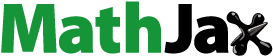
ABSTRACT
The pandemic has placed unprecedented pressures upon staff and students alike. Yet performance management of academics including Student Evaluation of Teaching (SET) persists. The American Association of University Professors (AAUP) has intervened on this issue. We develop new methods enabling better treatment of pandemic-era SET. Analysis of UK National Student Survey (NSS) data suggests 85% of institutions meet reasonable performance expectations during the pandemic. Results emphasize the need for a more sensitive treatment of pandemic-era SET.
I. Introduction
SETs remain a ‘ubiquitous but controversial’ part of universities (Boysen Citation2020). Though potentially informative about teaching problems arise when SET is used to review faculty (Sproule Citation2000). SETs have been termed ‘student perception data’ (Linse Citation2017) with students ill-equipped to judge teaching quality. SETs may contribute to grade inflation (Deem and Baird Citation2020; Marchant et al. Citation2020), display racial/gender biases and discriminate against quantitative subjects (Marchant et al. Citation2020). Low response rates (Bacon, Johnson, and Stewart Citation2016) and respondent anonymity (Raworth Citation2017) may encourage extreme outcomes.
The pandemic has raised concerns over low student-satisfaction levels (Sangster, Stoner, and Flood Citation2020). The AAUP has emphasized the need to protect faculty from SETs during the pandemic (Boysen Citation2020). Sources of student dissatisfaction may lie outside instructors’ control e.g. library access and IT infrastructure (Kerzic et al. Citation2021) and the effects of social restrictions (Park and Koo Citation2022). This adds to long-standing concerns about confounding factors associated with SET (Deem and Baird Citation2020).
The above reflects a long-standing need to analyse numerical teaching data (Sproule Citation2000) highlighted by the pandemic (Sangster, Stoner, and Flood Citation2020). Thus, we develop new methods to analyse pandemic-era SET. An application to NSS data suggests around 85% of institutions achieve reasonable performance expectations given the pandemic.
The layout of this paper is as follows. Section II quantifies the effect of the pandemic upon SETs. Section III develops a statistical model later applied to NSS data. Section IV concludes.
II. Quantifying the effect of the pandemic
The Chartered Association of Business Schools (CABS) collect NSS data. The effect of the pandemic can be measured by comparing institutions submitting to both the 2019 and 2021 exercises. Summary statistics in show the pandemic is associated with lower student-satisfaction levels and more variable responses. A paired t-test gives evidence of a significant difference in student satisfaction levels (t = 10.058, df = 142, p = 0.000). The pandemic thus results in reduced student satisfaction once we control for different institutions. The effect can be estimated as
Table 1. Summary statistics of NSS data: Proportion of students reporting being satisfied with their course.
EquationEquation (1)(1)
(1) suggests the pandemic is associated with an inevitable 10% reduction in student satisfaction. Karadag (Citation2021) obtains similar estimates.
III. Modelling student satisfaction
We model student satisfaction as follows. Suppose a respondent is satisfied with a course with probability . We assume independence of different respondents.Footnote1 Given n responses the probability that r people are satisfied is
Bayesian statistics allows us to estimate the probability the satisfaction level lies above/below a certain threshold. A reasonable target in non-pandemic times might be
. Consistent with other commonly-used teaching metrics this is just below average pre-pandemic satisfaction levels (see ). EquationEquation (1)
(1)
(1) suggests a more reasonable pandemic-era target would be
.
Using a standard Be() prior distribution for
(Lee Citation2012) means the posterior distribution for
given data in (2) is
Using a standard Jeffrey’s prior (Jeffreys Citation1998) with in (3) gives
From (4) the probability that the process is on-target is
where denotes the
CDF. There is thus no evidence student-satisfaction levels are unduly low unless Pr(
)<0.05.
We analyse data for business students during the 2021 NSS. shows once the pandemic is accounted for only 25/162 institutions clearly miss the target of . This result remains robust to the specification of alternative prior distributions. Results reflect unprecedented efforts devoted to pandemic-era teaching (Sangster, Stoner, and Flood Citation2020). Around 85% of institutions achieve reasonable performance expectations given the pandemic.
Table 2. Student satisfaction during the pandemic: probability the process is in control.
IV. Conclusions
The pandemic results in an estimated 10% reduction in student satisfaction (Karadag Citation2021). Much student dissatisfaction is likely unavoidable (Kerzic et al. Citation2021; Park and Koo Citation2022). The AAUP has itself intervened on SET usage during the pandemic. Using NSS data we estimate around 85% of institutions meet reasonable performance expectations. This figure is probably an under-estimate given the need to analyse SET sensitively (Deem and Baird Citation2020). These high-performance levels emphasize the need for a kinder evaluation of pandemic-era SET.
Disclosure statement
No potential conflict of interest was reported by the author.
Notes
1 A reasonable starting assumption pre-pandemic this is likely further enhanced by pandemic-era social restrictions. Generalized linear mixed models can resolve correlations between survey responses (Brint and Fry Citation2021).
References
- Bacon, D. R., C. J. Johnson, and K. A. Stewart. 2016. “Nonresponse Bias in Student Evaluations of Teaching.” Marketing Education Review 26 (2): 93–104. doi:10.1080/10528008.2016.1166442.
- Boysen, G. A. 2020. “Student Evaluations of Teaching During the COVID-19 Pandemic.” Scholarship of Teaching and Learning in Psychology forthcoming.
- Brint, A., and J. Fry. 2021. “Regional Bias When Benchmarking Services Using Customer Satisfaction Scores.” Total Quality Management & Business Excellence 32 (3–4): 344–358. doi:10.1080/14783363.2019.1568867.
- Deem, R., and J. -A. Baird. 2020. “The English Teaching Excellence (And Student Outcomes) Framework: Intelligent Accountability in Higher Education?” Journal of Educational Change 21 (1): 215–243. doi:10.1007/s10833-019-09356-0.
- Jeffreys, H. 1998. Theory of Probability. third ed. Oxford: Oxford University Press.
- Karadag, E. 2021. “Effect of COVID-19 Pandemic on Grade Inflation in Higher Education in Turkey.” PLoS One 16 (8): e0256688. doi:10.1371/journal.pone.0256688.
- Kerzic, D., J. K. Alex, R. Pamela Balbontin Alvarado, D. R. S. Bezerra, M. Cheraghi, B. Dobrowolska, A. F. Fagbamigbe, et al. 2021. “Academic Student Satisfaction and Perceived Performance in the E-Learning Environment During the COVID-19 Pandemic. Evidence Across ten Countries.” PLoS One 16 (10): e0258807. doi:10.1371/journal.pone.0258807.
- Lee, P. 2012. Bayesian Statistics. fourth ed. Chichester: Wiley.
- Linse, A. R. 2017. “Interpreting and Using Student Ratings Data: Guidance for Faculty Serving as Administrators and on Evaluation Committees.” Studies in Educational Evaluation 54: 94–106. doi:10.1016/j.stueduc.2016.12.004.
- Marchant, C. L., A. M. Ade, P. Clark, and J. Marion. 2020. “Bias and Trends in Student Evaluations in Online Higher Education Settings.” Collegiate Aviation Review International 38 (2): 34–50. doi:10.22488/okstate.20.100213.
- Park, M., and J. Koo. 2022. “It Takes a Village During the Pandemic: Predictors of students’ Course Evaluations and Grades in Online Team-Based Marketing Courses.” Marketing Education Review 32 (3): 255–264. forthcoming. doi:10.1080/10528008.2021.2023577.
- Raworth, K. 2017. “.” In Doughnut Economics: Seven Ways to Think Like a21st Century Economist (London: RH Business Books).
- Sangster, A., G. Stoner, and B. Flood. 2020. “Insights into Accounting Education in a COVID-19 World.” Accounting Education 29 (5): 431–562. doi:10.1080/09639284.2020.1808487.
- Sproule, R. 2000. “Student Evaluation of Teaching: A Methodological Critique of Conventional Practices.” Education Policy Analysis Archives 8: 1–23. doi:10.14507/epaa.v8n50.2000.