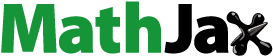
ABSTRACT
We provide an up-to-date quantitative synthesis of the evidence on the effect of unemployment on wellbeing based on 46 samples reported in 29 studies published between 1990 and 2020. Our sample includes longitudinal studies focusing on developed economies (e. g., EU-15 countries, UK, US, and Australia). We advance existing knowledge by exploring a wider range of wellbeing measures (both mental health and subjective wellbeing) and an extensive set of moderators capturing individual characteristics and country-level factors. In addition to the well-established negative impact upon mental health, our results present a negative relationship between unemployment and life satisfaction. In line with previous work, this negative association is stronger for men than women, and the longer the duration of unemployment, the larger the impact. We contribute to the existing evidence by pointing to the significant role in this relationship of gender, social and economic context, and norms/societal expectations regarding work. Finally, by utilizing longitudinal data and meta-analytic cross-lagged structural equation modelling, we present preliminary evidence on the existence of a reciprocal relationship between unemployment and wellbeing over time. While unemployment reduces wellbeing, poor wellbeing also leads to unemployment, indicating that individuals can become trapped in a cycle of unemployment and poor wellbeing.
Introduction
There has been a large and growing interest in the relationship between unemployment and wellbeing across multiple disciplines including economics, sociology, work and organizational psychology, and public health. There is a consensus that employment means more than earning a living; it brings social status, structure during the day, enables individuals to socialize, and creates a sense of purpose or meaning in life (Fryer, Citation1992; Jahoda, Citation1982; Warr, Citation1987). These positive elements are essential for psychological growth, promoting both subjective and eudaimonic wellbeing (Ryan & Deci, Citation2001; Waterman, Citation1993). It is, therefore, unsurprising that empirical work shows persistent negative effects of unemployment on wellbeing even after controlling for income, pointing to the significant non-pecuniary costs of unemployment. A number of systematic reviews and meta-analyses (McKee-Ryan et al., Citation2005; Murphy & Athanasou, Citation1999; Paul & Moser, Citation2009) have provided quantitative syntheses of the unemployment-wellbeing literature.
While it is well-established that unemployment is bad for wellbeing, we know less about whether the effect of unemployment on wellbeing is the same for all or if the impact is comparable across various wellbeing measures. Although previous meta-analyses have looked at important social, demographic, and economic factors likely to influence the association between unemployment and wellbeing, these moderator analyses exclusively focused on mental health and relied mainly on cross sectional data. The broader relationship with other wellbeing measures and how these factors might moderate the relationship between unemployment and wellbeing over time are not explored.
Perhaps most importantly, the latest meta-analysis on the unemployment-wellbeing association only included studies published up to 2004 (Paul & Moser, Citation2009). K. G. Shojania et al. (Citation2007) argue that the average life span of a systematic review of the literature is relatively short, with its findings having a median duration of survival of 5.5 years. There is an urgent need to update and expand the evidence base, particularly given significant changes in the social, institutional and economic context over the last decade or so. These changes include the severe financial crisis and recession that hit the Western economies in 2008, increases in women’s labour market participation, changes in gendered work norms (Fernández & Fogli, Citation2009), and changing attitudes to treatment of mental health (Ilic et al., Citation2015).
We make three key contributions to the literature on unemployment and wellbeing. Firstly, we provide an up-to-date review of the evidence base. Our meta-analysis provides a quantitative synthesis of the longitudinal studies investigating the impact of unemployment on wellbeing published between 1990 and 2020.Footnote1 This not only allows us to review the evidence based on a sufficiently long period of time but also to evaluate the evidence published after 2004. By introducing year of publication and data collection into our analyses, we also test formally whether the conclusions of the previous work remain valid. In parallel, we extend the set of contextual factors previously considered to include country-level measures of gender inequality and work norms.
Second, we consider broader measures of wellbeing, reflecting their increased use in the more recent academic and policy literatures (Clark et al., Citation2018; Grant et al., Citation2007; Hicks et al., Citation2013). The main focus in earlier meta-analyses was on mental health outcomes (McKee-Ryan et al., Citation2005; Murphy & Athanasou, Citation1999; Paul & Moser, Citation2009), while our wellbeing measures also include subjective wellbeing. Subjective wellbeing comprises assessments of life satisfaction, positive affect (e. g., joy and enthusiasm) and the relative absence of negative affect (e. g., lack of anxiety and feeling calm; Diener, Citation1984). Mental health is captured by measures such as psychological distress, depression, anxiety, and minor psychiatric disorders. Although related, we consider subjective wellbeing and mental health as distinct constructs (Daniels et al., Citation2018), for instance, a person can be mentally healthy but still experience negative affect. By taking a broad and multi-dimensional definition of wellbeing and addressing subjective wellbeing and mental health separately, we can meta-analyse any variation in the effect of unemployment on these wellbeing outcomes, which previous work was unable to explore fully. Moreover, incorporating indicators of subjective wellbeing provides a more thorough picture of the wellbeing consequences of unemployment that can inform targeted intervention approaches for the welfare of different groups (Hicks et al., Citation2013).
Finally, previous work acknowledges possible selection into unemployment, such that individuals with poor wellbeing are more likely to be unemployed (i. e., a reverse causal link between wellbeing and unemployment), as well as a reciprocal relationship between the two constructs. However, the selection effect has been shown to be weak (Paul & Moser, Citation2009) and the reciprocal association has not been tested formally. In contrast, our focus on longitudinal studies allows us to test a set of preliminary meta-analytic cross-lagged structural equation models. In so doing, we offer a more comprehensive understanding of the causal processes underpinning unemployment and wellbeing, by systematically comparing different models of causality and providing initial evidence on the reciprocal association between the two.
Previous meta- analyses
Building on narrative reviews on the topic, Murphy and Athanasou (Citation1999) provided the first meta-analysis on the relationship between unemployment and mental health based on 9 longitudinal studies published between 1986 and 1996. Although the authors noted that, on average, the unemployed suffer from poorer mental health than their employed counterparts, the causal evidence on the effect of moving into unemployment on mental health was less clear due to the limited number of studies with relatively small sample sizes.
Focusing mainly on cross-sectional evidence published between 1985 and 2002, McKee-Ryan et al. (Citation2005) investigated a wider range of wellbeing outcomes including overall life satisfaction, domain-specific satisfaction, and mental health. Their meta-analysis pointed to a medium-sized effect (according to Cohen’s (Citation1988) categorization), with the unemployed experiencing poorer mental health (average effect size corrected for unreliability (δ) = −.57; sample-size weighted average effect size (d) = −.52) and lower satisfaction with life (δ = −.48; d = −.44), marriage and family (δ = −.21; d = −.20). While some moderating effects, such as the total unemployment rate and degree of unemployment protection across the countries were also explored, due to reduction in sample size, these moderator analyses produced generally statistically insignificant results.
With mental health as the main wellbeing outcome of interest, an insightful meta-analysis by Paul and Moser (Citation2009) focused on studies published between 1963 and 2004. Once again, higher distress levels were reported for the unemployed (δ = .60; d = .54). Using a subset of longitudinal data their meta-analysis also noted that moving into unemployment was associated with increased symptoms of mental health problems, with an average effect size of d = .19. Paul and Moser (Citation2009) shed light on the cross-sectional impact of various individual factors, such as age, gender, and occupational status, on the relationship between unemployment and mental health, in addition to more macro-level moderators indicating socio-economic conditions and labour market policies (e. g., GDP to proxy economic development, the Gini index of income inequality, and the unemployment rate and unemployment protection system to account for labour market opportunities). These analyses showed that gender moderated the relationship between unemployment and mental health, and the negative effect on mental health was larger in countries with smaller GDP and high Gini-coefficients. Additionally, in a previous meta-analysis, the authors pointed to the important role of work commitment in explaining the mental health effects of (un)employment. For the unemployed group, the unfavourable mental health outcomes were more pronounced for those with a stronger work commitment (Paul & Moser, Citation2006).
Overall, previous meta-analyses were mainly based on cross-sectional data, while analyses of longitudinal data (including exploration of moderating factors) only covered mental health. Most importantly, previous work captured studies published until 2004 and naturally the data employed in these studies are even older. In fact, majority of the studies included in previous meta-analyses were published in 1990s and based on data sets covering 1980s or early 1990s. Therefore, there is little overlap between studies included in our meta-analysis and the ones included in previous meta-analyses. This further adds to the value of our contribution to updating the evidence base.
Unemployment and Wellbeing
There is no overarching theoretical framework for the experience of unemployment and associated wellbeing outcomes. However, the theories outlined below complement each other to provide a comprehensive understanding of the damaging effect of unemployment on individuals’ wellbeing.
The most influential theoretical explanation for the damaging effect of unemployment on wellbeing has been Jahoda’s functional model (Jahoda, Citation1981, Citation1982) which differentiates between the manifest and latent functions of employment. Aside from the financial rewards that enable people to earn a living (manifest benefits of employment), Jahoda points to five latent by-products of employment: Firstly, employment imposes a time structure of a working day. Second, it provides social contacts and shared experiences with people outside the family. Third, employment motivates individuals’ participation in a collective purpose beyond their own goals and aspirations. Fourth, employment is strongly linked to personal identity and status. Finally, work imposes regular activity (Jahoda, Citation1981, p. 23). Since unemployment excludes people from the social institution of employment and associated latent benefits, and frustrates the fulfilment of psychological needs, the unemployed suffer psychologically, feel disheartened, and lose their sense of time, self-worth, and respect.
Expanding the benefits of working life, Warr’s (Citation1987) model introduces nine “vitamins” that define wellbeing. These are: i) opportunity for control; ii) opportunity for skill use; iii) externally generated goals; iv) variety; v) environmental clarity; vi) availability of money; vii) physical security; viii) contact with others; and ix) valued social position. Within this framework, moving from employment to unemployment is interpreted as the result of moving to an environment associated with fewer vitamins. This loss of vitamins damages the wellbeing of people who transition into unemployment. In his later work, Warr introduced additional vitamins (e. g., supportive supervision, career outlook) which capture further beneficial elements of a job (Warr, Citation2007, Citation2017).
Both Jahoda’s functional model and Warr’s vitamin model emphasize institutional dimensions but model unemployed people as “passive” actors. In contrast, another body of literature views the unemployed as “active” individuals seeking to make sense of their own circumstances, and building strategies to cope with unemployment (see, for example, Fryer & Payne, Citation1984; Leana & Feldman, Citation1988, Citation1990). Building on stress and coping models (Folkman & Lazarus, Citation1985; Lazarus & Folkman, Citation1984), the damaging effect of unemployment on wellbeing is explained as the result of how people perceive their situation, i. e., appraisal of losing a job as a negative event/stressor (see, for example, Hamilton et al., Citation1993; Kinicki & Latack, Citation1990).
While they vary in terms of the weight they attribute to institutional dimensions or the individual, these theories lay the foundations for our understanding of the damaging effect of unemployment on wellbeing. We argue that the unemployed should neither be considered only as individuals or as members of a group. To understand the experience of unemployment fully, it is important to acknowledge the interrelationship between the self and the wider environment, and factors which may differentiate the experience of unemployment. For this purpose, we draw upon two additional theoretical explanations: The social identity approach (Hogg et al., Citation1995; Hornsey, Citation2008; Tajfel, Citation1981; Tajfel & Turner, Citation1986; Turner & Reynolds, Citation2010) and conservation of resources theory (Hobfoll, Citation1989, Citation2001; Hobfoll et al., Citation2018).
The social identity approach (comprising social identity and self-categorization theories) emphasizes the relevance of social identity in shaping individual identity. Accordingly, individuals may affiliate with a social group which represents a social identity that characterizes their attributes as members of that group (Hogg et al., Citation1995; Hornsey, Citation2008; Turner & Reynolds, Citation2010). Studies using social identity approach attribute the unfavourable wellbeing outcomes of unemployment to threatened or lost social identity upon losing a job (see, for example, Cassidy, Citation2001; Knabe et al., Citation2016; McFadyen, Citation1995; Schöb, Citation2013, Citation2021). By deviating from the social category of “the employed” and breaking the social norm to work, the unemployed individual faces the stigma associated with being unemployed, resulting in lower utility and satisfaction with life (Carroll, Citation2007; Howley & Knight, Citation2021; McFadyen, Citation1995). This approach explains why unemployment hurts more when the social norm to work is strong (Clark, Citation2003; AE Clark et al., Citation2010). The importance of the normative effect is also noted by the meta-analysis by Paul and Moser (Citation2006) looking into the role of work commitment (captured by measures such as work involvement and the Protestant work ethic) in the relationship between (un)employment and mental health. Their findings indicate that both the employed and unemployed individuals are strongly committed to work. For the unemployed, this represents an “incongruence” and the more they are committed to work (i. e., the stronger their normative belief about the value of work in life), the higher the distress associated with being unemployed (Paul & Moser, Citation2006).
Conservation of resources (COR) theory provides additional insights into the harm of unemployment. Broadly speaking, COR theory defines psychological stress as a reaction to a threat to or actual loss of resources, and the lack of resource gain following investing in resources (Hobfoll, Citation1989, Citation2001). COR theory has been applied to the experience of unemployment: becoming unemployed implies loss of a resource (i. e., employment) or threat to resources (i. e., money, financial security, status, acknowledgement of accomplishment) and the job search process typically requires greater investment of resources (e. g., energy and time; Lim et al., Citation2016; Virick & McKee-Ryan, Citation2017), which may not pay off. This negative spiral of resource loss results in wellbeing being impaired.
These theoretical frameworks inform the set of hypotheses about the relationship between unemployment and wellbeing, as well as potential moderating factors we explore in this meta-analysis. Accordingly, our first hypothesis is:
Hypothesis 1: Unemployment has a negative effect on wellbeing.
Potential moderators
Socio-demographic factors
Studies tend to find a larger negative effect of unemployment on men’s wellbeing when compared to women (e. g., Blanchflower & Oswald, Citation2004; Clark, Citation2003; Winkelmann, Citation2009). Masculine identity stressing work centrality, as well as gender roles, norms and societal expectations attributing domestic activities to women, all contribute to unemployment being more socially acceptable for women (Carroll, Citation2007; Winkelmann & Winkelmann, Citation1998). We therefore expect that:
Hypothesis 2: The negative effect of unemployment on wellbeing is more pronounced for men than women.
However, increasingly gender egalitarian attitudes mean that market-oriented work has become an important part of women’s lives as well (Fernández & Fogli, Citation2009; International Labour Organization [ILO], 2017). Recent evidence also suggests a convergence in the negative wellbeing effects of unemployment for men and women (Carroll, Citation2007; Strandh et al., Citation2013). By using more recent data we may be able to assess this shift in the gender dynamics influencing the unemployment-wellbeing association.
Next, we investigated the role of age. We expect that unemployment may be more damaging for younger individuals as they might be more focused on their career goals (Zacher & Frese, Citation2009) while older individuals may see unemployment as a time to satisfy relational goals as they place more importance on relationships and seeking emotional meaning (Carstensen, Citation2006). Age was not a statistically significant moderator in Paul and Moser (Citation2009)`s meta-analysis. Our results could be similar, or if the moderating effect of age turns out to be statistically significant, we would expect that:
Hypothesis 3: Unemployment has a less damaging effect on older individuals.
Finally, we explored the role of education. Building on COR theory, human capital may act as an important resource in the event of a job loss. Highly educated individuals have more educational resources and might cope better with unemployment, regarding it as a temporary situation and expecting that their human capital will assist them in finding a satisfactory job after a spell of unemployment (Kanfer et al., Citation2001; McKee-Ryan et al., Citation2005). We therefore expect that:
Hypothesis 4: The negative effect of unemployment on wellbeing is less strong for highly educated individuals.
Country-level moderators
We included a range of country-level measures to explore the impact of wider social, economic and institutional aspects. For example, unemployment can be less damaging in socially and economically developed countries (Paul & Moser, Citation2009), due to a more equal income distribution, greater social cohesion, and better access to resources such as education, training or health care. These are important resources for the unemployed when coping with unemployment or finding a new job (Hobfoll, Citation2001; Jahoda, Citation1982; McKee-Ryan & Kinicki, Citation2002; Warr, Citation1987).
In order to capture these elements, we used the Human Development Index (HDI) which summarizes the development of a nation along three key dimensions: health, education and standard of living. Relative to monetary measures of development such as the Gini index of income inequality and GDP as used in Paul and Moser (Citation2009), HDI captures broader aspects through the inclusion of health and education indices. Additionally, using the Gender Inequality Index (GII), we investigated the gender dimension of human development. The GII measures gender inequality related to reproductive health, empowerment, and labour market participation. While GII complements HDI in terms of social and economic development, it also assists in exploring the impact of gender. For instance, in addition to unfavourable social/economic conditions increasing the negative impact of unemployment, being unemployed can be more costly for men in countries characterized by more conservative gender roles. Due to insufficient information in the primary studies on which this meta-analysis is based, we could not test this three-way interaction or estimate the effect of GII separately for men and women. Whilst any evidence on gender roles can only be considered as initial and indirect, using GII as an additional moderator enables an exploration of the impact of gender inequality on the unemployment-wellbeing relationship which was not investigated by the previous meta-analyses. Thus, we expect to find that:
Hypothesis 5: Social and economic development (captured by HDI and GII) weaken the negative effect of unemployment on wellbeing.
Our analysis also explores the role of broader work norms. We categorized countries according to a Norm to Work (NTW) index developed by Roex and Rözer (Citation2017), which captures the social expectations related to being employed (i. e., to what degree people in a society are expected to work). Following self-categorization (Hogg & Abrams, Citation1988) and social identity (Tajfel & Turner, Citation1986) theories, social norms provide the basis for self-identity and self-definition. By conforming to social norms, individuals fulfil their need for social approval and acceptance, and boost their self-esteem and sense of worth, consequently sustaining individual wellbeing (Haslam et al., Citation2009). Therefore, given that being unemployed would mean breaking the norm or custom (Carroll, Citation2007), we expect to find that:
Hypothesis 6: The negative link between unemployment and wellbeing is more pronounced in countries with stronger norms to work.
The degree of employment protection and labour market flexibility in countries may also influence the impact of unemployment on wellbeing (McKee-Ryan et al., Citation2005; Paul & Moser, Citation2009). We used an Employment Protection Index developed by the Organisation for Economic Co-operation and Development (OECD, Citation2019; i. e., an indicator of strictness of individual and collective dismissal regulation for workers on regular contracts) to investigate these factors, and we also differentiated between European and non-European countries. We expect that making staff redundant could be harder in countries with a less flexible labour market and high degrees of employment protection. Therefore, losing a job could be a more uncommon outcome, increasing the likelihood of negative stereotypes and the stigmatization of unemployment. Hence,
Hypothesis 7: The negative effect of unemployment on wellbeing is stronger in countries with less flexible labour markets and high degrees of employment protection.
Finally, Paul and Moser (Citation2009) tested the moderating effect of the year of data collection to explore the changing effects of unemployment over time. The authors argued that, given the rising unemployment rates, being unemployed may have become normalized and may thus be more socially acceptable than before. Although we utilized the NTW index which is a more direct proxy of normative influence (see H6), we also tested publication year and year of data collection, which can further help to identify any trends over time (for example, improvements in research design and methodology). This also allowed us to verify the stability of relationships between unemployment and wellbeing reported by earlier studies.
Duration of unemployment
We also consider whether the negative impact on wellbeing persists or increases with longer spells of unemployment or whether people adapt. COR theory would point to a more detrimental effect of long-term unemployment since people are using up more resources and so experiencing higher stress while coping with unemployment. The empirical evidence suggests that longer term unemployment is generally more damaging to wellbeing or the negative association between unemployment and wellbeing is not sensitive to the length or frequency of unemployment (Booker & Sacker, Citation2012; Clark & Clark, Citation2006; Hahn et al., Citation2015; Lucas et al., Citation2004; Oesch & Lipps, Citation2013). The previous meta-analysis by McKee-Ryan et al. (Citation2005) found a weak link between duration of unemployment and wellbeing, whereas Paul and Moser (Citation2009) showed a stronger association. Given that, overall, the evidence suggests people suffer more the longer they remain unemployed, we expect to find:
Hypothesis 8: The duration of unemployment exacerbates the negative impact on wellbeing.
The reciprocal relationship between unemployment and wellbeing
Finally, although the causal link from unemployment to wellbeing is the main research question, the possibility of reverse causality (selection into unemployment) is commonly noted in the literature. Similarly, those with lower levels of wellbeing are less likely to be re-employed (see, Gedikli et al. (Citation2018) for a review of international evidence on reverse causality). An implication of COR theory is that people who lack resources are vulnerable to further resource loss (Hobfoll, Citation2001, p. 354), which can explain the impact of poor wellbeing on subsequent unemployment. In parallel, unemployed people need to invest in resources (such as energy and time) while seeking a job. The longer they remain unemployed, the greater the depletion of their resources, resulting in a loss spiral of unemployment (Virick & McKee-Ryan, Citation2017) unless they have personal resources such as high self-esteem or optimism to break the loss spiral or buffer against the negative effects of unemployment (Achdut & Refaeli, Citation2020; Lim et al., Citation2016; Merino et al., Citation2019). In other words, unemployment harms wellbeing which, in turn, leads to increased probability of future state of unemployment and so on, feeding into a reciprocal relationship between unemployment and wellbeing. Therefore, we predict that:
Hypothesis 9: Poor wellbeing is positively associated with unemployment over time.
Hypothesis 10: There is a reciprocal relationship between unemployment and wellbeing.
To clarify, here we are interested in investigating the reciprocal relationship between the constructs of unemployment and wellbeing over time, and this section of the analysis does not focus on transitions from employment to unemployment (or vice versa).
Method
The meta- analytic database
Literature search and inclusion/exclusion criteria
The starting point of the meta-analytic database is the set of primary studies retrieved by our initial systematic review of the literature (Gedikli et al. (Citation2017)), which explored the effect of transitioning into and out of a wide range of worklessness states (e. g., sickness, parental leave and retirement in addition to unemployment) on wellbeing. Concluded in September 2016, this review included 99 longitudinal empirical papers published after 1990 (see supplementary material A for details about the literature search and selection criteria). Only studies using data from advanced, developed economies (e. g., EU-15 countries, UK, US and Australia) were included because of the greater similarity in their labour market, welfare state and health systems.
Whilst this initial review captured worklessness states in general, for the purpose of the present meta-analysis, we only selected the studies focusing on unemployment and wellbeing. Consequently, 58 of the 99 studies were retained. Further, to capture the most recent evidence, we performed an additional systematic search which we concluded in November 2020. Here, while we used the same methodology as with the previous review we completed in 2016 (in particular, the same search terms capturing all forms of worklessness to achieve consistency), we only selected those studies looking at the impact of unemployment on wellbeing. This update yielded 10 additional studies published after September 2016 (see supplementary material A for details).
Further inclusion/exclusion criteria were applied at this stage. First, studies were included only if they reported an effect size for the relationship between unemployment and wellbeing or sufficient statistics to calculate it. Second, studies were retained only if they contained data not already used in other articles. When papers were based on the same national dataset (i. e., British Household Panel Survey, BHPS; European Community Household Panel, ECHP; German Socio-Economic Panel, GSOEP; Household, Income and Labour Dynamics in Australia, HILDA), we always opted for the study using the longest time period. While this ensured the statistical independence among the primary studies and prioritized longer datasets, it reduced the number of entries in the meta-analysis. When a study included independent samples and when data were presented separately by gender, age groups, or country, we coded them separately. Finally, multi-year data for the same paper were averaged across years. This screening process led to the retention of 46 samples reported in 29 primary studies.
Measurement of wellbeing
Our wellbeing measures included both subjective wellbeing, operationalized as life satisfaction, happiness, and positive affect (based on the measures used in the primary studies), and mental health captured by measures such as psychological distress, depression, and the General Health Questionnaire (GHQ; Goldberg, Citation1972; for a list of the included mental health measures see supplementary material B). Measures of eudaimonic wellbeing, the other component of psychological wellbeing (Waterman, Citation1993), were not reported in any of the selected studies.
Effect size
We used the standardized mean difference (Cohen’s d) to express effect sizes, reflecting the difference in wellbeing between employed and unemployed individuals. When possible (16 samples), Cohen’s d was computed directly from means, standard deviations and sample sizes of the two groups (i. e., employed and unemployed) by using the formula for independent groups (e. g., Borenstein et al., Citation2009; Schmidt & Hunter, Citation2015).
When primary studies did not include such information, we calculated the effect size from other measures of association (following formulae in Borenstein et al., Citation2009; Lipsey & Wilson, Citation2001; Schmidt & Hunter, Citation2015). Specifically, Cohen’s ds were obtained from correlation coefficients (r, 3 samples), odds ratios (12 samples), frequency distribution tables (2 samples), chi-square statistic (2 samples), and, when none of these statistics was available (11 samples), standardized regression coefficients (Peterson & Brown, Citation2005). We incorporated all the estimates into the meta-analyses, but we systematically performed sensitivity analyses by excluding effect sizes computed from standardized regression coefficients to gauge any possible distortions due to the inclusion of such estimators (Peterson & Brown, Citation2005; Schmidt & Hunter, Citation2015).
Since our primary studies were based on longitudinal designs, time was accounted for in three ways. In some cases, Cohen’s ds were calculated for the multiple years of the data collection and then averaged across these multiple data points (i. e., as repeated cross-sectional effects) to satisfy the assumption of independence between studies. Moreover, when possible, standardized mean differences measured the relationship of being unemployed (e. g., at Time 1) with consequent wellbeing (e. g., at Time 2). Finally, as 4 studies (6 samples) reported differential scores for the wellbeing outcomes for the two groups, we obtained the standardized mean differences in changes in wellbeing between employed and unemployed individuals across time. In other words, these effect sizes capture the longitudinal change in wellbeing due to unemployment and were analysed separately.
Coding process and moderator variables
For each primary study, any statistical information on the association between unemployment and wellbeing that could be used to compute Cohen’s d was coded (see supplementary material B for details). To conduct moderator analysis, we extracted the following information from the primary study, when available: average age, level of education, country, publication year, first year of data collection, and number of measurement occasions (i. e., length of the dataset). Moreover, the HDI and the GII, retrieved from the Human Development Reports (United Nations Development Programme [UNDP], 1990–2019) as well as the OECD Employment Protection Index (OECD, Citation2019) were coded for each country represented in the meta-analysis. The measurement year closest to the year of the data collection in the primary studies was used. In addition, we coded the NTW score for each country (Roex & Rözer, Citation2017; data provided by the authors).
The primary studies were coded independently by two raters to calculate inter-rater reliabilities, which ranged between .55 and 1 (inter-rater reliability mean score = .92 for the effect sizes and = .97 for the continuous moderators; see supplementary material B for details).
Statistical analyses
Meta-analytic procedure
In line with previous meta-analyses on the topic (McKee-Ryan et al., Citation2005; Paul & Moser, Citation2009), we applied the random-effects meta-analytic procedure of Schmidt and Hunter (Citation2015). It has the advantage of estimating the variability across studies in the underlying population parameters, and correcting the meta-analytic effect size not only for sampling error but also for artefacts (in our meta-analyses, measurement error in the outcome variable) that could bias the population estimates.
In total, we performed five primary meta-analyses of Cohen’s d, expressing the standardized mean differences in wellbeing between employed and unemployed individuals.
For each meta-analysis, along with the sample-size weighted average effect size (d), we computed the corrected average effect size adjusted for measurement error (i. e., the estimated true mean differences δ) by using the reliability artefact distributions (Schmidt & Hunter, Citation2015; see supplementary material B for details on unreliability correction). For this purpose, we transformed the primary studies’ effect size (Cohen’s d) into correlations, performed the meta-analyses on the correlations, and transformed the outcomes back to d (Schmidt & Hunter, Citation2015).
The first meta-analysis included all the various wellbeing indicators together (e. g., life satisfaction, mental health symptoms, positive affect). When studies (3 studies, 4 samples) reported effect sizes for more than one wellbeing measure (e. g., life satisfaction and mental health), we calculated composite effect sizes (Schmidt & Hunter, Citation2015, pp. 442, 445). The two following meta-analyses separately explored whether the effect of unemployment varied across the two main wellbeing outcomes of life satisfaction and mental health. Finally, two further meta-analyses (one on overall wellbeing, the other on life satisfaction) were performed for the 4 studies (6 samples) that reported differential scores in wellbeing. We also ran a set of sensitivity analyses and meta-analytic structural equation models, as described below.
Moderator analysis
We applied the 75% rule concerning variance accounted for by artefacts (Schmidt & Hunter, Citation2015) and the width of the 80% credibility intervals (Whitener, Citation1990) as rules of thumb to detect heterogeneity across study results. We performed two sets of moderator analyses.
The first set investigated the role of the categorical moderators using sensitivity analysis to calculate the effect size across studies measuring long-term unemployment and performing subgroup meta-analyses for the moderators gender and country-level characteristics. For the country-level moderators, we first compared European countries (i. e., Germany, Ireland, Portugal, Spain, Sweden, France, Belgium, including the UK and Switzerland) with Australia, Canada, and US. We then considered three additional criteria to group countries and run subsets of meta-analyses. First, based on the median split (= 1.43) of the OECD Employment Protection Index (which varies between 0 = very loose and 5 = very strict), we categorized countries into two groups characterized by i) low levels (Canada, US, Ireland, Switzerland, and Australia); and ii) high levels (Germany, Spain Sweden, Portugal, UK, Belgium, and France) of employment protection. Second, based on the median split (= 6.35) of the NTW score (Roex & Rözer, Citation2017; ranging between 1 = weak and 10 = strong), we identified two groups of countries: i) Canada, Ireland, UK, US, Spain, France, and Belgium scored low on NTW values while ii) Germany, Portugal, Sweden, and Switzerland exhibited strong norms. Third, based on the median split (= .13) of the GII (varying between 0 and 1, with higher values indicative of higher gender inequality), we created two additional groups: i) Sweden, Germany, Switzerland, Spain, Belgium, and France reported low GII scores while ii) Canada, UK, Ireland, US, Australia, and Portugal scored higher on the index.
To examine our continuous moderators (age, level of education, length and starting year of the dataset, publication year of the study, and the country-level indices of HDI, GII, and NTW in their continuous form), the second set of moderator analysis employed the correlational method (Schmidt & Hunter, Citation2015, Chapter 9). The method consists of correlating the moderating variables with the effect sizes of each primary studies and correcting the resulting correlation for sampling error (Schmidt & Hunter, Citation2015).
Meta-analytic structural equation models
Meta-analytic cross-lagged structural equation modelling (SEM; Viswesvaran & Ones, Citation1995; Zyphur et al., Citation2020) was used to test the direction of effects at two distinct points in time between the two constructs of wellbeing and unemployment (i. e., “being unemployed). We specified a set of nested models (i. e., stability, causal, reversed, and reciprocal models) while controlling for their stability over time using model fit indices. The stability model (Mod0) only included the autoregressive paths between each pair of variables across time; unemployment and wellbeing were allowed to covary freely, as our previous meta-analyses reported a significant association between them. The causality model (Mod1) specified the autoregressive paths and the expected causal path from unemployment at T1 to wellbeing at T2. In the reverse causation model (Mod2), a structural path from T1 wellbeing to T2 unemployment was added to Mod0. This reverse causation model tests selection into being unemployed. Finally, the reciprocal causation model (Mod3) estimated all paths as specified in Mod0, Mod1 and Mod2, testing for a reciprocal association between unemployment and wellbeing.
We tested the meta-analytic cross-lagged models in Mplus 8.3 Muthén and Muthén (Citation1998–2017) using maximum likelihood estimation, manifest indicators without correction for measurement error, and the harmonic mean sample size (Viswesvaran & Ones, Citation1995). Model fit was assessed using the common chi-square (χ2) statistic, the comparative fit index (CFI), the root-mean-square error of approximation (RMSEA), and the standardized root-mean-square residual (SRMR; Hu & Bentler, Citation1998; Kline, Citation2016). Adequate fit is associated with a non-significant chi-square (χ2) statistic, although this is influenced by sample size and can easily return a statistically significant result (Bollen, Citation1989); a CFI greater than .90; a RMSEA equal to or smaller than .08; and a SRMR lower than .08 (Hu & Bentler, Citation1998; Kline, Citation2016). To evaluate differences between the four nested models, since the reciprocal model (Mod3) is just-identified and, therefore, the most common indices (i. e., χ2 and CFI) and their differences cannot be computed, we used the −2 log-likelihood difference, which follows the chi-square distribution. A significant difference between two models indicates that the model with more freely estimated parameters is superior to the model in which these parameters were not estimated. Additionally, we compared the Akaike (AIC; Akaike, Citation1974) and Bayesian information criteria (BIC) between the models, with lower values indicating a better fitting model (Kline, Citation2016).
Results
The findings from the general meta-analyses and the related sensitivity analyses are reported in . These results should be interpreted in terms of differences in wellbeing between employed and unemployed individuals. Negative estimated true mean differences (δ) indicate lower wellbeing scores in the unemployed group. All the 95% confidence intervals excluded zero. For reference, Cohen (Citation1988) described standardized mean differences of .20 as small, .50 as medium, and .80 as large effects.
Table 1. Meta-analyses for unemployment and wellbeing.
For the overall meta-analysis using the composite measures of wellbeing, the estimated true mean difference was −.32 (M1 in ) when correcting for unreliability in the wellbeing measures. This reveals a negative association between unemployment and wellbeing; in other words, unemployed individuals reported lower wellbeing than their employed counterparts. The results did not change when we excluded the effect sizes based on standardized regression coefficients (M1b). However, when we omitted two studies (Luo, Citation2020; Van Hoorn & Maseland, Citation2013) given their disproportionate sample and effect sizes, the population mean difference slightly increased to −.38 (M1a).
Results from analyses looking at wellbeing measures separately produced relatively smaller effect sizes. The estimated effect size was −.25 when we exclusively focused on life satisfaction (M2), and marginally dropped to −.22 when we only included indicators of mental health (M3). These results were unaltered when we excluded the samples based on standardized regression coefficients (M2a, M3b) and that used by Schmitz (Citation2011) with a somewhat different operationalization of health (i. e., satisfaction with health; M3a). Moreover, the subset of analyses that distinguished between the GHQ-12 measure of mental health and other measures of depression (see supplementary material B for a list of these measures) indicated a slightly smaller effect size for GHQ-12 (δ = −.19, M3c) than that obtained for the other operationalizations (δ = −.24, M3d).
Finally, some evidence on the negative association between unemployment and wellbeing over time was offered by the two additional meta-analyses of changes in wellbeing and life satisfaction following unemployment. The true mean differences were negative, suggesting that unemployment led to a drop in wellbeing (δ = −.25, M4) and a (slightly larger) drop in life satisfaction (δ = −.28, M5). Therefore, our first hypothesis is supported.
Results from the Moderator Analysis
The variance explained by sampling and measurement error in the wellbeing variable fell below the 75% guideline, and the 80% credibility intervals were wide. Therefore, we proceeded with moderator analyses. The results of the subset meta-analyses for categorical moderators are presented in . In all the subset meta-analyses, a variation in the average effect sizes across the subsets was evident (the confidence intervals did not overlap) and a reduction in the average corrected variance in the subsets was observed, confirming the moderating effects.
Table 2. Meta-analyses of categorical moderators.
Socio-demographic Factors: Gender, Age and Education
A variation in the average effect sizes across the subsets (M6-9) and a reduction in the corrected variance (general meta-analysis: σρ = .033 vs. subset: σρ = .025; life satisfaction meta-analysis: σρ = .031 vs. subset: σρ = .024) supported the moderating role of gender in line with Hypothesis 2 (Schmidt & Hunter, Citation2015).Footnote2 The negative effect of unemployment on wellbeing was stronger for men than for women, when the outcome measure was overall wellbeing (Men: δ = −.72 vs. Women: δ = −.48) and, especially, life satisfaction (Men: δ = −.80 vs. Women: δ = −.52). When we omitted the effect sizes derived from the standardized regression coefficients, the meta-analytic mean differences in wellbeing remained unchanged for women but decreased for men (δ = −.65), although the latter effect was still larger than that for women and for the entire sample (δ = −.32, M1 in ).
Our hypotheses on age (H3) and education (H4) were not supported. Potentially due to the small number of studies (K) on which the correlational analyses were based, age (K = 13) and education (K = 11–15) did not produce statistically significant moderating effects (see Table SM1 in supplementary material B).
Country-level Factors
In terms of our moderators which captured the social and economic development of countries, HDI did not produce statistically significant results (see Table SM1 in supplementary material B). With regards to GII, the subset meta-analyses showed variation in the average effect size across subsets and a lower average corrected variance in the subsets (σρ = .028 vs. σρ = .033 in the general meta-analysis). Counter to our hypothesis (H5), countries with higher GII scores (high levels of gender inequality) reported a smaller meta-analytic effect size (δ = −.23, M11) in comparison to countries with lower levels of gender inequality (δ = −.49, M10a).
The societal norm to work (NTW; Roex & Rözer, Citation2017) also showed a moderating effect (as attested by the variation in the average effect sizes and the lower average corrected variance in the subsets, i. e., σρ = .030 vs. σρ = .033), with results in line with our hypothesis (H6). As expected, the largest meta-analytic mean difference was found in those countries with strongest social expectations related to work (M13), especially when excluding the Luo (Citation2020) study (δ = −1.25, M13a). The additional correlational moderator analyses for GII and NTW, however, generated statistically insignificant results (Table SM1 in supplementary material B).
The subset meta-analysis comparing European and non-European countries also revealed that the average effect size varied from subset to subset and the corrected average variance was lower in the subsets (σρ = .016 vs. σρ = .033). Specifically, the European group showed higher average effect size (δ = −.41, M15) than the non-European cluster (δ = −.25, M14), even when we eliminated two effect sizes based on standardized regression coefficients (δ = −.37, M15a).
The moderating role of the countries’ employment protection index was evident from the variation in the average effect size across subsets (M16-17) and the lower average corrected variance in the subsets (σρ = .002 vs σρ = .033). Countries characterized by high levels of employment protection showed a small meta-analytic mean difference (δ = −.10) when compared to countries with low levels (δ = −.42). However, these differences disappeared when we re-ran the analyses after the exclusion of two studies with large sample and effect sizes (Luo, Citation2020; Oesch & Lipps, Citation2013; see M16a and M17a). Since these latter results may be an indication of the two studies’ dominating effect biasing estimations, for interpretation and comparison purposes, we relied on the meta-analytic effect sizes produced by the exclusion of the two studies. Therefore, we only found partial evidence to support our hypothesis (H7) that the negative effect of unemployment is more pronounced in countries with less flexible labour markets and high degrees of employment protection.
Finally, the correlations of d with publication year, length of the dataset, and first year of data collection with were all non-significant (Table SM1 in supplementary material B).
Duration of Unemployment
As expected, the meta-analysis of measures of long-term unemployment (i. e., being unemployed for more than one or two consecutive years, consistent with the measurement in the primary studies) produced a very large effect size (δ = .76, M18 in ), which was statistically different from the overall meta-analytic effect size (M1 in ; t = 3.1768 (39), p < .01). The effect barely varied when a study with a slightly different measure (i. e., being repeatedly unemployed; Breslin & Mustard, Citation2003) was eliminated (M18a). Although the meta-analytic mean differences decreased both when we excluded two effect sizes derived from standardized regression coefficients (M18b) and when we included a further indicator of unemployment duration (i. e., past unemployment, Clark et al., Citation2001, M18c), they were still larger (δ = .53; .54, respectively) than the one from the overall meta-analysis (δ = .32; M1), confirming the more pronounced negative effect of long-term unemployment on wellbeing.
Results from the Meta- analytic SEM
Meta-analytic SEM requires a complete matrix of meta-analytic correlations. For this purpose, we used the correlational estimate (ρ) resulting from our meta-analysis on all wellbeing measures for the cross-sectional association between unemployment and wellbeing at T1.Footnote3 This was also used to estimate the cross-sectional meta-analytic correlation between the two variables at T2. For the longitudinal relationships, we conducted additional meta-analyses of correlations on those primary studies retrieved from our literature search that reported a correlational estimate of the association between T1 unemployment and T2 wellbeing as well as on an additional pool of articles identified in the previous systematic review (Gedikli et al. (Citation2017)), which included estimates of the effect of wellbeing on unemployment across time. Due to the limited number of studies, we focused on wellbeing without distinguishing between different facets (i. e., life satisfaction vs. mental health). These meta-analytic correlations are presented in . The harmonic mean sample size was 9,402.
Table 3. Meta-analyses of cross-lagged correlations between unemployment and wellbeing.
shows the fit indices of the meta-analytic nested models and the model comparison based on the −2 log-likelihood difference and the AIC and BIC values. Overall, the models fitted the data sufficiently well, although the stability (Mod0) and causality (Mod1) models reported a RMSEA value (= .09) slightly higher than the recommended threshold (Brown & Cudeck, Citation1992; Hu & Bentler, Citation1998).
Table 4. Fit indices and comparison for the different cross-lagged meta-analytic structural equation models.
With regard to model comparison, the causality (Mod1), reversed (Mod2), and reciprocal (Mod3) models showed a better fit to the data than the stability model (Mod0), as the −2 log-likelihood difference was significant and the AIC and BIC values smaller (see, ). Moreover, the reciprocal model (Mod3) fitted the data better than the causal (Mod1) and reversed causation (Mod2) models, indicating that the reciprocal model (Mod3) was the best fitting one. Its standardized parameter estimates are presented in . When taking into account the auto-regressive paths for unemployment and wellbeing across time, unemployment at T1 had a significant negative effect on wellbeing at T2, providing additional support for Hypothesis 1. Additionally, the effect of wellbeing at T1 on unemployment at T2 was significant, negative, and stronger than the one from T1 unemployment on T2 wellbeing. Altogether, Hypotheses 9 and 10 are supported.
Publication bias checks
As recommended by Kepes et al. (Citation2012), publication bias was tested on each of the principal five general meta-analyses based on 10 studies or more as well as separately on the moderator subgroups via multiple techniques. We did not find strong evidence for publication bias, and a detailed description of the methods and results is presented in supplementary material B.
Discussion
We provide an up-to-date quantitative synthesis of the evidence on the link between unemployment and wellbeing alongside the impact of factors which may act to exacerbate or ameliorate the relationship. In line with previous work, our meta-analyses indicated that unemployed people suffer from poorer wellbeing than those who are in employment and, the longer the spell of unemployment, the larger the impact. We found a smaller population effect size for the overall relationship between unemployment and wellbeing (δ = .32) than the one reported by Paul and Moser (Citation2009; δ = .60). This is likely to be related to the different meta-analytic databases used for the analyses, as we only included studies analysing the effect of unemployment and wellbeing over multiple measurement occasions, which may result in smaller correlations among constructs across time when compared to cross-sectional associations.
Moreover, unlike previous meta-analytic work, our data captured the 2008 financial crisis, which profoundly affected most of the countries included in our meta-analytic dataset. The high unemployment rates following the crisis meant unemployment was more widely experienced, perhaps normalizing and attenuating its salience and the associated negative consequences for one’s identity and happiness (Gonza & Burger, Citation2017). Consequently, given that employment has been less strong as a norm, the negative influence of unemployment of wellbeing may have been reduced. Furthermore, with regard to the interpretation of our meta-analytic effect size, Cohen’s (Citation1988) benchmarks for small, medium, and large effects have been found to correspond to approximately the 33rd, 73rd and 90th percentiles in the field of applied psychology, based on an extensive meta-analysis (Bosco et al., Citation2015). Following Bosco and colleagues’ (2015) updated and field-specific classification, our estimated population correlation for the overall unemployment-wellbeing association corresponds to the 50th percentile, indicating a medium effect ( in Bosco et al., Citation2015, p. 436). That is to say, the negative effect of unemployment on wellbeing persists and the insignificant effects noted for the year of data collection and publication year confirm that previous conclusions on the damaging effect of unemployment remain valid, although the underlying dynamics might have changed.
Expanding the previous work, our meta-analysis also offers preliminary evidence on the direction of effects between unemployment and wellbeing. Specifically, the meta-analyses based on a sample of longitudinal studies with information on the changes in wellbeing levels upon unemployment point to the potential causal link that runs from unemployment to wellbeing. These results are further supported by the meta-analytic cross-lagged SEM showing that unemployment leads to a significant decline in individual wellbeing across two distinct measurement occasions. In addition, impaired wellbeing is associated with future state of unemployment with an even stronger effect than the one we observe from unemployment to wellbeing, signalling a reciprocal process and the potential operation of a loss spiral.
Building on COR theory (Hobfoll, Citation1989), it can be argued that unemployed people invest in resources (such as energy and time) while searching for jobs. As part of the job search process, they may use up these resources without gaining any new resource in the meantime. Financial hardship and losing social contacts, the key resources, and components of manifest and latent functions of employment as introduced in Jahoda’s model, could be expected to increase fatigue during the job search (Lim et al., Citation2016). These result in a further drop in wellbeing. The unemployed, thereby, enter a loss spiral of resources; which is a vicious cycle – unless people have personal resources such as optimism which may help them to “break” the cycle or at least buffer against the negative effects on wellbeing. Additionally, poor performance during job interviews or stigmatization of individuals with mental health problems as low-quality job applicants can be amongst the potential factors hindering employment trajectories (Baldwin & Marcus, Citation2014; Kiely & Butterworth, Citation2014). In fact, poor wellbeing can hinder the job search process of the unemployed by influencing their effort, motivation, and job search self-efficacy (Kim et al., Citation2019). Furthermore, job seekers with mental health problems may bear a double stigma (i. e., being unemployed and suffering from mental illness) and experience discrimination, lowering their chances of being employed or re-employed. Indeed, the intersection of unemployment and mental health problems has been found to undermine an individual’s job search self-efficacy and worsen job seeking outcomes, contributing to long-term unemployment (Staiger et al., Citation2018).
Overall, these considerations help explain the reverse causation effect into unemployment emerging from our cross-lagged SEM (i. e., the selection effect from T1 wellbeing to T2 unemployment) – an effect stronger than that of causation. Interestingly, this finding contrasts with the weak selection effects previously reported by Paul and Moser (Citation2009). Such differences can be attributed to the diverse pool of primary studies underlying our analyses and, especially, the different analytical techniques used, as our cross-lagged MA-SEM analyses did not focus on transitions between employment and unemployment but on the pattern of relationships between “being unemployed” and wellbeing over time.
Although we must exercise caution in interpreting these initial results, as we acknowledge in the study limitations, this is the first meta-analysis to use cross-lagged meta-analytic SEMs to investigate the causal processes between unemployment and wellbeing. Cross-lagged SEMs are considered dynamic models and preferable to static ones since they facilitate testing of the direction of causal influence among variables by allowing the causal variables to precede the outcomes via lagged predictors (Zyphur et al., Citation2020). Moreover, they permit the examination of alternative models, including the analysis of bidirectional effects between the constructs of interest. Unlike previous work, which relied on a set of meta-analytic comparisons between distinct groups of individuals to examine the selection effect into unemployment (as in Paul & Moser, Citation2009), the cross-lagged meta-analytic models allowed us to specify the direction of effects among the constructs of (un)employment and wellbeing and test formally the reciprocal relationship between them across time in a structured model, effectively including causation and selection in the same model. Therefore, the meta-analytic models extend the conclusions of previous meta-analyses, which assessed the unemployment-wellbeing longitudinal association by focusing on one direction at time (i. e., the causation or selection effect separately).
Moreover, compared to the previous meta-analyses which tended to base their discussion primarily on mental health outcomes, focusing on a wider set of wellbeing outcomes enabled a more thorough examination of the damaging effect of unemployment. Our findings were robust to the different wellbeing measures used; unemployment had an equally strong negative association with life satisfaction when compared to several mental health measures. This indicated that, as with mental health outcomes, unemployment has damaging effects on measures of cognitive and affective evaluation of one’s life. These results reinforce the importance of considering multi-faceted operationalizations of wellbeing (Grant et al., Citation2007), centred not only on mental health symptoms (e. g., anxiety, depression, indicators of cognitive functioning) but also on people’s evaluations of their lives (i. e., subjective wellbeing and life satisfaction in particular). More comprehensive measurements of the adverse effect of unemployment on wellbeing outcomes can inform policies and interventions targeted at different groups and wellbeing outcomes (Hicks et al., Citation2013).
We were able to shed light on several factors which might influence the link between unemployment and wellbeing. One of the most important findings is the role of gender. We found that the negative association between unemployment and wellbeing is stronger for men than women. This difference in effect sizes is particularly pronounced for life satisfaction, implying men’s evaluation of their subjective wellbeing is affected more strongly by unemployment, leading to a lower overall assessment of their life. Societal norms and expectations related to gender roles and greater non-employment roles for women may help to explain this effect. Indeed, as illustrated by our additional country-level moderator of NTW index, the negative role of unemployment on wellbeing was shown to be particularly significant in countries with stronger societal expectations to work. Given the societal expectations attaching work to male identity, our country-level finding could be interpreted as a further indication of the role of societal expectations contributing to a more pronounced negative impact for men.
Our results showed that unemployment is more damaging in countries that are characterized by low GII, indicating lower levels of gender inequalities. While from a social and economic development perspective, this result was unexpected, it may speak to the role of underlying gender roles and the meaning of work in modern societies. It is reasonable to assume that market-oriented work will be more common for women in more gender-equal countries where their access to education, health and the labour market are better and to expect a less pronounced gendered division of labour at home and in the labour market. Therefore, like men, work could be more central to how women define themselves, making unemployment a more stressful event. It might follow that the negative effect might be less strong for men in countries with lower gender inequalities, as they are less likely to confront with norms/societal expectations that regard them as the “provider” of the family. Due to limited sample size, we were not able to explore the moderating effect of GII separately for men and women. Therefore, we do not know whether our result reflects the dominating effect of women’s experiences.
Although we were not able to investigate this three-way interaction, the more pronounced negative effect of unemployment in countries characterized by more gender equal social and economic development is a valuable finding which could be interpreted on the grounds of meaning of work in more gender equal, modern societies. Recent work by (Longhi et al (Citation2018)) indicates that women, as well as men, who hold more gender egalitarian attitudes are more attached to the labour market and, experience larger drop in their life satisfaction upon unemployment when compared to those holding more traditional attitudes. Our result might reflect the role of these attitudes, given that they will be more common in countries with low GII.
Finally, in line with previous research (McKee-Ryan et al., Citation2005; Paul & Moser, Citation2009), we also investigated the influence of labour market structures on the role of unemployment on wellbeing. The negative impact of unemployment on wellbeing was more pronounced in Europe than in North America/Australia, but was similar across countries with differing levels of employment protection. Therefore, we only found partial evidence for our prior hypothesis that unemployment is a more damaging experience in less flexible labour markets.
Our results reinforce existing evidence on the negative impact of unemployment upon wellbeing and show that this holds for the young/old, better/less well educated in richer/poorer developed economies. In other words, unemployment is a bad experience for everyone and, aside from the role of gender, work norms and employment protection, we found very little evidence that the negative effect can be attenuated; long-term unemployment is even worse. These findings have important practical implications, they suggest that creating good quality, sustainable jobs are crucial to people’s wellbeing. While the interventions aimed at mitigating the negative effect on wellbeing should be targeted at all those experiencing unemployment, priority could be given to the long term unemployed who suffer the most. In parallel, our results signal a reciprocal relationship between unemployment and wellbeing and a loss spiral of unemployment. Thus, policies should maintain and supplement resources for individuals; for example, by supporting more effective job search techniques for the unemployed, to prevent those searching becoming disheartened by the lack of positive outcomes. In this sense, provision of financial and emotional support and, promoting social activities enabling creation of support networks and career contacts are essential in assisting individuals to cope with unemployment and enabling a successful transition to employment. Although indirectly, our results point to the changing gender roles, increasing the importance of work for women. Therefore, policies supporting women in employment are crucial. These include affordable childcare, work and family reconciliation policies along with parental leave policies fostering an equal distribution of labour at home and in the labour market. Gender-equal policies could also encourage men to take on work outside of employment traditionally undertaken by women and not to consider unemployment as an identity threat.
Limitations and future research
Amongst the limitations of our meta-analysis, most relate to data constraints. Some potentially crucial moderators, such as education and age, did not produce statistically significant effects, possibly due to the limited number of studies providing relevant information. Similarly, we were not able to identify further aspects such as marital status, social status and personality traits. The data limitations also prevented us from differentiating between different wellbeing measures within our moderator analyses. These factors should be explored in future work should more data become available.
Further limitations pertain to the test of our structural models. First, as seen in previous meta-analyses, the models are based on a correlation (rather than covariance) matrix, which can cause an overestimation of standard errors; this, however, leads to a more conservative test of parameters (Cudeck, Citation1989). Furthermore, we need to exercise caution when drawing causal conclusions based on a correlational matrix. Additionally, the primary longitudinal studies which provided the basis for our structural models (i. e., the meta-analyses presented in ) were based on various time lags between the measurement points of unemployment and wellbeing, and we could not control for such differences. This prevents us from identifying an optimal time point to examine the relationship between the two variables and from drawing any conclusions on the short- or long-term effects of unemployment on wellbeing. Moreover, since we could not find primary data on the cross-sectional association between the two variables at T2, we imputed it with the estimate of the T1 cross-sectional meta-analytic correlation (as also seen in other studies, e. g., Martin et al., Citation2016). This choice was justified by the arbitrariness of the time lag between unemployment and wellbeing across the primary studies. To summarize, we call for future longitudinal studies to verify the reciprocal causal associations between unemployment and wellbeing, to examine the temporal characteristics of this relationship by investigating how long it takes to the wellbeing detrimental effects of unemployment to unfold and even prompt a potential loss spiral.
Finally, our meta-analysis is based on primary studies conducted in developed countries. Therefore, a cautionary note should be introduced when applying the findings to less developed countries because of different institutions. For example, informal employment tends to be much more important in less developed countries and could alter the meaning and consequences of unemployment.
Conclusion
Overall, based on an up-to-date synthesis of the literature, strong evidence remains that unemployment is damaging to wellbeing, and we now have evidence on a new set of policy-relevant moderators (gender, work norms and employment protection). Promoting work for the unemployed is crucial for improving individuals’ wellbeing and priority might be given to long-term unemployed where the loss in wellbeing is greatest. However, the link between unemployment and wellbeing is not so straightforward and there are wider social and institutional factors that need to be taken into account. These elements are not isolated from each other and calls for a multidimensional, coordinated approach and a recognition that the experience of unemployment may be more damaging in some cases and more support for the unemployed is needed both in terms of coping and in getting back to work.
Supplemental Material
Download MS Word (112.2 KB)Acknowledgments
This work is part of the Work, Learning and Well-being programme of the What Works Well-being Centre (https://whatworkswellbeing.org/). We acknowledge the support of our funding partners, administered through Economic and Social Research Council Grant ES/N003586/1. We would like to thank Balazs Stadler for helping with the coding of the data.
Disclosure statement
No potential conflict of interest was reported by the author (s).
Supplementary material
Supplemental data for this article can be accessed online at https://doi.org/10.1080/1359432X.2022.2106855
Additional information
Funding
Notes
1. This literature was relatively sparse prior to 1990 – very few studies would have met our inclusion criteria discussed below. However, the combination of the unemployment crisis in developed economies in the 1980s, the availability of longitudinal data over a necessary timeframe and the development of relevant statistical techniques, led to the rapid development of the literature which justifies our choice of 1990 as a start point for this analysis.
2. σρ denotes the corrected variance resulting from the meta-analyses of correlations (see Method Section, Statistical Analyses, Meta-Analytic Procedure).
3. As explained in the Meta-analytic Procedure section, our meta-analyses were performed on correlations and the outcomes were transformed back to Cohen’s d, as suggested by Schmidt and Hunter (Citation2015). Therefore, we directly used the results of the meta-analysis using correlations as effect size.
References
- *Binder, M., & Coad, A. (2015). Unemployment impacts differently on the extremes of the distribution of a comprehensive well- being measure. Applied Economics Letters, 22(8), 619– 627. https://doi.org/10.1080/13504851.2014.962219
- *Blasco, S., & Brodaty, T. (2016). Chômage et santé mentale en France. Économie et Statistique, 486(1), 17– 44. https://doi.org/10.3406/estat.2016.10688
- *Booker, C. L., & Sacker, A. (2012). Psychological well- being and reactions to multiple unemployment events: Adaptation or sensitisation? Journal of Epidemiology and Community Health, 66(9), 832–838 doi:https://doi.org/10.1136/jech.2010.126755 .
- *Boyce, C. J., Wood, A. M., & Brown, G. D. A. (2010). The dark side of conscientiousness: conscientious people experience greater drops in life satisfaction following unemployment. Journal of Research in Personality, 44(4), 535– 539. https://doi.org/10.1016/j.jrp.2010.05.001
- *Breslin, F. C., & Mustard, C. (2003). Factors influencing the impact of unemployment on mental health among young and older adults in a longitudinal, population- based survey. Scandinavian Journal of Work and Environmental Health, 29(1), 5– 14. https://doi.org/10.5271/sjweh.698
- *Carroll, N. (2007). Unemployment and psychological well- being. Economic Record, 83(262), 287– 302. https://doi.org/10.1111/j.1475-4932.2007.00415.x
- *Clark, A. E. (2003). Unemployment as a social norm: psychological evidence from panel data. Journal of Labor Economics, 21(2), 323– 351. https://doi.org/10.1086/345560
- *Clark, A. E., Flèche, S., Layard, R., Powdthavee, N., & Ward, G. (2018). The origins of happiness: The science of well-being over the life course. Princeton University Press.
- *Dockery, A. M. (2005). The happiness of young Australians: empirical evidence on the role of labour market experience. Economic Record, 81(255), 322– 335. https://doi.org/10.1111/j.1475-4932.2005.00272.x
- *Dooley, D., & Catalano, R. (1994). Depression and unemployment: panel findings from the epidemiologic catchment area study. American Journal of Community Psychology, 22(6), 745. https://doi.org/10.1007/BF02521557
- *Dooley, D., & Prause, J. (1995). Effect of unemployment on school leavers’ self-esteem. Journal of Occupational and Organizational Psychology, 68(3), 177– 192. https://doi.org/10.1111/j.2044-8325.1995.tb00580.x
- *Fernández-Valera, M. M., Soler-Sánchez, M.-I., García- Izquierdo, M., & Meseguer de Pedro, M. (2019). Personal psychological resources, resilience and self- efficacy and their relationship with psychological distress in situations of unemployment. Revista de Psicología Social, 34(2), 331– 353. https://doi.org/10.1080/02134748.2019.1583513
- *Ferreira, J. A., Reitzle, M., Lee, B., Freitas, R. A., Santos, E. R., Alcoforado, L., & Vondracek, F. W. (2015). Configurations of unemployment, re- employment, and psychological well- being: A longitudinal study of unemployed individuals in Portugal. Journal of Vocational Behavior, 91, 54– 64. https://doi.org/10.1016/j.jvb.2015.09.004
- *Green, F. (2011). Unpacking the misery multiplier: How employability modifies the impacts of unemployment and job insecurity on life satisfaction and mental health. Journal of Health Economics, 30(2), 265– 276. https://doi.org/10.1016/j.jhealeco.2010.12.005
- *Griep, Y., Hyde, M., Vantilborgh, T., Bidee, J., De Witte, H., & Pepermans, R. (2015). Voluntary work and the relationship with unemployment, health, and well- being: A two- year follow- up study contrasting a materialistic and psychosocial pathway perspective. Journal of Occupational Health Psychology, 20(2), 190– 204. https://doi.org/10.1037/a0038342
- *Hammarström, A., & Janlert, U. (1997). Nervous and depressive symptoms in a longitudinal study of youth unemployment – Selection or exposure? Journal of Adolescence, 20(3), 293– 305. https://doi.org/10.1006/jado.1997.0086
- *Hammer, T. (1993). Unemployment and mental health among young people: A longitudinal study. Journal of Adolescence, 16(4), 407– 420. https://doi.org/10.1006/jado.1993.1033
- *Huegaerts, K., Puig-Barrachina, V., & Vanroelen, C. (2017). The mental health of unemployed brussels youth: The role of social and material resources. Archives of Public Health, 75(1), 1– 11. https://doi.org/10.1186/s13690-017-0187-7
- *Kassenboehmer, S. C., & Haisken-DeNew, J. P. (2009). You’re fired! The causal negative effect of entry unemployment on life satisfaction. Economic Journal, 119(536), 448– 462. https://doi.org/10.1111/j.1468-0297.2008.02246.x.
- *Latif, E. (2010). Crisis, unemployment and psychological wellbeing in Canada. Journal of Policy Modeling, 32(4), 520– 530. https://doi.org/10.1016/j.jpolmod.2010.05.010
- *Lee, J. O., Jones, T. M., Yoon, Y., Hackman, D. A., Yoo, J. P., & Kosterman, R. (2019). Young adult unemployment and later depression and anxiety: does childhood neighbourhood matter? Journal of Youth and Adolescence, 48(1), 30– 42. https://doi.org/10.1007/s10964-018-0957–8
- *Luo, J. (2020). A pecuniary explanation for the heterogeneous effects of unemployment on happiness. Journal of Happiness Studies, 21(7), 2603– 2628. https://doi.org/10.1007/s10902-019-00198-4
- *Marcus, J. (2013). The effect of unemployment on the mental health of spouses – evidence from plant closures in Germany. Journal of Health Economics, 32(3), 546– 558. https://doi.org/10.1016/j.jhealeco.2013.02.004
- *Mossakowski, K. N. (2009). The influence of past unemployment duration on symptoms of depression among young women and men in the United States. American Journal of Public Health, 99(10), 1826– 1832. https://doi.org/10.2105/AJPH.2008.152561
- *Mulligan, K., & Zieba, M. (2020). Well-being and unemployment during the Great Recession: An empirical analysis across UK local authority districts. Regional Studies, Regional Science, 7(1), 267– 287. https://doi.org/10.1080/21681376.2020.1784779
- *Oesch, D., & Lipps, O. (2013). Does unemployment hurt less if there is more of it around? A panel analysis of life satisfaction in Germany and Switzerland. European Sociological Review, 29(5), 955– 967. https://doi.org/10.1093/esr/jcs071
- *Powdthavee, N. (2012). Jobless, friendless and broke: what happens to different areas of life before and after unemployment? Economica, 79(315), 557– 575. https://doi.org/10.1111/j.1468-0335.2011.00905.x
- *Rodriguez, E., Allen, J. A., Frongillo, E. A., & Chandra, P. (1999). Unemployment, depression, and health: A look at the African- American community. Journal of Epidemiology and Community Health, 53(6), 335– 342. https://doi.org/10.1136/jech.53.6.335
- *Schmitz, H. (2011). Why are the unemployed in worse health? The causal effect of unemployment on health. Labour Economics, 18(1), 71– 78. https://doi.org/10.1016/j.labeco.2010.08.005
- *Strandh, M., Novo, M., & Hammarström, A. (2011). Mental health among the unemployed and the unemployment rate in the municipality. European Journal of Public Health, 21(6), 799– 805. https://doi.org/10.1093/eurpub/ckq147
- *Strandh, M., Hammarström, A., Nilsson, K., Nordenmark, M., & Russel, H. (2013). Unemployment, gender and mental health: The role of the gender regime. Sociology of Health & Illness, 35(5), 649– 665. https://doi.org/10.1111/j.1467-9566.2012.01517.x
- *Taris, T. W. (2002). Unemployment and mental health: A longitudinal perspective. International Journal of Stress Management, 9(1), 43– 57. https://doi.org/10.1023/A:1013067101217
- *Urbanos-Garrido, R. M., & Lopez-Valcarcel, B. G. (2015). The influence of the economic crisis on the association between unemployment and health: an empirical analysis for Spain. The European Journal of Health Economics, 16(2), 175– 184. https://doi.org/10.1007/s10198-014-0563-y
- *van Hoorn, A., & Maseland, R. (2013). Does a protestant work ethic exist? Evidence from the well- being effect of unemployment. Journal of Economic Behavior & Organization, 91, 1– 12. https://doi.org/10.1016/j.jebo.2013.03.038
- Achdut, N., & Refaeli, T. (2020). Unemployment and psychological distress among young people during the covid- 19 pandemic: psychological resources and risk factors. International Journal of Environmental Research and Public Health, 17(19), 7163. https://doi.org/10.3390/ijerph17197163
- Akaike, H. (1974). A new look at the statistical model identifícation. IEEE Transactions on Automatic Control, 19(6), 716– 723. https://doi.org/10.1109/TAC.1974.1100705
- Baldwin, M. L. M., & Marcus, S. C. (2014). The impact of mental and substance- use disorders on employment transitions. Health Economics, 23(3), 332– 344. https://doi.org/10.1002/hec.2936
- Blanchflower, D. G., & Oswald, A. J. (2004). Well-being over time in Britain and the USA. Journal of Public Economics, 88(7–8), 1359– 1386. https://doi.org/10.1016/S0047-2727(02)00168-8
- Bollen, K. A. (1989). Structural equations with latent variables. Wiley & Sons.
- Borenstein, M., Hedges, L. V., Higgins, J. P. T., & Rothstein, H. R. (2009). Introduction to meta-analysis. Wiley.
- Bosco, F. A., Aguinis, H., Singh, K., Field, J. G., & Pierce, C. A. (2015). Correlational effect size benchmarks. Journal of Applied Psychology, 100(2), 431– 449. https://doi.org/10.1037/a0038047
- Brown, M. W., & Cudeck, R. C. (1992). Alternative way of assessing model fit. Sociological Methods & Research, 21(2), 230– 258. https://doi.org/10.1177/0049124192021002005
- Carstensen, L. L. (2006). The influence of a sense of time on human development. Science, 312(5782), 1913– 1915. https://doi.org/10.1126/science.1127488
- Cassidy, T. (2001). Self-categorization, coping and psychological health among unemployed mid- career executives. Counselling Psychology Quarterly, 14(4), 303– 315. https://doi.org/10.1080/09515070110102800
- Clark, A. E., Georgellis, Y., & Sanfey, P. (2001). Scarring: The psychological impact of past unemployment. Economica, 68(270), 221– 241. https://doi.org/10.1111/1468-0335.00243
- Clark, A. E., & Clark, A. E. (2006). A note on unhappiness and unemployment duration. Applied Economics Quarterly, 52(4), 291– 308.
- Clark, A. E., Knabe, A., & Ratzel, S. (2010). Boon or bane? Others’ unemployment, well-being and job insecurity. Labour Economics, 17(1), 52– 61. https://doi.org/10.1016/j.labeco.2009.05.007
- Cohen, J. (1988). Statistical power analysis for the behavioural sciences (2nd ed.). Erlbaum.
- Cudeck, R. (1989). Analysis of correlation matrices using covariance structure models. Psychological Bulletin, 105(2), 317– 327. https://doi.org/10.1037/0033-2909.105.2.317
- Daniels, K., Connolly, S., Ogbonnaya, C., Tregaskis, O., Bryan, M. L., Robinson-Pant, A., & Street, J. (2018). Democratisation of wellbeing: stakeholder perspectives on policy priorities for improving national wellbeing through paid employment and adult learning. British Journal of Guidance & Counselling, 46(4), 492– 511. https://doi.org/10.1080/03069885.2017.1408776
- Diener, E. (1984). Subjective well- being. Psychological Bulletin, 95(3), 542– 575. https://doi.org/10.1037//0033-2909.95.3.542
- Fernández, R., & Fogli, A. (2009). Culture: an empirical investigation of beliefs, work, and fertility. American Economic Journal: Macroeconomics, 1(1), 146– 177. https://doi.org/10.1257/mac.1.1.14
- Folkman, S., & Lazarus, R. S. (1985). If it changes it must be a process: A study of emotion and coping during three stages of a college examination. Journal of Personality and Social Psychology, 48(1), 150– 170. https://doi.org/10.1037/0022-3514.48.1.150
- Fryer, D., & Payne, R. (1984). Proactive behaviour in unemployment: Findings and implications. Leisure Studies, 3(3), 273– 295. https://doi.org/10.1080/02614368400390231
- Fryer, D. M. (1992). Psychological or material deprivation: Why does unemployment have mental health consequences? In E. McLauglin (Ed.), Understanding unemployment: new perspectives on active labour market policies. Routledge.
- Gedikli, C., Bryan, M., Connolly, S., Daniels, K., Watson, D., Semkina A., & Vaughn, O. (2017). Worklessness, exits from worklessness and wellbeing: a systematic review, What Works Centre for Wellbeing. https://www.whatworkswellbeing.org/product/retirement-and-wellbeing/ and https://www.whatworkswellbeing.org/product/unemployment-reemployment-and-wellbeing/
- Gedikli, C., Bryan, M., Connolly, S., Daniels, K., Watson, D., Etuknwa A., & Vaughn, O. (2018). Finding and keeping work: Impact of low wellbeing. What Works Centre for Wellbeing. https://whatworkswellbeing.org/resources/finding-and-keeping-work-impact-of-low-wellbeing/
- Goldberg, D. (1972). The detection of psychiatric illness by questionnaire. Oxford University Press.
- Gonza, G., & Burger, A. (2017). Subjective well- being during the 2008 economic crisis: identification of mediating and moderating factors. Journal of Happiness Studies, 18(6), 1763– 1797. https://doi.org/10.1007/s10902-016-9797-y
- Grant, A. M., Christianson, M. K., & Price, R. H. (2007). Happiness, health, or relationships? Managerial practices and employee well- being tradeoffs. Academy of Management Perspectives, 21(3), 51– 63. https://doi.org/10.5465/amp.2007.26421238
- Hahn, E., Spech, J., Gottschling, J., & Spinath, F. M. (2015). Coping with unemployment: the impact of unemployment duration and personality on trajectories of life satisfaction. European Journal of Personality, 29(6), 635– 646. https://doi.org/10.1002/per.2034
- Hamilton, V. L., Hoffman, W. S., Broman, C. L., & Rauma, D. (1993). Unemployment, distress and coping: A panel of autoworkers. Journal of Personality and Social Psychology, 65(2), 234– 247. https://doi.org/10.1037/0022-3514.65.2.234
- Haslam, A. S., Jetten, J., Postmes, T., & Haslam, C. (2009). Social identity, health and well- being: an emerging agenda for applied psychology. Applied Psychology: An International Review, 58(1), 1– 23. https://doi.org/10.1111/j.1464-0597.2008.00379.x
- Hicks, S., Tinkler, L., & Allin, P. (2013). Measuring subjective well- being and its potential role in policy: perspectives from the UK office for national statistics. Social Indicators Research, 114(1), 73– 86. https://doi.org/10.1007/s11205-013-0384-x
- Hobfoll, S. E. (1989). Conservation of resources. A new attempt at conceptualizing stress. The American Psychologist, 44(3), 513– 524. https://doi.org/10.1037/0003-066X.44.3.513
- Hobfoll, S. E. (2001). The influence of culture, community, and the nested- self in the stress process: Advancing conservation of resources theory. Applied Psychology: An International Review, 50(3), 337– 370. https://doi.org/10.1111/1464-0597.00062
- Hobfoll, S. E., Halbesleben, J., Neveu, J.-P., & Westman, M. (2018). Conservation of resources in the organizational context: the reality of resources and their consequences. Annual Review of Organizational Psychology and Organizational Behavior, 5(1), 103– 128. https://doi.org/10.1146/annurev-orgpsych-032117-104640
- Hogg, M. A., & Abrams, D. (1988). Social identifications: A social psychology of intergroup relations and group processes. Routledge.
- Hogg, M. A., Terry, D. J., & White, K. M. (1995). A tale of two theories: A critical comparison of identity theory with social identity theory. Social Psychology Quarterly, 58(4), 25– 26. https://doi.org/10.2307/2787127
- Hornsey, M. J. (2008). Social identity theory and self- categorization theory: A historical review. Social and Personality Psychology Compass, 2(1), 204– 222. https://doi.org/10.1111/j.1751-9004.2007.00066.x
- Howley, P., & Knight, S. (2021). Staying down with the joneses: differences in the psychological cost of unemployment across neighbourhoods Work. Employment and Society. https://doi.org/10.1177/09500170211003483
- Hu, L. T., & Bentler, P. M. (1998). Fit indices in covariance structure modelling: sensitivity to underparameterized model misspecification. Psychological Methods, 3(4), 424– 453. https://doi.org/10.1037/1082-989X.3.4.424
- Ilic, N., Henderson, H., Henderson, C., Evans-Lacko, S., & Thornicroft, G. Attitudes towards mental illness. In R. Craig, R. Craig, & R. Craig (Eds.), Health survey for England 2014: health, social care and lifestyles. London: Health and Social Care Information Centre.
- International Labor Organization. (2017). Towards a better future for women and work: Voices of women and men. http://www.ilo.org/global/about-the-ilo/newsroom/news/WCMS_545963/lang–en/index.htm?utm_content=buffer40605&utm_medium=social&utm_source=twitter.com&utm_campaign=buffer
- Jahoda, M. (1981). Work, employment, and unemployment: values, theories, and approaches in social research. American Psychologist, 36(2), 184–191 doi:https://doi.org/10.1037/0003-066X.36.2.184. .
- Jahoda, M. (1982). Employment and unemployment. A social-psychological analysis. Cambridge University Press.
- Kanfer, R., Wanberg, C. R., & Kantrowitz, T. M. (2001). Job search and employment: A personality- motivational analysis and meta- analytic review. Journal of Applied Psychology, 86(5), 837– 855. https://doi.org/10.1037/0021-9010.86.5.837
- Kepes, S., Banks, G. C., McDaniel, M., & Whetzel, D. L. (2012). Publication bias in the organizational sciences. Organizational Research Methods, 15(4), 624– 662. https://doi.org/10.1177/1094428112452760
- Kiely, K. M., & Butterworth, P. (2014). Mental health selection and income support dynamics: Multiple spell discrete- time survival analyses of welfare receipt. Journal of Epidemiology and Community Health, 68(4), 349– 355. https://doi.org/10.1136/jech-2013-203179
- Kim, J. G., Kim, H. J., & Lee, K. H. (2019). Understanding behavioural job search self- efficacy through the social cognitive lens: A meta- analytic review. Journal of Vocational Behavior, 112, 17– 34. https://doi.org/10.1016/j.jvb.2019.01.004
- Kinicki, A. J., & Latack, J. C. (1990). Explication of the construct of coping with involuntary job loss. Journal of Vocational Behaviour, 36(3), 339– 360. https://doi.org/10.1016/0001-8791(90)90036-2.
- Kline, R. B. (2016). Principles and practice of structural equation modelling (4th ed.). Guilford Press.
- Knabe, A., Schöb, R., & Weimann, J. (2016). Partnership, gender, and the well- being cost of unemployment. Social Indicators Research, 129(3), 1255– 1275. https://doi.org/10.1007/s11205-015-1167-3
- Lazarus, R., & Folkman, S. (1984). Stress, appraisal and coping. Springer.
- Leana, C. R., & Feldman, D. C. (1988). Individual responses to job loss: perceptions, reactions and coping behaviours. Journal of Management, 14(3), 375– 389. https://doi.org/10.1177/014920638801400302
- Leana, C. R., & Feldman, D. C. (1990). Individual responses to job loss: empirical findings from two field studies. Human Relations, 43(11), 1155– 1181. https://doi.org/10.1177/001872679004301107.
- Lim, V. K. G., Chen, D., Aw, S. S. Y., & Tan, M. (2016). Unemployed and exhausted? Job- search fatigue and re- employment quality. Journal of Vocational Behavior, 92, 68– 78. http://dx.doi.org/10.1016/j.jvb.2015.11.003.
- Lipsey, M. W., & Wilson, D. B. (2001). Practical meta-analysis. Sage.
- Longhi, S., Nandi, A., Bryan, M., Connolly, S., Gedikli, C. (2018). Unhappiness in unemployment – is it the same for everyone? Working Papers 2018007, The University of Sheffield, Department of Economics.
- Lucas, R. E., Clark, A. E., Georgellis, Y., & Diener, E. (2004). Unemployment alters the set point for life satisfaction. Psychological Science, 15(1), 8– 13. https://doi.org/10.1111/j.0963-7214.2004.01501002.x
- Martin, R., Guillaume, Y., Thomas, G., Lee, A., & Epitropaki, O. (2016). Leader–member exchange (LMX) and performance: A meta‐analytic review. Personnel Psychology, 69(1), 67– 121. https://doi.org/10.1111/peps.12100
- McFadyen, R. G. (1995). Coping with threatened identities: unemployed people’s self-categorizations. Current Psychology: A Journal for Diverse Perspectives on Diverse Psychological Issues, 14(3), 233– 256. https://doi.org/10.1007/BF02686910
- McKee-Ryan, F. M., & Kinicki, A. J. (2002). Coping with job loss: A life- facet Perspective. In C.L. Cooper & I.T. Robertson, (Eds.), International Review of Industrial and Organizational Psychology, 17, 1– 29. https://doi.org/10.1002/9780470696392.ch1
- McKee-Ryan, F., Song, Z., Wanberg, C. R., & Kinicki, A. J. (2005). Psychological and physical well- being during unemployment: A meta- analytic study. The Journal of Applied Psychology, 90(1), 53– 76. https://doi.org/10.1037/0021-9010.90.1.53
- Merino, M. D., Privado, J., & Arnaiz, R. (2019). Is there any relationship between unemployment in young graduates and psychological resources? An empirical research from the conservation of resources theory. Journal of Work and Organizational Psychology, 35 1 , 1– 8. https://doi.org/10.5093/jwop2019a1
- Murphy, G. C., & Athanasou, J. A. (1999). The effect of unemployment on mental health. Journal of Occupational and Organizational Psychology, 72(1), 83– 99. https://doi.org/10.1348/096317999166518
- Muthén, L., & Muthén, B. O. (1998-2017). Mplus user’s guide. Muthén and Muthén.
- Organisation for Economic Co-operation and Development (OECD) (2019). OECD Indicators of Employment Protection. http://www.oecd.org/employment/emp/oecdindicatorsofemploymentprotection.htm
- Paul, K. I., & Moser, K. (2006). Incongruence as an explanation for the negative mental health effects of unemployment: meta- analytic evidence. Journal of Occupational and Organizational Psychology, 79(4), 595– 621. https://doi.org/10.1348/096317905X70823
- Paul, K. I., & Moser, K. (2009). Unemployment impairs mental health: meta- analyses. Journal of Vocational Behavior, 74(3), 264– 282. https://doi.org/10.1016/j.jvb.2009.01.001
- Peterson, R. A., & Brown, S. P. (2005). On the use of beta coefficients in meta- analysis. Journal of Applied Psychology, 90(1), 175– 181. https://doi.org/10.1037/0021-9010.90.1.175
- Roex, K. L. A., & Rözer, J. J. (2017). The social norm to work and the well- being of the short- and long- term unemployed. Social Indicators Research, 139(3), 1037– 1064. https://doi.org/10.1007/s11205-017-1723-0
- Ryan, R. M., & Deci, E. D. (2001). On happiness and human potentials: A review of research on hedonic and eudaimonic well- being. Annu. Rev. Psychol, 52(1), 141– 166. https://doi.org/10.1146/annurev.psych.52.1.141
- Schmidt, F. L., & Hunter, J. E. (2015). Methods of meta-analysis. correcting error and bias in research findings (3rd ed.). Sage.
- Schöb, R. (2013). Unemployment and Identity. CESifo Economic Studies, 59(1), 149– 180. https://doi.org/10.1093/cesifo/ifs040
- Schöb, R. (2021). Labor market policies, unemployment, and identity. IZA World of Labor, 2021, 270v2. https://doi.org/10.15185/izawol.270.v2
- Shojania, K. G., Sampson, M., Ansari, M. T., Ji, J., Doucette, S., & Moher, D. (2007). How quickly do systematic reviews go out of date? A survival analysis. Annals of Internal Medicine, 147, 224– 233. https://doi.org/10.7326/0003-4819-147-4-200708210-00179
- Staiger, T., Waldmann, T., Oexle, N., Wigand, M., & Rüsch, N. (2018). Intersections of discrimination due to unemployment and mental health problems: the role of double stigma for job- and help- seeking behaviours. Social Psychiatry and Psychiatric Epidemiology, 53(10), 1091– 1098. https://doi.org/10.1007/s00127-018-1535-9
- Tajfel, H. (1981). Human groups and social categories. Cambridge University Press. Cambridge.
- Tajfel, H., & Turner, J. C. (1986). The social identity theory of intergroup behaviour. In S. Worchel & W. G. Austin (Eds.), Psychology of intergroup relations (pp. 7– 24). Nelson-Hall.
- Turner, J. C., & Reynolds, K. J. (2010). The story of social identity. In T. Postmes & N. R. Branscombe (Eds.), Rediscovering social identity (pp. 13– 32). Psychology Press.
- United Nations Development Programme (UNDP) (1990-2019). Human Development Reports 1990-2019. Oxford University Press. http://hdr.undp.org/en/data
- Virick, M., & McKee-Ryan, F. M. (2017). The willingness to be underemployed: A study of unemployed professionals. Journal of Managerial Issues, 29(4), 322–342. https://www.jstor.org/stable/45176556 .
- Viswesvaran, C., & Ones, D. S. (1995). Theory testing: combining psychometric meta‐analysis and structural equations modelling. Personnel Psychology, 48(4), 865– 885. https://doi.org/10.1111/j.1744-6570.1995.tb01784.x
- Warr, P. B. (1987). Work, unemployment and mental health. Oxford University Press.
- Warr, P. B. (2007). Work, happiness, and unhappiness. Erlbaum.
- Warr, P. B. (2017). Happiness and mental health: A framework of vitamins in the environment and mental processes in person. In J. C. Quick & C. L. Cooper (Eds.), Handbook of stress and health: A guide to research and practice (pp. 57– 74). Wiley.
- Waterman, A. S. (1993). Two conceptions of happiness: contrasts of personal expressiveness (eudaimonia) and hedonic enjoyment. Journal of Personality and Social Psychology, 64(4), 678– 691. https://doi.org/10.1037/0022-3514.64.4.678
- Whitener, E. M. (1990). Confusion of confidence intervals and credibility intervals in meta- analysis. Journal of Applied Psychology, 75(3), 315– 321. https://doi.org/10.1037/0021-9010.75.3.315
- Winkelmann, L., & Winkelmann, R. (1998). Why are the unemployed so unhappy? Evidence from panel data. Economica, 65(257), 1– 15. https://doi.org/10.1111/1468-0335.00111
- Winkelmann, R. (2009). Unemployment, social capital, and subjective well- being. Journal of Happiness Studies, 10(4), 421– 430. https://doi.org/10.1007/s10902-008-9097-2
- Zacher, H., & Frese, M. (2009). Remaining time and opportunities at work: relationships between age, work characteristics, and occupational future time perspective. Psychology and Aging, 24(2), 487– 493. https://doi.org/10.1037/a0015425
- Zyphur, M. J., Allison, P. D., Tay, L., Voelkle, M. C., Preacher, K. J., Zhang, Z., Hamaker, E. L., Shamsollahi, A., Pierides, D. C., Koval, P., & Diener, E. (2020). From data to causes I: building a general cross- lagged panel model GCLM). Organizational Research Methods, 23(4), 651– 687. https://doi.org/10.1177/1094428119847278