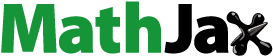
ABSTRACT
Theories of intertemporal price discrimination imply that prices must be chosen using mixed strategies, with retailers changing their prices randomly over time. Otherwise, consumers will learn which retailer has the lowest price, and eventually, all customers will patronize the lowest price retailer, or all retailers will charge the same price. We test whether price dispersion is explained by intertemporal price discrimination strategies using a dataset of identical products sold through the PriceSpy price comparison website. Our results show that there are clusters of retailers with similar pricing within each cluster, but with different price levels between clusters even after controlling for retailer heterogeneity. Retailers also remain in the same price cluster over time, suggesting that consumers have ample opportunities to learn which retailers belong to which price cluster. Intertemporal price discrimination is thus unlikely to have caused the observed price dispersion.
I. Introduction
In his seminal article, Stigler (Citation1961) pointed out the extensive price dispersion for homogeneous products sold in well-developed markets. Several studies have since then confirmed his findings, even in markets with low search costs. In a recent study of price dispersion covering 1.4 million goods in 54 markets in the US, Kaplan and Menzio (Citation2015) found that the average standard deviation of prices was 19% for identical goods sold in the same geographical market during the same quarter, while it was 36% for close but not identical substitutes.
The most commonly used models for explaining such price dispersion are clearinghouse models. In a clearinghouse model, retailers must simultaneously appeal to two types of customers: shoppers who search and use the available price list to buy from the retailer offering the lowest price, and non-shoppers who do not engage in search but learn prices over time as they visit stores’ or retailers’ webpages. For price dispersion to remain, there must be some consumers who are non-shoppers, and prices must be chosen using mixed strategies, with retailers changing their prices randomly (Varian Citation1980; Lach Citation2002). Otherwise, consumers will eventually learn which retailer has the lowest price, and all customers will either patronize the lowest price retailer, or all retailers will charge the same price. The reasons for not being a shopper can differ. Consumers might have strong preferences for a specific retailer (Rosenthal Citation1980; Narasimhan Citation1988), or not have access to the clearinghouse price list (Varian Citation1980).
The most widely cited clearinghouse model was developed by Varian (Citation1980), but there have been several followers extending that model in different directions (Narasimhan Citation1988; Stahl Citation1989). Recent extensions include models with heterogeneous search costs (Chen and Zhang Citation2011), unknown production costs (Jansen et al., Citation2011), non-shoppers with some but limited information (Parakhonyak Citation2014), costly recall for consumers (Jansen and Parakhonyak Citation2014), and geographical consumer segregation (Obradovits Citation2017). A common feature of these models is that the use of mixed strategies creates price dispersion in equilibrium. Clearinghouse models have also been widely used as a motive for empirical studies relating price dispersion to the size of search costs in different markets (Bayliss and Perloff Citation2002; Lach Citation2002; Baye, Morgan, and Scholten Citation2004; Seim and Sinkinsson, Citation2016; Pennerstorfer et al. Citation2020).
The use of mixed strategies has empirically testable implications. First, there can be no grouping of retailers having similar, and thus predictable, price strategies that remain over time. Second, the position of individual retailers within a cross-sectional price distribution will change randomly over time. Therefore, there will be no distinguishable patterns in a transition matrix of prices, and the probability of remaining in the same position in the transition matrix should be low.
There are three previous studies that formally test if both the necessary (remaining price dispersion) and sufficient (randomization of prices) conditions for clearinghouse models are fulfilled. Evidence from these studies are mixed, with Bayliss and Perloff (Citation2002) rejecting the clearinghouse model, while Lach (Citation2002) and Baye, Morgan, and Scholten (Citation2004) support it. However, Bayliss and Perloff (Citation2002) and Baye, Morgan, and Scholten (Citation2004) do not account for retailer heterogeneity in their transition matrix analysis, while Lach (Citation2002) arbitrarily groups retailers into quartiles when investigating price movements.
We test the predictions from clearinghouse models using a dataset of identical products sold through the PriceSpy price comparison website. In contrast to previous studies, we account for retailer heterogeneity and use cluster analysis to determine the size and number of retail clusters with similar prices. As such, we contribute to the literature by adding a current and methodologically comprehensive test of clearinghouse models. We find that data support the necessary condition of remining price dispersion from a clearinghouse model, but not the sufficient condition of retailers randomizing their prices.
II. Method
Compared to previous studies (Bayliss and Perloff Citation2002; Lach Citation2002; Baye, Morgan, and Scholten Citation2004), we consider a large set of products (14) that are observed with a higher frequency (daily) and for a longer period (up to 42 months). An important feature of our data is that the products are identical between retailers and that there is no ambiguity in product representation on the price comparison website. However, the average coefficient of variation for the price of these products () still reveals substantial price dispersion.
Table 1. Cluster characteristics and transition matrix results
Following Lach (Citation2002), we control for time-invariant retailer heterogeneity using the following model:
where is the CPI-adjusted price of product i on day t,
is a retailer-specific fixed effect and
is a day-specific fixed effect. The residual variation
for each individual retailer represents the percentage deviation of a retailer’s price from the geometric mean in the group of retailers when controlling for both retailer and time heterogeneity (Lach Citation2002).
Lach (Citation2002) divided the residuals from the estimation of EquationEquation (1)(1)
(1) into quartiles and then investigated the frequency and likelihood of price movements between these quartiles to determine whether price movements followed the predictions of intertemporal price discrimination models. However, the grouping of prices into quartiles is arbitrary, and both the number and size of retailer price clusters are likely to differ between products. Therefore, we adopt clustering techniques to let the data identify the number and size of retail price clusters instead of arbitrarily dividing the data into quartiles. If there are groups of retailers gathered in well-defined price clusters, these clusters remain over long periods, and the ranking of an individual retailer in a Markovian transition matrix of these clusters is likely to stay the same, this indicates that retailers do not follow an intertemporal price discrimination strategy.
There are some characteristics common to retail prices that might confound this analysis. For example, if a retailer changes price in advance of competitors, even by a day, it can influence whether that store belongs to a cluster of retailers or not, even if a similar pricing pattern is otherwise clearly visible. To mitigate this issue, we use the methods of dynamic time warping (Rath and Manmatha Citation2003) and piecewise aggregation approximation (Keogh et al. Citation2001). We then use the ‘elbow method’ (Thorndike Citation1953) to determine the appropriate number of clusters and the number of retailers belonging to each cluster. Finally, we determine to which extent retailers move between clusters using Markovian transition matrices.Footnote1 A pattern of retailer transition probabilities that align with the diagonal of a K x K transition matrix indicates that intra-distribution mobility is low. Following Lach (Citation2002), we use monthly transition matrices.
III. Results
shows that there are between 2 and 5 price clusters, depending on the product considered, and that the average amount of time that a retailer spends in a specific cluster ranges from 19 to 246 days. We also present the likelihood of observing an individual retailer along the diagonal, i.e., remaining in the same price cluster as in the month before, in . In a K x K transition matrix, the likelihood of randomly being observed in the diagonal will equal 1/K, while the likelihood of being observed in the off-diagonal will equal [(K-1) x K)/(K x K)]. Thus, in a 2 × 2 transition matrix, pricing using mixed strategies should give a likelihood for the diagonal of 0.5, while the likelihood for the diagonal for the two-cluster products ranges from 0.72 to 0.85 in our data. For a 3 × 3 transition matrix, we expect to observe the diagonal in 1/3 of all cases (0.33), but the averages instead range from 0.43 to 0.78. For no product do we reach the expected number according to intertemporal price discrimination models.Footnote2
IV. Discussion
Clearinghouse models predict that price dispersion can remain for identical products sold in markets with low search costs, given that retailers randomly change their prices over time. We have applied clustering techniques to test these predictions using data on identical products from the price comparison website PriceSpy. We found support for the prediction that price dispersion remains even after controlling for heterogeneity in retailer offerings, but no support that retailers randomize prices. On the contrary, there are clusters of retailers that maintain persistently high, mid-range, or low prices over time. The clearinghouse model is thus not supported by our analysis, suggesting that intertemporal price discrimination is unlikely to explain the observed price dispersion. One possible interpretation is that the share of consumers using price comparison websites in Sweden is still low enough to make it profitable for some retailers to focus on the group of uninformed consumers, while also listing their products on the price comparison website.
Supplemental Material
Download MS Word (40.8 KB)Acknowledgments
Research funding from the Swedish Retail and Wholesale Council, grant number 2018:773, is gratefully acknowledged. The authors would also like to thank Mark Dougherty and the participants at the Microdata analysis seminar (6 December 2019) for their valuable comments and suggestions.
Disclosure statement
No potential conflict of interest was reported by the authors.
Supplementary material
Supplemental data for this article can be accessed here.
Additional information
Funding
Notes
1 These methods are explained in more detail in appendices A and B in the supplemental online material.
2 Since the results are contingent on the time horizon considered, we also present results using biweekly and bimonthly transition matrices in appendix B in the online material. Except for the bimonthly result for the Apple iPhone, we observe the above expected probabilities along the diagonal of the transition matrices for all products. Additionally, for a direct comparison with Lach (Citation2002), we present results for retailer prices divided into quartiles in appendix C in the online material. This analysis also shows higher than expected probabilities along the diagonal of the transition matrices.
References
- Baye, M. R., J. Morgan, and P. Scholten. 2004. “Temporal Price Dispersion: Evidence from an Online Consumer Electronics Market.” Journal of Interactive Marketing 18 (4): 101–115. doi:10.1002/dir.20016.
- Bayliss, K., and J. M. Perloff. 2002. “Price Dispersion on the Internet: Good Firms and Bad Firms.” Review of Industrial Organization 21 (3): 305–324. doi:10.1023/A:1020415304514.
- Chen, Y., and T. Zhang. 2011. “Equilibrium Price Dispersion with Heterogeneous Searchers.” International Journal of Industrial Organization 29 (6): 645–654. doi:10.1016/j.ijindorg.2011.03.007.
- Jansen, M. C. W., and A. Parakhonyak. 2014. “Consumer Search Markets with Costly Revisits.” Economic Theory 55 (2): 481–514.
- Jansen, M. C. W., P. Pichler, and S. Weidenholzer. 2011. “Oligopolistic Markets with Sequential Search and Production Cost Uncertainty.” The Rand Journal of Economics 42 (3): 444–470. doi:10.1111/j.1756-2171.2011.00144.x.
- Kaplan, G., and G. Menzio. 2015. “The Morphology of Price Dispersion.” International Economic Review 56 (4): 1165–1206. doi:10.1111/iere.12134.
- Keogh, E., K. Chakrabarti, M. Pazzani, and S. Mehrotra. 2001. “Dimensionality Reduction for Fast Similarity Search in Large Time Series Databases.” Knowledge and Information Systems 3 (3): 263–286. doi:10.1007/PL00011669.
- Lach, S. 2002. “Existence and Persistence of Price Dispersion: An Empirical Analysis.” Review of Economics and Statistics 84 (3): 433–444. doi:10.1162/003465302320259457.
- Narasimhan, C. 1988. “Competitive Promotional Strategies.” The Journal of Business 61 (4): 427–449. doi:10.1086/296442.
- Obradovits, M. 2017. “Search and Segregation.” International Journal of Industrial Organization 55: 137–165. doi:10.1016/j.ijindorg.2017.09.004.
- Parakhonyak, A. 2014. “Oligopolistic Competition and Search without Priors.” The Economic Journal 124 (576): 594–606. doi:10.1111/ecoj.12142.
- Pennerstorfer, D., P. Schmidt-Dengler, N. Schutz, C. Weiss, and B. Yontcheva. 2020. “Information and Price Dispersion: Theory and Evidence.” International Economic Review 61 (2): 871–899. doi:10.1111/iere.12443.
- Rath, T. M., and R. Manmatha. 2003. “Word Image Matching Using Dynamic Time Warping.” Proceedings of the IEEE Computer Society Conference on Computer Vision and Pattern Recognition 2: 521–527.
- Rosenthal, R. W. 1980. “A Model in Which an Increase in the Number of Sellers Leads to A Higher Price.” Econometrica 48 (6): 1575–1579. doi:10.2307/1912828.
- Seim, K., and M. Sinkinson. 2016. “Mixed Pricing in Online Marketplaces.” Quantitative Marketing and Economics 14 (2): 129–155. doi:10.1007/s11129-016-9168-3.
- Stahl, D. O. 1989. “Oligopolistic Pricing with Sequential Consumer Search.” The American Economic Review 79 (4): 700–712.
- Stigler, G. J. 1961. “The Economics of Information.” The Journal of Political Economy 69 (3): 213–225. doi:10.1086/258464.
- Thorndike, R. L. 1953. “Who Belongs in the Family.” Psychometrika 18 (4): 267–276. doi:10.1007/BF02289263.
- Varian, H. R. 1980. “A Model of Sales.” The American Economic Review 70 (4): 651–659.