, Pereira da Silva P. 2012. The challenges of determining the employment effects of renewable energy. Renewable and Sustainable Energy Reviews. 16(7):4667–4674. doi:10.1016/j.rser.2012.03.072.
- Lehr U, Nitsch J, Kratzat M, Lutz C, Edler D. 2008. Renewable energy and employment in Germany. Energy Policy. 36(1):108–117. doi:10.1016/j.enpol.2007.09.004.
- Lehr U, Lutz C, Edler D, O’Sullivan M, Nienhaus K, Nitsch J, Breitschopf B, Bickel P, Ottmüller M. 2011 February. Kurz- und langfristige Auswirkungen des Ausbaus der erneuerbaren Energien auf den deutschen Arbeitsmarkt, Studie im Auftrag des Bundesministeriums für Umwelt. Berlin (Karlsruhe, Stuttgart): Naturschutz und Reaktorsicherheit, Osnabrück, p. 2011.
- Lehr U, Lutz C, Edler D. 2012. Green jobs? Economic impacts of renewable energy in Germany. Energy Policy. 47:358–364. doi:10.1016/j.enpol.2012.04.076.
- Llera E, Scarpellini S, Aranda A, Zabalza I. 2013. Forecasting job creation from renewable energy deployment through a value-chain approach. Renewable and Sustainable Energy Reviews. 21:262–271. doi:10.1016/j.rser.2012.12.053.
- Lütkepohl H. 2005. New introduction to multiple time series analysis. Berlin: Springer.
- Manne AS, Wene CO. 1992. MARKAL-MACRO: a linked model for energy-economy analysis. Brookhaven National Laboratory and Chalmers University of Technology, Gothenberg, Sweden.
- McCollum DL, Echeverri LG, Busch S, Pachauri S, Parkinson S, Rogelj J, Krey V, Minx JC, Nilsson M, Stevance A-S, et al. 2018. Connecting the sustainable development goals by their energy inter-linkages. Environmental Research Letters. 13(3):1–23. doi:10.1088/1748-9326/aaafe3.
- Messner S, Schrattenholzer L. 2000. MESSAGE-MACRO: linking an energy supply model with a macroeconomic module and solving it iteratively. Energy. 25(3):267–282. doi:10.1016/S0360-5442(99)00063-8.
- Meyer I, Sommer MW. 2014. Employment effects of renewable energy supply A meta analysis. Austrian Institute of Economic Research (WIFO), Vienna, Austria.
- Montt G, Fraga F, Harsdorff M. 2018. The future of work in a changing natural environment: climate change, degradation and sustainability. In: ILO research paper series. Geneva: International Labour Office.
- Moreno B, López AJ. 2008. The effect of renewable energy on employment. The case of Asturias (Spain). Renewable and Sustainable Energy Reviews. 12(3):732–751. doi:10.1016/j.rser.2006.10.011.
- Mu Y, Cai W, Evans S, Wang C, Roland-Holst D. 2018. Employment impacts of renewable energy policies in China: a decomposition analysis based on a CGE modeling framework. Applied Energy. 210:256–267. doi:10.1016/j.apenergy.2017.10.086.
- Neuwahl F, Löschel A, Mongelli I, Delgado L. 2008. Employment impacts of EU biofuels policy: combining bottom-up technology information and sectoral market simulations in an input-output framework. Ecological Economics. 68(1–2):447–460. doi:10.1016/j.ecolecon.2008.04.018.
- OECD. 2018. Impacts of green growth policies on labour markets and wage income distribution: a general equilibrium application to climate and energy policies, Working Party on Integrating Environmental and Economic Policies, ENV/EPOC/WPIEEP(2016)18/FINAL, Environment Directorate, OECD.
- Ortega M, Río P, Del Ruiz P, Thiel C. 2015. Employment effects of renewable electricity deployment. A novel methodology. Energy. 91:940–951. doi:10.1016/j.energy.2015.08.061.
- Pedroni P. 1999. Critical values for cointegration tests in heterogeneous panels with multiple regressors. Oxford Bulletin of Economics and Statistics. 61(s1):653–670. doi:10.1111/1468-0084.61.s1.14.
- Pereira da Silva P, Oliveira C, Coelho D. 2013. Employment effects and renewable energy policies: applying input-output methodology to Portugal. International Journal of Public Policy. 9(3):147–166. doi:10.1504/IJPP.2013.055702.
- Pesaran MH. 2007. A simple panel unit root test in the presence of cross section dependence. Journal of Applied Econometrics. 22(2):265–312. doi:10.1002/jae.951.
- Ragwitz M, Schade W, Breitschopf B, Walz R, Helfrich N, Rathmann M, Resch G, Panzer C, Faber T, Haas R, et al. 2009. The impact of renewable energy policy on economic growth and employment in the European Union. Brussels (Belgium): European Commission, DG Energy and Transport.
- Riekkola AK, Ahlgren EO, Söderholm P. 2011. Ancillary benefits of climate policy in a small open economy: the case of Sweden. Energy Policy. 39(9):4985–4998. doi:10.1016/j.enpol.2011.06.015.
- Riekkola AK, Berg C, Ahlgren E, Söderholm P. 2013. Challenges in soft-linking: the case of EMEC and TIMES-Sweden. National Institute of Economic Research, Stockholm, Sweden.
- Rivers N. 2013. Renewable energy and unemployment: a general equilibrium analysis. Resource and Energy Economics. 35(4):467–485. doi:10.1016/j.reseneeco.2013.04.004.
- Ryan L, Convery F, Ferreira S. 2006. Stimulating the use of biofuels in the European Union: implications for climate change policy. Energy Policy. 34(17):3184–3194. doi:10.1016/j.enpol.2005.06.010.
- Schneider K. 1997. Involuntary unemployment and environmental policy: the double dividend hypothesis. Scandinavian Journal of Economics. 99(1):45–59. doi:10.1111/1467-9442.00046.
- Scholz C. 1998. Involuntary unemployment and environmental policy: the double dividend hypothesis: comment. Scandinavian Journal of Economics. 100(3):663–664. doi:10.1111/1467-9442.00128.
- Stavropoulos S, Burger MJ. 2020. Modelling strategy and net employment effects of renewable energy and energy efficiency: a meta-regression. Energy Policy. 136(111047):111047. doi:10.1016/j.enpol.2019.111047.
- UNEP ILO, Ituc IOE. 2008. Green Jobs: towards decent work in a sustainable, low-carbon world, United Nations environment programme, international labour organization, international organization of employers, international trade union confederation, Report produced by WorldWatch Institute with technical assistance from Cornell University, Global Labour Institute.
- Walz R, Schleich J. 2008. The economics of climate change policies: macroeconomic effects, structural adjustments and technological change. Heidelberg: Springer.
- Walz R, Ragwitz M, Schleich J. 2008. Regulation and innovation: the case of renewableenergy. DIME Working Papers on Environmental Innovation No. 2.
- Wind Europe. 2020. Wind energy in Europe in 2019. Brussels: Trends and Statistics. (Accessed on 24 May 2022) https://windeurope.org/wp-content/uploads/files/aboutwind/statistics/WindEurope-Annual-Statistics-2019.pdf.
APPENDIX
Table A1. Stability test for EU PVAR model
Table A2. Optimal lag selection for EU sample model
Table A3. Pesaran (Citation2007) CIPS unit root test
Table A4. Panel cointegration test