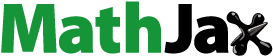
ABSTRACT
Innovation within companies is generated by a combination of different types of knowledge from external and geographically dispersed sources. Although the geographical dimension of collaboration has previously been investigated, studies have not distinguished between different types of knowledge involved in collaboration. This article analyses how the number of collaborations between pharmaceutical multinational companies (MNCs) and external organizations is affected by geographical distance, distinguishing between four knowledge types: basic science, clinical science, core knowledge, and exploration knowledge. We use co-publications as a proxy for collaborations. Our results show that collaborations in basic science and core knowledge areas are more negatively affected by geographical distance than collaborations within clinical science and exploration knowledge areas. This suggests that the importance of geographic proximity depends on the type of knowledge that is being transferred in R&D collaborations. Our results have implications for companies´ collaboration strategies and their choices for the R&D sites´ location.
1. Introduction
Innovation in a firm is the result of combining existing knowledge and new knowledge (Smith, Collins, and Clark Citation2005). In times of rapid technological change, it is difficult for a company to develop all necessary knowledge in-house. Companies increasingly use external collaboration to complement their in-house knowledge and to expand their global network to achieve competitive advantages (Freeman Citation1991; Powell and Grodal Citation2005). Therefore firms carry out a high proportion of their R&D activities in collaboration with both geographically close and distant external organizations (Scherngell Citation2013).
The geographical dimension of R&D collaboration has received wide attention in academic literature. Studies have mainly focused on the organizational level and identified country-specific and sector-specific mechanisms that have an impact on the role of geographical proximity in R&D collaboration (for example, Almeida Citation1996; Ponds, van Oort, and Frenken Citation2007; Hoekman, Frenken, and van Oort Citation2009; Waltman, Tijssen, and van Eck Citation2011; Plotnikova and Rake Citation2014). However, an increasing number of studies have questioned the dominant role of local collaboration and suggested that knowledge creation takes place in a combination of both geographically close and distant settings (Bathelt, Malmberg, and Maskell Citation2004; Owen-Smith and Powell Citation2004; Boschma Citation2005). So far this literature has not considered the heterogeneity of knowledge involved (Mattes Citation2012). Instead, different types of knowledge have only been indirectly taken into account by arguing that geographical proximity is important in knowledge transfer because of the difficulty of transferring tacit knowledge. We argue that different types of knowledge activities may require different geographical logics. This issue is at the core of this study.
We are contributing to the existing literature on R&D collaboration and geography in several ways. First, we look below the organizational level and focus on different types of knowledge within the organization. Second, we go beyond the traditional distinction between tacit and codified knowledge that has been criticized as conceptually vague and difficult to empirically identify (Cowan, David, and Foray Citation2000) and introduce a categorization that takes into account the heterogeneity of knowledge within the firm by considering the innovation phase, the nature of knowledge and the level of internal knowledge. Third, much of the literature on MNCs and geography has focused on cost reduction, market potential and competitive advantages as factors influencing companies’ location strategies (Alcácer and Chung Citation2007; Basile and Kayam Citation2015). In this paper, we focus instead on the link between different types of knowledge in R&D collaborations and the importance of geographical distance as a location driver for MNCs.
The empirical analysis of the effect of the geographical distance on research collaboration involving different knowledge types is carried out in the pharmaceutical sector, one of the most knowledge-intensive industries (Kessel Citation2011). The R&D process in the pharmaceutical industry is a complex trajectory driven by the search for fundamental understanding of a phenomenon and skills to manage large-scale clinical trials (Henderson, Orsenigo, and Pisano Citation1999). This process requires access to specialized knowledge from different disciplines spread around the globe. Therefore, pharmaceutical companies carry out a high proportion of their R&D activities in collaboration with both geographically close and distant external actors (Calero, Van Leeuwen, and Tijssen Citation2007). The pharmaceutical sector is thus a suitable arena in which to study the issue addressed in this paper.
We use co-publications from 6 multinational pharmaceutical companies covering the period 2000–2012 to explore the link between geographical proximity and type of knowledge in collaborations. Co-publications have been commonly used in the literature to measure collaborations between organizations. The R&D process in pharma includes two different stages: drug discovery and drug development, in which different types of knowledge, skills and resources are needed. A distinction is also often made between core and exploration areas representing different levels of internal knowledge in companies. Therefore, each publication is classified into knowledge types based on two different dichotomies: (1) basic science or clinical science, and (2) core and exploration knowledge areas of the firms. The geographical distance between an MNC and a collaborator was determined by the location of the two organizations.
The paper is structured as follows. In section 2, we present earlier literature on the geographical and knowledge dimensions of R&D collaboration. Section 3 introduces the geographical logic of the knowledge types in collaboration in the pharmaceutical industry and derives the hypotheses. The methodology, the models and the estimation results are presented in sections 4, 5 and 6. In section 7, we discuss the results.
2. Geography and knowledge heterogeneity of R&D collaborations
The knowledge-based theory of the firm considers knowledge to be the most important resource of a firm (Grant Citation1996; Kogut and Zander Citation1992). In recent decades, the knowledge creation process has become increasingly complex, involving a heterogeneity of actors and organizations outside the firm. External collaborations are considered an efficient way to obtain access to the newest knowledge and highly specialized skills (Zucker, Darby, and Armstrong Citation2002). At the same time, collaborations break up established paths, avoiding lock-in situations.
The geographical dimension of R&D collaboration has generated a lot of attention in the literature (for example, Owen-Smith and Powell Citation2004; Ponds, van Oort, and Frenken Citation2007; Hoekman, Frenken, and van Oort Citation2009; Plotnikova and Rake Citation2014). Researchers have typically indicated that innovation is a highly localized phenomenon and geographical proximity matters in the knowledge transfer process (Audretsch and Feldman Citation1996; Jaffe, Trajtenberg, and Handerson Citation1993; Ponds, van Oort, and Frenken Citation2007). These studies argue that short distances facilitate communication and knowledge exchange through face-to-face interaction, personal relations, casual and unintended meetings (Audretsch and Feldman Citation1996; Paci and Usai Citation2000). In addition, geographic proximity favours social interactions and trust building (Boschma Citation2005; Ponds, van Oort, and Frenken Citation2007). Distance collaborations, on the other hand, require high transmission costs, meetings to be planned in advance and a more defined collaboration strategy. Consequently, it is more difficult to exchange knowledge over long distances (Audretsch and Feldman Citation1996).
However, an increasing number of studies have questioned the dominant role of relying on only local collaboration. They argue that knowledge creation takes place in a combination of both geographically close and distant settings (for example Kale, Singh, and Perlmutter Citation2000; Vatne Citation2001; Gertler Citation2003; Bathelt, Malmberg, and Maskell Citation2004; Owen-Smith and Powell Citation2004; Boschma Citation2005). Distant collaborations are required in order to access information about market trends and the newest technologies (Bathelt, Malmberg, and Maskell Citation2004). Owen-Smith and Powell (Citation2004) have shown, in the case of the Boston biotechnology industry, that access to new knowledge not only results from local and regional interaction. It is often acquired through strategic partnerships with interregional and international actors. Boschma (Citation2005) argued that local collaborations may become more beneficial for the company if they are combined with distant partnerships that promote recombination of ideas and mitigate the problem of spatial knowledge lock-in. Thus, both local and distant collaborations make complementary contributions to the knowledge pool of a firm (Moodysson, Coenen, and Asheim Citation2008). Accordingly, companies, and in particular MNCs, have adopted a combination of local and distant collaborations.
One limitation of current studies discussing the geographical dimension of R&D collaboration, is that they treat the knowledge internal to a firm as a homogenous phenomenon. This is surprising given that knowledge is a heterogeneous entity that can be classified in many different ways, for example according to the nature of the knowledge, the actors involved, and the innovation phase. In the literature, the most common way to differentiate between knowledge types is the dichotomy between tacit and codified knowledge which was first introduced by Polanyi (Citation1960). Tacit knowledge is highly embedded in particular physical and social contexts and cannot be adequately articulated by verbal means (Polanyi Citation1960). It is mostly transmitted through face-to-face interaction and personal relations that need a short distance between partners (Hippel Citation1994; Feldman and Lichtenberg Citation2000). Codified knowledge, on the other hand, can be transferred by means of written documents, which more easily facilitate long-distance collaboration (David and Foray Citation1995).
While the distinction between tacit and codified has been useful in understanding R&D processes, the approach has also been criticized as being difficult to measure (Cowan, David, and Foray Citation2000). Consequently, there is a need to provide a differentiation of knowledge types that can be measured empirically. In this paper, we therefore suggest measurable conceptualization of knowledge types for the pharmaceutical sector by distinguishing between basic science and clinical science knowledge, and core and exploration knowledge areas. Although these distinctions are connected to the differentiation between tacit and explicit knowledge, they are more robust in identifying and measuring the heterogeneity of knowledge involved in different innovation phases, the nature of knowledge produced in collaborations, and the level of internal knowledge presented within pharmaceutical firms when engaging in collaborations. In the next section, we provide insight in these different types of knowledge and hypothesize about how they are related to the geographical dimension of R&D collaboration in the pharmaceutical sector.
3. The link between geographical distance and knowledge of R&D collaboration and hypothesis formulation
Before exploring the geographical dimensions of different types of knowledge, we build a base-line hypothesis on the role of geographical distance in pharmaceutical MNC collaborations.
The pharmaceutical industry is one of the most-R&D intensive industries (Gassmann and Reepmeyer Citation2005), relying on highly complex knowledge. Knowledge comes from a broad range of different scientific disciplines such as chemistry, physiology, toxicology, pharmacology, medicine and biology (Henderson Citation1994). Previous research on innovation shows that access to knowledge and skills is an essential determinant of a pharmaceutical firm´s capabilities and its success in bringing drugs to market. For example, Plotnikova and Rake (Citation2014), studied the pharmaceutical industry, and found that geographical proximity has a significant positive relation to the collaboration intensity between countries. Ponds, van Oort, and Frenken (Citation2007) found that in the life science sector geographic proximity is important but it is even more important for collaborations between academic and non-academic organizations than for collaborations between academic organizations. Zucker, Darby, and Armstrong (Citation2002) concluded that the success of life science firms is positively related to the number of collaborating academic star scientists, defined as highly productive researchers, in their geographical area. In sum, the complexity of the knowledge base and the coordination between the myriad of different actors involved from different scientific disciplines and sectors suggest the importance of geographic proximity.
Consequently, we formulate our baseline hypothesis on the role of geographical proximity in collaborations involving pharmaceutical MNCs as follows:
H1: The number of collaborations between a pharmaceutical MNC and external organizations is negatively affected by geographical distance.
In order to further investigate the geographical dimension of collaboration in the pharmaceutical industry, we introduce the first classification of knowledge type based on the dichotomy between basic science and clinical science knowledge. The search for a new drug takes place in two different stages: discovery and development. In the former, basic science is the basis for knowledge creation while, in the latter, clinical science knowledge is needed.
Basic science knowledge refers to the understanding of a phenomenonFootnote1 and it is relatively tacit although it is partly based on codified information provided by other researchers. According to Zucker, Darby, and Armstrong (1996) new information tends to be produced in tacit forms and tacitness in knowledge increases as a function of distance from prior knowledge, especially breakthrough knowledge. In the pharmaceutical sector, basic science knowledge includes the attempts to reveal the mechanism and the process of a disease (Lim Citation2004). This knowledge is involved in the first phases of the drug discovery process, which includes the search for a disease target and the selection of drug candidates for preclinical tests on animals (Henderson and Cockburn Citation1996). The drug discovery process is recursive and involves a trial-and-error process (Moodysson, Coenen, and Asheim Citation2008). Therefore, basic science knowledge is not generated from a linear process. It is highly dependent on serendipity instead. Furthermore, this knowledge is highly tacit because it cannot be articulated verbally and it is based instead on experience accumulated in individuals (Lim Citation2004). The knowledge is mainly embedded in individual academic researchers and therefore collaborations with universities are crucial to the generation of basic science knowledge in firms (Arora and Gambardella Citation1990; Owen-Smith and Powell Citation2004).
Clinical science knowledge is needed in the development stages to carry out clinical trials, where compounds are tested in humans to assess the safety and efficacy of a drug (Henderson, Orsenigo, and Pisano Citation1999; Cockburn and Henderson Citation2001). The clinical trials are divided into three phases. In phase I, the compound is tested in healthy volunteers to ensure that it is safe. In phase II, the compound is tested in a small group of patients to establish efficacy, and in phase III the compound is tested in very much larger numbers of more diverse patients to establish both safety and efficacy. These trials are conducted in hospitals and must follow the guidelines from the regulatory authorities (for example the U.S. Food and Drug Administration and other national and international regulatory frameworks) in countries where the drug is intended to be registered and commercialized. As a consequence, clinical science knowledge originates from a strongly regulated process, which leaves little room for spontaneity in knowledge creation (Moodysson Citation2009). More specifically, all steps of the clinical trials need to be thoroughly documented and justified. This explains the highly codified nature of the clinical science knowledge.
Based on these definitions, we test whether the role of geographical proximity differs in the two knowledge types. Basic science knowledge is more tacit and originates from a more unpredictable process. It requires short distance and face-to-face interaction in order to be transferred. In addition, basic science knowledge is often generated by scientists within an academic setting and, for industries to get access to this knowledge, geographical proximity is necessary (Zucker and Darby Citation1996; Abramovsky et al. Citation2011). Clinical science knowledge, on the other hand, is mainly codified and originates from a more linear R&D process that can be controlled at a distance. Collaborations in clinical science knowledge areas are therefore less dependent on geographical proximity than basic science knowledge areas.
From these arguments, we can derive our second hypothesis:
H2: The number of collaborations between a pharmaceutical MNC and an external organization is more negatively affected by geographical distance in basic science than in clinical science knowledge areas.
To further explore the relationship between knowledge type and geographical proximity in collaboration, we add to the basic science and clinical science knowledge dimension, the internal knowledge of the firm (exploration and core knowledge areas). The concept of absorptive capacities was introduced by Cohen and Levinthal (Citation1989, Citation1990) and underlines the importance of taking into consideration the level of a firm´s internal knowledge when studying R&D collaborations. Companies generally have a few core knowledge areas in which they have a high level of internal R&D knowledge and absorptive capacities that facilitate the acquisition of external knowledge. However, they also have a number of exploration knowledge areas where the level of internal R&D knowledge is lower than in core areas. The distinction between core and exploration knowledge areas can be linked to the concepts of exploration and exploitation knowledge areas first introduced by March (Citation1991). He underlined the value of collaboration for an organization’s learning process in accessing new knowledge outside the firm´s core boundaries, knowledge exploration, and leveraging existing internal knowledge with external, known as knowledge exploitation.
In the pharmaceutical sector, companies organize their activities around therapeutic areas, such as cancer, cardiovascular, neurological diseases etc. These therapeutic areas can be divided into core and exploration knowledge areas. Core knowledge areas are critical for the company because they are the main sources of its innovations and revenues. They are characterized by solid in-house knowledge that guarantees certainty, speed, and clarity in collaborations (Hansen, Nohria, and Tierney Citation1999). Furthermore, several authors argue that companies in core areas rely more on strong ties characterized by recurrent relations with the same partners in which they closely control the knowledge transfer (Kale, Singh, and Perlmutter Citation2000; Cantwell and Mudambi Citation2011). Consequently, it can be expected that collaborations in core areas are easier carried out in geographical proximity. For example, Eli Lilly has been a leader in the field of diabetic therapy for over a hundred years. Therefore, the therapeutic area of endocrinology can be considered a core knowledge area. Exploration knowledge areas are represented by new therapeutic areas in which the company decides to diversify its market. These areas require a flexible collaboration network allowing access to a diversity of skills and continuous scanning for new technological opportunities (Hansen, Nohria, and Tierney Citation1999). Collaborations in exploration knowledge areas thus need input from a network of external partners globally dispersed. For example, Pfizer and GlaxoSmithKline decided to join forces and created a joint venture, ViiV Healthcare, to develop expertise in the development of HIV drugs. Infectious diseases could, therefore, be considered an exploration knowledge area for both companies.
Based on the distinction between basic science and clinical science knowledge, we add the dichotomy of core and exploration knowledge areas. We obtain 4 different knowledge types (basic science-core knowledge area, basic science-exploration knowledge area, clinical science-core knowledge area, and clinical science-exploration knowledge area).
In basic science-core knowledge areas, collaborations are related to the production of highly tacit and critical knowledge for the company. Consequently, firms need frequent short-distance interaction for the nature of the knowledge as well as to avoid knowledge leakage to achieve competitive advantages. Examples of this knowledge type are the early research projects in the well-established cardiovascular therapeutic area of Pfizer. In basic science-exploration knowledge areas, firms need to get access to the most novel knowledge from the academic community spread around the world (Gittelman Citation2007). In other words, access to basic science knowledge, highly tacit, involves more long-distance collaborations when a firm operates in an exploration area (Kale, Singh, and Perlmutter Citation2000; Cantwell and Santangelo Citation2000). In the case of MNCs with several R&D sites around the globe, firms in basic science-exploration knowledge areas are less likely to operate from a single local R&D centre. An example is the collaboration of Pfizer in the immunology field with Alliance for Lupus Research (ALR), in which ALR and Pfizer co-fund novel translational research projects driven by leading academic medical centres for lupus around the globe (Pfizer Citation2013).Footnote2
Thus we can derive our third hypothesis:
H3: The number of collaborations between a pharmaceutical MNC and an external organization is more negatively affected by geographical distance in basic science-core knowledge areas than in basic science-exploration knowledge areas.
In clinical science-core knowledge areas, the company has already experienced the clinical trial process for several previous drugs in the same therapeutic areas and it can more easily predict the success of a new compound based on internal knowledge accumulated (Henderson, Orsenigo, and Pisano Citation1999). Thus, this type of knowledge is expected to be more easily transferred across long distances. However, in clinical science-exploration knowledge areas, companies have to create routines and trust for a well-planned clinical trials process. Garud and Rappa (Citation1994) argued that geographical proximity helps to develop beliefs, routines, and technologies into well-defined marketable products. Consequently, we can hypothesize that the effect of geographical distance in exploration knowledge areas is more important than that in core knowledge areas in clinical science collaborations.
Thus we can derive the fourth hypothesis:
H4: The number of collaborations between a pharmaceutical MNC and an external organization is more negatively affected by geographical distance in clinical science-exploration knowledge areas than in clinical science-core knowledge areas.
4. Data sample
To test the hypotheses, we used scientific publications of pharmaceutical MNCs. Publications have been a common source to measure and analyse collaborations between organizations (Tijssen, Waltman, and van Eck Citation2012). More specifically, a bibliometric record of a publication is a source of empirical information as it describes many characteristics of collaborations: composition (number of authors, year of publication), author´s affiliation(s) (name of the institution, country and city) and the knowledge areas in which the collaboration takes place (Tijssen Citation2009). Studying authors’ affiliation, therefore, makes it possible to identify interaction both inside (between a company’s different R&D sites and departments) and outside the company, and to identify the geographical distance between co-authors.
Focusing on the role that geographical proximity plays in collaborations, we selected publications from six pharmaceutical MNCs which belong to the top-20 largest companies according to revenue and R&D expenditure in 2012. From the top-20 largest companies, we selected 6 companies (Pfizer, AstraZeneca, Eli Lilly, GlaxoSmithKline, Novartis, and Takeda) taking into account the heterogeneity of MNCs in terms of strategies, cultural and geographic differences. Based on interviews with R&D managers of these firms, we also confirmed that they used different collaboration strategies. Selecting MNCs with different strategies, collaboration strategies, culture and geographic distribution make the results more generalized. For each of the companies, all publications from the period 2000–2012 were collected from Scopus. Only articles published in collaboration with at least one external organization were selected.
In order to categorize these co-publications according to the four knowledge types (basic science and clinical science, and core and exploration knowledge areas), we used the Medical Subject Heading (MeSH) terms, a comprehensive controlled vocabulary to classify publications and books in life science.Footnote3 Each article is characterized by a few keywords that describe the content of the publication. These keywords mainly consist of MeSH terms. The use of the MeSH terms allowed us to capture the heterogeneity among papers within each journal since each article is classified according to its knowledge areas (Leydesdorff, Rotolo, and Rafols Citation2012). This approach is more specific than the traditional CHI index that classifies the article based on the journal in which the article was published (Hamilton Citation2003).
The publications were categorized by the first dichotomy of knowledge types, basic science vs. clinical science knowledge area, using a methodology developed by Weber (Citation2013). Following Weber´s classification, each article was first categorized into three broad topic areas, depending on the MeSH term of the article, cells and molecules (C), animals and other complex organisms (A) and humans (H). Then these three topic areas were clustered into basic science and clinical knowledge classification. More specifically, articles categorized with an A and/or C but not an H were considered to contribute to the basic science knowledge area. This is because most of the research at this level is carried out on the cellular or molecular level or in simple animal models (pre-clinical phase). Articles categorized with at least H were considered to be contributing to the clinical science knowledge area. It is not until a drug enters the clinical phase that drugs are tested in humans. Articles that could not be categorized as A, C and H were excluded from the dataset.
In order to classify the articles according to the second dichotomy of knowledge types, core vs. exploration knowledge areas, we identified two core and two exploration knowledge areas for each of the six MNCs. To identify these areas we used information about a company’s drug pipeline and products on the market. We defined core knowledge areas as therapeutic areas in which the company already had in-house competencies. More specifically, these were areas where the companies carried out most of their research and clinical trials, and already had products on the market. Exploration knowledge areas are therapeutic areas that were not considered, yet, to be core knowledge areas, but were explored and researched in the company. The number of ongoing clinical trials in these areas was low or there were none and there were no products on the market.
To identify the areas we used information from of the company’s annual reports covering the period 2000 to 2012. More specifically, for each single year between 2000 and 2012, we classified the core areas of the company as the two areas with both the highest number of components in pre-clinical and clinical phases, the highest R&D expenditures, and the highest number of products already on the market. The exploration areas were the two areas with the lowest number of drugs in the pipeline, components in preclinical and phase 1, the lowest R&D expenditures, and no products on the market yet. Based on these criteria we obtained a list of the two core and two exploration areas for each year-company combination. Then, the two most frequently occurring core and exploration knowledge areas in the 12-year time period for each company were selected. To validate the final selection of core and exploration knowledge areas, we contacted R&D directors from each of the six selected companies and we asked them to identify two core and two exploration knowledge areas of their company from the yearly list. These R&D directors have a broad overview of the company’s historical and ongoing pipeline. They confirmed the final section of the core and exploration knowledge areas.
After the identification of the two core and the two exploration therapeutic areas for each of the companies, we extracted the publications belonging to these therapeutic areas, using the MeSH terms. MeSH terms are organized as a hierarchical tree. Each therapeutic area corresponds to several MeSH sub-heading terms that correspond to specific diseases. For more details, see in the Appendix 1.
Next, all author addresses of the final dataset were cleaned to eliminate spelling variations and a single standardized name was added to each external organization and MNC R&D sites. An article can list multiple different affiliations, both external organizations and MNCs, and multiple addresses of the same organization. In this study the definition of external organization is location-based, meaning that different locations of an organization are considered to be different collaborators. For example, University of Washington, New York, is a different external organization from University of Washington, Seattle. The same definition is used for the MNC R&D sites; Pfizer, London, is a different organization from Pfizer, New York.
Finally, the cities of the external organizations and MNC R&D sites were used to determine the geographical coordinates (longitude, latitude) of each location based on Google Maps. In addition, the external organizations were classified according to the type of sector: industry, hospital, university, institute and other to account for the importance of university and hospital to basic science and clinical knowledge as mentioned before.
The final dataset included 24 561 publications: 4 707 in basic science and 19 854 in clinical science, 2 321 in basic science-core knowledge areas, 2 386 in basic science-exploration knowledge areas, 11 659 in clinical science-core knowledge areas, and 8 195 in clinical science-exploration knowledge areas. In the next sections 5 and 6, we first investigate the geographical distribution of the collaborations from the dataset of 24 561 publications and then we build a regression model to test the hypotheses.
5. Geographical distribution of the number of collaborations
We observe that the firms collaborate intensively with external organizations at different geographical distances. summarizes the basic statistics of the number of collaborations and geographical distances between an MNC R&D site and an external organization classified according to knowledge type. The number of collaborations in clinical science knowledge areas is higher than in basic science knowledge areas. Looking at geographical distance, the geographical distance of collaboration with the external organizations ranges from 0 km to almost 20 000 km. The distribution of the distance is skewed as 25% of collaborations takes place within 400 km. In addition, 10% of the collaborations in clinical science core knowledge areas and clinical science exploration knowledge areas are clustered between 6 000 km and 7 000 km. This cluster is related to collaborations at the distance between Europe and USA, the two major players in the pharmaceutical industry. In the literature, other studies have found different sharp drop-offs in collaborations at a specific distance. Bottazi and Peri (Citation2003) found, using patent data, that research and development spillovers exist within a boundary of 480 km and fall sharply beyond that point. Gittelman (Citation2007) studied biotechnology firms and found that 20% of collaborative research papers have an average distance among coauthors of less than 80 km. More information about the distribution of the distance in the knowledge areas is provided in in the Appendix 1.
Table 1. Descriptive statistics of the pair between MNC R&D sites and external organizations with at least one collaboration.
explores the relationship between the mean of the number of collaborations and geographical distance in the different knowledge areas. In graph 1, the x-axis shows the distance between an MNC R&D site and an external organization clustered in geographical distance intervals, and the y-axis shows the mean number of collaborations between an external organization and an MNC R&D site at each interval of distances. The mean of number of collaborations is calculated clustering the collaborations in eight geographical distance intervals (0–100 km, 100–500 km, 500–1 000 km, 1 000–2 000 km, 2 000–5 000 km, 5 000–8 000 km, 8 000–10 000 km, >10 000 km). distinguishes between basic science knowledge areas and clinical science knowledge areas. compares basic science-core knowledge areas, basic science-exploration knowledge areas, clinical science-core knowledge areas and clinical science-core knowledge areas.
Graph 1. Mean number of collaborations for different geographical distance intervals between MNC R&D sites and external organizations with confidence intervals. Geodist intervals (0–100 km, 100–500 km, 500–1 000 km, 1 000–2 000 km, 2 000–5 000 km, 5 000–8 000 km, 8 000–10 000 km, >10 000 km).
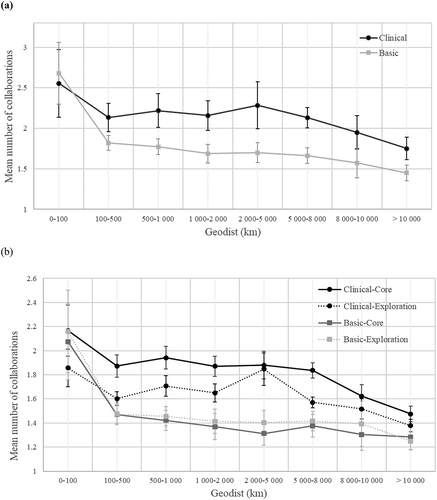
The mean number of collaborations decreases with geographical distance in all knowledge areas. In , the mean number of collaborations with external organizations within 100 km is higher for basic science knowledge areas than for clinical science knowledge areas. Beyond the 100 km, we observed the opposite result. The mean of the number of clinical science knowledge area collaborations is always higher than that of basic science areas. In , the mean of the number of collaborations is higher in clinical science-core knowledge areas than in clinical science exploration knowledge areas in all the intervals. We observed the opposite result in basic science knowledge areas. In more detail, we can observe the highest decrease of the mean number of collaborations between the first (within 100 km) and the second interval (100–500 km) in both and . In , the mean number of collaborations in basic science knowledge areas decreases by 32% compared to a 16 % decrease in clinical science knowledge areas. In , the mean number of collaboration decreases by 29% in basic science-core knowledge areas, 32% in basic science-exploration knowledge areas, 13% in clinical science-core knowledge areas, and 14% clinical science-exploration knowledge areas.
6. Regression model
To further explore the link between geographical distance and number of collaborations and to test the hypotheses on the differential effect in different knowledge areas, we built a regression model. The dataset of the 24 561 publications involves 742 MNC R&D sites and 17 631 external organizations. However, we observed that some external organizations collaborate with all the six MNCs studied, some with few, and some with only one MNC. We therefore assume that all external partners of an MNC R&D site are also potential external partners of all other MNC R&D sites in our dataset. This resulted in a matrix of collaborations that pairs all the possible 742 MNC R&D sites with the 17 631 external organizations. This matrix includes a large number of zeros that has to be corrected for as the majority of MNC R&D site-external organization pairs do not collaborate at all. Hence our choice for the zero-inflated model which we explain below. The total number of observations amounts to 742 × 17 631 = 13 082 202 pairs. Because our analysis distinguished between basic and clinical science, the collaborations between a MNC R&D site and an external organization are doubled resulting in 13 082 202 × 2 = 26 164 404 pairs. The same logic applies when we add the classification in exploration and core therapeutic areas, resulting in 26 164 404 × 2 = 52 328 808 observations.
In the next section, we build a zero inflated gravity model on the matrixes of collaborations.
6.1. Zero-inflated gravity model
In the empirical analysis, we adopted a spatial interaction modelling perspective to explain how geographical distance influences the number of collaborations between a pharmaceutical MNC R&D sites and an external organization, depending on the knowledge dimensions involved. In the geography literature, the gravity model has been used to model the spatial interaction between locations (Ponds, van Oort, and Frenken Citation2007; Hoekman, Frenken, and van Oort Citation2009; Plotnikova and Rake Citation2014; Berge Citation2016). The gravity model for spatial interaction is based on Newton´s law of universal gravity. It is defined by a function characterizing the origin i of an interaction, a function characterizing the destination j of an interaction and a function characterizing the interaction between i and j. The basic equation can be expressed as:
where is the interaction intensity between the two entities i and j, i.e. the number of collaborations between an MNC R&D site and an external organization. We indicated the city-level location of the six MNCs’ R&D sites by i = 1,…,m and the city-level location of the external organizations by j = 1,…,n.
is an m-by-n matrix of the possible R&D collaborations between MNC R&D sites and external organizations. Traditionally,
are, respectively, called mass of origin and destination; in the context of this paper,
characterizes the total number of collaborations of an MNC R&D site i;
characterizes the number of publications of the external organizations j with the six MNCs selected.
The focus of interest of the study is on the separation function that integrates all the effects of the geographical and knowledge types on the number of collaborations between pharmaceutical MNC i and external organization j. The separation factor between the two entities
can be expressed by:
where is a factor that measures the separation between the two entities, i.e. the spatial distance between the two organizations.
are the parameters to be estimated.
We include separation measures.
is the spatial distance (in km) between the locations of the city of the MNC i and of the external organization j involved in the collaboration.
represents whether the collaboration takes place within the same country or not. In addition, we added 2 knowledge measures describing the knowledge area of the collaborations.
is a knowledge variable that distinguishes whether the pair represents co-publications classified as basic science or clinical science.
is a knowledge variable that classifies whether the pair represents co-publication as belonging to an exploration knowledge area or a core knowledge area of the company.
and
are, respectively, the interaction terms of
and
with
. The specification of these variables is discussed in more detail in section 6.2.
Integrating the origin, destination, and separation functions into EquationEquation (1)(1)
(1) , the empirical model can be written in this way:
At this point we are interested in estimating the parameters ,
and
. Since the dependent variable (
) is a non-negative and an integer variable, we cannot estimate the model using Ordinary Least Squares (OLS). The basic count model is the Poisson estimation that requires equidispersion, where the variance equals the mean. In order to correct for overdispersion in the dataset, by letting the parameters for each pair of MNC R&D site and external organization have a random distribution of its own, we used the negative binominal regression model. In addition, our dataset exhibits an excess of zero counts (the incidence of zero counts is greater than would be expected for the Poisson distribution as most of the pairs of an MNC and an external organization do not collaborate together) and we need to correct for it in order to avoid incorrect and biased estimations. Consequently, the examination of the factors affecting the geographical logic of pharmaceutical collaborations is analysed through a zero-inflated regression model. This approach uses two-component mixture models, combining a point mass at zero with a count distribution such as negative binomial. The first part contains a logit regression estimating the effect of the predictor variables on the probability that there is no interaction between a big pharma and an external organization at all. The second part contains a negative binomial regression on the probability of each count for the group that has a non-zero probability of count other than zero. Thus, the zero-inflated model distinguishes between two sources of zero: strictly zero counts and a group having a non-zero probability of counts other than zero (Burger, van Oort, and Linders Citation2009). Finally, we tested whether the choice of the negative binomial model is appropriate for the Vuong test for all the regressions mentioned above. The likelihood ratio test of overdispersion is significant, showing that the zero-inflated binomial model is favoured over a zero-inflated Poisson model in all cases.
6.2. Variables definition of the gravity model
The gravity model estimates the effect of geographical distance on the number of collaborations between MNCs and external organizations. The mass of an MNC
and an external collaboration
is included in the model by their product in order to monitor the expected collaboration intensity between the two organizations,
The separation and knowledge terms or independent variables are defined as:
, called Geodist, is a continuous positive variable of the spatial distance (in km) between the location of the city of the MNC R&D sites i and of the external organization j involved in the collaboration. The value is calculated between the longitudinal and latitudinal points of the towns where the external partner and the MNC are located (min = 0). Note that we add 1 to the
variable to allow the logarithmic transformation. This transformation prevents the results from being determined by skewed or high values derived from longer distances.
, called Country, is a dummy variable coded 1 if the collaboration takes place within the same country and 0 otherwise. This variable is important in order to capture the differences in distance and policy and the legal system between countries.
, called Clin, is a dummy variable that is coded 1 for clinical science knowledge and 0 for basic science knowledge publications.
is the interaction terms of
, called Expl, is a dummy variable that is coded 1 for exploration knowledge and 0 for core knowledge area publications.
is the interaction terms of
.
In addition to these independent variables, we take into account the characteristics of the collaboration and the type of the external organization, creating three dummy variables: BP_Loc, Univ as university, and Hosp as hospital. BP_Loc is a dummy variable coded 1 if the external organization is located in the same city as any of the R&D sites of the collaborative MNC, 0 otherwise. This variable refers to any R&D site of the collaborative MNC and not necessarily the R&D site that is part of that respective pair. It captures the importance of being situated in the same city as an MNC that can function as a temporary location for collaborations at long distance (Davids and Frenken Citation2017). University and hospital are the most important partners of pharmaceutical companies when it comes to collaboration in basic and clinical science knowledge (Henderson and Cockburn Citation1996, Cockburn and Henderson Citation2001). We also considered the characteristics of the big pharmaceutical companies creating categorical variables for each of the selected six MNCs (), since companies may develop and adopt different strategies concerning collaborations.
From the Kernel density analysis in in the Appendix 1, we observed that the dataset is clustered in particular geographic distances. In the gravity model, we have controlled for the cluster distances adding a control variable for each cluster. However, the results of the estimation do not change compared to the ones without these control variables. For simplicity of the model, we decided not to include the control variables for cluster distances in the gravity model.
Explaining the different measures of distances and the control variables, the complete gravity equation to be estimated is:
6.3. Results of the regression model
shows the descriptive statistics of the key variables from the matrixes of collaborations. The mean of the intensity of collaborations is very small, due to the large majority of collaborations being equal to zero.
Table 2. Descriptive statistics of all possible pairs between R&D sites and external organizations.
shows the correlation matrix to identify a possible multi-correlation effect. The correlation values are low for all the variables considered.
Table 3. Correlation matrix. *p < 0.05.
To test the 4 hypotheses, we estimated 6 different models. Model A is the baseline model with only the separation term LnGeodist. Model B includes the knowledge term Clin to test the impact of the knowledge dimensions, basic science vs. clinical science, and its interaction term with LnGeodist. Finally, module C adds the other knowledge term Expl and its interaction with LnGeodist to the model B, referring to the other 4 knowledge areas (basic science-core knowledge areas, basic science-exploration areas, clinical science-core knowledge areas, and clinical science-exploration knowledge areas). Models D, E and F add the other separation term Country variable to models A, B and C in order to capture the differences in distance, in policy and in the legal system in Europe, the USA and Japan. Models A and D test hypothesis 1, the influence of geographical proximity on the number of collaborations. Model C and E test hypothesis 2 that compares the influence of geographical proximity on the number of collaborations in basic science and clinical science knowledge areas. Finally, models D and F compare the influence of geographical proximity on the number of collaborations in basic science-core, basic science-exploration, clinical science–core, and clinical science-exploration knowledge areas that are tested in hypotheses 3 and 4.
and present the estimates of the six regression models, showing a negative binomial regression part, a zero-inflated part, and some general fit statistics. Specifically, shows the results of the first three regression models (A, B, C). In model A, the elasticity of the number of collaborations with respect to LnGeodist is significant and negative. Geographical distance negatively affects the number of collaborations, which shows strong support for hypothesis 1 and is in line with the previous literature. Model B includes the dummy variable Clin and its interaction effect LnGeodist*Clin. The interaction term between LnGeodist and Clin is positive and significant (
The number of collaborations is more negatively affected by geographical distance in basic science knowledge areas than in clinical science knowledge areas, thus providing support for hypothesis 2.
Table 4. Zero-inflated negative binomial regression model testing the number of collaborations of pharmaceutical MNCs for the period 2000–2012. Dependent variable: number of collaborations.
Table 5. Zero-inflated negative binomial regression model testing the number of collaborations of pharmaceutical MNCs for the period 2000–2012. Dependent variable: number of collaborations.
Finally, model C adds the other knowledge term Expl and its interaction term LnGeodist*Expl to model B. The interaction term between LnGeodist and Expl variables is positive and significant ( In both basic science and clinical science knowledge areas, the number of collaborations is more negatively affected by geographical distance in core knowledge areas than in exploration knowledge areas. These results support hypothesis 3 but reject hypothesis 4.
shows the results of the other 3 models (D, E and F), adding the other spatial separation term, Country, to models A, B and C. The inclusion of the Country variable diminishes the elasticity of the number of collaborations with respect to LnGeodist. The variable is significant and highly positive in all three models, indicating that a pair of external organization and MNC R&D site belonging to the same country collaborates more frequently than a pair from different countries. Models D, E, and F arrive at the same conclusions as models A, B, and C. The results provide strong support for hypotheses 1, 2, and 3 but reject hypothesis 4.
Considering the control variables, the BP_Loc variable is always negative and significant in all the models, which indicates that the number of collaborations decreases when the external organization is located in the same city as the MNC R&D site. The Hosp variable has a significant and positive influence on the number of external collaborations. However, the Univ variable is positive and significant only in models D, E and F.
7. Discussion and conclusions
This paper has examined the influence of geographical proximity on the number of R&D collaborations in pharmaceutical MNCs in different knowledge types. Previous literature has investigated the geographical distance of collaborations. However, the link between the geographical dimension and types of knowledge is largely understudied. This paper contributes to filling this gap by providing a better understanding of the factors that influence a firm to collaborate with close or distant partners. In doing so, we look beyond the organizational level and find that different types of knowledge involved in collaboration are associated with different geographical distances in a pharmaceutical MNC’s collaborations.
The baseline results confirm previous literature that shows that geographical proximity matters in collaborations established by pharmaceutical MNCs (Ponds, van Oort, and Frenken Citation2007; Douglas et al. Citation2010; Abramovsky et al. Citation2011; Plotnikova and Rake Citation2014). Being located in the same country facilitates the number of collaborations. We add that the number of collaborations is less affected by geographical distance in clinical science than in basic science, which confirms our second hypothesis. Clinical science knowledge is developed from a strongly regulated process that is thoroughly documented. Therefore this codified knowledge can more easily be transferred through collaborations that are more geographically distant. Basic science knowledge, on the other hand, is performed in more local networks because of its highly tacit and serendipitous nature. That is in line with the results from D’Amore et al. (Citation2013). In addition, firms located close to their partners can respond quickly to emerging innovation opportunities and hire highly skilled professionals, such as star scientists, that are crucial to the development of basic science knowledge (Zucker, Darby, and Armstrong Citation2002). The importance of geographical proximity in basic science can be seen for example in the ‘Centers for Therapeutic Innovation (CTI)’ program created by Pfizer.Footnote4 Pfizer created local centres to build bilateral collaboration, combining top expertise in basic science from academia with development capabilities and research technologies from industry. The CTI laboratories are small, flexible and semi-autonomous units. Pfizer employees work side by side with researchers from the academic medical centres. Finally, it is important to point out that the globalization of the pharmaceutical industry and in particular of clinical trial activity can be an additional explanation of the longer distance in collaboration in clinical areas observed (Glickman et al. Citation2009; Hoekman et al. Citation2012). Pharmaceutical companies have started to offshore clinical trials to non-traditional clinical research countries away from the R&D sites of the pharmaceutical companies or coordinated from distant R&D sites. This geographic change in the clinical trials is widely attributed to cost reductions and efficiency improvement in data generation trials (Glickman et al. Citation2009). However, Haeussler and Rake (Citation2016) have shown that the host countries’ knowledge base is also an important determinant of countries´ attractiveness for offshored clinical trials.
In both basic science and clinical science, the number of collaborations is more influenced by geographical distance in core knowledge areas than in exploration knowledge areas. These results confirm the third hypothesis but reject the fourth. Collaborations in core knowledge areas often rely on trust and strong ties that have been built up over the years. Frequent face-to-face interaction is necessary, which is more easily achieved when partners are closely located. In addition, products in core areas are the main income sources and companies therefore need to prevent knowledge leakage to achieve competitive advantages. In clinical science core knowledge areas MNCs have compounds at the last phases of clinical trials. This is a result of large investments in time, resources and capital. To be able to profit from direct feedback from clinical scientists and properly manage these trials and involved partners, geographical proximity is important. However, in exploration areas companies are exploring possible new areas to invest in. Therefore, it is necessary to search for the newest and most relevant knowledge independently of where partners are located.
The traditional economic literature has mainly focused on physical infrastructure, policy instruments, labour costs, the phase of a firm’s life cycle and the interaction between the strength of local externalities and firms´ skills as location driver factors for MNCs (Basile and Kayam Citation2015). According to the knowledge-based theory, however, knowledge is the most important resource of a firm. But knowledge has only been investigated to a limited extent in the MNC location strategy literature. In this article, we have shown that the type of knowledge is an important location driver to take into account when understanding a company’s collaboration strategy.
From a policy perspective, the importance of knowledge type in R&D collaboration supports the view that a country’s knowledge base, as well as the availability of science talent, determines countries’ attractiveness for offshored R&D services (Manning, Silvia, and Lewin Citation2008; Lewin, Massini, and Peeters Citation2009). This adds to existing studies by claiming that knowledge development activities are not merely relocated to non-traditional countries because of cost advantages and diversification of market (Haeussler and Rake Citation2016). In addition, the association between geography and knowledge types in R&D collaborations puts emphasis on explicit considerations of geography in R&D management and funding efforts at multiple spatial scales.
Acknowledgments
We acknowledge the financial support of the Ragnar Söderberg foundation (Grant E99/12).
Disclosure statement
No potential conflict of interest was reported by the authors.
Notes
1 National Science Foundation, 2016. https://www.nsf.gov/statistics/2017/nsf17305/#definitions.
2 (Pfizer Citation2013). ‘Pfizer Annual Report.’
3 Medical Subject Headings (MeSH®) https://meshb.nlm.nih.gov/search.
4 Centers for Therapeutic Innovation (Pfizer) https://www.pfizercti.com.
References
- Abramovsky, L., E. Battistin, E. Fitzsimons, A. Goodman, and H. Simpson. 2011. “Providing Employers with Incentives to Train Low-Skilled Workers: Evidence from the UK Employer Training Pilots.” Journal of Labor Economics 29 (1): 153–193. doi:10.1086/656372.
- Alcácer, J., and W. Chung. 2007. “Location Strategies and Knowledge Spillovers.” Management Science 53 (5): 760–776. doi:10.1287/mnsc.1060.0637.
- Almeida, P. 1996. “Knowledge Sourcing by Foreign Multinationals: Patent Citation Analysis in the U.S. Semiconductor Industry.” Strategic Management Journal 17 (S2): 155–165. doi:10.1002/smj.4250171113.
- Arora, A., and A. Gambardella. 1990. “Complementarity and External Linkages: The Strategies of the Large Firms in Biotechnology.” The Journal of Industrial Economics 38 (4): 361. doi:10.2307/2098345.
- Audretsch, D. B., and M. P. Feldman. 1996. “R&D Spillovers and the Geography of Innovation and Production R&D Spillovers and the Geography of Innovation and Production.” Source: The American Economic Review 86 (3): 630–640. doi:10.2307/2118216.
- Basile, R., and S. Kayam. 2015. “Empirical Literature on Location Choice of Multinationals.” Complexity and Geographical Economics 325–351. doi:10.1007/978-3-319-12805-4_13.
- Bathelt, H., A. Malmberg, and P. Maskell. 2004. “Clusters and Knowledge: Local Buzz, Global Pipelines and the Process of Knowledge Creation.” Progress in Human Geography 28 (1): 31–56. doi:10.1191/0309132504ph469oa.
- Berge, L. R. 2016. “Network Proximity in the Geography of Research Collaboration.” Papers in Regional Science 2016. doi:10.1111/pirs.12218.
- Boschma, R. A. 2005. “Proximity and Innovation: A Critical Assessment.” Regional Studies 39 (1): 61–74. doi:10.1080/0034340052000320887.
- Bottazzi, L., and G. Peri. 2003. “Innovation and Spillovers in Regions: Evidence from European Patent Data.” European Economic Review 47.4: 687–710. doi:10.1016/S0014-2921(02)00307-0
- Burger, M., F. van Oort, and G. J. Linders. 2009. “On the Specification of the Gravity Model of Trade: Zeros, Excess Zeros and Zero-Inflated Estimation.” In Spatial Economic Analysis 4: 167–190. doi:10.1080/17421770902834327.
- Calero, C., T. N. Van Leeuwen, and R. J. W. Tijssen. 2007. “Research Cooperation within the Bio-Pharmaceutical Industry: Network Analyses of Co-Publications within and between Firms.” Scientometrics 71 (1): 87–99. doi:10.1007/s11192-007-1650-y.
- Cantwell, J., and G. D. Santangelo. 2000. “Capitalism, Profits and Innovation in the New Techno-Economic Paradigm.” Journal of Evolutionary Economics 10 (1–2): 131–157. doi:10.1007/s001910050009.
- Cantwell, J., and R. Mudambi. 2011. “Physical Attraction and the Geography of Knowledge Sourcing in Multinational Enterprises.” Global Strategy Journal 1 (3–4): 206–232. doi:10.1111/j.2042-5805.2011.00024.x.
- Cockburn, I. M., and R. M. Henderson. 2001. “Scale and Scope in Drug Development: Unpacking the Advantages of Size in Pharmaceutical Research.” Journal of Health Economics 20 (6): 1033–1057. doi:10.1016/S0167-6296(01)00108-4.
- Cohen, W. M., and D. A. Levinthal. 1989. “Innovation and Learning: The Two Faces of R & D.” The Economic Journal 99 (397): 569. doi:10.2307/2233763.
- Cohen, W. M., and D. A. Levinthal. 1990. “Absorptive Capacity: A New Perspective on Learning and Innovation.” Administrative Science Quarterly 35 (1): 128. doi:10.2307/2393553.
- Cowan, R., P. A. David, and D. Foray. 2000. “The Explicit Economics of Knowledge Codification and Tacitness.” Industrial and Corporate Change 9 (2): 211–253. doi:10.1093/icc/9.2.211.
- D’Amore, Rosamaria, Roberto Iorio, Sandrine Labory, and Agnieszka Stawinoga. 2013. “Research Collaboration Networks in Biotechnology: Exploring the Trade-Off between Institutional and Geographic Distances.” Industry and Innovation 20 (3): 261–276. doi:10.1080/13662716.2013.791127.
- David, P. A., and D. Foray. 1995. “Accessing and Expanding the Science and Technology Knowledge Base.” Sciente Technology Industry Review 16 (94). https://infoscience.epfl.ch/record/52986
- Davids, M., and K. Frenken. 2017. “Proximity, Knowledge Base and the Innovation Process: Towards an Integrated Framework.” Regional Studies. March 1–12. doi:10.1080/00343404.2017.1287349.
- Douglas, F. L., V. K. Narayanan, L. Mitchell, and R. E. Litan. 2010. “The Case for Entrepreneurship in R&D in the Pharmaceutical Industry.” Nature Reviews Drug Discovery. Nature Publishing Group. doi:10.1038/nrd3230.
- Feldman, M. P., and F. R. Lichtenberg. 2000. “The Impact and Organization of Publicly-Funded Research and Development in the European Community.” In Economics and Econometrics of Innovation (pp. 177–200). Boston, MA: Springer. doi:10.3386/w6040.
- Freeman, C. 1991. “Networks of Innovators: A Synthesis of Research Issues.” Research Policy 20 (5): 499–514. doi:10.1016/0048-7333(91)90072-X.
- Garud, R., and M. A. Rappa. 1994. “A Socio-Cognitive Model of Technology Evolution: The Case of Cochlear Implants.” Organization Science 5 (3): 344–362. doi:10.1287/orsc.5.3.344.
- Gassmann, O., and G. Reepmeyer. 2005. “Organizing Pharmaceutical Innovation: From Science-Based Knowledge Creators to Drug-Oriented Knowledge Brokers.” Creativity and Innovation Management 14 (3): 233–245. doi:10.1111/j.1467-8691.2005.00344.x.
- Gertler, M. S. 2003. “Tacit Knowledge and the Economic Geography of Context, or the Undefinable Tacitness of Being (There).” Journal of Economic Geography 3 (1): 75–99. doi:10.1093/jeg/3.1.75.
- Gittelman, M. 2007. “Does Geography Matter for Science-Based Firms? Epistemic Communities and the Geography of Research and Patenting in Biotechnology.” Organization Science 18 (4): 724–741. doi:10.1287/orsc.1070.0249.
- Glickman, S. W., J. G. McHutchison, E. D. Peterson, C. B. Cairns, R. A. Harrington, R. M. Califf, and K. A. Schulman. 2009. “Ethical and Scientific Implications of the Globalization of Clinical Research.” New England Journal of Medicine 360 (8): 816–823. doi:10.1056/NEJMsb0803929.
- Grant, R. M. 1996. “Prospering in Dynamically-Competitive Environments: Organizational Capability as Knowledge Integration.” Organization Science 7 (4): 375–387. doi:10.1287/orsc.7.4.375.
- Haeussler, C., and B. Rake. 2016, October. “The Changing Geography of Clinical Research: A Critical Analysis of Its Drivers.” SSRN Electronic Journal. doi:10.2139/ssrn.2846672.
- Hamilton, K. 2003. “Subfield and Level Classification of Journals. Document regarding the CHI classification from CHI Research.” Inc. CHI 2012 (2003).
- Hansen, M. T., N. Nohria, and T. Tierney. 1999. “What’s Your Strategy for Managing Knowledge?” Harvard Business Review 77 (2): 106–16, 187.
- Henderson, R. 1994. “The Evolution of Integrative Capability: Innovation in Cardiovascular Drug Discovery.” Industrial and Corporate Change 3 (3): 607–630. doi:10.1093/icc/3.3.607.
- Henderson, R., and L. Cockburn. 1996. “Scale, Scope, and Spillovers: The Determinants of Research Productivity in Drug Discovery.” The RAND Journal of Economics Journal of Economics 27 (1): 32–59. doi:10.2307/2555791.
- Henderson, R., L. Orsenigo, and G. P. Pisano. 1999. “The Pharmaceutical Industry and the Revolution in Molecular Biology: Interactions among Scientific, Institutional, and Organizational Change.” The Sources of Industrial Leadership, 267–311. doi:10.1023/A:1011124621952.
- Hippel, E. V. 1994. “‘Sticky Information’ and the Locus of Problem Solving: Implications for Innovation.” Management Science 40 (4): 429–439. doi:10.1287/mnsc.40.4.429.
- Hoekman, J., K. Frenken, D. De Zeeuw, and H. L. Heerspink. 2012. “The Geographical Distribution of Leadership in Globalized Clinical Trials.” PLoS One7 (10).doi:10.1371/journal.pone.0045984.
- Hoekman, J., K. Frenken, and F. van Oort. 2009. “The Geography of Collaborative Knowledge Production in Europe.” Annals of Regional Science 43 (3 SPEC. ISS.): 721–738. doi:10.1007/s00168-008-0252-9.
- Jaffe, A., M. Trajtenberg, and R. Handerson. 1993. “Geographic Localization of Knowledge Spillovers as Evidenced by Patent Citation.” The Quarterly Journal of Economics 108 (3): 577–598. doi:10.2307/2118401.
- Kale, P., H. Singh, and H. Perlmutter. 2000. “Learning and Protection of Proprietary Assets in Strategic Alliances: Building Relational Capital.” Strategic Management Journal 21 (3): 217–237. doi:10.1002/(SICI)1097-0266(200003)21:3<217::AID-SMJ95>3.3.CO;2-P.
- Kessel, M. 2011. “The Problems with Today’s Pharmaceutical Business—An Outsider’s View.” Nature Biotechnology 29 (1): 27–33. doi:10.1038/nbt.1748.
- Kogut, B., and U. Zander. 1992. “Knowledge of the Firm, Combinative Capabilities, and the Replication of Technology.” Organization Science 3 (3): 383–397. doi:10.1287/orsc.3.3.383.
- Lewin, A. Y., S. Massini, and C. Peeters. 2009. “Why are Companies Offshoring Innovation? the Emerging Global Race for Talent.” Journal of International Business Studies 40 (6): 901–925. doi:10.1057/jibs.2008.92.
- Leydesdorff, L., D. Rotolo, and I. Rafols. 2012. “Bibliometric Perspectives on Medical Innovation Using the Medical Subject Headings of PubMed.” Journal of the American Society for Information Science and Technology 63 (11): 2239–2253. doi:10.1002/asi.22715.
- Lim, K. 2004. “The Relationship between Research and Innovation in the Semiconductor and Pharmaceutical Industries (1981-1997) Research.” Research Policy 33 (2): 287–321. doi:10.1016/j.respol.2003.08.001.
- Manning, S., M. Silvia, and A. Y. Lewin. 2008. “A Dynamic Perspective on Next-Generation Offshoring: The Global Sourcing of Science and Engineering Talent.” Academy of Management Perspectives 22 (3): 35–54. doi:10.5465/AMP.2008.34587994.
- March, J. G. 1991. “Exploration and Exploitation in Organizational Learning.” Organization Science 2 (1): 71–87. doi:10.1287/orsc.2.1.71.
- Mattes, J. 2012. “Dimensions of Proximity and Knowledge Bases: Innovation between Spatial and Non-Spatial Factors.” Regional Studies 46 (8): 1085–1099. doi:10.1080/00343404.2011.552493.
- Moodysson, J. 2009. “Principles and Practices of Knowledge Creation: On the Organization of ‘Buzz’ and ‘Pipelines’ in Life Science Communities.” Economic Geography 84 (4): 449–469. doi:10.1111/j.1944-8287.2008.00004.x.
- Moodysson, J., L. Coenen, and B. Asheim. 2008. “Explaining Spatial Patterns of Innovation: Analytical and Synthetic Modes of Knowledge Creation in the Medicon Valley Life-Science Cluster.” Environment and Planning A 40 (5): 1040–1056. doi:10.1068/a39110.
- Owen-Smith, J., and W. W. Powell. 2004. “Knowledge Networks as Channels and Conduits: The Effects of Spillovers in the Boston Biotechnology Community.” Organization Science 15 (1): 5–21. doi:10.1287/orsc.1030.0054.
- Paci, R., and S. Usai. 2000. “The Role of Specialisation and Diversity Externalities in the Agglomeration of Innovative Activities.” Rivista Italiana Degli Economisti, SIE - Societa’ Italiana Degli Economisti 2 (2): 237–268. doi:10.2139/ssrn.192948.
- Pfizer Company. 2013. Pfizer Annual Report 2013. https://www.pfizer.com/files/investors/financial_reports/annual_reports/2013/index.htm
- Plotnikova, T., and B. Rake. 2014. “Collaboration in Pharmaceutical Research: Exploration of Country-Level Determinants.” Scientometrics 98 (2): 1173–1202. doi:10.1007/s11192-013-1182-6.
- Polanyi, M. 1960. “Personal Knowledge: Towards a Post-Critical Philosophy.” American Sociological Review 25 (4): 582. doi:10.2307/2092944.
- Ponds, R., F. van Oort, and K. Frenken. 2007. “The Geographical and Institutional Proximity of Research Collaboration.” Papers in Regional Science 86 (3): 423–443. doi:10.1111/j.1435-5957.2007.00126.x.
- Powell, W. W., and S. Grodal. 2005. “Networks of Innovators.” The Oxford Handbook of Innovation78. doi:10.1093/oxfordhb/9780199286805.003.0003.
- Scherngell, T. 2013. The Geography of Networks and R&D Collaborations. No. DEMO-BOOK-2017-025. Heidelberg: Springer. doi:10.1007/978-3-319-02699-2.
- Smith, K. G., C. J. Collins, and K. D. Clark. 2005. “Existing Knowledge, Knowledge Creation Capability, and the Rate of New Product Introduction in High-Technology Firms.” Academy of Management Journal 48 (2): 346–357. doi:10.5465/AMJ.2005.16928421.
- Tijssen, R. J. W. 2009. “Internationalisation of Pharmaceutical R&D: How Globalised are Europe’s Largest Multinational Companies?” Technology Analysis and Strategic Management 21 (7): 859–879. doi:10.1080/09537320903182330.
- Tijssen, R. J. W., L. Waltman, and N. J. van Eck. 2012. “Research Collaboration and the Expanding Science Grid : Measuring Globalization Processes Worldwide.”arXiv preprint arXiv:1203.4194.
- Vatne, E. 2001. “Local versus Extra-Local Relations: The Importance of Ties to Information and the Institutional and Territorial Structure of Technological Systems.” Annual Residential Conference of the IGU Commission on the Dynamics of Economic Spaces in Turin.
- Waltman, L., R. J. W. Tijssen, and N. J. van Eck. 2011. “Globalisation of Science in Kilometres.” Journal of Informetrics 5 (4): 574–582. doi:10.1016/j.joi.2011.05.003.
- Weber, G. M. 2013. “Identifying Translational Science within the Triangle of Biomedicine.” Journal of Translational Medicine 11 (1): 126. doi:10.1186/1479-5876-11-126.
- Zucker, L. G., and M. R. Darby. 1996. “Star Scientists and Institutional Transformation: Patterns of Invention and Innovation in the Formation of the Biotechnology Industry.” Proceedings of the National Academy of Sciences 93 (23): 12709–12716. doi:10.1073/pnas.93.23.12709.
- Zucker, L. G., M. R. Darby, and J. S. Armstrong. 2002. “Commercializing Knowledge: University Science, Knowledge Capture, and Firm Performance in Biotechnology.” Management Science 48 (1): 138–153. doi:10.1287/mnsc.48.1.138.14274.
Appendix 1
Table A1. Classification of the articles into therapeutic areas through the heading MeSH term.