Abstract
Objectives
Understanding longitudinal patterns of rural–urban mental health disparities is vital for effective intervention and policy development in China. However, few studies have estimated separate effects of birth-cohort and ageing and examined the role of community resources on health inequalities.
Methods
Drawing data from the China Health and Retirement Longitudinal Study (2011–2018), this study employed multilevel modelling to identify the mental health trajectories of rural, peri-urban, urban older adults by cohort and the community effects.
Results
The changes in the mental health gap between rural, peri-urban and urban older adults over time varied by birth cohorts. Among younger cohorts aged under 65, the mental health disparities between rural, peri-urban and urban residents increased as they got older. Underdeveloped community infrastructure greatly explained the rural health disadvantage.
Conclusion
The study indicates increasing rural–urban health disparities at the onset of later life. Improving community infrastructure in rural and peri-urban areas is vital to minimise rural–urban health gaps.
Introduction
The mental health disparity between rural and urban residents in China is stark and longstanding. A meta-analysis showed that the pooled prevalence of depressive symptoms is almost 10% higher in rural than urban areas (Zhang et al. Citation2012). Moreover, a number of cross-sectional studies have demonstrated the rural–urban gap in mental health, especially among older Chinese adults and rural female (Cheng et al. Citation2019; Li et al. Citation2015). However, relatively few studies have explored changes in the mental health gap longitudinally over the life course and the temporal patterning of rural–urban mental health disparities. Some existing longitudinal studies report that the rural disadvantage in depressive symptoms and cognitive ability increases with age (Hu et al. Citation2018; Xu et al. Citation2017). Conversely, other evidence has shown that the changes in rural–urban health gap increase from age 45 to 75 but decrease after age 75 and reduce at very old age (75+) (Wang and Stokes Citation2020). However, this age-based variation in rural–urban health inequality might be due to birth cohorts differences rather than individual ageing effects (Mirowsky and Kim Citation2007; Yang and Lee Citation2009). Indeed, the recent studies suggest that the health trajectory in later life varies by birth cohorts (Chen, Yang, and Liu Citation2010; Li and Zhang Citation2014). For example, Li and Zhang (Citation2014) found that the rural disadvantage in mental health is smaller for younger cohorts (born in 1936–1947) compared with those who were born before 1936. The study shows the importance of disentangling ageing and cohort effects to understand health disparities longitudinally. However, this study used surveys which were not designed as nationally representative (Zeng, Citation2008) and did not contain younger cohorts (born after 1947) whose life course overlaps with rapid urbanisation and recent government initiatives to reduce the urban–rural gap.
Moreover, most studies limit their focus to a rural–urban binary. Considering the ongoing urbanisation process in China, the dichotomised categorisation neglects the heterogeneity within both rural and urban areas. Peri-urban areas, including town, townships and emerging residential types (e.g. ‘village in the city’), have received less attention in the previous studies (Zhu Citation2017). Since the late 1970s, in-situ urbanisation has been promoted by the Chinese government in townships, counties and urban–rural fringe areas by transforming farmlands into urban use and granting residents an urban Hukou (Zhang, Chiu, and Ho Citation2019; Zhu Citation2017). The living conditions and infrastructure in peri-urban areas are less developed than in cities, yet the adverse health effects of urbanisation have sprawled into these areas (Liu Citation2020). Recent studies found a substantial urban-periurban-rural gradient regarding the all-cause mortality rate and depression with urban advantages (Hu, Li, and Martikainen Citation2019; Yu and Zhang Citation2020). These findings indicate the importance of a finer definition of residential type beyond the rural–urban dichotomy in Chinese context. Another limitation of the previous studies is that potential mechanisms accounting for changes in rural–urban mental health disparities have not been fully explored. Cross-sectional studies have found that the community physical and social environment are greatly associated with depression and CVD risks (Li et al. Citation2016; Wang et al. Citation2018; Wang and Stokes Citation2020). However, few studies explore whether community social and physical environments account for life course rural–urban mental health disparities.
This study aims to contribute to understanding the mental health disparities among rural, peri-urban and urban residents in later life in China. Using a nationally representative dataset, this study employs multilevel modelling to investigate rural–urban mental health disparities longitudinally. Particularly, birth cohorts by time interaction terms were added in the models to examine whether mental health trajectories differ by birth cohorts. Age-vector graphs, a visual tool to present the model prediction of the mental health changes over the study period for each birth cohort, were used to illustrate the complex interaction effects of ageing, birth cohort and residential status in a nuanced manner. Additionally, the mechanisms underlying rural–urban mental health disparities, especially the unexplored impact of community environment, will be examined. The research questions of this study are: (1) Whether the mental health gap between urban, peri-urban and rural older Chinese adults changes as people age during the period 2011–2018? (2) Whether changes in the mental health gap between urban, peri-urban and rural older Chinese adults vary by birth cohort? (3) What explains the disparities between rural, peri-urban and urban older adults in mental health trajectories?
Theoretical framework
Cumulative inequality theory (CIT) provides the theoretical background for this study to understand rural–urban disparities in health trajectory across the life course (Ferraro, Shippee, and Schafer Citation2009). By integrating cumulative (dis)advantages and life course perspective, this theory presents more holistic and comprehensive explanations for how inequality accumulates over time. Two propositions of the theory informed the hypotheses of this current research: (1) Inequality is generated through two separate mechanisms: ‘developmental processes’ (age-linked stimuli, events and experiences) and ‘demographic processes’ (cohorts-linked stimuli, events and experiences); (2) The mechanism of the cumulative inequality should be understood on multiple levels (ecological context), including both individual and contextual effect of neighborhoods and social norms.
Developmental and demographic processes: rural–urban disparity in health trajectories
The development processes hypothesis argues that the health gap of different rural–urban residential groups increases with age. This hypothesis was developed from cumulative (dis)advantage theory which posits how differences in resources and opportunities acquisition during earlier life stages incur incremental advantages and disadvantages over the life course, thus shaping the health and wellbeing in later life (Dannefer Citation2003; O’Rand Citation1996).
Different from the ‘urban health penalty’ in the western societies, the previous studies have found an ‘urban advantage’ in China. A stringent household registration system (Hukou) was implemented since 1950s to control increasing internal migration and regulate a range of social welfare and economic opportunities (Ma Citation2002; Treiman Citation2012). Song and Smith (Citation2019) found that rural residents with rural Hukou are more likely to experience childhood adversities, have less access to health services in different life stages, have lower educational attainment and reduced social support. Health disadvantages have also found in peri-urban areas, which usually receive few resources due to their lower administrative level (Jones-Smith and Popkin Citation2010; Ma Citation2002). Some peri-urban areas, at the fringe of a city are usually occupied by low-end industries and are enclaves for mass migrants, characterised by overcrowding and dilapidated living conditions (Li et al. Citation2020; Lin, de Meulder, and Wang Citation2011). Being constantly exposed to these potential risk factors might have further detrimental consequences for long-term health.
Drawing upon the CIT and previous empirical findings in Chinese context, we argue that the social organisation in China results in the structural disadvantages of rural and peri-urban residents in China across the life course. Urban residents have more resources and information to live a healthier lifestyle than their rural counterparts. Thus, the inequalities in different social, economic and health domains will accumulate and accentuate as people age.
Hypothesis 1 (H1): The rural and peri-urban disadvantage in mental health increases as older adults age regardless of birth cohorts.
Demographic process hypothesis highlights the differences between birth cohorts, positing that different cohorts experience differential exposure to historical change and social context which might have long-lasting health consequences (Elder Citation1985; Ryder Citation1965). With the rapid socioeconomic transformation in China, it might be untenable to assume the health trajectories of rural and urban residents across cohorts are homogenous. During recent decades, the Chinese government has implemented a series of top-down measures to close the rural–urban gap and promote rural–urban integration. Investment has increased in rural infrastructure. Social security coverage further expanded in rural areas with the implementation of the ‘New Rural Cooperative Medical Scheme’ and the ‘New Rural Old-Age Insurance’ in 2003 and 2009. Therefore, younger cohorts born after 1950s are more likely to contribute to these social security schemes and enjoyed higher levels of reimbursement in later life. Moreover, these younger cohorts tend to experience more equal access to different resources and opportunities than cohorts born before 1950. They are more likely to take advantage of the emerging opportunities of the economic reform and the relaxation of Hukou restrictions since 1980s to achieve social mobility and improve their livelihood, given that they were still at their early adulthood after the reform (Treiman Citation2012). Li and Zhang (Citation2014) empirically examined the cohort variation in health trajectories and found that compared with older cohorts, younger cohorts are more likely to experience decreasing rural–urban health gaps in mental health. Therefore, we propose the following hypothesis:
Hypothesis 2 (H2): The increase in rural, peri-urban disadvantage in mental health with age is larger for older birth cohorts compared to younger cohorts (born after the 1950s).
Ecological context and rural–urban health disparity
As CIT suggests, ecological factors shape the development of inequality and their effects also unfold over the life course (Ferraro et al. Citation2009). To further understand the pathway(s) linking urban/rural settings to health in later life, this study adapts the ecological model developed by Klitzman et al.’s (Citation2006) thesis on the urban physical environment and health. The original model considers the ecological nature of the living environment and its impact on health, focussing on two spheres of influence: community-level factors and proximate-level factors. Drawing upon empirical research in China, we expanded the community-level factors to highlight the importance of contextual determinants on health disparities, including infrastructure deficiency, perceived environment, health facilities, social security, recreation facilities, social organisation, and average wage of the community. The proximate-level factors include demographic characteristics, socioeconomic status, health behaviour, Hukou status, early-life health and other individual- and household-level factors. Based on these ecological models, we expect that community-level factors can explain the longitudinal disparity of mental health trajectories across cohorts.
Hypothesis 3 (H3): The disparity in rural–urban health trajectories will reduce after controlling for community-level physical, social and institutional environmental factors.
Methods
Data
This study draws data from the China Health and Retirement Longitudinal Study (CHARLS), a nationally representative longitudinal survey of residents from mainland China aged 45 and older. CHARLS adopted a multistage-stratified probability proportional to size (PPS) sampling, covering 450 communities in 150 counties across 28 provinces in mainland China. The primary sampling units (PSU) is the lowest level of government organisation (community), consisting of administrative villages (cun) in rural areas and neighbourhood (juweihuiu) in urban area. Four waves of the survey were conducted in 2011, 2013, 2015 and 2018. At baseline, the response rate is 80.51% with 17,708 individuals in 10,257 household being included in the sample (Zhao et al. Citation2020). CHARLS contains rich data on individual socio-demographic characteristics, health, employment and wealth. At baseline, leaders of each chosen community completed a community survey, covering the information on natural, social and economic environment of the community. The current study only includes respondents aged between 50 and 90 at baseline. Considering that the life expectancy in rural China is lower than urban China, we dropped the respondents aged over 90 at baseline (N = 45),Footnote1 because rural residents who survived to age 90 and over are likely to be healthier and more resilient than their urban counterparts, which might bias the result. Only respondents with a baseline weight were kept in the sample. After dropping all observations with missing data, the final sample size was 10,581.
Measures
Mental health
Depressive symptoms were used to measure the mental health of older adults in China. The measure is derived from the 10-item Centre for Epidemiologic Studies Depression Scale (CESD), which is validated as a suitable scale to measure depression in older Chinese (Cheng and Chan Citation2005). For each item, the frequency of a particular depression-related feeling over the week prior to the interview was asked, with 0 indicating ‘rarely or never experienced this feeling’ and 3 indicating experiencing this feeling ‘most or all of the time’. The derived variable ranged from 0 to 30 with higher scores denoting higher depression symptoms.
Residency
The rural, peri-urban and urban residents were defined based on the type of the community they are living in, according to the CHARLS PSU dataset. Aligned with the National Bureau of Statistics of China, all the communities are first categorised as urban and rural. Within urban areas, only respondents living in main city zones are defined as urban residents. Respondents living in combined urban–rural areas, town centre, combined town-township or special districtsFootnote2 are defined as peri-urban residents.
Cohort and time
Respondents were grouped into 1-year birth cohorts based on their baseline age. This time-invariant variable was centred at age 60, with a range from −10 to 25. The passing of time was measured by years since the first wave with a range of 0–7. Different from studies using arbitrary-defined age group representing cohort, using one-year birth cohort further enables us to observe more nuanced cohort effects and gradual cohort changes. Methodologically, arbitrary-defined cohort should not be used without explicit rationale, as it imposes multiple constraints to the models with assumptions difficult to verify (Luo and Hodges Citation2016).
Covariates
At the individual level, we controlled for socio-demographic characteristics, including gender, marital status (1 = married/partnered, 0= widowed/separated/single/divorced), years of education, working status (1 = agricultural work, 2 = non-agricultural employed, 3 = non-agricultural self-employed or family business, 4 = retired, 5 = unemployed, or never worked). Given that rural and urban residents differ in health behaviours, which is a key determinant of health (Mao and Wu Citation2007), drinking history (1 = have consumed alcohol, 0 = never drunk), smoking history (1= have smoked, 0 = never smoked), Hukou status (1 = rural Hukou, 0 = urban/unified hukou) were also controlled in the models. To address the potential health selection effects, childhood health status (self-rated health up to age 15, 1 = poor, 0 = excellent/very good/good/fair) and migration experience (1= not living in the same county as birthplace, 0 = otherwise) were incorporated in the analysis. Household-level measures include household income and the number of children alive. The latter is controlled because rural households in China on average have more children than urban households due to the family planning policies and cultural norms. Children can provide instrumental and emotional support for older adults which might indirectly affect their mental health (Grundy and Read Citation2015).
All community-level variables were derived from items available in the community questionnaire. Infrastructure deficiency was an index derived from six items regarding: drinking water, fuel, road, sewage, waste management and toilet facilities. The infrastructure deficiency index is calculated as the mean of the six items with a range of 0–3, with 3 indicating the most deficient (Li et al. Citation2015). Perceived environment was assessed based on the interviewer’s observation about the community environment, including social economic status, the degree of crowdedness, the degree of community handicapped access, etc. A mean score of the 5 items, ranging from 0 to 7, was derived with higher scores indicating more pleasant environment. The measure for recreation facilities was the total number of the seven types of sports and entertainment amenities (0–7) such as swimming pool, outside exercising facilities and room for ping pong. Social organisation was measured by up to seven types of the community social groups (i.e. dancing teams, charity organisations, elderly associations, etc.) available for older adults. The average income of the community was the per-capita net income of this village/community in 2010. Health facility index was derived from what types of medical facility residents attend when seeking medical care. A score of 3 was assigned to hospitals (general, specialised and Chinese medicine hospital), 2 for community healthcare centre/clinic, and 1 for medical post or pharmacy. As the index is derived from a multiple response question, the score for the three items were totalled (0–6). Social security was the number of types of social security program that the community provided (0–6), such as unemployment subsidies, minimum living allowance, other poverty subsidies.
Analytic strategy
Individual weights with household and individual non-response adjustment at baseline were used to calculate descriptive statistics. Due to the data’s hierarchical structure, three-level multilevel modelling was used to estimate cohort specific changes of depressive symptoms by residency during the 7-year period. Specifically, the repeated health measures of each wave at level 1 were nested in each individual at level 2. To account for community-level contextual effects, a third level was added with each individual nested in their community. The multilevel modelling techniques allow us to distinguish the variance of health measures due to ageing processes within individuals and variance due to between-individual and between-community heterogeneity. All models were fitted using multilevel linear regression.
Minimally adjusted models (model 1) were estimated using time since baseline (time variant), cohort (time-invariant), residency, and their interactions were included in the models. To validate H1, we focussed on the interaction between time and residency. Cohort and its interaction with residency were also controlled as this allows us to investigate the net effect of time on mental health and its rural–urban disparity. We tested H2 by examining the three-way interaction between time, cohort and residency. A set of individual-level and household-level covariates were added into partially adjusted models (model 2) and all covariates including the community-level covariates were controlled for in the fully adjusted models (model 3). In all models, the random effects on intercept and time since baseline were added, as we assume that the mean level and rate of changes in mental health might vary by individual (see Annex 2 for model details). The changes in the coefficients of residency and its interaction terms with wave and cohort will evidence to what extent the covariates explain rural–urban disparities observed. Our model specification is slightly different from traditional growth curve models as it does not model age but decomposes age into time-constant age at baseline and the passing time over the study period, which helps to distinguish the developmental processes from intercohort trends and allows the changes with age vary by generations (Mirowsky and Kim Citation2007). Considering the complex interaction effect was hard to interpret, age-vector graphs were drawn to visualise the prediction of the model presented above. They mapped the linear changes of mental health across the different birth cohorts (Mirowsky & Kim, Citation2007).
We used inverse probability weighting adjustment (IPW) to attenuate potential selection bias caused by attrition in this study (see Annex 1 for attrition pattern and detailed weighting strategy). Each observation in a particular wave was reweighted according to their characteristics which might contribute to sample loss with higher weights assigned to those more likely to drop out. Multiple imputation (MI) was also conducted to address data missingness due to item-nonresponse and non-participation for robustness checks. The results using MI largely align with the final results (see technical detail and results in Annex 3)
Results
Descriptive analysis
shows the characteristics of the rural, peri-urban and urban residents in the sample at baseline. Urban respondents had better socioeconomic conditions on average, with 7.7 years of education, 20,900 RMB household income per capita, more likely to be in non-agricultural work (21.90%) and retired (64.26). The Peri-urban sample was in-between rural and urban samples for all socioeconomic indicators. The rural–periurban–urban health gradient was significant during childhood with more rural residents reporting poor childhood health.
Table 1. Baseline sample characteristics of CHARLS respondents by residency
Furthermore, there were stark differences among rural, peri-urban and urban samples at the community level. The social and physical community environment was more developed and age-friendlier in urban areas with a greater variety of health facilities and social infrastructures available than rural and peri-urban ones.
The cohort-specific trajectories of rural–urban mental health disparity
presents the results of multilevel linear regression models on the urban–rural differences in depressive symptoms. Predicted trajectories of depressive symptoms by residency across birth cohorts were presented in . As a comparison, presents the aggregated age trajectoriesFootnote3 for depressive symptoms without controlling the cohort differences.
Figure 2. Minimally adjusted (a) and fully-adjusted (b) aggregated age trajectories for depressive symptoms
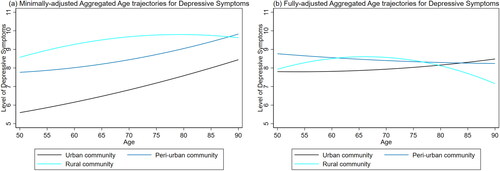
Table 2. Multilevel models for depressive symptoms by rural–urban residency
depicts the prediction of the minimally-adjusted models, suggesting that there was urban–periurban–rural gradient in mental health for all birth cohorts across later life. Rural residents had higher depressive levels than urban residents, whilst the mental health trajectories of peri-urban residents were mostly in-between and more similar to rural residents.
To examine hypothesis 1, we focussed on the within-cohort changes of mental health over time in . Overall, there were no consistent patterns for the changes of mental health gap among rural, peri-urban and urban adults across different cohorts. As model 1 suggests, when controlling for cohort effects, the interaction term of wave and residence was non-significant, indicating that there was no statistically significant change in the mental health gap between rural, peri-urban and urban residents as people age. However, the interaction effect only indicates the average ageing effects that were the same across all birth cohorts.
To test Hypothesis 2, we examined the changes of mental health over time between cohorts. The three-way interaction of time, age-cohort and rural residency in model 1 (b = −0.0138, p < 0.1) indicates that the change of rural–urban gap in depressive symptoms with age varied by birth cohort. As shows, for cohorts aged between 50 and 64 at baseline (mostly born before 1950s), although both rural and urban residents showed an increase in depression symptoms over the seven years, the rate of increase was faster among rural residents. Accordingly, the rural–urban gap in depressive symptoms increased over time with a more rapid increase among younger cohorts. However, the patterns reversed among older cohorts aged over 65 at baseline. Depressive symptoms declined with age for rural older cohorts, with the rate of decline steeper for those born before 1950s. Conversely, urban older cohorts reported increasing depressive symptoms across all cohorts as they age. Consequently, the rural–urban gap in depressive symptoms narrowed for older cohorts over the 7 years. Comparing peri-urban with urban residents, the mental health trajectories of peri-urban nearly paralleled with urban residents among the youngest cohorts (aged between 50 and 55 at baseline), yet their trajectories converged more substantially among cohorts older than 55 at baseline over the seven years. Additionally, the changes of mental health over time were smaller among most birth cohorts of peri-urban residents than rural and urban residents.
The results suggest that the mental health gap among rural, peri-urban and urban older adults over time varied by birth cohort. Hence, we found only partial support for Hypothesis 1 as rural–urban gap in depressive symptoms only increased with age among younger cohorts (age below 65 at baseline). Hypothesis 2 was rejected because the rural–urban divergence in depressive symptoms trajectories was more pronounced among younger cohorts. Additionally, comparing the aggregated age trajectories () with cohort-specific age trajectory () revealed how the former graphs masked the cohort variation in mental health trajectories. For example, in , the age trajectories from aged 50 to 60 of a rural and urban adult were nearly parallel. However, in , it is clear that the mental health gap between rural and urban residents from the cohort who are aged 50 at baseline grew much wider during the 7 years than the mental health gap of those aged 55 at baseline. This result indicates that the aggregated trajectory fails to separate the age and cohort effects, further highlighting the importance of taking account of the cohort differences when exploring the rural–urban mental health disparities.
To explain the rural–urban differences in mental health trajectories, individual-level and community-level factors were added in model 2 and model 3, respectively. shows that after adjusting for all covariates, the rural–urban gaps in depressive symptoms were largely minimised. In model 2, the cohort coefficients became negative after controlling individual-level covariates, which suggests that higher depressive symptoms for older cohorts might be explained by individual-level characteristics, such as their relatively lower educational status and poorer health in childhood. After adding community-level factors (model 3), the coefficient of rural residency became non-significant. The analysis of the fixed effects indicates that community infrastructure and wealth largely explained the rural–urban disparities in mental health. Residents in poorer communities where the infrastructure was more deficient (e.g., no paved road, tap water, and sewage system) reported higher depressive symptoms than their counterparts in richer communities. As the descriptive analysis shows, more rural and peri-urban residents lived in more deprived communities with underdeveloped infrastructure. Thus, the underdeveloped infrastructure and lower income might contribute to the unfavourable mental health condition of rural adults over time.
Discussion
This study examined the mental health disparity of older rural, peri-urban and urban residents in China from a longitudinal perspective, exploring how the rural–urban mental health gap changes with age and varies by birth cohort. We also explored the underlying mechanisms explaining these longitudinal patterns in rural–urban health inequality. The results suggest that: (1) H1 only holds for younger cohorts (aged below 65 at baseline). Contrary to H1, the mental health gap between rural, peri-urban and urban older adults reduced as they get older. (2) Moreover, contrary to H2, the mental health trajectory of rural and urban residents diverged among younger cohort but converged among older cohorts; (3) In relation to H3, community-level factors, especially the disparities in the development of infrastructure and poverty greatly contributed to explaining the mental health disparity in rural, peri-urban and urban residents.
This study highlights the scale of mental health disadvantage experienced by rural residents in China. The mental health advantage of urban residents compared with rural and peri-urban residents are persistent over the later life course. Even the highest level of depressive symptoms for urban residents (at age 90), is lower than the lowest level of depressive symptoms for rural residents (at age 50). Consistent with existing research on rural–urban disparities in mental health, this study extended previous findings by demonstrating the persistent health inequality across the later life course, lending stronger support to the ‘urban advantage’ hypothesis in China (Hu et al., Citation2019; Li et al., Citation2015, Citation2016; Zimmer et al., Citation2010). Beyond the rural–urban dualism, this study contributes to previous research by examining the mental health trajectories of peri-urban residents. Previous studies have mainly submerged this group into urban residents, neglecting the proliferating peri-urban areas during China’s rapid urbanisation. The results from this study suggest that the mental health trajectories of peri-urban residents are more similar to rural residents than urban residents, especially for older cohorts. Rather than well-developed and wealthy residential areas as in developed countries, most urban–rural fringe areas in China are still undergoing urbanisation. As shown in the descriptive analysis, infrastructure, public facilities and social organisations were still under developed compared with major cities. These results highlight that the mental health problems among older adults in peri-urban areas require urgent attention from policymakers.
We found that cohort plays an important role in shaping individual’s health trajectory and rural–urban health inequality. There are significant cohort differences regarding the changes of the rural–urban mental health gap across the seven years, indicating that cohort-related risks and opportunities have long-lasting structuring effects on life course inequality. As demographic process of CIT suggested, cohorts provide the context for individual development. This finding also concurs with previous studies from the United States and the UK focussing on the cohort variation of mental health trajectory (Bell Citation2014; Yang Citation2008). However, the cohort heterogeneity is more striking in the Chinese context (the trend reverses for younger cohorts), reflecting the unprecedented social, political, and cultural changes in contemporary China in the form of economic reform, large-scale internal migration and rapid urbanisation. From a methodological perspective, the study demonstrates that aggregated age trajectories mask the heterogeneity of mental health trajectories of different cohorts and potentially bias the relationship between residency and mental health over time. It foregrounds the need to disentangle developmental (chronological age) and demographic (cohorts) factors to understand the determinants of health inequality.
Our results do not fully support H2, which predicts the increasing mental health inequality with age is more pronounced among older cohorts. Instead, we found increasing mental health disparities among younger cohorts (aged below 65 at baseline). These cohorts were mostly born in 1950 and 1960, whose adulthood and midlife largely paralleled rapid urbanisation and rural-to-urban migration processes. With the easing of Hukou restrictions, these rural-born cohort members, especially who are healthier and better educated, have more opportunities to migrate to urban areas, leaving more deprived and unhealthy peers behind. The ‘healthy migrant’ effect in China has been documented in the early studies and the effect can persist over the later life course (Lu and Qin Citation2014; Xu et al. Citation2017). This within-cohort compositional change might lead to the increasing rural–urban health disparity for younger cohorts. Moreover, although both rural and urban areas have undergone rapid socioeconomic development in the recent decades, the pace of development might vary. The existing urban advantage may allow urban residents to take advantage of new opportunities during socioeconomic development and gain more benefits (Treiman, Citation2012), especially among younger urban cohorts. The finding indicates that despite the achievement in social security coverage and poverty reduction in rural areas in the recent decades, improvements have not yet fully translated into narrowing health disparities. More mental health interventions targeted at rural and peri-urban areas are therefore required, especially at the onset of later life.
Contrary to the development process hypothesis of the CIT, the study found a narrowing mental health gap between rural and urban residents in very old age. This finding is against previous studies which found increasing health inequality with age among different social groups in China (Hu et al. Citation2019; Xu Citation2019). The inconsistency might result from the fact that these studies estimate the aggregated age trajectory for all populations without considering the cohort variation. One possible reason for the convergence is that this trend is only observed among the very old, for whom the universal biological ageing process might outweigh social differentiation as postulated by age-as-leveller theory (O’Rand and Henretta Citation1999). Despite the onset of health deterioration emerging earlier for rural adults, the deterioration process is a shared experience for all in later ages. More specifically, the results show that rural and peri-urban residents from older cohorts decreased in depressive symptoms with age, as opposed to the increasing trend of older urban residents. It is likely that the older cohorts in rural and peri-urban areas had experienced the material deprivation most of their life (Treiman, Citation2012). The rapid infrastructural development and improvement in living standard in the last decade has greatly reduced their daily stress and increased their sense of optimism and hope toward life. However, although we took several measures to attenuate the selection effects, we cannot completely rule out the fact that rural older adults who live past average life expectancy are likely to be selected for good health.
In addition to depicting the health trajectories of different residential groups, this study further explores what factors might account for observed mental health inequalities and longitudinal trends. According to CIT, social systems produce inequality on multiple levels and ecological contexts shape the opportunities to access social and economic resources (Ferraro et al. Citation2009). The results substantiate this proposition, suggesting that community-level factors, especially the uneven infrastructure and economic development among urban, peri-urban and rural areas largely explained the gap in the level of depressive symptoms across each cohort between 2011 and 2018. These findings also concur with the previous studies which found rural–urban health disparities can be partly explained by community social and physical environments (Wang et al. Citation2018; Wang and Stokes Citation2020), and our study extends the previous research via its longitudinal design. However, the covariates considered in this study could not fully account for the diverging trend of rural and urban residents in younger cohorts. Future studies could further explore the increasing gap in these cohorts by separating potential compositional effects from causal and contextual effects.
This study has several limitations. First, the period covered by longitudinal data used is relatively short, which poses a challenge to isolating age and cohort effects as the rural–urban gap in mental health may take longer to manifest. Second, potential selection effects might bias the results for older age-cohorts. Although methods, including IPW and MI, were applied to attenuate selective survival, the bias might still remain due to the rural–urban difference in life expectancy. Third, the categorisation of rural, peri-urban and urban residents in this study may not capture the extensive heterogeneity in the level of urbanicity of different regions in China. In our study, towns and rural–urban combination zones were treated as the same, yet the development stage and level of urbanicity could vary in these communities and change over time. Moreover, as the community data was only available at baseline, all the community-level covariates were treated as time-invariant in the models. However, the community social and physical environment, and even the rural/urban classification, could have changed due to either market-driven urbanisation or government reclassification, which might lead to measurement bias and thus limit the explanatory power of community-level characteristics. Given the unavailability of more recent community-level data, the limitations are difficult to overcome in this current study. Future research could adopt a more dynamic definition of rural and urban communities and more updated measurements of community environment.
Despite the limitation, our study enriches the CIT by demonstrating nuanced cohort variations in the ageing process. It shows that the demographic process and developmental process are not isolated but contingent on each other. It also incorporated the ecological context in the analysis of the mental health trajectory. Although CIT stresses the importance of ecological context in shaping one’s life context, it does not explicitly illustrate how CIT applies in differential macro-level social and cultural context. Situated in China, the study contests the applicability of CIT to a developing country with drastic social changes in recent decades. In addition to theoretical contributions, this study also has policy implications. Health policy aiming at improving mental health among older adults should be tailored to the people’s age and birth cohort. It highlights the importance of further developing infrastructure in rural and peri-urban areas and narrowing the income gap to reduce rural–urban health disparities.
Supplemental Material
Download MS Word (159 KB)Acknowledgements
We would like to thank Professor James Nazroo for his helpful comments on earlier drafts of this article.
Disclosure statement
No potential conflict of interest was reported by the author(s).
Additional information
Funding
Notes
1 We also conducted analysis including the 45 people, which yielded similar results.
2 Special district includes industrial zones, newly-built industrial development zone and or district for state-own agricultural enterprise, usually located in peri-urban area. We were unable to accurately retrieve the nature of special districts because the communities are anonymous. However, the potential bias it might cause should be minimal due to the low number of observations from special districts (0.67% of the sample).
3 “Aggregated” indicates that the age trajectory is an aggregate of all cohorts.
References
- Bell, A. (2014). Life-course and cohort trajectories of mental health in the UK, 1991–2008 – a multilevel age–period–cohort analysis. Social Science & Medicine (1982), 120, 21–30. https://doi.org/10.1016/j.socscimed.2014.09.008
- Chen, F., Yang, Y., & Liu, G. (2010). Social change and socioeconomic disparities in health over the life course in China: A cohort analysis. American Sociological Review, 75(1), 126–150. https://doi.org/10.1177/0003122409359165
- Cheng, S.-T., & Chan, A. C. M. (2005). The Center for Epidemiologic Studies Depression Scale in older Chinese: Thresholds for long and short forms. International journal of geriatric psychiatry, 20(5), 465–470. https://doi.org/10.1002/gps.1314
- Cheng, Y., Gao, S., Li, S., Zhang, Y., & Rosenberg, M. (2019). Understanding the spatial disparities and vulnerability of population aging in China. Asia & the Pacific Policy Studies, 6(1), 73–89. https://doi.org/10.1002/app5.267
- Dannefer, D. (2003). Cumulative advantage/disadvantage and the life course: Cross-fertilizing age and social science theory. Journal of Gerontology B: Psychology Society, 58(6), S327-S337. https://doi.org/10.1093/geronb/58.6.S327
- Elder, G. H. (1985). Life Course Dynamics: Trajectories and Transitions, 1968-1980. edited by Social Science Research Council (U.S.). Ithaca, NY: Cornell University Press.
- Ferraro, K. F., Shippee, T. P., & Schafer, M. H. (2009). Cumulative inequality theory for research on aging and the life course. in Handbook of theories of aging (pp. 413–433), 2nd ed. New York, NY: Springer Publishing Company.
- Grundy, E., & Read, S. (2015). Pathways from fertility history to later life health: Results from analyses of the English longitudinal study of ageing. Demographic Research, 32, 107–146. https://doi.org/10.4054/DemRes.2015.32.4
- Hu, H., Cao, Q., Shi, Z., Lin, W., Jiang, H., & Hou, Y. (2018). Social support and depressive symptom disparity between urban and rural older adults in China. Journal of affective disorders, 237, 104–111. https://doi.org/10.1016/j.jad.2018.04.076
- Hu, Y., Li, P., & Martikainen, P. (2019). Rural–urban disparities in age trajectories of depression caseness in later life: ‘The China Health and Retirement Longitudinal Study’ edited by E. van Exel. PLOS One, 14(4), e0215907. https://doi.org/10.1371/journal.pone.0215907
- Jones-Smith, J. C., & Popkin, B. M. (2010). Understanding community context and adult health changes in China: Development of an Urbanicity Scale. Social Science & Medicine, 71(8), 1436–1446. https://doi.org/10.1016/j.socscimed.2010.07.027
- Klitzman, Susan, Matte, Thomas D., Kass, Daniel E., Cities and the Health of the Public, The urban physical environment and its effects on health, Freudenberg, Nicholas, Galea, Sandro, Vlahov, David (Eds.), Nashville, TN: Vanderbilt University Press, 2006, 61-84,
- Li, L. W., Liu, J., Xu, H., & Zhang, Z. (2016). Understanding rural–urban differences in depressive symptoms among older adults in China. Journal of aging and health, 28(2), 341–362. https://doi.org/10.1177/0898264315591003
- Li, L. W., Liu, J., Zhang, Z., & Xu, H. (2015). Late-life depression in rural china: do village infrastructure and availability of community resources matter?: Late-life depression in rural China. International journal of geriatric psychiatry, 30(7), 729–736. https://doi.org/10.1002/gps.4217
- Lin, Y., de Meulder, B., & Wang, S. (2011). Understanding the “village in the city” in Guangzhou: Economic integration and development issue and their implications for the urban migrant. Urban Studies, 48(16), 3583–3598. https://doi.org/10.1177/0042098010396239
- Liu, L. (2020). Rural–urban disparities in cardiovascular disease mortality among middle-age men in China. Asia Pacific Journal of Public Health, 32(8), 436–439. https://doi.org/10.1177/1010539520956446
- Li, Z., Yu, S., Han, X., Liu, J., & Yao, H. (2020). Changes to cardiovascular risk factors over 7 years: A prospective cohort study of in situ urbanised residents in the Chaoyang District of Beijing. BMJ Open, 10(3), e033548. https://doi.org/10.1136/bmjopen-2019-033548
- Li, T., & Zhang, Y. (2014). Growth curve trajectories of elderly people’s health indicators in China: Cohort variations and rural–urban disparities. Population Research, 38(2), 18–35.
- Lu, Y., & Qin, L. (2014). Healthy migrant and salmon bias hypotheses: A study of health and internal migration in China. Social Science & Medicine (1982), 102, 41–48. https://doi.org/10.1016/j.socscimed.2013.11.040
- Luo, L., & Hodges, J. S. (2016). Block constraints in age–period–cohort models with unequal-width intervals. Sociological Methods & Research, 45(4), 700–726. https://doi.org/10.1177/0049124115585359
- Ma, L. J. C. (2002). Urban transformation in China, 1949–2000: A review and research agenda. Environment and Planning A: Economy and Space, 34(9), 1545–1569. https://doi.org/10.1068/a34192
- Mao, Z., & Wu, B. (2007). Urban–rural, age and gender differences in health behaviours in the Chinese population: Findings from a survey in Hubei, China. Public health, 121(10), 761–764. https://doi.org/10.1016/j.puhe.2007.02.015
- Mirowsky, J., & Kim, J. (2007). Graphing age trajectories: Vector graphs, synthetic and virtual cohort projections, and virtual cohort projections, and cross-sectional profiles of depression. Sociological Methods & Research, 35(4), 497–541. https://doi.org/10.1177/0049124106296015
- O’Rand, A. M. (1996). The precious and the precocious: Understanding cumulative disadvantage and cumulative advantage over the life course. Gerontologist, 36(2), 230–238. https://doi.org/10.1093/geront/36.2.230
- O’Rand, A., & Henretta, J. C. (1999). Age And Inequality: Diverse Pathways Through Later Life. Boulder, CO: Westview Press.
- Ryder, N. B. (1965). The cohort as a concept in the study of social change. American Sociological Review, 30(6), 843–861. https://doi.org/10.2307/2090964
- Song, Q., & Smith, J. P. (2019). Hukou system, mechanisms, and health stratification across the life course in rural and urban China. Health & Place, 58, 102–150. https://doi.org/10.1016/j.healthplace.2019.102150
- Treiman, D. J. (2012). The “difference between heaven and Earth”: Urban–rural disparities in well-Being in China. Research in Social Stratification and Mobility, 30(1), 33–47. https://doi.org/10.1016/j.rssm.2011.10.001
- Wang, Y., Chen, Y.-C., Shen, H.-W., & Morrow-Howell, N. (2018). Neighborhood and depressive symptoms: A comparison of rural and urban chinese older adults. The Gerontologist, 58(1), 68–78. https://doi.org/10.1093/geront/gnx063
- Wang, H., & Stokes, J. E. (2020). Trajectories of rural–urban disparities in biological risks for cardiovascular disease among Chinese middle-aged and older adults. Health & Place, 64, 102354. https://doi.org/10.1016/j.healthplace.2020.102354
- Xu, H. (2019). Physical and mental health of Chinese grandparents caring for grandchildren and great-grandparents. Social science & medicine (1982), 229, 106–116. https://doi.org/10.1016/j.socscimed.2018.05.047
- Xu, H., Dupre, M. E., Gu, D., & Wu, B. (2017). The impact of residential status on cognitive decline among older adults in China: Results from a longitudinal study. BMC geriatrics, 17(1), 107. https://doi.org/10.1186/s12877-017-0501-9
- Yang, Y. (2008). Social inequalities in happiness in the United States, 1972 to 2004: An age-period-cohort analysis. American Sociological Review, 73(2), 204–226. https://doi.org/10.1177/000312240807300202
- Yang, Y., & Lee, L. C. (2009). Sex and race disparities in health: Cohort variations in life course patterns. Social Forces, 87(4), 2093–2124. https://doi.org/10.1353/sof.0.0183
- Yu, X., & Zhang, W. (2020). All-cause mortality rate in China: Do residents in economically developed regions have better health?’ International Journal for Equity in Health, 19(1), 12. https://doi.org/10.1186/s12939-020-1128-6
- Zeng, Y. (2008). Introduction to the Chinese Longitudinal Healthy Longevity Survey (CLHLS). In. Healthy longevity in China: Demographic, socioeconomic, and psychological dimensions, demographic methods and population analysis (pp. 23–38), Y. Zeng, D. L. Poston, D. A. Vlosky, & D. Gu (Eds.), Dordrecht, Netherlands: Springer Netherlands.
- Zhang, T., Chiu, R. L. H., & Ho, H. C. (2019). Suburban neighborhood environments and depression: A Case Study of Guangzhou, China. Journal of Transport & Health, 15, 100624. https://doi.org/10.1016/j.jth.2019.100624
- Zhang, L., Xu, Y., Nie, H., Zhang, Y., & Wu, Y. (2012). The prevalence of depressive symptoms among the older in China: A meta-analysis. International journal of geriatric psychiatry, 27(9), 900–906. https://doi.org/10.1002/gps.2821
- Zhao, Y., Strauss, J., Chen, X., Wang, Y., Gong, J., Meng, Q., Wang, G., & Wang, H. (2020). China health and retirement longitudinal study wave 4 user’s guide. Beijing, China: National School of Development, Peking University.
- Zhu, Y. (2017). In situ urbanization in China: Processes, contributing factors, and policy implications. China Population and Development Studies, 1(1), 45–66. https://doi.org/10.1007/BF03500917
- Zimmer, Z., Wen, M., & Kaneda, T. (2010). A multi-level analysis of urban/rural and socioeconomic differences in functional health status transition among Older Chinese. Social science & medicine (1982), 71(3), 559–567. https://doi.org/10.1016/j.socscimed.2010.03.048