ABSTRACT
We investigate what fosters or inhibits data sharing behaviour in a sample of 173 innovation management researchers. Theoretically, we integrate resource-based arguments with social exchange considerations to juxtapose the trade-off between data as a proprietary resource for researchers and the benefits that reciprocity in academic relations may provide. Our empirical analysis reveals that the stronger scholars perceive the comparative advantage of non-public datasets, the lower the likelihood of data sharing. Expected communal benefits may increase the likelihood of data sharing, while negative perceptions of increased data scrutiny are consequential in inhibiting data sharing. Only institutional pressure may help to solve this conundrum; most respondents would therefore like to see journal policies that foster data sharing.
1. Introduction
In 2015, Carlos Moedas (Citation2015), the European Commissioner for Research, Science and Innovation at this time, introduced the three Os – open innovation, open science, and open to the world – as goals for research and innovation policies in the EU. Open innovation focuses on firms collaborating with their environment to gather and supply new approaches and technologies (Chesbrough Citation2003). Open science concentrates on improving the input and impact to and from research (Nosek et al. Citation2015). Open to the world highlights the need for partnerships across country or disciplinary borders (Moedas Citation2015).
In this article, our focus is on open science, a concept often connoted with transparency, accessibility, collaboration, and above all, sharing: ‘Open science is transparent and accessible knowledge that is shared and developed through collaborative networks’ (Vicente-Sáez and Martínez-Fuente Citation2018: 428). Within the science domain, we investigate a research field that should – by definition – be more enamoured of openness: innovation research, where researchers have strongly advocated the importance of openness for all types of innovation outcomes (e.g. Bogers et al. Citation2017; von Hippel Citation2017). Numerous studies in the innovation literature argued for the benefits of open data sharing and collaborations for more innovative outcomes (see West et al. (Citation2014) and the references therein). In addition, innovation management scholars showed that knowledge-sharing and collaboration not only advances organisational innovation activities, but also benefits society (Lee et al. Citation2018).
Yet, despite the widely acclaimed benefits that data sharing could offer for subsequent research and societal benefits (Molloy Citation2011), many researchers in the management sciences prefer to keep their data private (Tenopir et al. Citation2011). DataVerse features more than 98,000 open analysable datasets (as of July 2020), of which 41,813 are attributed to the social sciences in general, but only 582 to Business and Management. Only recently have researchers in the innovation community started to make their research data accessible to a broader audience (Marx and Fuegi Citation2020; Reynolds Citation2007; Sorenson et al. Citation2016; Yu et al. Citation2017).
Nevertheless, research data sharing is still rare among management scholars in general, and innovation scholars, in particular although the latter field is enamoured of openness as a cornerstone of academic inquiry but does not yet seem to embrace openness as a part of their own research process and behaviour. Around this notion, we formulate our research question: If and why do innovation management scholars engage in or refrain from open data sharing and what factors increase or reduce the likelihood of data sharing.
To answer this research question, we develop a theoretical model around the factors that hinder or motivate scholars to share their data openly. We generate a set of hypotheses that integrate the resource-based view (RBV) and social exchange theory (SET) to predict data sharing behaviour. We draw on RBV logic to explain why some researchers might refrain from making their datasets publicly available. We set forth that scholars regard their underlying research data as strategic resources that help them to gain a comparative advantage in their academic careers (Barney Citation2001; Wade and Hulland Citation2004). We extend this view by introducing social exchange theory, which helps us to explicitly address the social relations among researchers that facilitate data sharing through the creation of reciprocal relations. We postulate possible positive antecedents to data sharing behaviour that may sway the cost-benefit considerations towards data sharing.
An integration of the RBV and SET is well positioned to explain the perceived trade-of between access to idiosyncratic resources and the costs and benefits of potential reciprocal relations. In fact, prior research in the area of outsourcing and partnerships has benefited from combining these two theoretical perspectives (e.g. Ahmed et al. Citation2018; Chang et al. Citation2015) to explain why some firms share valuable resources and others not.
To test our theoretical model, we explore the perceptions and practices of data sharing in the management sciences with a survey among 173 innovation researchers. In line with the RBV, we find that the stronger scholars perceive that non-public datasets provide them with an advantage over other researchers, the lower their likelihood of data sharing. Using a SET lens, we then show that although innovation researchers are aware of the community benefits arising from open data, they prefer to not disclose their datasets. Only institutional pressure may help to solve this conundrum.
We make several contributions to the literature. Our study is comprehensively built around resource-based theories to explain the individual reluctance to data sharing on the one hand and social exchange theory on the other hand to develop the notion of community wide benefits and incentives for data sharing. We therefore clearly delineate the individual and community wide costs and benefits that come with data sharing.
Prior work on open science and open data has tended to overemphasise and over-generalise the benefits that open data might provide without acknowledging the prevailing scientific paradigm in which these calls are situated in. We explicitly show that because researchers regard their underlying data as a strategic resource, making data publicly available would render their empirical work imitable. Because publications derived from these datasets are paramount for academic careers, the absence of other reward mechanisms makes data sharing less likely.
We also extend prior analyses by conjecturing that social exchange mechanisms (Homans Citation1961; Thibaut and Kelley Citation1959) could sway the balance between the costs and benefits of open data and therefore might help to overcome sharing reluctance. We especially argue that institutional arrangements are necessary to overcome individual career advantages of privately kept data. Because opening up data for others dilutes the value for individual researchers, incentives have to be put in place to overcome the fact that competing researchers can imitate and compete away publication opportunities. We therefore add to the literature the empirical observation that if individual researchers believe that data sharing might be reciprocated by the community, that it can enable replication studies, or that it is encouraged by institutions, then data sharing is more likely to occur.
2. Context: evidence and practices of sharing research data in different disciplines
Researchers across disciplines are restrictive in providing access to their data. Wicherts et al. (Citation2006) received data from only 38 papers (~27% of their requests). These results are not isolated instances but confirmed by other studies (44% for economics articles (Krawczyk and Reuben Citation2012); 25% for pharmaceutical researchers (Kirwan Citation1997); only one article from the British Medical Journal provided data upon request (Reidpath and Allotey Citation2001)). Nevertheless, Tenopir et al. (Citation2015) found an increasing acceptance of and willingness to engage in data sharing, as well as an increase in actual data sharing behaviour. At the same time, the authors also reported an increased perceived risk associated with data sharing and concluded that many barriers to data sharing persist (Tenopir et al. Citation2015). Social science researchers were the most averse to share data (Fecher et al. Citation2017). In summary, many scholars from different scientific disciplines do not engage in open data practices, despite calls for data sharing and policies of funding organisations (in Europe at least) implying that scholars should make the empirical data behind their research publicly accessible (Muscio, Quaglione, and Vallanti Citation2013).
Alas, data sharing in management research brings us into surprisingly uncharted territory. There is sparse theoretical work that highlights factors that may explain data sharing in the management sciences, and there is even less empirical work on the antecedents to data sharing practices in the discipline of innovation management, those who presumably should be most enamoured of openness (Kim and Stanton Citation2016). As Friesike et al. (Citation2015: 581) concluded, ’while academic studies on open innovation are burgeoning, most research on the topic focuses on the later phases of the innovation process. So far, the impact and implications of the general tendency towards more openness in academic and industrial science at the very front-end of the innovation process have been mostly neglected.’
In the following, we pick up this torch and develop a theoretical framework to better understand the potential benefits and caveats that come with open data. Based on this framework, we generate a set of hypotheses that link individual incentives, costs, and trade-offs with the institutional environment in which academic data collection efforts, research, and publishing, are embedded.
3. Theory and hypotheses development
Going back to Merton (Citation1969), there are strong arguments that scholars should have a right to priority for an eventually made discovery so to provide them incentives to engage in the process of risky and uncertain discovery in the first place. In economics and management research, the ‘property’ that drive research often is data. Data, the technology to analyse this data, and the information that materialises from the data represent the key resources on which empirical researchers draw to advance science – and their individual careers (Heckman and Singer Citation2017).
In the following, we draw on RBV and SET perspectives because they effectively help to address the two sets of relationships vital for understanding why researchers share or do not share their data. RBV emphasises the notion that a researcher’s idiosyncratic data and the ability to exploit the data for scientific output are fundamental for scientific discovery and thus also the career success of said researchers. We subsequently introduce an SET perceptive to shed light on the social relations among researchers that could facilitate data sharing through the creation of reciprocal relations. For these social exchange and coordination processes to unfold, involved researchers have to barter the costs and benefits of these repeated interactions.
Although there has been extensive work using the RBV to explain comparative advantages in more general settings (Penrose Citation1955; Wernerfelt Citation1984), we maintain that invoking this perspective can help to explain the factors that hinder researchers from sharing their data (similar to companies not sharing their strategic resources). When it comes to the sharing of knowledge, Bogers (Citation2011a) pointed out that individuals weigh off benefits and costs when considering whether or not they want to openly share intellectual resources. Along these lines, prior work invoking SET argued that individuals are more likely to exchange resources with other individuals, if they get something valuable (or access to something valuable) in return (Cropanzano and Mitchell Citation2005). Although social interactions and exchanges between academics are emphasised in many inquiries, a comprehensive examination of how social exchanges and the expectation of reciprocal behaviour might foster data sharing is lacking. We therefore argue in the development of our hypotheses that the two sets of relations are interdependent and that considerations of reciprocity embedded in SET may affect individual incentives and could sway the balance between the costs and benefits of data sharing towards more data sharing behaviour.
3.1. Data as a strategic resource for academics
Control over specific resources can lead to comparative and competitive advantages (Penrose Citation1955; Wernerfelt Citation1984). Scholars have historically used ‘resources’ as a very broad term to describe inputs converted into products or services through organisational processes (Ricardo Citation1981). Resources include all ‘assets, … information, knowledge’ controlled by a firm (Barney Citation1991:101) that can be used to develop and implement their strategy. In essence, the RBV argues that firms achieve competitive advantages by creating or acquiring bundles of strategic resources that are valuable, rare, inimitable and not substitutable (Barney Citation2001; Wade and Hulland Citation2004). Numerous studies argued that access to and utilisation of data can constitute a strategic resource (Chae, Olson, and Sheu Citation2013; Kuo et al. Citation2013; Piccoli and Ives Citation2005; Wade and Hulland Citation2004). More specifically, Chae, Olson, and Sheu (Citation2013) and Kuo et al. (Citation2013) showed that companies with access to superior data often exhibit competitive advantages.
Beck et al. (Citation2020) discussed that open innovation management theories can not only be applied to research and development in public and private companies, but also to academic research. We argue that academic data constitutes a strategic resource in the sense of RBV theory because it is valuable, rare, difficult to imitate and difficult to substitute (Barney Citation1991). The following paragraphs outline our argument in detail.
First, there exists little doubt that research data are valuable for academics. High quality datasets often allow scholars to address various research questions and thus publish several articles without facing the need to collect data for every single individual publication (Kirkman and Chen Citation2011). With publications being the ‘gold standard’ (Altbach Citation2015: 6) of academic productivity, high-quality datasets give their owner a significant comparative advantage over their fellow researchers with whom they compete for publication spots, job and tenure positions as well as grant funding (Kwiek Citation2015). This is especially important as prior studies have identified path and state-dependencies in research productivity with accumulative patterns of discovery and research status (Merton Citation1969).
Moreover, data collection, curation and preparation often require substantial time and efforts, increasing the value of the data set for its originator. These activities are costly for scholars to conduct, because they reduce the available time and efforts they can spend on other research, teaching or commercialisation as well as on family and recreation (Defazio et al. Citation2020). This adds to researchers’ perceived value of data (McAfee, Mialon, and Mialon Citation2010). At times, the public sharing of data may even further increase the costs associated with it (and in turn increase its perceived value) as the data need to be curated and organised with appropriate metadata so that they could be used by others (Levin and Leonelli Citation2017).
In other words, the higher researchers perceive the direct costs that arise in connection with the data collection, preparation and sharing, the higher is their perceived value of the data. Consequently, we would expect scholars associating high personally borne costs with data collection, preparation and sharing activities to share their data less often:
Hypothesis 1a: The larger researchers perceive the direct personal costs associated with the data to be shared, the lower the likelihood of data sharing.
Second, primary and secondary data, including combinations of one or both types of data from various sources, are quintessentially rare. Especially hand collected datasets or data that originates from unique sources are in high demand. Moreover, data are generally collected and/or combined for a specific research purpose and to answer particular research questions. As such, as long as researchers do not publicly share their data, they remain rare.
Third, research datasets are usually difficult to imitate. Factors like funding resources, contact databases, time and locations play crucial roles especially when collecting social scientific data and make it less likely that another researcher can easily use or re-use uniquely accessed or collected data (Stanton Citation2006). Prior work has also pointed out how measurement procedures might affect the possibility to generate the same results in empirical research (Boyd, Gove, and Hitt Citation2005; Flake and Fried Citation2020). Oftentimes, researchers have a different understanding as to how theoretical concepts should be measured empirically. Releasing research data to the public often requires editing and formatting it in order to ensure that other researchers recognise the data and can work with it; a structure that might be absent in some analyses that ought be shared (King Citation2011). Alas, even the exact same setting and the same respondents do not guarantee the same data (Hursh Citation1984).
Fourth, while data is substitutable per se, the academic incentives for engaging in the costly process of finding and/or gathering an equivalent are low. Clearly, many different methods can answer the same research questions (Dasgupta and David Citation1994). However, preparing, conducting, and analysing experiments or surveys as well as constructing datasets of secondary data require considerable time and effort (Stanton Citation2006). This substantially reduces the publication chances for those gathering similar data, because management journals prefer publishing novel findings (Miller, Taylor, and Bedeian Citation2011). Therefore, while it might be possible for scholars to gather similar data, or analyse data with different methods, this substitute often does not give them the same outcome, making research data sets difficult to substitute.
Sharing gathered data publicly abolishes the rareness and inimitability of data. As researchers compete for a limited number of publication spots (and tenured positions), ‘free riders’ could submit (and hence publish) more papers in shorter periods than the scholars who also engage in data collection. Longo and Drazen (Citation2016) feared that having access to original authors’ data might open the door to ‘research parasites’, i.e. researchers gaining unearned benefits from the data collection efforts of others, which may, at worst, undermine the original publication. The most extreme form is data thievery which occurs when scholars share their data while still writing their paper (Teixeira da Silva and Dobranszki Citation2015). Other researchers take the data and publish an article with the same research question before the scholars who conducted the data collection can publish their article. In turn, the original authors lose out on the publication opportunity, but are still stuck with the direct costs of the data collection (Teixeira da Silva and Dobranszki Citation2015). As a case in point, Walsh and Hong (Citation2003) discussed that scientific competition is the main factor explaining why many scholars do not discuss their ongoing research projects openly anymore.
Fecher et al. (Citation2015: 4) therefore relate to the ’fear of competitive misuse’ that prevents individuals from sharing data. Researchers tend to keep gathered data as their ’trade secrets’ (Pfenninger et al. Citation2017: 212). Existing research highlighted that researchers perceiving their field as particularly competitive were more likely to withhold their data (Haeussler et al. Citation2014). A potential sharing of data may therefore be at odds with the academics’ self-interest, the protection of the main resource for their scientific lead. Thus, academics are ‘incentivised to withhold information as they are in a winner takes-all publishing competition’ (Defazio et al. Citation2020: 5). As data made available to others is more easily imitable, scholars therefore do not share it to prevent imitation. We therefore formulate the following hypothesis:
Hypothesis 1b: The stronger researchers perceive that possessing non-public datasets provide them with an advantage over other researchers, the lower the likelihood of data sharing.
3.2. A social exchange theory perspective on data sharing behaviour
Scholarly communication entails the process of research, discovery, and knowledge dissemination. Data provision and data discovery are part of the socio-technical exchange practice in academia (Gregory et al. Citation2020). As such, the sharing of intellectual resources is a social exchange, an ‘activity, tangible or intangible, and more or less rewarding or costly, between at least two persons (Homans Citation1961:13).’ The reciprocal mechanism underlying social exchanges clearly applies to scholars considering data sharing: Researchers risk losing their competitive advantage by putting their data into the public domain. Through this lens, data sharing (or not sharing) is the pondering about a potentially reciprocal process, where individuals consider an exchange of data with others for individual as well as mutual benefit (Cropanzano and Mitchell Citation2005). Consequently, researchers may only engage in reciprocal relationships if the costs of a relationship outweigh its perceived cost. Data sharing therefore ‘(…) must provide rewards and/or economies in costs which compare favourably with those in other competing relationships or activities available to the two individuals’ (Thibaut and Kelley Citation1959: 49).
3.2.1. Community benefits of data sharing
In academic research, advances in information technology and the nearly worldwide dissemination of English as the language of sciences have fiercely increased competition for publication spots (Di Bitetti and Ferreras Citation2017). To withstand this pressure, more and more scholars have teamed-up to collectively conduct and publish research (Lee and Bozeman Citation2005). Task division and specialisation have enabled those teams to produce more creative and profound articles at higher rates (Ductor Citation2015; Manton and English Citation2007). In this context, publishing research data allows other interested researchers (e.g. those working on similar topics) to receive a better picture of what research is currently underway (Ross and Krumholz Citation2013). This might lead to researchers from different areas with different skills and knowledge offering to join the research team, thus further amplifying its investigations and profoundness (Edwards et al. Citation2011). As a working example, Walport and Brest (Citation2011) argue that open data strongly enhances collaborations between scholars. In some fields, disclosing preliminary data and results is even necessary to find collaborators (Thursby et al. Citation2018).
Open data also reduces the need for individual data collection. Researchers can skip the often costly and resource intensive steps of data collection (Uhlir and Schröder Citation2007). Hence, scholars can publish related studies quicker and therefore spend more time on other discoveries (Fischer and Zigmond Citation2010). The accelerated research process is not only beneficial for scholars, as they can publish more papers in a shorter period, but also for society as problem solving and technology development take less time (Kaye et al. Citation2009).
Evidently, researchers may only engage in reciprocal relationships if the costs of a relationship outweigh its perceived cost. This of course, pertains to both sides of the reciprocal relationship: if data is shared it does not necessarily imply that this data will be used by others. Wallis, Rolando, and Borgman (Citation2013) analysed how often prepared data (that took many weeks to prepare) is sought by scholars. Yet they find that only relatively little data is shared, asked for, and subsequently re-used. They therefore conclude that ‘the effort to make data discoverable is difficult to justify, given the infrequency with which investigators are asked to release their data’ (Wallis, Rolando, and Borgman Citation2013: 14).
Consequently, if researchers only perceive high costs for procuring external data but do not perceive that they are rewarded proportionately for their provided open data, they might consider to not share their data and thus save on time, risk, and effort. Summing up, individuals sharing data, information, or knowledge would expect reciprocal benefits and/or reputational effects based on their sharing (Bogers Citation2011a). Hence, if scholars envision community-wide benefits (increased reputational benefits and/or reciprocating and complimentary research actions by other scholars) for their shared data, this should increase their likelihood of data sharing. We therefore propose:
Hypothesis 2a: The more affirmative researchers are to the community-wide benefits of data sharing, the higher the likelihood of data sharing.
3.2.2. Institutional policies enforcing data sharing
Researchers are embedded into the wider academic community and as such are amenable to institutional logics (Defazio et al. Citation2020). Institutional norms are crucial ways in which a scientific community affects peers and individual academics. These norms may equally attest to appropriate behaviour or sanction inappropriate behaviour, thus, providing guidance (Azoulay, Bonatti, and Krieger Citation2017). While an unbalanced trade-off between incentives and costs lowers the likelihood of data sharing by individual researchers, institutional considerations may be helpful in fostering exchange behaviour.
Many scholars reported that they would like to see the introduction of more data policies and data citation opportunities (Savage and Vickers Citation2009). In fact, more and more journal policies require authors to make their data available to the public (Andreoli-Versbach and Mueller-Langer Citation2014), and there is a rather high prevalence of authors’ statements indicating that data would be available upon request (Piwowar and Chapman Citation2008). Moreover, making data publicly available could create additional rewards. As a case in point, Nature’s author policy encourages the citation of datasets, thus increasing the citation counts of authors who share their datasets (Piwowar, Day, and Fridsma Citation2007). Consequently, institutional mechanisms could affect how scholars perceive the benefits of open data and sway the cost-benefit considerations in favour of data sharing. We therefore suggest:
Hypothesis 2b: The more affirmative researchers are to institutional pressure to increase data sharing, the higher the likelihood of data sharing.
3.2.3. Proliferation of replication studies to enforce data sharing
Various disciplines have been plagued by research scandals and questions about the reproducibility of research findings (e.g. Hopp and Hoover Citation2017, Citation2019). Consequently, some management journals have begun to institutionalise replication studies (Bettis, Helfat, and Shaver Citation2016; Clapp-Smith et al. Citation2017; Celi et al. Citation2019). In the context of these replication studies, data sharing increases the credibility of existing research. It is the only way to enable direct replications, which requires the availability of the primary dataset (Schmidt Citation2009). Data sharing also gives journals the chance to detect erroneous data or analyses prior to publishing (Morey et al. Citation2016). Using data in peer review, for example, can act as the ‘the first line of defence’ (Honig et al. Citation2014, 16) against potential academic misconduct.
As a prime example of how these principles can be applied, researchers at the CERN and the Tevatron at Fermi Labs both undertook a simultaneous search for the Higgs Boson in 2012. Both were involved in replications of each other’s findings using the very same data. Research that has an impact needs to be replicated to avoid misconceptions (Aaltonen et al. Citation2012a, Citation2012b). Initial data from CERN was subsequently repeated and corroborated by other institutions and the consensus among involved scientists was, that only if the same signals were obtained from each institution, evidence in favour of the missing piece in the particle physics standard model would be announced (Reich Citation2012). This eventually led to the Nobel Prize for Francois Englert and Peter Higgs in 2013 (Adam-Bourdarios et al. Citation2015).
In the social sciences, direct replication would had helped in identifying researchers behaving unethically in faking experimental data. Yet the majority of replications conducted in the social sciences are not direct but conceptual replications (Coles et al. Citation2018). While conceptual replications may shed light on the replicability of previously tested relations without access to the original data (Schmidt Citation2009), having this very access allows an opportunity to exactly replicate the original findings as many operationalisations and measurements are made at the author´s discretion.
Consequently, conducting a direct replication may even constitute a good starting point for extensions and follow-up studies (Hopp and Pruschak Citation2020; Meslec et al. Citation2020;). Hence, if scholars believe that replications increase the trustworthiness of existing research and that it ensures the continued credibility and sustainability of their academic field, they should also be more inclined to share data more openly. This leads us to formulate the following hypothesis:
Hypothesis 2 c: The more affirmative researchers are to the proliferation of replication studies, the higher the likelihood of data sharing.
Despite the many benefits that replications may bring about, they could at times also harm the original scholars’ reputations. If data and code become available immediately at or even before publication of the corresponding research article, other researchers can exert scrutiny over the corresponding research making the original analyses refutable. Whereas ‘disagreement likely facilitates the development of new ideas, contributing to creativity and innovation’ (Wang and Noe Citation2010: 124), failed replication attempts using the shared data might defile scholars’ reputation (Barry and Bannister Citation2014). This might result in corrections or even retractions. At worst, it might ruin researchers’ careers because ‘[t]here is a fundamental misconception that retractions are “bad” without pausing to ask why the retraction took place’ (Barbour et al. Citation2017, 1964). In fact, scholars receive 6.9% less citations for publications published after a retraction of one of their prior publications (Lu et al. Citation2013). These considerations may prevent researchers to share their data.
We therefore stipulate that scholars’ fears of revealing flaws and errors through data sharing negatively moderates the positive relationship between the proliferation of replication studies (H2c); the more researchers attest to the unwanted exposure that comes with an increased tendency to replicate empirical research, the less likely will they share their data. The following hypothesis captures this moderation:
Hypothesis 2d: The more affirmative researchers are to the proliferation of replication studies, but the stronger concerns they express over reputational fears, the lower their likelihood of data sharing.
graphically depicts our conceptual model and the corresponding hypotheses.
4. Data and methodology
4.1. Survey distribution
To explore the factors driving and impeding data sharing among (innovation) management scholars, we conducted two empirical surveys using Qualtrics. To identify respondents, we used the participant lists of the World Open Innovation Conferences (WOIC) from 2013 to 2017 and the Druid conferences from 2011 to 2018. We selected those two conferences to capture insights on innovation scholars with academic, but also with practitioners’ backgrounds.
In 2018, we sent emails containing the survey link and a short description of the aim and scope of the research project to the 736 participants of the WOIC conferences between 2013 and 2017. After updating our contact directory from information contained in bounce-back emails that indicated changes of email addresses, we sent out a reminder email two weeks later. Overall, the first survey distribution wave generated 141 replies.
As the data received from this pre-test seemed plausible, we sent the same questionnaire also to the 2,429 participants of the Druid conferences between 2011 and 2018. We only included email addresses that were not already included in the WOIC contact directory. In addition, we prohibited ballot-boxing by allowing each IP-address to only take the survey once. We updated the email addresses based on bounce-backs and sent a reminder email two weeks later. In total, we received 242 responses. The final sample consists of 173 respondents.Footnote1 The numbers of observations vary slightly in the succeeding tables, due to respondents answering ‘N/A’ at times or omitting answers entirely.
The sample drawn is representative of the broad innovation management community. Both conferences did not only attract eminent scholars of innovation management, but also gave junior scholars a chance for participation and presentation. The share of PhD students that replied to the survey (23.12%) nearly mirrors the share of PhD students (26.07% on 18 April 2020) in the Technology and Innovation Management (TIM) division of the Academy of Management.Footnote2
Furthermore, due to the anonymous responses, we cannot identify who participated and who did not participate in the study. Nevertheless, we assessed the potential implications of non-respondent analysis by comparing the characteristics of early respondents (those that replied before we sent the reminder email) to late respondents (those that replied after we sent the reminder email). The largest difference lies within non-European researchers, as they constitute about 20% of the early respondents, but only about 10% of the late respondents. Nevertheless, the actual models in the following section include controls for all characteristics.Footnote3
Table 1. Descriptive statistics and correlation matrix
Table 2. Regression antecedents to data shared
Table 3. Model robustness statistics for data shared based on Young & Holsteen (, Citation2017)
4.2. Variables and methodology
The survey asked for sociodemographic and job-related related information as well as respondents’ experiences with and attitudes towards open data. Furthermore, we asked respondents to indicate their opinions about costs and benefits associated with open data on five-point Likert-scales ranging from ‘strongly disagree’ to ‘strongly agree’. Separate questions focused on respondents’ attitudes towards institutional pressure and replications.
4.2.1. Dependent variable
We conceptualise Data Shared as the dependent variable to capture the public data sharing behaviour of innovation scholars. We derive this variable from respondents’ answers on a scale ranging from 0 to 100 to the statement ‘In my estimation, the following percentage of my data is openly available for everyone’. This extends previous research (Kim and Stanton Citation2016) as prior work used scholars’ intentions to share data as the dependent variable. As the goal of this study is to highlight the state of the art of data sharing among innovation management scholars, we employ the actual amount of shared data. By asking respondents to indicate the percentage of scholars’ shared data we reduce common method bias as we employ two different scales for the dependent and the independent variables (Podsakoff et al. Citation2003). We further aimed to reduce common method bias by implicitly and explicitly assuring respondents that all responses are anonymous. We sent out the same questionnaire link to all respondents individually instead of sending individual links to individual respondents which implicitly ensures anonymity. Also, we included the following statement in the first paragraph of the first page of the questionnaire which explicitly was aimed to ensure anonymity: ‘We are aware that we touch upon a potentially sensitive area and will therefore ensure that all responses and participant information will be anonymized.’
4.2.2. Explanatory variables
We operationalise our hypotheses as follows. We employ scholars’ standardised agreements with several statements anchored on a five-point Likert scale ranging from strongly disagree to strongly agree. For analysing Hypotheses 1a we use a single item statement addressing respondents’ opinions towards the Direct Costs of data sharing. For analysing Hypotheses 1b we use a single item statement on data as Proprietary Source.
For addressing the set of Hypotheses 2a to c, we employ common factor analysis (principal axis factoring option in Stata 15) to generate three reflective latent composite variables, Community Benefits, Institutional Pressure and Replications, out of four statements each. In line with our theoretical reasoning for Hypothesis 2a, Community Benefits composite standardised statements address transparency in research, reducing fraud opportunities and increasing collaboration opportunities. Researchers’ opinions on whether journals should implement policies enforcing data sharing (for review, at publication or after a twelve months grace period) as well as whether publishers should establish licencing policies for the free reuse of data represent the underlying statements for Institutional Pressure (Savage and Vickers Citation2009). Replications capture innovation scholars’ attitudes towards both, direct and conceptual replications as well as on replications of their own work and the work of others (Schmidt Citation2009; Hopp and Hoover Citation2019). We employ a single item statement on the fear of Reputational Costs arising from data sharing as the moderating variable in hypothesis 2d.Footnote4
4.2.3. Control variables
We control for sociodemographic and job-related factors for each respondent. Leahey (Citation2006) showed that gender differences exist in regard to academic productivity. Hence, we include respondents’ gender (Female = 1, 0 otherwise). Furthermore, we control for the location of the university at which respondents work (Europe = 1, 0 otherwise) as cultural habits affect research and publishing processes (Salita Citation2010). We also control for the professional level (Full Professor = 1, 0 otherwise) (Carayol and Matt Citation2006). In addition, we consider the number of peer-reviewed Articles (0 = no articles, 1 = 1 to 5 articles, 2 = 6 to 10 articles, 3 = 10 to 20 articles, 4 = more than 20 articles) and the number of FT-50 Articles (0 = no articles, 1 = 1 to 5 articles, 2 = 6 to 10 articles, 3 = 10 to 20 articles, 4 = more than 20 articles)Footnote5 published from 2013 to 2018. Furthermore, we consider whether respondents acted as reviewer for an FT-50 journal in the year leading up to the survey (Reviewed for FT-50 = 1, 0 otherwise) and whether or not they have held editorships at an FT-50 journal since 2013 (Editor at FT-50 = 1, 0 otherwise). Last, existing literature highlights that sharing qualitative data might in fact be less common than sharing quantitative data (Van Den Berg Citation2005; Aguinis and Solarino, Citation2019). Therefore, we control for respondents’ research approaches (Quantitative = 1, 0 otherwise; Qualitative = 1, 0 otherwise, Theoretical = 1, 0 otherwise).Footnote6
5. Results
5.1. Descriptive Statistics
For the dependent variable, depicts the Data Shared by respondents. We find that scholars in our sample indicate to have shared on average 28.95% of their data (red line) with a standard deviation of 28.67%. This implies that an overwhelming majority of the respondents have shared only a quarter or even less of their data, with 24 respondents having never shared any of their data. The spike in the middle of the histogram indicates 12 respondents who have shared half of their data. Interestingly, five respondents indicate to have shared all their data.Footnote7
5.2. Validity and Reliability
Assessing empirically the validity of Community Benefits, Institutional Pressure and Replications, we find that all composite variables possess an Eigenvalue greater than 1 and therefore fulfil the Kaiser criterion (Kaiser Citation1960). Furthermore, all component loadings are higher than 0.65, indicating the high relevance of the observed individual variables for the composite variables (DeCoster Citation1998). Regarding the reliability of the composite variables, Cronbach’s Alphas corresponds to 0.70 for Community Benefits, 0.83 for Institutional Pressure, and 0.72 for Replications.Footnote8
shows the correlation matrix for all variables. The absolute highest pairwise correlation exists between Institutional Pressure and Community Benefits with a correlation coefficient corresponding to 0.54. As this value is far below the problematic cases of 0.8 to 0.9, our analysis does not seem to suffer from multicollinearity (Mansfield and Helms Citation1982). In addition, all variance inflation factors are smaller than two and hence we do not face any multicollinearity issues (Dormann et al. Citation2010).
We further employ the correlation matrix to check for common method bias by using the K* = 1/r rule (Siemsen, Roth, and Oliveira Citation2010). The smallest bivariate correlation between our explanatory variables and the dependent variables amounts to −0.1465 between Data Shared and Reputational Fears. Hence, we need to include at least 10 independent variables (explanatory and control variables) in the regression to fulfil the K* = 1/r rule. As our full model contains in total 14 explanatory variables, our regression estimates are not very likely to suffer from common method bias. As common-method variance equates to an omitted variable problem, we also address the implications of omitted variables and endogeneity in our limitation section.
5.3. Regression analysis
presents the regression results of the effect of scholars’ opinions on their own data sharing. We report coefficients from negative binominal regressions to account for the over-dispersed count variable distribution of the dependent variable. Model (1) solely contains the sociodemographic and job-related control variables. We find that those who have Reviewed for FT-50 journals share less data, and Quantitative researchers share more data, though both coefficients are only marginally significant at the ten percent level. In Model (2), we find evidence in favour of Hypothesis 1a: Researchers believing that open data carries Direct Costs share significantly less data. As it relates to Hypothesis 1b, the coefficient associated with Proprietary Source is significantly negative in Model (3).
Regarding the Community Benefits, Model (4) shows support for Hypothesis 2a, as the coefficient is significantly positive. Moreover, we find support in favour of Hypothesis 2b, scholars envisioning more Institutional Pressure share more of their data (Model (5)). Model (6) includes the perceived benefits of Replications and finds that this significantly positively relates to data sharing, thus supporting Hypothesis 2 c. Preparing for the suggested moderating hypothesis, we also include the Reputational Fears. Interestingly, the coefficient of Reputational Fears in Model (7) is insignificant.
Model (8) employs the full set of explanatory variables in one full model. We still find support for Hypothesis 1a and 1b. Furthermore, the positive coefficient of Community Benefits turns negative. Institutional Pressure (H2b) still positively relates to data sharing, whereas the Replications coefficient is not significant anymore (H2c).
The final Model (9) adds an interaction term between Replications and Reputational Fears to the full model to assess Hypothesis 2d. Whereas this does not alter the implications for all already included coefficients, the coefficient of the interaction term is significantly negative. Thus, those scholars that are more affirmative of replication studies but who fear the reputational effects that might come with it, are less likely to share data. This supports Hypothesis 2d. We assess the robustness of these estimates in the following.
5.4. Robustness tests
We estimate several model variants of the results reported in . Estimating all models with non-robust standard errors does not affect any significance level. Running standard OLS regressions instead of negative binominal regressions does not qualitatively affect the results. We also re-estimated the results using only the observations where information is available for all variable calculations (123). The results, available in Table A3 in the Online Appendix, do not qualitatively differ from the ones reported in . Not standardising the variables and taking the mean instead of employing principal component analysis to generate the four composite variables does not change any directions and implications.
Nevertheless, given the discrepancy of coefficient estimates across the models in , we conduct a series of robustness checks. Obviously, the changing coefficients signs and levels of significance give substantial discretion to researchers as to which model to pick and which model to report. There exist many degrees of freedom in choosing the models we could report due to the availability of various variable inclusion and exclusion settings. This makes it very difficult to assess the robustness of the findings. To avoid the impression of curated model specifications (reporting only those models that are more reporting significant findings or those that support our hypotheses) we follow the approach suggested in Young and Holsteen (Citation2017) and provide coefficient estimates for all variables using all possible variable combinations. Results are reported in .
First, we show that the robustness ratio (the mean divided by the total standard error) is −2.02 for Direct Costs (H1a) and −2.13 for Proprietary Source (H1b) and t. By language of a standard t-test, this can be regarded as significant (at the 5 percent level). While the coefficient for Direct Costs (H1a) is only (marginally) significant in 71 (86) percent of the models, the coefficient for Proprietary Source (H1b) is significant in 77 percent and marginally significant in 91 percent of the models estimated. Yet both coefficients report the same sign (negative coefficients) in all models estimated. We hence consider Hypotheses 1a and 1b to be robust. We also depict the kernel density estimates of both coefficients in . While there exists some variation in the magnitude of the coefficients, the magnitude never includes zero.
Figure 3. Kernel density for independent variables based on .Young and Holsteen (Citation2017)
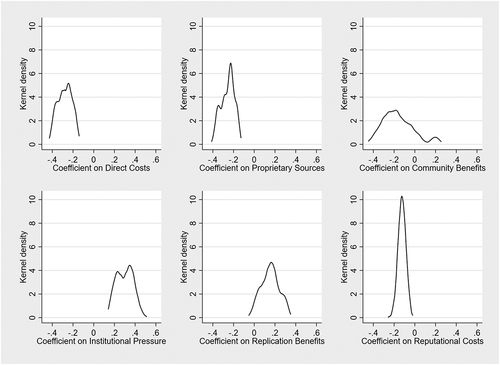
Regarding Community Benefits (H2a), reports that the coefficient is statistically insignificant based on the robustness ratio, yet the sign of the coefficient (negative) is stable in 86 percent of the models estimated but only (marginally) significant in 21 (36) percent of the models. When inspecting the kernel density distribution of the coefficient estimate in , one can infer that there is substantial probability mass to the left of zero. Again, given the small sample size it could be that there simply are not enough observations to accurately estimate the coefficient for community benefits. It would therefore be important for future research to examine this effect using a larger sample size, especially because the coefficient might imply evidence to the contrary of Hypothesis 2a.
For Institutional Pressure (H2b), we find that the robustness ratio (1.99) indicates a robust coefficient estimate. This is corroborated by the high sign stability (positive) and the coefficient is also (marginally) significant in 72 (89) percent of the models estimated. All in all, this provides support for the coefficient reported in and evidence in favour of Hypothesis 2b.
The coefficient for Replications is not significant based on the robustness ratio. Yet again, the high sign stability (97 percent positive) and the low significance rate (only 11 percent) suggest that the failure to find significant effects is most likely related to the small sample size. This is supported by the graphical depiction of the coefficient estimate that shows most of the probability mass being to the right of zero. A larger sample size would probably find more instances where replications show a positive and significant effect.
While, due to complexity and multicollinearity reasons we refrain from including the interaction term in , we can infer that based on the mean and sampling standard errors the coefficient of Reputational Costs would be marginally significant (and negative) (b = −0.12, SE = 0.099). Yet based on the inclusion of the modelling standard error, the overall standard error becomes larger rendering the overall robustness ratio insignificant. Noteworthy, the coefficient estimate for reputational costs is very stable (negative in 100 percent of the models estimated) but only (marginally) significant in 3 (16) percent of the models. The coefficient is corroborated in , where despite fluctuations of the coefficient estimates, the kernel density does not include zero.Footnote9
Summing up, we can fully corroborate the significantly negative effect of Direct Costs (H1a) and Proprietary Source (H1b) and the positive effect of Institutional Pressure (H2b). Moreover, Table A4 in the Online Appendix shows evidence for a moderate negative effect for the subcomponent Transparency of the Community Benefits construct (H2a), which interestingly contradicts our developed hypothesis. While the sign appears robust the coefficient is not significant. This calls for further empirical analysis using a larger sample. Last, the coefficient for Replications (H2c) is very stable in the sign (supporting the hypothesis), yet again fails to be statistically significant. A larger sample size may yet again provide more clarity. For Reputational Costs we can confirm a negative sign for the coefficient, in line with our hypothesis, but due modelling uncertainty we cannot confirm the statistical significance in our (probably too small) sample. As for the moderating effect, the coefficient of the interaction term is significant on the 5% level in and remains significant in the individual robustness assessments. Therefore, we overall find support for Hypothesis 2d.
6. Discussion
The importance of open data for science and society is unquestionable. Yet achieving communal benefits is conditional on how individual researchers perceive the advantages and disadvantages of open data sharing. As it relates to the costs of data sharing, we find that the larger the direct costs, the lower is the likelihood of data sharing among innovation scholars. This extends prior research by emphasising that data collection, curation, and preparation binds critical time and resources, which make the data more valuable to the researcher and consequently establish a hurdle against data sharing. Moreover, we find that those researchers, who believe that their datasets are trade secrets, less often shared their data publicly. This corresponds to existing resource-based theory research and implies that researchers, in fact, view data as a strategic resource. Our research therefore contributes to prior work with the empirical observation that it is important to overcome the potential loss in future publication opportunities to encourage follow-up knowledge re-use through data disclosure.
When it comes to potential ramifications that could ensure more future data sharing, we find evidence in favour of our hypotheses regarding the beneficial effects of institutional pressure. Our data reveals that innovation scholars that positively attest to journal policies for data sharing also made their data publicly accessible and also intend to engage in open data sharing more often. Essentially, this would increase the pressure to release data for everyone and would not single out researchers that need to weigh the costs and benefits individually.
We find mixed evidence regarding the role of replication studies. Although many of our respondents are in favour of exact and conceptual replications, we find mixed evidence that an increased emphasis on replication studies leads to an increased willingness to share data. Given the fragility of our estimates, that could stem from the somewhat small sample studied, we would urge researchers to explore this notion somewhat further. Arguably, the sign stability suggests that future studies may find significant and more robust effects that are in line with our developed hypotheses. As it results from our study, the findings regarding replications are somewhat ironic: when researchers want others to replicate their research, they also need to share their data so that others can conduct the replication.
Along these lines, our findings highlight the non-negligible fact that researchers pay close attention to potential reputational pitfalls associated with data sharing. Here, we extend prior findings by showing that fearing embarrassment and a loss of reputation from flawed code or data prevents researchers from sharing their datasets, even if the same scholars advocate for more replication studies in general.
6.1. Implications
Our analysis confirms a paradoxical tension perceived by scholars in the (innovation) management sciences: While the communal benefits from open data are seen as manifold, the overall costs and risks and the limited prevailing individual benefits of data sharing demotivate individual researchers to open their data. Evidently, the root causes for not sharing data lie in the academic incentive system. Considering data from both a resource-based perspective and a social exchange perspective provides a comprehensive explanation for the reluctance to share data, even upon and after publication. Researchers think of research data as their private strategic resource, for which exclusive possession provides a competitive advantage in the academic system and for which the expected data sharing benefits do simply not warrant the relinquishment of their proprietary resource.
This situation is unlikely to be overcome by an individual researcher alone, despite good intentions and knowledge of the public benefits for the scientific system of data sharing. Our research has revealed that even scholars in the innovation management discipline, whose core theories and academic discourses promote an open approach to science and innovation (Bogers et al. Citation2017; von Hippel Citation2017; West et al. Citation2014), do not share data openly. Alas, researchers are, after all, amenable to weighing of costs and incentives.
Bogers (Citation2011b:110) concludes, ‘the use of a knowledge exchange strategy in general and licensing in particular is the way in which firms shape the dimensions in the tension field to balance the sharing and protection of knowledge.’ We therefore envision two different approaches to overcome this open innovation paradox in academia (Bogers Citation2011b; Beck et al. Citation2020): (i) on the institutional level, changing the incentive system for researchers to stimulate data sharing; and (ii) on the individual level, educating researchers in practices of ‘strategic openness’ (Alexy et al. Citation2018, 1704), which allows them to freely reveal their data and still profit from this behaviour individually, even under unchanged incentive regimes on the institutional level. We will elaborate on both directions in more detail in the following.
On the institutional level, we need to amend the academic incentive system. To increase researchers’ willingness to share their data, we echo Wilbanks and Friend (Citation2016) who call for an academic performance measure system that does not only reward the publication of an article, but also the publication of its data. Researchers are receptive to publication incentives, with all its’ shortcomings. As such, they should react favourably to open data incentives. Realising those requires several concrete measures:
First, datasets need to be ‘publishable and citable’ (Reichman, Jones, and Schildhauer Citation2011: 704) so that we can include them in scholars’ publication and citation counts. This demands a clear standard and syntax for the references of datasets. Publishers (such as Wiley or Elsevier) and open source repositories such as DataVerse already provide researchers with the opportunity to publish a dataset with a unique digital object identifier (DOI). A general approach in this area are the FAIR principles, a multinational initiative to provide guidelines for the publication of research datasets or code in a manner that makes them ‘Findable, Accessible, Interoperable, and Reusable (FAIR)’ (Wilkinson et al. Citation2016). On the national level, currently large-scale schemes are taking place to build (national) research data infrastructures (Mons et al. Citation2017). In the management sciences, such initiatives, however, are largely unknown or, at least, not high on the awareness level compared to the life or natural sciences. Professional education and awareness building for these initiatives, for example by professional organisations like PDMA or ISPIM for the field of innovation management, or AACSB and AOM for management research in general, would be an important element to foster open data sharing by management scholars.
Second, obviously sufficient data needs to be supplied into these infrastructures. For this, data sharing by scholars has to become visible. Therefore, data needs to be searchable in the same literature databases used to find research results (papers). This would allow the users of academic impact measures (like recruitment or tenure committees, academic associations, or grant-giving institutions) to recognise not only how often a researcher has published in prestigious journals, but also whether her or his results have been confirmed and replicated by others or not. In addition to incentivising researchers (who are, as our data indicates, by and large open to replication studies) to share data more openly when they themselves receive credit for replicated studies, the central outcome would be the overall benefit from replication studies for the scientific process. For the individual researcher, a system that honours high citation counts of revealed data sets would foster good ‘citizen behaviour’ of scholars, making their service of data sharing to the academic community measurable.
Third, there are also short-term measures than can be implemented immediately by editors of management journals. Kidwell et al. (Citation2016) showed that low-cost nudging can already increase the level of data sharing. The journal Psychological Science, for example, introduced badges that visually signalled that data and material was available for interested readers of said articles. Data sharing increased from less than three percent to almost 40 percent within a two-year timespan (Kidwell et al. Citation2016).
Fourth, university and research institutions should support researchers in the data curation and publication process. As a case in point, some university library scholars believe that curation, preparation, and publication of data should be taken over by university librarians who could focus on this task (Koltay Citation2019), especially as their actual jobs of collecting books, etc. get reduced substantially.
All these measures, however, demand that other academics make use of the published data sets. After all, Peters et al. (Citation2016) showed that about 85% of the citable available datasets remain uncited. While one can argue that this ratio is not worse than the citation records of many journal articles (Judge et al. Citation2007), we consider building more demand for open data as a core measure. This is a fruitful area for future research, investigating the adoption and usage drivers (and barriers) of freely revealed research data.
In addition, there are also several measures related to the individual level that could help to foster data sharing, addressing a proactive strategic behaviour of scholars beyond their reaction to institutional incentives. Obviously, intellectual property rights in academic research are very difficult to enforce and accordingly, other mechanisms might come into play to create incentives for data sharing. Mechanisms that enforce and govern the reuse of knowledge (and ideas) are hardly contractible and rewards for intermediate disclosure (before a researcher has conclude his research agenda with a given dataset) reduce incentives and limits the researchers’ ability to control the reuse of his data collected. Evidently there is a trade-off between providing incentives for researchers to make costly investments into the research effort, while at the same time encouraging follow-up knowledge re-use (through disclosure, among others). While intellectual property rights to data might increase the incentives for researchers to engage in data collection, they may in fact deter re-use.
Here, transferring the concept of strategic openness to the context of open data could provide a fresh perspective. Alexy et al. (Citation2018) proposed strategic openness in the context of open innovation, suggesting that organisations should voluntarily forfeit the control over strategically relevant resources instead of securing them through IP protection. While such a behaviour intuitively would hurt the organisation, Alexy et al. (Citation2018) showed that companies can still maximise profitability, if, for example, they open parts of their resource base or use openness to find/create complementary services. The concept of strategic openness can be transferred to researchers considering sharing their data.
Research data are just part of a larger bundle of (research) resources, like data acquisition instruments, code for their analysis, data storage and management, or reporting tools helping to navigate the data. Hence, researchers openly sharing their data (i.e. giving away their strategic resource according to the RBV) could actually increase the value of the entire bundle of research resources connected with this data, if they only offered controlled access to the other resources. For example, to be able to fully understand how researchers arrived at prior conclusions, access to data coding and analysis files as well are likely necessary (Hopp et al. Citation2018).
Researchers further can derive competitive advantage from open data if the data has idiosyncratic features, that is, if the original researcher has superior information about and/or superior complementarities with the open resource a priori: The open data source may be available to everyone, but because the original researcher created it prior to release, he or she should hold superior information about what can be done with the data and superior complementarities on how to leverage it with other proprietary resources. Consequently, the original researcher can publish much faster than anyone trying to free ride on the data collection. This would also be of theoretical promise, as one may expand the theoretical view taken in this paper by studying data-sharing from a resource-dependency perspective to better understand how researchers team up and how they can combine their capabilities to achieve better research and publication outcomes (Pfeffer and Salancik Citation1978).
Concluding, our previous arguments indicate that researchers can be both ‘pulled’ into openly sharing their data by institutional actors (like journals or academic societies) setting new incentives motivating this behaviour and actively ‘push’ data sharing by developing strategic openness as their own strategy to strive in the academic system. We see many opportunities for further research in studying these approaches in more details, either in experimental studies or by observing behaviour of researchers in the field.
6.2. Limitations and future research
No study is perfect, and ours is no exception. We contacted 2,716 innovation researchers with valid contact details. This number is only a bit smaller than the 3,468 active members of the Technology and Innovation Management (TIM) division of the Academy of Management listed on its website as of September 2019. Considering that our final sample consists of 173 respondents, the approximate response rate is 6.40%. This compares to other recently conducted online surveys among scholars investigating research practices and academic misconduct (Hopp and Hoover Citation2017; Liao et al. Citation2018). As those innovation scholars highly interested in open science might more often be intrinsically motivated to take their time and efforts to complete the questionnaire, our results might suffer from sample selection bias. However, those innovation scholars highly interested in open science are also those who might more often engage in open practices like data sharing. Hence, our results at least provide an upper boundary for the level of data sharing among all innovation scholars.
This article only provides insights into the topic of open data eliciting opinions of innovation scholars. While this certainly limits the generalisability of the findings and makes them context-specific, it is important to bear in mind that this community explicitly studies the merits of openness. It therefore stands to reason that other fields of management research might emphasise even more pronounced costs of data sharing and place a lower emphasis on the benefits that it might provide. As such, a follow-up study could extend the research question beyond innovation scholars. Hereby, the focus could lie on increasing the sample by investigating differences in data sharing between various management fields or even between various social science disciplines. Also, an increase in sample size would help to resolve some ambiguity about coefficient estimates, sign stability, and significance levels reported. Moreover, a follow-up questionnaire could also include qualitative questions to elicit the reasonings and backgrounds of certain interesting responses like those scholars who shared all their datasets in-depth.
Our research only elicited personal viewpoints, and individuals may certainly state that they share research data when in fact they never do so in real-life. A substantial number of innovation scholars indicate that they share their research data upon request. However, as noted in the literature review, existing empirical research points out that many scholars do not provide data upon request even if they included such data sharing statements in their articles (Wicherts et al. Citation2006; Krawczyk and Reuben Citation2012; Reidpath and Allotey Citation2001; Savage and Vickers Citation2009). Evidently, this may introduce a common-method variance bias, an omitted variable problem that potential invites the risk of endogeneity. Future studies might therefore rely on instrumental variable techniques to recover true parameter estimates.
Last, our study revealed that qualitative researchers struggle more with making their data public (Pratt, Kaplan, and Whittington Citation2020). Especially in light of the growing interest in replicating qualitative research, this seems to be a promising area for further research (e.g. Aguinis and Solarino, Citation2019; King Citation1995). It would be very interesting to see whether new technologies and processes exist that could help researchers to share qualitative data – and assist others in using this data (Antes et al. Citation2018).
7. Conclusion
Our research was sparked by the observations that, despite the proclaimed benefits of openness, many researchers do not openly share the research data behind their articles. Consequently, we analysed this conundrum and elicited innovation scholars’ attitudes towards open data and assessed their data sharing behaviours building on resource based-theory and social exchange theory. Our findings indicate that most scholars would be open to share their data upon request. Yet data sharing is generally not very prevalent. Despite the generally acclaimed societal benefits, researchers refrain from putting their data into the public space. We identify antecedents and more importantly, inhibitors to data sharing behaviour that could potentially provide policy implications. First, researcher behaviour is susceptible to potential costs and threats that open data might provide. Essentially, when considering the ‘social dilemma’ of open data (Linek et al. Citation2017: 1), the identified personal incentives to open data sharing might not outweigh the burden open data places on individual researchers. In summary, if open data sharing is to catch up, the burden for data preparation cannot be put on the individual researcher. Rather, institutional mechanisms on the level of the academic community need to be put into place: That could either be incentives that give more credit to data sharing, or journal policies that make data sharing a mandatory requirement for all publication. Yet even with increased incentives the effort for data preparation rests upon the individual researcher. As an institutional remedy one might, for example, consider more administrative help for individual researchers.
Acknowledgments
We would like to thank all survey participants for their time and efforts. Furthermore, we would like to acknowledge Oliver Fabel and Rudolf Vetschera for their valuable comments. We are grateful to three anonymous reviewers who provided excellent suggestions on improving the paper. Last, we would like to thank the participants of the 16th International Open and User Innovation Conference in New York and the 1st Open Innovation in Science (OIS) Research Workshop in Vienna for their helpful feedback and precious inputs.
Data Availability Statement
The authors confirm that the data supporting the findings of this study are available within its supplementary materials.
Disclosure statement
No potential conflict of interest was reported by the author(s).
Correction Statement
This article has been republished with minor changes. These changes do not impact the academic content of the article.
Notes
1 We exclude 27 respondents due to them indicating their occupation as ‘member or founder of a company (“industry”)’ or ‘Other’. Furthermore, 42 respondents who did not answer all sociodemographic and job-related questions located at the first page of the questionnaire as well as those that neither conducted qualitative nor quantitative data were pruned from the sample.
2 The share of scientists with industry affiliations is higher for WOIC participants. This derives from the mere fact that WOIC focuses more on practitioners’ work and problems than Druid. The only large other differences in characteristics occur for qualitative researchers. While about half of the WOIC participants conduct qualitative research, only about one-third of the Druid participants conduct qualitative research. The remainder of the characteristics (e.g. gender, reviewers for FT-50, …) do not differ significantly between the two groups.
3 We also analysed models including dummies for WOIC/DRUID participation and early/late respondents. The implications of these models do not differ from the implications arising from . Full results of the WOIC vs. Druid analyses and the early vs. late respondents analyses are available from the authors upon requests.
4 The items used to measure each single-time variable and all constructs as well as reliability and validity statistics are provided in Table XX in the Online Appendix.
5 To help respondents identify which journals are FT-50 journals, the survey included a link to the website of the Financial Times listing the FT-50 journals as of the 12th of September 2016.
6 The variable coding is not exclusive. For example, scholars can engage in quantitative and theoretical research (Quantitative = 1; Qualitative = 0; Theoretical = 1).
7 The descriptive statistics for all control variables and independent variables are available in . More detailed statistics on the control and independent variables are available in Table A1 and Table A2 in the Online Appendix.
8 As our sample consists of innovation scholars only, and as the observed variables only contain about 150 observations, Communal Benefits and Individual Costs and Risk just exceed the ubiquitous thresholds for Cronbach’s alpha of 0.7. Bernardi (Citation1994) empirically showed that small homogenous samples often tend to have lower Cronbach’s alphas. Following Nunnally and Bernstein (Citation1994), we conclude to have reliable variables.
9 We corroborate our analysis by demonstrating the robustness of the single factors of our latent composite variables, namely Community Benefits, Institutional Pressure, and Replications in Table A4 in the Appendix. For Community Benefits we conclude that the effects of two main driving factors, Transparency and Collaboration, are working in opposite directions (Transparency: stable negative; Collaboration: stable positive) The model robustness for Institutional Pressure indicates that three out of four factors support our findings for the composite variable (Data at Publication, Data on Submission, Data Licencing Options are all stable positive, though only Data on Submission is robustly significant). One variable, Data 12 M after Publication, remains insignificant. Just as in the main analysis, results for Replications remain insignificant. Noteworthy the component Conceptual Replications (Own) is positive and stable in all models, yet only significant in 26 percent of the models. There is weak indication that scholars sharing more data would appreciate being subject to conceptual replication, which calls for replications of the effect in larger samples.
References
- Aaltonen, T., V. M. Abazov, B. Abbott, B. S. Acharya, M. Adams, T. Adams, et al. 2012b. “Evidence for a Particle Produced in Association with Weak Bosons and Decaying to a Bottom-Antibottom Quark Pair in Higgs Boson Searches at the Tevatron.” Physical Review Letters 109 (7): 071804.
- Aaltonen, T., B. A. González, S. Amerio, D. Amidei, A. Anastassov, A. Annovi, J. Antos, et al. 2012a. “Search for the Standard Model Higgs Boson Decaying to Abb¯ Pair in Events with Two Oppositely Charged Leptons Using the Full CDF Data Set.” Physical Review Letters 109 (11): 111803. doi:https://doi.org/10.1103/PhysRevLett.109.111803.
- Adam-Bourdarios, C., G. Cowan, C. Germain-Renaud, I. Guyon, B. Kégl, and D. Roussea. 2015. “The Higgs Machine Learning Challenge.” Journal of Physics: Conference Series 664 (7): 072015.
- Aguinis, H., and A. M. Solarino. 2019. “Transparency and Replicability in Qualitative Research: The Case of Interview with Elite Informants.” Strategic Management Journal 40 (8): 1291–1315.
- Ahmed, A., F. M. Khuwaja, N. A. Brohi, and I. B. L. Othman. 2018. “Organizational Factors and Organizational Performance: A Resource-Based View and Social Exchange Theory Viewpoint.” The International Journal of Academic Research in Business and Social Sciences 8 (3): 594–614. doi:https://doi.org/10.6007/IJARBSS/v8-i3/3951.
- Alexy, O., J. West, H. Klapper, and M. Reitzig. 2018. “Surrendering Control to Gain Advantage: Reconciling Openness and the Resource-Based View of the Firm.” Strategic Management Journal 39 (6): 1704–1727. doi:https://doi.org/10.1002/smj.2706.
- Altbach, P. G. 2015. “What Counts for Academic Productivity in Research Universities?” International Higher Education 79 (4): 6–7. doi:https://doi.org/10.6017/ihe.2015.79.5837.
- Andreoli-Versbach, P., and F. Mueller-Langer. 2014. “Open Access to Data: An Ideal Professed but Not Practised.” Research Policy 43 (9): 1621–1633. doi:https://doi.org/10.1016/j.respol.2014.04.008.
- Antes, A. L., H. A. Walsh, M. Strait, C. R. Hudson-Vitale, and J. M. DuBois. 2018. “Examining Data Repository Guidelines for Qualitative Data Sharing.” Journal of Empirical Research on Human Research Ethics 13 (1): 61–73. doi:https://doi.org/10.1177/1556264617744121.
- Azoulay, P. J., A. Bonatti, and J. L. Krieger. 2017. “The Career Effects of Scandal: Evidence from Scientific Retractions.” Research Policy 46 (9): 1552–1569. doi:https://doi.org/10.1016/j.respol.2017.07.003.
- Barbour, V., T. Bloom, J. Lin, and E. Moylan. 2017. “Amending Published Articles: Time to Rethink Retractions and Corrections?” F1000Research 6 (1): 1960. doi:https://doi.org/10.12688/f1000research.13060.1.
- Barney, J. 1991. “Firm Resources and Sustained Competitive Advantage.” Journal of Management 17 (1): 99–120. doi:https://doi.org/10.1177/014920639101700108.
- Barney, J. 2001. “Resource-Based Theories of Competitive Advantage: A Ten-Year Retrospective on the Resource-Based View.” Journal of Management 27 (6): 643–650. doi:https://doi.org/10.1177/014920630102700602.
- Barry, E., and F. Bannister. 2014. “Barriers to Open Data Release: A View from the Top.” Information Polity 19 (12): 129–152. doi:https://doi.org/10.3233/IP-140327.
- Beck, S., C. Bergenholtz, M. Bogers, T. M. Brasseur, L. M. Conradsen, D. Di Marco, A. P. Distel, et al. 2020. “The Open Innovation in Science Research Field: A Collaborative Conceptualisation Approach.” Industry and Innovation 1–50. Published Online First 4th of August, 2020. doi:https://doi.org/10.1080/13662716.2020.1792274.
- Bernardi, R. A. 1994. “Validating Research Results When Cronbach’s Alpha Is below .70: A Methodological Procedure.” Educational and Psychological Measurement 54 (3): 766–775. doi:https://doi.org/10.1177/0013164494054003023.
- Bettis, R. A., C. E. Helfat, and J. M. Shaver. 2016. “The Necessity, Logic, and Forms of Replication.” Strategic Management Journal 37 (11): 2193–2203. doi:https://doi.org/10.1002/smj.2580.
- Bogers, M. 2011a. “Knowledge Sharing in Open Innovation: An Overview of Theoretical Perspectives on Collaborative Innovation.” In Open Innovation in Firms and Public Administrations, edited by C. de Pablos Heredero and D. L. Berzosa, 1–14. Hershey: Information Science Reference.
- Bogers, M. 2011b. “The Open Innovation Paradox: Knowledge Sharing and Protection in R&D Collaborations.” European Journal of Innovation Management 14 (1): 93–117. doi:https://doi.org/10.1108/14601061111104715.
- Bogers, M., A. K. Zobel, A. Afuah, E. Almirall, S. Brunswicker, L. Dahlander, L. Frederiksen, et al. 2017. “The Open Innovation Research Landscape: Established Perspectives and Emerging Themes across Different Levels of Analysis.” Industry and Innovation 24 (1): 8–40. doi:https://doi.org/10.1080/13662716.2016.1240068.
- Boyd, B. K., S. Gove, and M. A. Hitt. 2005. “Construct Measurement in Strategic Management Research: Illusion or Reality?” Strategic Management Journal 26 (3): 239–257. doi:https://doi.org/10.1002/smj.444.
- Carayol, N., and M. Matt. 2006. “Individual and Collective Determinants of Academic Scientists’ Productivity.” Information Economics and Policy 18 (1): 55–72. doi:https://doi.org/10.1016/j.infoecopol.2005.09.002.
- Celi, L. A., L. Citi, M. Ghassemi, T. J. Pollard, and L. A. Mueck. 2019. “The PLoS ONE Collection on Machine Learning in Health and Biomedicine: Towards Open Code and Open Data.” PloS ONE 14 (1): e0210232. doi:https://doi.org/10.1371/journal.pone.0210232.
- Chae, B. K., D. Olson, and C. Sheu. 2013. “The Impact of Supply Chain Analytics on Operational Performance: A Resource-Based View.” International Journal of Production Research 52 (16): 4695–4710. doi:https://doi.org/10.1080/00207543.2013.861616.
- Chang, H. H., Y. C. Tsai, S. H. Chen, G. H. Huang, and Y. H. Tseng. 2015. “Building Long-Term Partnerships by Certificate Implementation: A Social Exchange Theory Perspective.” Journal of Business & Industrial Marketing 30 (7): 867–879. doi:https://doi.org/10.1108/JBIM-08-2013-0190.
- Chesbrough, H. 2003. Open Innovation: The New Imperative for Creating and Profiting from Technology. Cambridge, MA: Harvard Business School Publishing.
- Clapp-Smith, R., M. Carsten, J. Gooty, S. Connelly, A. Haslam, N. Bastardoz, and S. Spain. 2018. “Special Registered Report Issue on Replication and Rigorous Retesting of Leadership Models.” The Leadership Quarterly 29: IIIIV.
- Coles, N., L. Tiokhin, A. Scheel, P. Isager, and D. Lakens. 2018. “The Costs and Benefits of Replication Studies.” Behavioral and Brain Sciences 41 (1): e124. doi:https://doi.org/10.1017/S0140525X18000596.
- Cropanzano, R., and M. S. Mitchell. 2005. “Social Exchange Theory: An Interdisciplinary Review.” Journal of Management 31 (6): 874–900. doi:https://doi.org/10.1177/0149206305279602.
- Dasgupta, P., and P. A. David. 1994. “Toward a New Economics of Science.” Research Policy 23 (5): 487–521. doi:https://doi.org/10.1016/0048-7333(94)01002-1.
- DeCoster, J. 1998. “Overview of Factor Analysis.” Stat-Help. Accessed 26 September 2019. http://www.stat-help.com/factor.pdf
- Defazio, D., C. Kolympiris, A. Salter, and M. Perkman. 2020. “Busy Academics Share Less: The Impact of Professional and Family Roles on Academic Withholding Behaviour.” Studies in Higher Education 1–20. Published Online First 17th of July, 2020. doi:https://doi.org/10.1080/03075079.2020.1793931.
- Di Bitetti, M. S., and J. A. Ferreras. 2017. “Publish (In English) or Perish: The Effect on Citation Rate of Using Languages Other than English in Scientific Publications.” Ambio 46 (1): 121–127. doi:https://doi.org/10.1007/s13280-016-0820-7.
- Dormann, C. F., J. Elith, S. Bacher, C. Buchmann, G. Carl, G. Carre, J. R. G. Marquéz, et al. 2010. “Collinearity: A Review of Methods to Deal with It and A Simulation Study Evaluating Their Performance.” Ecography 36 (1): 27–46. doi:https://doi.org/10.1111/j.1600-0587.2012.07348.x.
- Ductor, L. 2015. “Does Co-Authorship Lead to Higher Academic Productivity?” Oxford Bulletin of Economics and Statistics 77 (3): 385–407. doi:https://doi.org/10.1111/obes.12070.
- Edwards, P. N., M. S. Mayernik, A. L. Batcheller, G. C. Bowker, and C. L. Borgman. 2011. “Science Friction: Data, Metadata, and Collaboration.” Social Studies of Science 41 (5): 667–690. doi:https://doi.org/10.1177/0306312711413314.
- Fecher, B., S. Firesike, M. Hebing, S. Linek, and A. Sauermann. 2015. “A Reputation Economy: Results from an Empirical Survey on Academic Data Sharing.” DIW Berlin Discussion Paper No. 1454.
- Fecher, B., S. Friesike, M. Hebing, and S. Linek. 2017. “A Reputation Economy: How Individual Reward Considerations Trump Systemic Arguments for Open Access to Data.” Palgrave Communications 3 (17051): 1–10. doi:https://doi.org/10.1057/palcomms.2017.51.
- Fischer, B. A., and M. J. Zigmond. 2010. “The Essential Nature of Sharing in Science.” Science and Engineering Ethics 16 (4): 783–799. doi:https://doi.org/10.1007/s11948-010-9239-x.
- Flake, J. K., and E. I. Fried. 2020. “Measurement Schmeasurement: Questionable Measurement Practices and How to Avoid Them.” Advances in Methods and Practices in Psychological Science 3 (4): 456–465. doi:https://doi.org/10.1177/2515245920952393.
- Friesike, S., B. Widenmayer, O. Gassmann, and T. Schildhauer. 2015. “Opening Science: Towards an Agenda of Open Science in Academia and Industry.” The Journal of Technology Transfer 40 (4): 581–601. doi:https://doi.org/10.1007/s10961-014-9375-6.
- Gregory, K. M., H. Cousijn, P. Groth, A. Scharnhorst, and S. Wyatt. 2020. “Understanding Data Search as a Socio-Technical Practice.” Journal of Information Science 46 (4): 459–475. doi:https://doi.org/10.1177/0165551519837182.
- Haeussler, C., L. Jiang, J. Thursby, and M. Thursby. 2014. “Specific and General Information Sharing among Competing Academic Researchers.” Research Policy 43 (3): 465–475. doi:https://doi.org/10.1016/j.respol.2013.08.017.
- Heckman, J. J., and B. Singer. 2017. “Abducting Economics.” American Economic Review 107 (5): 298–302. doi:https://doi.org/10.1257/aer.p20171118.
- Homans, G. C. 1961. Social Behavior: Its Elementary Forms. New York: Hardcourt Brace.
- Honig, B., J. Lampel, D. Siegel, and P. Drnevich. 2014. “Ethics in the Production and Dissemination of Management Research: Institutional Failure or Individual Fallibility?” Journal of Management Studies 51 (1): 118–142. doi:https://doi.org/10.1111/joms.12056.
- Hopp, C., F. J. Greene, B. Honig, T. Karlsson, and M. Samuelsson. 2018. “Revisiting the Influence of Institutional Forces on the Written Business Plan: A Replication Study.” Management Review Quarterly 68 (4): 361–398. doi:https://doi.org/10.1007/s11301-018-0143-9.
- Hopp, C., and G. A. Hoover. 2017. “How Prevalent Is Academic Misconduct in Management Research?” Journal of Business Research 80 (3): 73–81. doi:https://doi.org/10.1016/j.jbusres.2017.07.003.
- Hopp, C., and G. A. Hoover. 2019. “What Crisis? Management Researchers’ Experiences with and Views of Scholarly Misconduct.” Science and Engineering Ethics 25 (5): 1549–1588. doi:https://doi.org/10.1007/s11948-018-0079-4.
- Hopp, C., and G. Pruschak. 2020. “Is There Such A Thing as Leadership Skill? A Replication and Extension of the Relationship between High School Leadership Positions and Later-Life Earnings.” The Leadership Quarterly 101475. Published Online First 22nd of October, 2020. doi:https://doi.org/10.1016/j.leaqua.2020.101475.
- Hursh, S. R. 1984. “Behavioral Economics.” Journal of the Experimental Analysis of Behavior 42 (3): 435–452. doi:https://doi.org/10.1901/jeab.1984.42-435.
- Judge, T. A., D. M. Cable, A. E. Colbert, and S. L. Rynes. 2007. “What Causes a Management Article to Be Cited?” Academy of Management Journal 50 (3): 491–506. doi:https://doi.org/10.5465/amj.2007.25525577.
- Kaiser, H. F. 1960. “The Application of Electronic Computers to Factor Analysis.” Educational and Psychological Measurement 20 (1): 141–151. doi:https://doi.org/10.1177/001316446002000116.
- Kaye, J., C. Heeney, N. Hawkins, J. De Vries, and P. Boddington. 2009. “Data Sharing in Genomics – Re-shaping Scientific Practice.” Nature Reviews Genetics 10 (5): 331–335. doi:https://doi.org/10.1038/nrg2573.
- Kidwell, M. C., L. B. Lazarevic, E. Baranski, T. E. Hardwicke, S. Piechowski, L. S. Falkenberg, C. Kennett, et al. 2016. “Badges to Acknowledge Open Practices: A Simple, Low-Cost Effective Method for Increasing Transparency.” PLoS Biology 14 (5): e1002456. doi:https://doi.org/10.1371/journal.pbio.1002456.
- Kim, Y., and J. M. Stanton. 2016. “Institutional and Individual Factors Affecting Scientists’ Data‐Sharing Behaviors: A Multilevel Analysis.” Journal of the Association for Information Science and Technology 67 (4): 776–799. doi:https://doi.org/10.1002/asi.23424.
- King, G. 1995. “Replication, Replication.” Political Science and Politics 28 (3): 444–452. doi:https://doi.org/10.1017/S1049096500057607.
- King, G. 2011. “Ensuring the Data-Rich Future of the Social Sciences.” Science 331 (6018): 719–721. doi:https://doi.org/10.1126/science.1197872.
- Kirkman, B. L., and G. Chen. 2011. “Maximizing Your Data or Data Slicing? Recommendations for Managing Multiple Submissions from the Same Dataset.” Management and Organization Review 7 (3): 433–446. doi:https://doi.org/10.1111/j.1740-8784.2011.00228.x.
- Kirwan, J. R. 1997. “Making Original Data from Clinical Studies Available for Alternative Analysis.” The Journal of Rheumatology 24 (5): 625–822.
- Koltay, T. 2019. “Accepted and Emerging Roles of Academic Libraries in Supporting Research 2.0.” The Journal of Academic Librarianship 45 (2): 75–80. doi:https://doi.org/10.1016/j.acalib.2019.01.001.
- Krawczyk, M., and E. Reuben. 2012. “(Un)available upon Request: Field Experiment on Researchers’ Willingness to Share Supplementary Materials.” Accountability in Research 19 (3): 175–186. doi:https://doi.org/10.1080/08989621.2012.678688.
- Kuo, T. C., C. W. Hsu, S. H. Huang, and D. C. Gong. 2013. “Data Sharing: A Collaborative Model for A Gren Textile/Clothing Supply Chain.” International Journal of Computer Integrated Manufacturing 27 (3): 266–280. doi:https://doi.org/10.1080/0951192X.2013.814157.
- Kwiek, M. 2015. “Academic Generations and Academic Work: Patterns of Attitudes, Behaviors, and Research Productivity of Polish Academics after 1989.” Studies in Higher Education 40 (8): 1354–1376. doi:https://doi.org/10.1080/03075079.2015.1060706.
- Leahey, E. 2006. “Gender Differences in Productivity: Research Specializations as a Missing Link.” Gender & Society 20 (6): 754–780. doi:https://doi.org/10.1177/0891243206293030.
- Lee, M., J. J. Yun, A. Pyka, D. Won, F. Kodama, G. Schiuma, H. Park, et al. 2018. “How to Respond to the Fourth Industrial Revolution, or the Second Information Technology Revolution? Dynamic New Combinations between Technology, Market, and Society through Open Innovation.” Journal of Open Innovation: Technology, Market, and Complexity 4 (3): 21. doi:https://doi.org/10.3390/joitmc4030021.
- Lee, S., and B. Bozeman. 2005. “The Impact of Research Collaboration on Scientific Productivity.” Social Studies of Science 35 (5): 673–702. doi:https://doi.org/10.1177/0306312705052359.
- Levin, N., and S. Leonelli. 2017. “How Does One Open Science? Questions of Value in Biological Research.” Science, Technology, & Human Values 42 (2): 280–305. doi:https://doi.org/10.1177/0162243916672071.
- Liao, Q., Y. Zhang, Y. Fan, M. Zheng, Y. Bai, G. D. Eslick, X. He, S. Zhang, H. H. Xia, and H. He. 2018. “Perceptions of Chinese Biomedical Researchers Towards Academic Misconduct: A Comparison between 2015 and 2010.” Science and Engineering Ethics 24 (2): 629–645.
- Linek, S. B., B. Fecher, S. Friesike, and M. Hebing. 2017. “Data Sharing as Social Dilemma: Influence of the Researcher’s Personality.” PLoS ONE 12 (8): e0183216. doi:https://doi.org/10.1371/journal.pone.0183216.
- Longo, D. L., and J. M. Drazen. 2016. “Data Sharing.” The New England Journal of Medicine 374 (3): 276–277. doi:https://doi.org/10.1056/NEJMe1516564.
- Lu, S. F., G. Z. Jin, B. Uzzi, and B. Jones. 2013. “The Retraction Penalty: Evidence from the Web of Science.” Scientific Reports 3 (3146): 1–5. doi:https://doi.org/10.1038/srep03146.
- Mansfield, E. R., and B. P. Helms. 1982. “Detecting Multicollinearity.” The American Statistician 36 (3a): 158–160.doi:https://doi.org/10.2307/2683167.
- Manton, E. J., and D. E. English. 2007. “The Trend toward Multiple Authorship in Business Journals.” Journal of Education for Business 82 (3): 164–168. doi:https://doi.org/10.3200/JOEB.82.3.164-168.
- Marx, M., and A. Fuegi. 2020. “Reliance on Science: Worldwide Front-Page Patent Citations to Scientific Articles.” Strategic Management Journal 41: 1572–1594. Forthcoming. doi:https://doi.org/10.1002/smj.3145.
- McAfee, R. P., H. M. Mialon, and S. H. Mialon. 2010. “Do Sunk Costs Matter?” Ecnomic Inquiry 48 (2): 323–336. doi:https://doi.org/10.1111/j.1465-7295.2008.00184.x.
- Merton, R. K. 1969. “Behavior Patterns of Scientists.” The American Scholar 57 (1): 1–23.
- Meslec, N., P. L. Curseu, O. C. Fodor, and R. Kenda. 2020. “Effects of Charismatic Leadership and Rewards on Individual Performance.” The Leadership Quarterly 31 (6): 101423. doi:https://doi.org/10.1016/j.leaqua.2020.101423.
- Miller, A. N., S. G. Taylor, and A. G. Bedeian. 2011. “Publish or Perish: Academic Life as Management Faculty Live It.” Career Development International 16 (5): 422–445. doi:https://doi.org/10.1108/13620431111167751.
- Moedas, C. 2015. “Open Innovation, Open Science, Open to the World.” European Commission, June 22. https://europa.eu/rapid/press-release_SPEECH-15-5243_en.htm
- Molloy, J. C. 2011. “The Open Knowledge Foundation: Open Data Means Better Science.” PLoS Biology 9 (12): e100195. doi:https://doi.org/10.1371/journal.pbio.1001195.
- Mons, B., C. Neylon, J. Velterop, M. Dumontier, L. O. B. Da Silva Santos, and M. D. Wilkinson. 2017. “Cloudy, Increasingly FAIR: Revisiting the FAIR Data Guiding Principles for the European Open Science Cloud.” Information Services & Use 37 (1): 49–56. doi:https://doi.org/10.3233/ISU-170824.
- Morey, R. D., C. D. Chambers, P. J. Etchells, C. R. Harris, R. Hoekstra, D. Lakens, S. Lewandowsky, et al. 2016. “The Peer Reviewers’ Openness Initiative: Incentivizing Open Research Practices through Peer Review.” Royal Society Open Science 3 (1): 150547. doi:https://doi.org/10.1098/rsos.150547.
- Muscio, A., D. Quaglione, and G. Vallanti. 2013. “Does Government Funding Complement or Substitute Private Research Funding to Universities?” Research Policy 42 (1): 63–75. doi:https://doi.org/10.1016/j.respol.2012.04.010.
- Nosek, B. A., G. Alter, G. C. Banks, D. Borsboom, S. D. Bowman, S. J. Breckler, S. Buck, et al. 2015. “Promoting an Open Research Culture.” Science 348 (6242): 1422–1425. doi:https://doi.org/10.1126/science.aab2374.
- Nunnally, J. C., and I. H. Bernstein. 1994. Psychometric Theory. 3rd ed. New York: McGraw-Hill.
- Penrose, E. 1955. “Limits to the Growth and Size of Firms.” American Economic Review 45 (2): 531–543.
- Peters, I., P. Kraker, E. Lex, C. Gumpenberger, and J. Gorraiz. 2016. “Research Data Explored: An Extended Analysis of Citations and Altmetrics.” Scientometrics 107 (2): 723–744. doi:https://doi.org/10.1007/s11192-016-1887-4.
- Pfeffer, J., and G. R. Salancik. 1978. The External Control of Organizations: A Resource Dependence Perspective. New York: Harper & Row.
- Pfenninger, S., J. DeCarolis, L. Hirth, S. Quoilin, and I. Staffell. 2017. “The Importance of Open Data and Software: Is Energy Research Lagging Behind?” Energy Policy 101 (3): 211–215. doi:https://doi.org/10.1016/j.enpol.2016.11.046.
- Piccoli, G., and B. Ives. 2005. “IT-Dependent Strategic Initiatives and Sustained Competitive Advantage: A Review and Synthesis of the Literature.” MIS Quarterly 29 (4): 747–776. doi:https://doi.org/10.2307/25148708.
- Piwowar, H. A., and W. Chapman. 2008. “A Review of Journal Policies for Sharing Research Data.” Paper presented at the International Conference on Electronic Publishing, Toronto, June 26-28.
- Piwowar, H. A., R. S. Day, and D. B. Fridsma. 2007. “Sharing Detailed Research Data Is Associated with Increased Citation Rate.” PLoS ONE 2 (3): e308. doi:https://doi.org/10.1371/journal.pone.0000308.
- Podsakoff, P. M., S. B. MacKenzie, J. Y. Lee, and N. P. Podsakoff. 2003. “Common Method Biases in Behavioral Research: A Critical Review of the Literature and Recommended Remedies.” Journal of Applied Psychology 88 (5): 879. doi:https://doi.org/10.1037/0021-9010.88.5.879.
- Pratt, M. G., S. Kaplan, and R. Whittington. 2020. “Editorial Essay: The Tumult over Transparency: Decoupling Transparency from Replication in Establishing Trustworthy Qualitative Research.” Administrative Science Quarterly 65 (1): 1–19. doi:https://doi.org/10.1177/0001839219887663.
- Reich, E. S. 2012. “Boost for Higgs from Tevatron Data.” Nature, March 7. Accessed 26 September 2019. https://www.nature.com/news/boost-for-higgs-from-tevatron-data-1.10167
- Reichman, O. J., M. B. Jones, and M. P. Schildhauer. 2011. “Challenges and Opportunities of Open Data in Ecology.” Science 331 (6018): 703–705. doi:https://doi.org/10.1126/science.1197962.
- Reidpath, D. D., and P. A. Allotey. 2001. “Data Sharing in Medical Research: An Empirical Investigation.” Bioethics 15 (2): 125–134. doi:https://doi.org/10.1111/1467-8519.00220.
- Reynolds, P. D. 2007. New Firm Creation in the United States: Initial Explorations with the PSED I Data Set. Hanover, MA: Now Publishers.
- Ricardo, D. 1891. Principles of Political Economy and Taxation. London: Bell & Sons.
- Ross, J. S., and H. M. Krumholz. 2013. “Ushering in a New Era of Open Science through Data Sharing.” Journal of the American Medical Association 309 (13): 1355–1356. doi:https://doi.org/10.1001/jama.2013.1299.
- Salita, J. T. 2010. “Authorship Practices in Asian Cultures.” The Write Stuff 19 (1): 36–38.
- Savage, C. J., and A. J. Vickers. 2009. “Empirical Study of Data Sharing by Authors Publishing in PLoS Journals.” PLoS ONE 4 (9): e7078. doi:https://doi.org/10.1371/journal.pone.0007078.
- Schmidt, S. 2009. “Shall We Really Do It Again? the Powerful Concept of Replication Is Neglected in the Social Sciences.” Review of General Psychology 13 (2): 90–100. doi:https://doi.org/10.1037/a0015108.
- Siemsen, E., A. Roth, and P. Oliveira. 2010. “Common Method Bias in Regression Models with Linear, Quadratic, and Interaction Effects.” Organizational Research Methods 13 (3): 456–476. doi:https://doi.org/10.1177/1094428109351241.
- Sorenson, O., V. Assenova, G. C. Li, J. Boada, and L. Fleming. 2016. “Expand Innovation Finance via Crowdfunding.” Science 354 (6319): 1526–1528. doi:https://doi.org/10.1126/science.aaf6989.
- Stanton, J. M. 2006. “An Empirical Assessment of Data Collection Using the Internet.” Personnel Psychology 51 (3): 709–725. doi:https://doi.org/10.1111/j.1744-6570.1998.tb00259.x.
- Teixeira da Silva, J. A. T., and J. Dobranszki. 2015. “Potential Dangers with Open Access Data Files in the Expanding Open Data Movement.” Publishing Research Quarterly 31 (4): 298–305. doi:https://doi.org/10.1007/s12109-015-9420-9.
- Tenopir, C., S. Allard, K. Douglass, A. E. Aydinoglu, L. Wu, E. Read, M. Manoff, and M. Frame. 2011. “Data Sharing by Scientists: Practices and Perceptions.” PLoS ONE 6 (6): e21101. doi:https://doi.org/10.1371/journal.pone.0021101.
- Tenopir, C., E. D. Dalton, S. Allard, M. Frame, I. Pjesivac, B. Birch, D. Pollock, et al. 2015. “Changes in Data Sharing and Data Reuse Practices and Perceptions among Scientists Worldwide.” PloS ONE 10 (8): e0134826. doi:https://doi.org/10.1371/journal.pone.0134826.
- Thibaut, J. W., and H. H. Kelley. 1959. The Social Psychology of Groups. New York: Wiley & Sons.
- Thursby, J. G., C. Haeussler, M. C. Thursby, and L. Jiang. 2018. “Prepublication Disclosure of Scientific Results: Norms, Competition, and Commercial Orientation.” Science Advances 4: eaar2133. doi:https://doi.org/10.1126/sciadv.aar2133.
- Uhlir, P. F., and P. Schröder. 2007. “Open Data for Global Science.” Data Science Journal 6: 36–53.doi:https://doi.org/10.2481/dsj.6.OD36.
- Van Den Berg, H. 2005. “Reanalyzing Qualitative Interviews from Different Angles: The Risk of Decontextualization and Other Problems of Sharing Qualitative Data.” Forum: Qualitative Social Research 6 (1): 30.
- Vicente-Sáez, R., and C. Martínez-Fuente. 2018. “Open Science Now: A Systematic Literature Review for an Integrated Definition.” Journal of Business Research 88 (1): 428–436. doi:https://doi.org/10.1016/j.jbusres.2017.12.043.
- von Hippel, E. 2017. Free Innovation. Cambridge, MA: MIT Press.
- Wade, M., and J. Hulland. 2004. “The Resource-Based View and Information Systems Research: Review, Extension and Suggestions for Future Research.” MIS Quarterly 28 (1): 107–142. doi:https://doi.org/10.2307/25148626.
- Wallis, J. C., E. Rolando, and C. L. Borgman. 2013. “If We Share Data, Will Anyone Use Them? Data Sharing and Reuse in the Long Tail of Science and Technology.” PloS One 8 (7): e67332. doi:https://doi.org/10.1371/journal.pone.0067332.
- Walport, M., and P. Brest. 2011. “Sharing Research Data to Improve Public Health.” The Lancet 377 (9765): 537–539. doi:https://doi.org/10.1016/S0140-6736(10)62234-9.
- Walsh, J. P., and W. Hong. 2003. “Secrecy Is Increasing in Step with Competition.” Nature 422: 801–802. doi:https://doi.org/10.1038/422801c.
- Wang, S., and R. A. Noe. 2010. “Knowledge Sharing: A Review and Directions for Future Research.” Human Resource Management Review 20 (2): 115–131. doi:https://doi.org/10.1016/j.hrmr.2009.10.001.
- Wernerfelt, B. 1984. “A Resource-Based View of the Firm.” Strategic Management Journal 5 (2): 171–180. doi:https://doi.org/10.1002/smj.4250050207.
- West, J., A. Salter, W. Vanhaverbeke, and H. Chesbrough. 2014. “Open Innovation: The Next Decade.” Research Policy 43 (5): 805–811. doi:https://doi.org/10.1016/j.respol.2014.03.001.
- Wicherts, J. M., D. Borsboom, J. Kats, and D. Molenaar. 2006. “The Poor Availability of Psychological Research Data for Reanalysis.” American Psychologist 61 (7): 726–728. doi:https://doi.org/10.1037/0003-066X.61.7.726.
- Wilbanks, J., and S. H. Friend. 2016. “First, Design for Data Sharing.” Nature Biotechnology 34 (4): 377–379. doi:https://doi.org/10.1038/nbt.3516.
- Wilkinson, M. D., M. Dumontier, I. J. Aalbersberg, G. Appleton, M. Axton, A. Baak, N. Blomberg, et al. 2016. “The FAIR Guiding Principles for Scientific Data Management and Stewardship.” Scientific Data 3 (1): 1–9. doi:https://doi.org/10.1038/sdata.2016.18.
- Young, C., and K. Holsteen. 2017. “Model Uncertainty and Robustness: A Computational Framework for Multimodel Analysis.” Sociological Methods & Research 46 (1): 3–40. doi:https://doi.org/10.1177/0049124115610347.
- Yu, S., S. Johnson, C. Lai, A. Cricelli, and L. Fleming. 2017. “Crowdfunding and Regional Entrepreneurial Investment: An Application of the CrowdBerkeley Database.” Research Policy 46 (10): 1723–1737. doi:https://doi.org/10.1016/j.respol.2017.07.008.