ABSTRACT
Government funding entities have placed data sharing at the centre of scientific policy. While there is widespread consensus that scientific data sharing benefits scientific progress, there are significant barriers to its wider adoption. We seek a deeper understanding of how researchers from different fields share their data and the barriers and facilitators of such sharing. We draw upon the notions of epistemic cultures and collective action theory to consider the enablers and deterrents that scientists encounter when contributing to the collective good of data sharing. Our study employs a mixed-methods design by combining survey data collected in 2016 and 2018 with qualitative data from two case studies sampled within two scientific communities: high-energy physics and molecular biology. We describe how scientific communities with different epistemic cultures can employ modularity, time delay, and boundary organisations to overcome barriers to data sharing.
1. Introduction
In September 2011, researchers in the Oscillation Project with Emulsion-tRacking Apparatus (OPERA) fired a 730-km beam of muon neutrinos from European Organisation for Nuclear Research (CERN) in Geneva, Switzerland to the Gran Sasso National Laboratory in central Italy at what appeared to be a velocity faster than the speed of light. Puzzled by this result, researchers uploaded the data, with unprecedented granularity, to the open access archive arXiv.org. The data included all the necessary procedural descriptions to enable other scientists to search for an explanation of this surprising violation of a physical law. Subsequently, more than 200 papers were posted on arXiv.org attempting to explain the anomalous result. With ruthless external scrutiny, the mystery was resolved within a year – the OPERA team announced the identification of two potential sources of timing error that had corrupted the measurements (Royal Society Citation2012). More recently, the COVID-19 pandemic has exemplified the value of scientific data sharing, as data sharing was critical for understanding the methods of transmission and infection of the SARS-CoV-2 virus, as well as the symptoms. Within a short time, the extensive and timely sharing of COVID-19-related data informed the rapid development of vaccines (e.g. EMBL-EBI COVID-19 data portal)Footnote1 (Fegan and Cheah Citation2021). There are numerous additional examples of the value of data sharing among scientific communities, although researchers across disciplines engage with data sharing in vastly different ways (Tenopir et al. Citation2015).
The relevance of data sharing has become prominent in recent years, as scientific research is generating ever-increasing volumes of data (Hey Citation2009). Some disciplines have a long tradition of working with high volumes of data, particularly the big science research infrastructures (Weinberg Citation1961) in physics and astronomy (Atkins et al. Citation2003; Borgman Citation2012, Citation2015; Carillo and Papagni Citation2014), whereas other scientific fields have just recently grown more data-intensive (EIROforum IT working group Citation2013). These disciplines include computational social science (Lazer Citation2009), digital humanities (Kaplan Citation2015), social media data (Plantin et al. Citation2018), citizen science research projects (Hochachka et al. Citation2012), and political science and public policy (Lee, Almirall, and Wareham Citation2016).
With the increased quantity of scientific data, perspectives on data sharing have evolved, leading to an increase in the role and status of data. Scientific data are now recognised as a scholarly object in their own right, with dedicated journals such as Nature-Scientific Data. With this shift in perspective, the increase in data-intensive methods has been labelled the ‘fourth paradigm’ in science (Atkins et al. Citation2003; Hey Citation2009), augmenting ‘the existing paradigms of experimental, theoretical, and computational science’ (Edwards et al. Citation2011, 670). As the potential of scientific big data grows, so too does the expectation to share data and allow others to mine, aggregate, and recombine them with other data for novel applications: ‘If the rewards of the data deluge are to be reaped, then researchers who produce those data must share them, and do so in such a way that the data are interpretable and reusable by others’ (Borgman Citation2012, 1059). Data reuse can be facilitated by making data Findable, Accessible, Interoperable, and Reusable (FAIR)Footnote2 (Wilkinson et al. Citation2016). Recent studies have estimated the annual financial cost of not sharing FAIR data to be at least €10.2bn for the European economy, while the impact of FAIR on potential economic annual growth is estimated to be €16bn annually (European Commission Citation2019).
The importance of sharing FAIR data comes as part of a more general ‘open’ movement, embracing greater transparency in science (Edwards Citation2019). Starting with open access publishing, the open movement extends to open scientific data, open standards, open repositories, open bibliography, open lab-notebooks, open-source software and hardware – a virtually endless list of ‘open’ qualifiers to all activities in the scientific realm (Friesike et al. Citation2015). The urgency of sharing FAIR data is not only based on concerns of reproducibility (Baker Citation2015) or scientific fraud (Kupferschmidt Citation2018), but also in recognition of the novel technological and scientific innovations that can result from data sharing (Borgman Citation2010). As such, government funding entities, particularly in Western Europe and the United States, have placed open data at the crux of scientific policy. As European Union Commissioner for Research, Science, and Innovation, Carlos Moedas made open research data one of the EU’s priorities in 2015. This led to the formation of several expert working groups (e.g. High-level expert group on FAIR data, the Open Science Policy Platform, Expert group on altmetrics) to provide advice on how to foster and promote research data sharing in Europe. In 2016, the EU launched the Open Science Cloud initiative, which is a federated data infrastructure with cloud-based services to provide the scientific community with an open environment for storing, sharing, and reusing scientific data. In parallel, many funding agencies now require that scientific data be publicly available: for example, the US National Institutes of Health (NIH) has required this since 2003 for grants over $500,000 (NIH Citation2003), the National Science Foundation (NSF) since 2010 (Borgman Citation2012), and the European Commission for the Horizon 2020 programme since 2014 (European Commission Citation2014). Accompanying policy, new private and public entities have emerged to facilitate the aggregation and publication of research data. Examples include the Research Data Alliance and the National Data Service, as well as for-profit publishers who attempt to build on existing structures (e.g. Mendeley Data) (Borgman Citation2015). Platforms such as Dataverse (King Citation2007), FigShare (Thelwall and Kousha Citation2016), Zenodo (Peters et al. Citation2017), DataHub (Bhardwaj et al. Citation2014), EUDat (Lecarpentier et al. Citation2013), and other data repositoriesFootnote3 that offer scholars new venues to archive and share their data have also emerged (Cragin et al. Citation2010).
Although scientific data sharing has been positively promoted for some time, several challenges that inhibit data sharing have become apparent. Critics have pointed out that data sharing imposes increased costs on scientists and their institutions without commensurate professional benefits (Borgman Citation2015; Edwards Citation2019; Edwards et al. Citation2011; Tenopir et al. Citation2015; Wallis et al. Citation2013). More importantly, while consistent with the Mertonian norm of sharing to build cumulative academic knowledge (Merton Citation1973), data sharing clashes with a system of scientific rewards where ‘the first person to discover a result gets the “prize” associated with discovery’ (Haeussler et al. Citation2014, 465; Dasgupta and David Citation1987; Stephan Citation1996). There is therefore a tension between Mertonian ideals and the actual incentives of scientists who perceive data sharing as costly without commensurate professional recognition (Hagstrom Citation1974; Dasgupta and David Citation1994; Murray and O’Mahony Citation2007). While the prevalence of limited data sharing is known (Blumenthal et al. Citation1996; Campbell and Bendavid Citation2002; Haeussler et al. Citation2014; Mukherjee and Stern Citation2009), there is a limited understanding of the complexities and intricacies of how – and where – actual data sharing occurs.
To understand the enablers and deterrents of scientific data sharing practices, we draw upon both cultural and economic perspectives. From one side, we draw upon the notion of ‘epistemic cultures’, which originates from the sociology of science and has been applied in organisational studies and Information Systems (IS) to understand information and knowledge sharing across communities (e.g. Kellogg, Orlikowski, and Yates Citation2006; Mørk et al. Citation2008). We follow anthropologist Knorr Cetina (Citation1999) to understand the challenges and processes involved in scientists’ epistemic work. This perspective predicts that researchers from different scientific communities will share relatively more – or less – compared to other scientific communities due to differences in disciplines’ shared norms. On the other side, taking an economic perspective, we employ collective action theory (Hess and Ostrom Citation2003; Olson Citation1965; Ostrom Citation1990) to understand the disincentives and deterrents that scientists face when considering data contributions to common information infrastructures for the collective good (Constantinides Citation2012; Constantinides and Barrett Citation2015; Vassilakopoulou, Espen, and Aanestad Citation2016). While both sociological and economic perspectives potentially offer theoretical explanations of differences in scientists’ data sharing practices, the processes that lead researchers from different scientific fields to share – or not share – are not well understood. Using a mixed-methods design (Venkatesh, Brown, and Bala Citation2013) (), this study seeks to address two key research questions:
1.1. RQ1: why and how do researchers from different scientific fields share their data?
1.1.1. RQ2: what mechanisms mitigate deterrents to researchers’ data sharing?
To address RQ1, we employ survey data collected in 2016 (n = 1,162) and 2018 (n = 1,029) to explore why and how scientists share their data, considering contextual differences across scientific communities as well as data-sharing behaviour at an individual level. For RQ2 we take a case study approach to identify the mechanisms that overcome data-sharing deterrents by comparing two distinct scientific communities: high-energy physics and molecular biology (Knorr Cetina Citation1999). High-energy physics is a field with a communitarian culture, whereas molecular biology is relatively individualistic (Knorr Cetina Citation1999), and have both achieved high data sharing levels, but in different ways. Substantial differences in the scientific technologies, processes, and norms between these two disciplines provide the variance needed to identify and qualify the levers and incentives that underpin data sharing practices.
The contributions of the paper are twofold. First, we provide evidence and develop theory about the enablers and deterrents of scientific data sharing behaviour. Specifically, we provide a nuanced understanding of how both individual and discipline-level factors interact to determine researchers’ data sharing attitudes and behaviours. Consistent with predictions from epistemic culture theory, we find that researchers from different fields vary in data sharing practices due to their experienced norms. In agreement with collective action theory, we also find that researchers will respond to their perceived individual incentives regarding data sharing. Additionally, our analysis identifies three concrete mechanisms – modularity, time delay, and boundary organisations – that overcome scientists’ limited data sharing as a collective resource of the scientific corpus. Elaboration of these mechanisms can inform open science policies struggling to shape scientists’ willingness to embrace scientific data sharing.
In the remainder of the paper, first we contextualise the research setting by reviewing the background concepts of data sharing from the organisational studies, IS, and Science and Technology Studies (STS) literature and identify the incentives and reasons for sharing – or not sharing – scientific data. We then review the theoretical foundations of our research study and sequentially describe our methods and results. Methods and results from the survey data are presented first and then the methods and results from the case studies (Venkatesh, Brown, and Bala Citation2013). Finally, we synthesise the findings and discuss the theoretical and practical implications of the study, its limitations, and future directions.
2. Conceptual background
‘Data are representations of observations, objects, or other entities used as evidence of phenomena for research or scholarship’ (Borgman Citation2015, 18). A more operational definition from Open Archival Information System (OAIS) defines data as ‘a reinterpretable representation of information in a formalized manner suitable for communication, interpretation, or processing’. Examples of data include: ‘sequence of bits, a table of numbers, the characters on a page, the recording of sounds made by a person speaking, or a moon rock specimen’ (Consultative Committee for Space Data Systems Citation2012, 10).
An important qualification is that the research context determines what becomes data and how data are processed (Kallinikos and Constantiou Citation2015). As such, it becomes paramount that all relevant contextual information is gathered in the description of the data; that is, the correct and complete metadata are critical to optimising the utility of data across disciplines, time, geographies, or application domains (Edwards et al. Citation2011). The genesis of data may also affect an operational decision of whether to preserve the data and for how long (National Science Board Citation2005). For instance, observational data are considered essential to preserve, because they are the most difficult to replicate. Nevertheless, the question remains as to who makes such decisions: who has the authority to decide whether to destroy, share, or withhold data?
Historically, the dominant perspective is that the ‘producers’ of scientific data hold the authority to disclose or withhold it. Yet, with the increasing volumes of data generated by publicly funded programmes and computationally intensive environments, the ‘scientist-as-owner’ paradigm obscures a rather complex and more subtle picture of the different stakeholders involved in data production. Whether it is the scientist, team, lab, repository, or other organisational layer, the allocation of data ownership requires a careful examination of how data-sharing incentives and deterrents interact across diverse institutional levels.
2.1. Enablers and deterrents for data sharing: two theoretical perspectives
The tenor of Isaac Newton’s famous quote – ‘If I have seen further than others, it is by standing upon the shoulders of giants’ (Kuhn Citation1962) – is still prevalent in scientific practice. The progression of science relies on the accumulation of knowledge and, thus, the sharing of the results of prior research. Yet even though the economic costs and needed efforts of data processing and storage are falling (Romasanta and Wareham Citation2021), how the accumulation and reuse of knowledge occurs within and across disciplines is often unclear, confounding scientists’ motivations for data sharing.
Prior literature suggests that while authors are initially the copyright holders of their academic publications, the jurisdiction over the data is more ambiguous: uncertainty around ownership, control, and access to the data generates tensions amongst stakeholders (Borgman Citation2015). ‘Even when individuals and groups assign authority for data, the rights and responsibilities may remain unclear’ (Bowker and Leigh Star Citation1999, 646). As a consequence, policymakers, funders, and academic institutions are working to increase awareness that, while the publications and knowledge derived from research data pertain to the authors, research data needs to be considered a public good so that its potential social and scientific value can be realised (European Commission Citation2014; OECD Citation2015; Järvenpää and Markus Citation2018; Vassilakopoulou, Espen, and Aanestad Citation2016).
2.2. Epistemic cultures
Knorr Cetina (Citation1999) coined the notion of epistemic cultures to describe ‘those amalgams of arrangements and mechanisms – bonded through affinity, necessity and historical coincidence – which, in a given field, make up how we know what we know’ (p. 1). The notion of epistemic culture claims that the nature of scientific activities, types of reasoning, and practices of establishing evidence are variable across scientific fields. The epistemic cultural approach disputes the ‘unity of science’ associated with the Vienna Circle (Knorr Cetina Citation1999) and ‘reveals the fragmentation of contemporary science’ (Mørk et al. Citation2008, 15).
The concept of different scholarly cultures can be drawn back to the idea of ‘styles of thought’ shared by ‘thought collectives’ (Knorr Cetina Citation1999); it also relates to a concept of ‘thought worlds’ (Dougherty Citation1992) or the idea of ‘communities of knowing’ (Boland and Tenkasi Citation1995). Haas (Citation1992) used the notion of ‘epistemic communities’ to define groups of people engaged in knowledge production. The general and universal idea across such notions is that knowledge is situated and local. ‘There is no “view from nowhere” – knowledge is always situated in a place, time, conditions, practices, and understandings’(Borgman Citation2012 p.37).
In the context of our study, Knorr Cetina’s ideas are useful because the definition of ‘culture’ is rooted in practice. The ‘epistemic machinery’ defines the shared tools, techniques, instruments, methods, and architectures of shared empirical practices that the epistemic subjects use to produce and distribute knowledge. She describes the making of science through interiorised processes where scientists, organisations, and collectives operate (e.g. labs and experiments) (Knorr Cetina Citation2007).
Employing Knorr Cetina’s lens, data sharing practices would be expected to be community-bound and largely determined by epistemic culture. This is logical if we consider the long cycles through which new members are trained; the specificities in the technology tools; the commonly accepted methods, funding sources, collaborative norms; and the ways in which responsibility and authorship are assigned. Differences in data sharing practices would also depend on whether the scientific community is more communitarian or individualistic: a communitarian culture should be predisposed to share, with fewer concerns about individual incentives and rewards; whereas an individualistic culture would be more driven by individual motivations that give weight to the costs of sharing data and the lack of clear individual compensations.
2.3. Collective action theory
To examine the mechanisms by which self-interested researchers would contribute to a data as a public good, we employ collective action theory and the idea of a data as commons. The term ‘commons’ designates a ‘resource shared by a group of people that is subject to social dilemmas’ (Hess and Ostrom Citation2003 p.3).
Collective action theory has been widely used in sociology and economics to understand individuals’ motivation to engage in collective action (Fulk et al. Citation2004; Monge et al. Citation1998). Collective action research originated with Olson’s work in the classic Logic of Collective Action (Olson Citation1965), from which Hardin (Citation1968) developed his ‘tragedy of the commons’ thesis, suggesting that uncontrolled individual self-interest pursuits can undermine common public resources (Greco and Floridi Citation2004). In other words, the tragedy of the commons can be viewed as a prisoner’s dilemma (with n-people) where the rational pursuit of self-interest results in suboptimal management of public resources and social goods (e.g. forests, fisheries) (Greco and Floridi Citation2004; Fletcher and Zwick Citation2000; Ostrom Citation1986).
As Hardin (Citation1982) described, communities benefit when the individual perceives gains from making contributions to the commons. If such benefits are not perceived, the shared pool of resources is ‘latent’ and can deteriorate without external intervention. What makes collective action useful in understanding scientific data sharing is its focus on how the appropriation of individual gains is determined by adjusting the costs and benefits that accrue with contributions to a common resource (Fulk et al. Citation2004; Ostrom Citation1990; Vitali, Mathiassen, and Rai Citation2018; Weill and Ross Citation2004).
summarises common reasons cited to justify the benefits of data sharing along with frequent explanations for deterrents. Interestingly, whereas arguments for data sharing reflect benefits to the scientific community, reasons not to share data are predominantly based on concerns that individual costs exceed individual benefits. While not exclusively so, the theory of epistemic cultures largely emphasises norms that favour data sharing, where collective action theory is predominantly concerned with self-interest and individual costs.
Table 1. Enablers and deterrents for data sharing in science according to prior literature and implications from collective action and epistemic cultures perspectives
3. Methods and results
We first present the methods and results of the analysis of the survey data. We then follow with the case studies and synthesise the findings from both (Venkatesh, Brown, and Bala Citation2013). The synthesis of the results in the discussion is a ‘bridging’ process (Creswell Citation2018), where we leverage the complementarities across the findings to enrich our empirical and theoretical understanding of scientific data sharing practices.
3.1. Survey
3.1.1. Methods and data
We developed a large-scale global online survey in the framework of the Open Science Monitor for the European CommissionFootnote4 in collaboration with a consortium of scholars including a major academic publisher, and responses were collected in 2016 and 2018. The survey data allow us to address RQ1- why and how do researchers share their data? The survey of 2016 was sent in June–July 2016 by the publisher and generated 1,162 responses, which represented a 2.3% response rate. The margin of error for 1,162 responses was estimated ± 2.87% at 95% confidence levels (see prior analysis of the survey and full dataset in Meijer et al. Citation2017). The survey of 2018 was sent in October–November 2018 to 40,991 individuals randomly selected from the Scopus author database, weighted to be representative of the researcher population (UNESCO counts of researchers, Citation2013), to which 1,029 researchers responded (2.5% response rate). The responses in both surveys were anonymous, not containing any personal identifying information. There were several differences between the 2016 and 2018 survey questionnaires: four additional questions were added in 2018 to assess the consequences of data sharing for scientists in their future collaborations with for-profit entities. Additional minor modifications were introduced in the 2018 questionnaire to improve semantic clarity.
By using random sampling methods to ensure that the individuals selected were representative of the researcher population at large, the study sought to mitigate potential selection bias. Nonetheless, we can compare UNESCO’s count of researchers across fields with the mix we received from respondents in the survey. As we report in the Appendix, we have overrepresentation in the natural sciences and slight underrepresentation in engineering.
In the first phase of the analysis, we explore which factors promote or inhibit data sharing among individual researchers. Specifically, we examine two aspects: 1) their willingness to let others access their research data, and 2) actual experience in sharing their data. By analysing both attitudes and behaviours, we obtain a more holistic picture of data sharing. These two variables were measured through a 5-point Likert scale.
The independent variables were the factors related to data sharing enumerated in the literature review. For each of these factors (), we identified relevant survey questions as proxies to represent each enabler or deterrent, which were either ordinal or binary. The ordinal variables were measured through a 5-point Likert scale of agreement with statements provided. The binary variables were from a question asking respondents about the benefits of data sharing in their field.
Table 2. Factors related to data sharing
Respondents with missing data or a response of N/A were removed from the analysis. This left us with 491 respondents with complete data across the different variables we wanted to test in . Due to the dominance of non-continuous variables, we used diagonally weighted least squares (DWLS) to estimate the model parameters through the lavaan package in R (Rosseel Citation2012). DWLS is robust against non-normality and works better with ordinal data than maximum likelihood methods (Mîndrilă Citation2010) and performs well with smaller sample sizes (Flora and Curran Citation2004).
In the second phase of the analysis, we explored the differences in data sharing practices across scientific communities. We looked at the same two aspects of researchers’ data sharing: willingness to let others access their data and actual experience sharing data. Considering the ordinal nature of these variables, we used the Kruskal-Wallis test to determine whether different research fields have distinct willingness and experiences in sharing data. Kruskal–Wallis is a nonparametric statistical test that assesses the differences among three or more groups (Kruskal and Allen Wallis Citation1952; McKight and Najab Citation2010). In contrast to one-way Analysis of Variance (ANOVA), which is used for normally distributed continuous variables, Kruskal–Wallis is more appropriate for ranked data such as those from our survey. To identify which specific fields share more – or less – than other fields, we followed up with Dunn’s test, the pairwise multiple-comparison procedure used when a Kruskal–Wallis test is rejected (Dunn Citation1961; Dinno Citation2015). To correct for the increased false positives that arise from conducting more statistical tests, we also present the results after Holm’s sequential adjustment (Holm Citation1979).
3.1.2. Findings of the survey
We began the analysis with a focus on data sharing for individual researchers. To do this, we used the various factors identified under epistemic cultures and collective action and related these to both researchers’ willingness and previous experience in data sharing.
We found support for epistemic norms stimulating data sharing. Our results suggest that researchers had positive data sharing outcomes in both attitude and behaviour, if they believed that the data are crucial to the advancement of their research field. Moreover, the push for data reproducibility, data reuse, and collaborations in their field also seemed to lead researchers to engage with data sharing (). Furthermore, supporting individual incentives to overcome collective action problems was related to positive data sharing outcomes. Compliance with funding bodies was related to increased willingness to share data. Receiving necessary skill training also seemed to lead to data sharing engagement. In summary, various individual-level factors seemed to motivate researchers’ sharing of data.
Table 3. Researcher willingness to share data for various reasons and experience of it
We present varying attitudes and behaviour towards data sharing among scientific disciplines in . Visual examination shows a mixed finding, where many disciplines are similar in data sharing, and some disciplines in the extremes share more or share less compared to the average. To make a better judgement of these disciplines’ differences, we used the Kruskal-Wallis test, which confirmed that certain disciplines differ in their data-sharing activity. By their willingness to allow others access to research data, we found differences across disciplines (statistic = 26.9, p-value = 0.003). Moreover, in their experience sharing of data, we also found significant differences (statistic = 25.7, p-value = 0.004).
Figure 2. Data sharing by discipline.
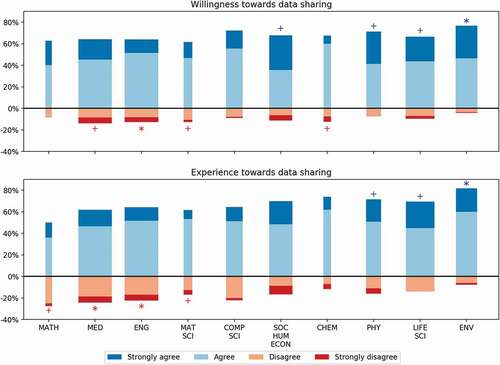
To identify which disciplines differed in data sharing, we used post-hoc pairwise Dunn test with Holm correction. Researchers in environmental sciences tend to be the most willing to allow others access to their research data. This is relative to the disciplines of medicine and engineering, where many respondents mentioned that they are not as engaged in data sharing. As for the remainder of the disciplines, we do not find significant differences in their sharing of data. In continuation, we explored the various outcomes of data sharing: with whom data is shared, where it is shared, and what type of data is shared. This information is summarised in .
Figure 3. Data sharing outcomes across all fields.
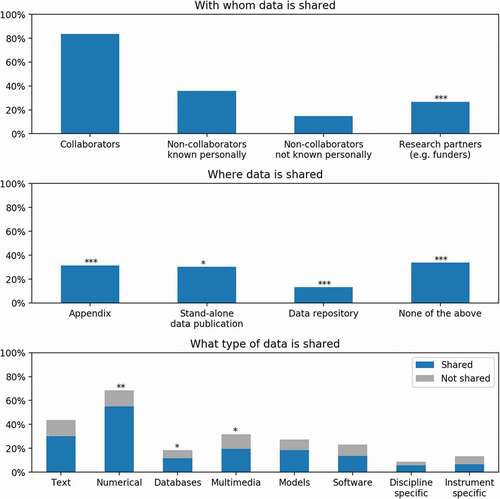
Regarding the question of with whom researchers tend to share their data, we find that they are highly discriminatory. Although they may grant non-collaborators access to their data through personal communication, we see that researchers tend to be most comfortable sharing with collaborators they know personally. This was consistent across all fields. The most variance we see across fields is whether they share data with their research partners such as funders ().
Location of data storage differed significantly among the disciplines. Certain fields prefer to publish data (1) in the appendix, (2) as stand-alone peer-reviewed data publications, (3) in data repositories, or (4) through other avenues altogether. Finally, fields also vary across the types of data they generate and, logically, the types of data they are willing to share. Across all fields, the primary type of data that researchers generate is numerical, followed by textual data, although fields do not vary in their rates of sharing such data.
What becomes clear from the survey results is that data sharing is a practice that varies not only across disciplines, but also between researchers from the same discipline. In other words, the results of the questionnaire thus far reveal a complex picture where discipline-level and individual-level factors are highly interlinked. Consistent with an epistemic culture explanation, we see that certain disciplines are more open to sharing than others. Additionally, consistent with a collective action perspective, we find that individual researchers within the same discipline can vary in their data sharing.
To contextualise our subsequent cases studies, we highlight the specific results for physics and astronomy and life science as they compare to all other fields across the survey items. Both the disciplines of Life Science and Astronomy/Physics share data at high levels as compared to the other fields surveyed. As seen in , they share the same motivators and deterrents in data sharing despite the theoretical differences previously emphasised in the literature. Comparing the two would provide useful cross-discipline variance for addressing RQ2.
Figure 4. Comparison of data sharing among disciplines, highlighting life sciences and physics/astronomy.
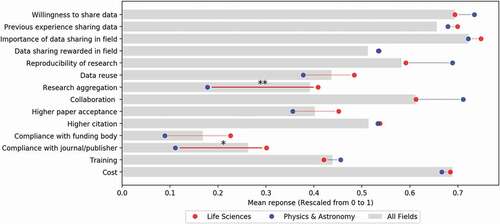
4. Case studies
4.1. Methods and data
To capture the data sharing practices of life science and physics, we thematically sampled (Creswell Citation2018) high-energy physics (HEP) and molecular biology (MB) communities’ practices anchored in two information infrastructures. Information infrastructures have been defined as ‘a digital library system based on commonly shared standards and containing information of both local and/or widespread interest’ (Kahn and Cerf Citation1988, 3), designed or intended ‘to augment our ability to search for, correlate, analyse and synthesize available information’ (Kahn and Cerf Citation1988, 11). Our decision to focus on information infrastructures (as opposed to less-institutionalised data sharing practices) is because the highest data sharing levels are in communities that actively use information infrastructures (Edwards et al. Citation2009; Ribes and Lee Citation2010). Both cases represent infrastructures based upon data commons, i.e. infrastructures that co-locate data, storage, and computing facilities with commonly used services and tools for analysing and sharing data for a defined base of users (Grossman et al. Citation2016).
4.1.1. Empirical context 1: molecular biology and open targets
The sequencing of the human genome (Human Genome Project, HGP) is recognised as ‘the largest undertaking in the history of biological science’ (Chaguturu, Murad, and Murad Citation2014, 35). Not only did it transform biology into a data-driven discipline with a deluge of new data and computational techniques, but it also opened the debate about research data sharing. Celera, a private undertaking, initially announced their intention to patent fully-characterised important structures amounting to 100–300 genetic targets, which prompted considerable outcry (Leonelli Citation2012). In March 2000, U.S. President Bill Clinton announced that the data on the Human Genome Project (HGP) sequence should be made freely available to the entire research community. The HGP propelled discourse and debates on open research data to the forefront of molecular biology research (Leonelli Citation2012) and spawned a new generation of information infrastructures to generate, integrate, and curate the growing data pools with commonly used tools and analytical methods (Grossman et al. Citation2016; Vamathevan et al. Citation2019). As a result, the discipline has been very active in developing infrastructures based upon data commons, one of them being Open Targets (Pujol Priego and Wareham Citation2018).
Open Targets (OT) is a MB consortium created in 2015 by the European Molecular Biology Laboratory-European Bioinformatics Institute (EMBL- EBI), Europe’s flagship laboratory for life science, with the Wellcome Sanger Institute, and five pharmaceutical companies (i.e. Biogen, Celgene, GSK, Sanofi, Takeda); it aims to accelerate knowledge about the links between genetic targets and disease development. The OT consortium includes publicly funded, non-profit, and for-profit organisations with vastly divergent institutional objectives and stakeholders. The infrastructure started as a result of pharmaceutical companies searching to scale requisite R&D capabilities by generating, integrating, and curating large data pools with commonly used tools and analytical methods about the early phases of drug discovery for the research community (Grossman et al. Citation2016; Vamathevan et al. Citation2019). The architecture, data policies, and procedures from researchers participating in OT provide insights about the mechanisms that effectively foster data sharing across the MB research community. As of this writing, OT infrastructure contains more than 27,717 genetic targets, 7,999,050 associations, 13,445 diseases, and 20 data sources (Open Targets Citation2020).
4.1.2. Empirical context 2: high-energy physics and reana
Big scientific research infrastructures within HEP, such as CERN, have a long tradition of embracing open data. Large volumes of data generated via expensive, unique, and elaborate experiments make data preservation and reuse important. Reana is a reusable and reproducible research data analysis infrastructure that was created at CERN in 2018 to facilitate data and code reuse. The infrastructure was initiated to combat the reproducibility crisis in the particle physics field. CERN built Reana as a data infrastructure to allow the different HEP experiments to adhere to FAIR principles and facilitate data sharing and reuse in the community. Reana allows the reuse and reinterpretation of the data shared by helping HEP scientists to structure their input data, analysis code, containerised environments, and computational workflows to run the analysis on remote clouds (Pujol Priego and Wareham Citation2019). What makes Reana attractive is that the infrastructure helps to generalise computational practices employed by HEP scientists, thereby systematising reproducibility. The infrastructure supports a plurality of ‘container technologies (Docker), workflow engines (CWL, Yadage), shared storage systems (Ceph, EOS) and compute cloud infrastructures (Ku- Kubernetes/OpenStack, HTCondor)’ used by the HEP scientific community (Simko et al. Citation2018, 1). The infrastructure sits on extant platforms and services provided by CERN to the HEP community; these include Zenodo, a free and open data repository, and CERN open data portal, both of which are precedents to Reana infrastructure (Pujol Priego and Wareham Citation2019).
4.1.3. Data sources and analysis
The study of both cases relies on the diverse primary and secondary data sources described in . Numerous discussions with managers from Open Targets and Reana were an integral part of the Open Science Monitor, published by the European Commission in separate reports (Pujol Priego and Wareham Citation2018, Citation2019). Primary data included 18 semi-structured interviews and direct observations from a study visit at the Wellcome Genome Campus for the OT open days (June 2019) and recurrent study visits at CERN from 2018–2020. As part of participation in the two additional EU H2020 funded projects, the authors benefited from extensive conversations with policymakers, research infrastructure managers, data architects, and programmers, in which they discussed data sharing practices and future open research data initiatives (CS3MESH4EOSC part of European Open Science Cloud and ATTRACT funded by Research Infrastructure Innovation H2020-INFRAINNOV). The interview process was concluded when no significant additional insights were obtained from the data, and theoretical saturation was achieved.
Table 4. Data collection sources for both Molecular Biology (MB) and High-Energy Physics (HEP)
Secondary sources included data from media outlets, with 47 publications resulting from OT and Reana, 1 tutorial and 12 runnable examples about how to use the infrastructures. Additionally, 8 blog posts, 25 release notes, 2 webinars, several workshop presentations, and information available in the different HEP experiments and OT organisations’ websites were used. These secondary sources were very useful in the first stages of the analytical processes, enabling us to have more technically informed conversations in both the infrastructures and data sharing practices. The combination of our primary data with secondary data allowed us to crosscheck findings and build our theoretical inferences from the cases.
Data were analysed by performing a two-stage inductive analysis, relying on established procedures for inductive research (Miles and Huberman Citation1994). The first stage was devoted to reading the abundant material available online about OT, HEP experiments, and Reana. We produced brief summaries that moved from technical descriptions of the infrastructure to managerial inferences. In-depth interviews were then conducted to understand how scientists use the infrastructures. We performed the interviews and analysis in several iterations, and thus earlier transcripts informed and incorporated information emerging from later interviews. In addition, we contrasted the transcripts from the interviews with our analysis of secondary sources. We generated research memos that synthesised the emergent themes identified in the analysis and compared them with prior research. Finally, to validate our findings, we applied respondent validation (Miles and Huberman Citation1994) by sharing our initial findings with the study participants.
4.2. Findings of the case studies
Preliminary observations about HEP and MB communities suggest two different epistemic cultures consistent with Knorr Cetina’s thesis, with HEP being more communitarian and MB more individualistic. When looking at how HEP data flows are organised, we first realised the importance of the institutional entity of ‘the experiment’. In HEP, a limited number of capital-intensive experiments have been designed and constructed over 20 years. For example, CERN currently hosts seven large experiments on the Large Hadron Collider, four of which are elaborate international collaborations (ATLAS, CMS, ALICE, LHCb). By contrast, MB is organised around the ‘laboratory’ – or even teams within a single institution. Very often, the molecular biologists are shaped by the conviction that they need to compete ‘for the priority of important findings’ (Knorr Cetina Citation1999), generating competition within – and across – laboratories.
When comparing how HEP and MB ascribe contributions to an individual scientist, we soon realised that HEP publications list a vast number of authors, as the construction and operation of HEP experiments often depends on many people; the record being over 5,000 authors on one article from CERN (Aad et al. Citation2015). In MB, although there are also challenges in ascribing results to individual scientists, the experiments are typically far less capital-intensive and permit differentiation in contributions within smaller teams. Finally, it is worth noting that some MB research is closer to commercial organisations (life sciences and pharma), whereas HEP is traditionally considered basic research with a more extended pathway towards any commercial outcome (Wareham et al. Citation2021; Romasanta et al. Citation2021). Accordingly, we would expect a more competitive culture with less data sharing in MB than HEP.
4.2.1. Open targets
The architecture of the OT data infrastructure is modular, containing different layers of access rights and data standards that employ a variety of mechanisms for researchers to be able to share their data (compliant with post-HGP norms). The stratified architecture grants different access rights to the data, where data generators are awarded complete access to a hidden layer, augmented by a public data layer (with different rights) that is accessible to any researcher willing to reuse the data. This modularity simultaneously allows researchers to grasp any individual or competitive benefits of being the generators of the data, while also being compliant with the collective norms of data transparency and sharing.
The modular architecture, with different access rights, also engages a time delay between the generation of the data and the publication of the data in the infrastructure that spans, on average, two years. As an informant explains: ‘Everybody understands that until there is a formal publication after the project, there is no disclosure.’
Finally, the information infrastructure acts as a ‘boundary organisation’ (O’Mahony and Bechky Citation2008); that is, ‘structures capable of effectively mediating between disparate constituencies and establishing common ground among the differing interests in the play’ (Perkmann and Schildt Citation2015, 1134). An interviewee explains: ‘There is a need to coordinate the integration of data into OT, both from the projects that generate data but also with the data providers such as Chembl and Uniprot and all the data that goes into the platform to keep it up to date. We also work with the developer team that creates some of the features that users will use to visualize the data coming through.’
Modularity and time delay are coordinated by the boundary organisation: normative governance on data access and reuse is embedded in the infrastructure, where the ownership and responsibilities over the data are explicit. These three mechanisms fit in a ‘logic of exchange’ that seeks to maximise benefits for the researchers (that is, the potential of data reuse and the commercial interests of data generators), while minimising the costs of sharing data (e.g. loss of potential commercial value, publication rights, recognition). This is achieved through protocols and data standards. The fact that for-profit companies form a significant part of the OT consortium suggests that the mechanisms developed are effective in balancing incentives to scientists while mitigating the risks of a competitive loss to other re-users of their data.
4.2.2. Reana
CERN built Reana upon data access and preservation policies agreed within the main experiments. Although the data policies may differ slightly across experiments, they all stratify the data generated by the HEP community in four main layers: (a) data directly related to the publications, which include the complete documentation for the published results; (b) simplified data formats devoted to training exercises within the physics community; (c) reconstructed data, simulations, and software analysis to facilitate research analysis; and finally, (d) the raw data and associated software, allowing access to the full potential of the experimental data’s reuse (Pujol Priego and Wareham Citation2019). Data sharing is concentrated in data layers (b) and (c). Raw data (d) are not made available to other researchers to reuse for pragmatic reasons. For instance, one of the core CERN experiments, CMS (Compact Muon Solenoid), produces on average 1 petabyte (100 gigabytes) of ‘raw’ data per second; similar data volumes characterise other experiments. As the Large Hadron Collider (LHC) data policy explainsFootnote5 ‘It is practically impossible to make the full raw data-set from scientific endeavours of the scale of high-energy physics easily usable in a meaningful way outside of the collaboration […]It should be noted that, for these reasons, direct access to the raw data is not even permitted to individuals within the collaboration, and that instead the production of reconstructed data is performed centrally.’
Experiments also employ a time delay between the generation of the experimental data and the time of sharing with the external research community. These periods are also referred to as embargo periods that allow the data generators within the experiment to publish the results. As explained in the LHC experiment data policy: ‘In general data will be retained for the sole use of the collaboration for a period commensurate with the substantial investment in the effort needed to record, reconstruct and analyse those data. After this period, some portion of the data will then be made available externally, with this proportion rising with time …. The portion of the data which LHCb would normally make available is 50% after five years, rising to 100% after ten years.’
The main idea behind Reana’s infrastructure is to preserve software and data workflows so that they can enhance collaborative scientific work and diffuse knowledge of the experimental procedures (Dphep Study Group Citation2009). Such data sharing protocols and preservation techniques are embedded in the Reana framework and reinforce the need for quality metadata: ‘Our own experience from opening up vast volumes of data is that openness cannot simply be tacked on as an afterthought at the end of the scientific endeavour. Besides, openness alone does not guarantee reproducibility or reusability, so it should not be pursued as a goal in itself. Focusing on data is also not enough: it needs to be accompanied by software, workflow, and explanations, all of which need to be captured throughout the usual iterative and closed research lifecycle, ready for a timely open release with the results’ (Chen et al. Citation2018).
Reana acts as a boundary organisation or ‘interface’ to the experiment’s knowhow, so that other researchers outside the experiment can reuse it. While normative governance defining data access rights and responsibilities exists, it applied aapplied at the experiment level, not the infrastructure level. As such, the infrastructure is required to respect distinct data policies.
provides a detailed description of the progression of our empirical analysis towards the three theoretical constructs: modularity, time delay, and boundary organisations. summarises the similarities and dissimilarities identified from the Open Targets (MB) and Reana (HEP) analyses. While both scientific communities employ similar mechanisms to overcome scientists’ deterrents to share data, they differ in how such mechanisms are used in their respective infrastructures and scientific communities.
Table 5. Theoretical progression of our analysis
Table 6. Similarities and differences observed between Reana (HEP) and open targets (MB)
5. Discussion
Our study aimed to answer two research questions: (1) Why and how do researchers from different scientific fields share their data? and (2) What mechanisms enable researchers to share their data? Regarding RQ1, we find that data sharing varies significantly across certain disciplines, although data sharing attitude and experience can be similar across fields. Most of the data sharing is carried out between collaborators on the same projects, suggesting that researchers adopt a discriminatory approach by sharing data with selected partners. Addressing RQ2, we find that communitarian and individualistic scientific communities employ three mechanisms (with some variation) to enable data sharing: (1) modularity, (2) time delay, and (3) boundary organisations. These mechanisms serve to establish transparent data governance and facilitate the identification of the ‘bona fide’ researcher.
Scientists are often professionally competitive. In this sense, individual incentives, professional recognition, and status are important components of a scientist’s career. However, nothing precludes collective norms and values from coexisting alongside individual motives; most scientists care about advancing science as a social good yet seek recognition for their contributions to it. Our survey evidence suggests that data sharing perceptions and practices are highly variable among academic disciplines. We infer that differences in the balance between individual and collective orientations explains, at least partially, some of the larger variance of data sharing across academic communities (Fulk et al. Citation2004; Hardin Citation1982; Ostrom Citation1990; Vitali, Mathiassen, and Rai Citation2018).
The nature of, and manner in which, science is conducted across disciplines is also highly determinative of data sharing (Borgman Citation2012; Knorr Cetina Citation1999, Citation2007; Gläser et al. Citation2015). HEP primarily conducts fundamental research with few immediate applications in industry; that is, while physics research has informed industrial development in a multitude of ways, the path to commercial applications is a longer one. MB, by contrast, is often more proximate to the life sciences and pharma industries. In fact, much MB research is funded by big pharma (Contreras and Vertinsky Citation2016; Vertinsky Citation2014; Cain Citation2012; Mittleman, Neil, and Cutcher-Gershenfeld Citation2013). So where HEP researchers have little reason to refrain from disclosing research data once academic credit is recognised, MB’s proximity to industries premised on finite periods of IP protection makes the calculus of disclosure far more complex.
With this background, our case analysis contrasted two information infrastructures that deployed mechanisms to align scientists’ professional incentives with data sharing practices ().
Figure 5. Mechanisms that enable HEP and MB researchers to share data. The (+) show significant motivator/deterrent of data sharing from our study.
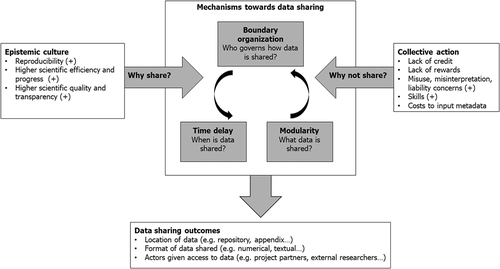
Our framework shows the tension between the community epistemic norms and the individual costs and benefits of data sharing. Our analysis identified three mechanisms to accommodate these tensions:
Data modularity enables data governance that acknowledges that research data are heterogeneous, as are the producers, audiences, and applications of such data. In HEP, more pragmatic considerations of the size and usability of data are determinative, while in MB, data modularity is conditioned by the applications of the data by its generators and consumers. Specifically, where most HEP research is publicly funded, MB research is funded by constellations of public and private sources. Consequently, demands for public disclosure need to be balanced with potential commercial appropriation for the private entities that have funded the research (Cain Citation2012; Mittleman, Neil, and Cutcher-Gershenfeld Citation2013).
Time delay also serves to balance any conflicting interests between the generators and consumers of data. In HEP, practical uncertainties about how data should be structured, analysed, or interpreted can require delays in its disclosure. In parallel, the requirements of the principal research teams who need sufficient time for data analysis and publication are also determinative. In MB, time delays serve a similar function: they permit the generators and funders of data to develop research leads towards commercial appropriability before releasing data into public platforms (Contreras Citation2010; Contreras and Vertinsky Citation2016).
Boundary organisations, finally, are of particular significance in their responsibility for data governance. These are defined by the different infrastructures required to conduct science in HEP and MB: the experiment or the information infrastructure. While both disciplines are data intensive, HEP requires particle accelerators, detection and imaging technologies of vast size, energies, and economic investment, that bind their operation to very large teams of scientists working on centrally coordinated and internationally funded experiments. This generates a highly collective culture with commensurate communal recognition and norms. Data collection, storage, and analysis are governed centrally, and are publicly transparent to a broad contingency of stakeholders whenever feasible. Most importantly, centralised data governance exists at the point of data genesis: for HEP, ‘data openness cannot simply be tacked on as an afterthought’ (Chen et al. Citation2018).
MB, by contrast, does not require the same level of public investment in infrastructure (it should, however, be noted that a significant amount of cell biology does transpire at larger synchrotron, free-electron laser, and neutron scattering facilities.) Many diagnostic and analytical instruments are owned and operated by individual organisations and laboratories; data generation is de-centralised. The functional units in MB are often smaller teams of researchers where the contributions of individual researchers are more transparent. Given the high status of much MB research, this fragmented structure can lead to competitive dynamics across research teams that inhibit data sharing. Additionally, a critical difference from HEP is that any decision to disclose MB data to a centrally governed information infrastructure is discretionary and most often occurs after the data genesis.
The appropriate governance of this subtle yet fundamental difference (mandatory data governance at data genesis, or discretionary data governance after data genesis) is supported by the time delays and modularity that are adequately adjustable to accommodate the divergent incentives and objectives of the researchers, institutions, and funders.
5.1. Implications for policy and practice
Our study reveals a complex interpretation of where a community’s norms mesh with individual incentives to share data for the collective benefit. The research community by and large is optimistic about the scientific benefits of data sharing. From our data, we find that 74% of researchers say that having access to other data would benefit them. There is a consensus that scientific data should be a public good: replicability and transparency are beneficial to science; FAIR data practices are desirable in principle; cooperation makes science more efficient and reduces scientific fraud. However, a closer examination of what scientists proclaim ideally, versus what they actually practice, reveals a more ambiguous situation. Scientists need assurance of recognition of their work, and private entities that fund research desire reasonable safeguards for a fair return on their financial investments. This implies that policies seeking to effectively boost scientific data sharing should aim to be tailored to meet the needs of discipline-specific practices and norms at both individual and institutional levels.
Accordingly, beyond the mechanisms identified in this study (data modularity, time delay, and boundary organisations), funding institutions and policymakers can consider additional levers that allow scientists to receive commensurate rewards for data cultivation and publication. Fundamentally, this means elevating the status of data curation from a necessary input to the scientific process, to a valid, high-status outcome in its own right. Inspiration can be found in the practices of patenting and software licencing which ensure that inventors share their knowledge in exchange for various rights. This implies that public agencies, academic institutions, and other arbiters of scientific merit, award data curation and publication greater status in funding decisions, recruitment and promotion processes, or other professional accolades. Such structural changes could potentially have shorter-term effects in the individual cost-benefit calculus of collective action (Fulk et al. Citation2004; Hardin Citation1982; Ostrom Citation1990; Vitali, Mathiassen, and Rai Citation2018), as well as longer-term effects in the evolution of the epistemic cultures (Borgman Citation2012; Knorr Cetina Citation1999, Citation2007; Gläser et al. Citation2015).
It is also important to highlight that there are additional considerations that determine data sharing attitudes and behaviours across disciplines. Scientists are often legitimately concerned with the potential misuse or erroneous interpretations of their results. The recent Covid-19 pandemic evidences the fact that data publication is not an end in itself, but must be tempered with qualified interpretation to inform public health policy appropriately. By extension, FAIR data practices entail costs of documenting metadata and scientific procedures in a manner facilitate appropriate interpretation and communication, which, for fields such as public health or environmental policy, are increasingly vital.
Many of these additional ‘costs’ (arbitration, interpretation, communication) are currently assumed by scientific organisations such as CERN, NASA, CDC, EMBL, etc. As such, our study informs the potential efforts of other scientific communities currently less reliant on the cultivation of large data quantities, but increasingly so. In addition to appropriate application of modularity, time delay, and boundary organisations, information infrastructures can be designed with complementary mechanisms that foresee data sharing implications of beyond immediate scientific communities, but policy makers and the public at large.
6. Limitations and future research
Our findings are subject to limitations that warrant further investigation. Although the sample sizes in our survey were large, given the relatively short interval between 2016 and 2018, this sampling is likely insufficient to detect long-term patterns of data sharing behaviour. Additional surveys in the coming years can enrich our current data to uncover confounding relationships in scientists’ willingness to share data. Research that purposefully examines heterogeneity in data sharing practices across disciplines can benefit from in-depth comparisons of high- and low- intensity data sharing communities. Furthermore, while HEP and MB represent disciplines that are very capital-intensive, a research design focusing on scientific contexts with different economic dynamics would be useful for extending our understanding of data sharing practices.
By using random selection methods to identify respondents representative of the researcher population at large, our survey sought to mitigate selection bias. However, we acknowledge a potential bias of researchers: those more inclined towards sharing scientific data could have a greater propensity to respond to the survey invitation. In addition, while the sponsorship of a major publisher in the survey might have also influenced survey response bias, we estimate that the additional involvement of an academic consortium and the European Commission could have partially counterbalanced any respondent bias.
Finally, regarding the case study analysis, we acknowledge that the challenge of the case method is to generalise the findings. Nevertheless, it is worth mentioning that there is a trade-off between internal and external validity. Our results are deeply grounded in the studied contexts, and we employed established procedures in inductive research to maximise the internal validity of our results. Consequently, we should be prudent in extrapolating our results to other contexts and scientific communities that do not display the same institutional and economic characteristics. We encourage additional in-depth research across other epistemic cultures and academic disciplines to better inform our understanding of how data sharing can be governed.
7. Conclusion
Data sharing is a practice intended for the collective benefit of scientific progress. Yet, reasons for its gradual and disparate adoption are less obvious. Scientific communities are far from united and display heterogeneous practices and norms in the way science is produced and how merit and status are allocated. Consequently, a delicate system of mechanisms needs to be established to align individual and collective incentives. The use of modularity, time delay, and boundary organisations are pivotal in the information infrastructures created by the scientific disciplines currently at the forefront of scientific data sharing. Other academic communities that seek to follow these examples can apply these mechanisms in a manner consistent with their own epistemic cultures and professional practices.
Acknowledgments
This study was funded by the Open Science Monitor (2017- 2019), a service contract with European Commission- DG RTD (Contract number PP-05622-2017) and implemented in collaboration with Elsevier, ESADE, Leiden University, and Lisbon Council.
Disclosure statement
No potential conflict of interest was reported by the author(s).
Additional information
Funding
Notes
1 EMBL-EBI COVID-19 data portal: https://www.covid19dataportal.org/support-data-sharing-covid19
2 The term FAIR was launched in the Lorentz workshop in 2014. The resulting FAIR principles were published in 2016. See https://www.go-fair.org/fair-principles/
3 re3data.org
4 Open Science Monitor: https://ec.europa.eu/info/research-and-innovation/strategy/goals-research-and-innovation-policy/open-science/open-science-monitor_en
5 Large Hadron Collider (LHC) data policy: https://twiki.cern.ch/twiki/pub/LHCb/LHCbDataPreservation/130321-LHCbDataAccessPolicy.pdf Retrieved 20th October 2021:
References
- https://en.unesco.org/sites/default/files/tab_usr15_s7_researchers_field_science_2013_en.pdf. Accessed January 2022
- Aad, G., B. Abbott, J. Abdallah, R. Aben, M. Abolins, O. S. AbouZeid, and Z. Barnovska. 2015. “Combined Measurement of the Higgs Boson Mass in P P Collisions at S= 7 and 8 TeV with the ATLAS and CMS Experiments.” Physical Review Letters, 114 (19), p.191803.Q.
- Atkins, D. E., K. K. Droegemeier, S. I. Feldman, H. Garcia-Molina, M. L. Klein, D. G. Messerschmitt, P. Messina, J. P. Ostriker, and M. H. Wright. 2003. “Revolutionizing Science and Engineering through Cyberinfrastructure.” National Science Foundation, Accessed January 2022http://www.nsf.gov/od/oci/reports/atkins.pdf
- Baker, M. 2015. “First Results from Psychology’s Largest Reproducibility Test.” Nature News.
- Bhardwaj, A., S. Bhattacharjee, A. Chavan, A. Deshpande, A. J. Elmore, S. Madden, and A. G. Parameswaran. 2014. “DataHub: Collaborative Data Science & Dataset Version Management at Scale.” ArXiv:1409.0798 [Cs].
- Blumenthal, D., E. G. Campbell, N. Causino, and K. S. Louis. 1996. “Participation of Life-Science Faculty in Research Relationships with Industry.” New England Journal of Medicine 335 (23): 1734–1739. doi:https://doi.org/10.1056/NEJM199612053352305.
- Boland, R. J., and R. V. Tenkasi. 1995. “Perspective Making and Perspective Taking in Communities of Knowing.” Organization Science 6 (4): 350–372. doi:https://doi.org/10.1287/orsc.6.4.350.
- Borgman, C. L. 2010. Scholarship in the Digital Age: Information, Infrastructure, and the Internet. Cambridge, MA: MIT Press.
- Borgman, C. L. 2012. “The Conundrum of Sharing Research Data.” Journal of the American Society for Information Science and Technology 63 (6): 1059–1078. doi:https://doi.org/10.1002/asi.22634.
- Borgman, C. L. 2015. Big Data, Little Data, No Data: Scholarship in the Networked World. Cambridge United States: MIT Press.
- Bowker, G. C., and S. Leigh Star. 1999. Sorting Things Out: Classification and Its Consequences. Cambridge, MA: MIT Press.
- Cain, C. 2012. “A Mind for Precompetitive Collaboration.” Science-Business eXchange 5 (19): 483. doi:https://doi.org/10.1038/scibx.2012.483.
- Campbell, E. G., and E. Bendavid. 2002. “Data-Sharing and Data-Withholding in Genetics and the Life Sciences: Results of a National Survey of Technology Transfer Officers.” Journal of Health Care Law & Policy 6: 241.
- Carillo, M. R., and E. Papagni. 2014. “‘Little Science’ and ‘Big Science’: The Institution of ‘Open Science’ as a Cause of Scientific and Economic Inequalities among Countries.” Economic Modelling 43: 42–56. doi:https://doi.org/10.1016/j.econmod.2014.06.021.
- Chaguturu, R., F. Murad, and F. Murad. 2014. Collaborative Innovation in Drug Discovery: Strategies for Public and Private Partnerships. Somerset, NJ: John Wiley & Sons.
- Chen, X., S. Dallmeier-Tiessen, R. Dasler, S. Feger, P. Fokianos, J. B. Gonzalez, H. Hirvonsalo, et al. 2018. “Open Is Not Enough.” Nature Physics 15 (2): 113–119. doi:https://doi.org/10.1038/s41567-018-0342-2.
- Constantinides, P. 2012. Perspectives and Implications for the Development of Information Infrastructures. Hershey PA: IGI Global.
- Constantinides, P., and M. Barrett. 2015. “Information Infrastructure Development and Governance as Collective Action.” Information Systems Research 26 (1): 40–56. doi:https://doi.org/10.1287/isre.2014.0542.
- Consultative Committee for Space Data Systems. 2012. “Reference Model for an Open Archival Information System (OAIS). Recommendation for Space Data System Standards.” Accessed January 1 2022. http://public.ccsds.org/publications/RefModel.aspx
- Contreras, J. L. 2010. “Prepublication Data Release, Latency, and Genome Commons.” Science 329 (5990): 393–394. doi:https://doi.org/10.1126/science.1189253.
- Contreras, J. L., and L. S. Vertinsky. 2016. “Pre-Competition.” North Carolina Law Review 95 (1): 67–132.
- Cragin, M. H., C. L. Palmer, J. R. Carlson, and M. Witt. 2010. “Data Sharing, Small Science and Institutional Repositories.” Philosophical Transactions of the Royal Society A: Mathematical, Physical and Engineering Sciences 368 (1926): 4023–4038. doi:https://doi.org/10.1098/rsta.2010.0165.
- Creswell, J. W. 2018. Designing and Conducting Mixed Methods Research. 3rd ed. Thousand Oaks, CA: SAGE.
- Dasgupta, P., and P. A. David. 1987. “Information Disclosure and the Economics of Science and Technology.” In: Feiwel G.R. (eds), Arrow and the Ascent of Modern Economic Theory, 519–542. London: Palgrave Macmillan. https://doi.org/https://doi.org/10.1007/978-1-349-07239-2_16. .
- Dasgupta, P., and P. A. David. 1994. “Toward a New Economics of Science. 1994.” Research Policy 23 (5): 487–521. doi:https://doi.org/10.1016/0048-7333(94)01002-1.
- Dinno, A. 2015. “Nonparametric Pairwise Multiple Comparisons in Independent Groups Using Dunn’s Test.” The Stata Journal: Promoting Communications on Statistics and Stata 15 (1): 292–300. doi:https://doi.org/10.1177/1536867X1501500117.
- Dougherty, D. 1992. “Interpretive Barriers to Successful Product Innovation in Large Firms.” Organization Science 3 (2): 179–202. doi:https://doi.org/10.1287/orsc.3.2.179.
- Dphep Study Group. 2009. Data Preservation in High Energy Physics . ArXiv:0912.0255 [Hep-Ex, Physics: Physics]. Accessed January 2022 https://arxiv.org/pdf/0912.0255.pdf
- Dunn, O. J. 1961. “Multiple Comparisons Among Means.” Journal of the American Statistical Association 56 (293): 52. doi:https://doi.org/10.1080/01621459.1961.10482090.
- Edwards, P. N. 2010. A Vast Machine: Computer Models, Climate Data, and the Politics of Global Warming. Cambridge: MIT Press.
- Edwards, P. N. 2019. “Knowledge Infrastructures under Siege: Climate Data as Memory, Truce, and Target.” In Data Politics: Worlds, Subjects, Rights, edited by D. Bigo, E. Isin, and E. Ruppert, 21–42. New York: Routledge.
- Edwards, P. N., G. C. Bowker, S. J. Jackson, and R. Williams. 2009. “Introduction: An Agenda for Infrastructure Studies.” Journal of the Association for Information Systems 10 (5): 6. doi:https://doi.org/10.17705/1jais.00200.
- Edwards, P. N., M. S. Mayernik, A. L. Batcheller, G. C. Bowker, and C. L. Borgman. 2011. “Science Friction: Data, Metadata, and Collaboration.” Social Studies of Science 41 (5): 667–690. doi:https://doi.org/10.1177/0306312711413314.
- EIROforum IT working group. 2013. “E-Infrastructure for the 21st Century.” Zenodo, November 8.
- European Commission. 2014. “Data Management - H2020 Online Manual.” accessed March 10 2020. https://ec.europa.eu/research/participants/docs/h2020-funding-guide/cross-cutting-issues/open-access-data-management/data-management_en.htm
- European Commission. 2019. “Cost-Benefit Analysis for FAIR Research Data: Cost of Not Having FAIR Research Data.“ Accessed January 2022. https://op.europa.eu:443/en/publication-detail/-/publication/d375368c-1a0a-11e9-8d04-01aa75ed71a1.
- Fecher, B., S. Friesike, M. Hebing, and R. S. Phillips. 2015. “What Drives Academic Data Sharing?” PLoS ONE 10 (2): 25. doi:https://doi.org/10.1371/journal.pone.0118053.
- Fegan, G., & Cheah, P. Y. (2021). Solutions to COVID-19 data sharing. The Lancet Digital Health, 3(1), e6.
- Fletcher, J. A., and M. Zwick. 2000. “Simpson’s Paradox Can Emerge from the N-Player Prisoner’s Dilemma: Implications for the Evolution of Altruistic Behavior.” Proceedings of The World Congress of the Systems Sciences and ISSS 2000, Toronto, Canada. Accessed January 2022 www.sysc.pdx.edu/download/papers/isss_fl_zw.pdf
- Flora, D. B., and P. J. Curran. 2004. “An Empirical Evaluation of Alternative Methods of Estimation for Confirmatory Factor Analysis with Ordinal Data.” Psychological Methods 9 (4): 466–491. doi:https://doi.org/10.1037/1082-989X.9.4.466.
- Friesike, S., B. Widenmayer, O. Gassmann, and T. Schildhauer. 2015. “Opening Science: Towards an Agenda of Open Science in Academia and Industry.” The Journal of Technology Transfer 40 (4): 581–601. doi:https://doi.org/10.1007/s10961-014-9375-6.
- Fulk, J., R. Heino, A. J. Flanagin, P. R. Monge, and F. Bar. 2004. “A Test of the Individual Action Model for Organizational Information Commons.” Organization Science 15 (5): 569–585. doi:https://doi.org/10.1287/orsc.1040.0081.
- Gläser, J., J. Bielick, R. Jungmann, G. Laudel, E. Lettkemann, G. Petschick, and U. Tschida. 2015. “Research Cultures as an Explanatory Factor.” Österreichische Zeitschrift Für Soziologie 40 (3): 327–346. doi:https://doi.org/10.1007/s11614-015-0177-3.
- Greco, G. M., and L. Floridi. 2004. “The Tragedy of the Digital Commons.” Ethics and Information Technology 6 (2): 73–81. doi:https://doi.org/10.1007/s10676-004-2895-2.
- Grossman, R. L., A. Heath, M. Murphy, M. Patterson, and W. Wells. 2016. “A Case for Data Commons: Toward Data Science as A Service.” Computing in Science & Engineering 18 (5): 10–20. doi:https://doi.org/10.1109/MCSE.2016.92.
- Haas, P. M. 1992. “Introduction: Epistemic Communities and International Policy Coordination.” International Organization 46 (1): 1–35. doi:https://doi.org/10.1017/S0020818300001442.
- Haeussler, C., L. Jiang, J. Thursby, and M. Thursby. 2014. “Specific and General Information Sharing among Competing Academic Researchers.” Research Policy 43 (3): 465–475. doi:https://doi.org/10.1016/j.respol.2013.08.017.
- Hagstrom, W. O. 1974. “Competition in Science.” American Sociological Review 39 (1): 1–18. doi:https://doi.org/10.2307/2094272.
- Hardin, G. 1968. “”The Tragedy of the Commons.” Science 162 (3859): 1243–1248. doi:https://doi.org/10.1126/science.162.3859.1243.
- Hardin, G. 1982. Collective Action. Baltimore, MD: Johns Hopkins University Press.
- Harley, D., S. K. Acord, and S. Earl-Novell. 2010. “Peer Review in Academic Promotion and Publishing: Its Meaning, Locus, and Future.” Center for Studies in Higher Education. Accessed January 2022 https://eric.ed.gov/?id=ED512030
- Hess, C., and E. Ostrom. 2003. “Ideas, Artifacts, and Facilities: Information as a Common-Pool Resource.” Law and Contemporary Problems 66 (1/2): 111–145.
- Hey, T. 2009. The Fourth Paradigm: Data-intensive Scientific Discovery. Redmond, WA: Microsoft Press.
- Hochachka, W. M., D. Fink, R. A. Hutchinson, D. Sheldon, W.-K. Wong, and S. Kelling. 2012. “Data-Intensive Science Applied to Broad-Scale Citizen Science.” Trends in Ecology & Evolution 27 (2): 130–137. doi:https://doi.org/10.1016/j.tree.2011.11.006.
- Holm, S. 1979. “A Simple Sequentially Rejective Multiple Test Procedure.” Scandinavian Journal of Statistics 6 (2): 65–70.
- Holzner, A., P. Igo-Kemenes, and S. Mele. 2009. “First Results from the PARSE.Insight Project: HEP Survey on Data Preservation, Re-Use and (Open) Access.” ArXiv:0906.0485 [Hep-Ex, Physics: Physics]. Accessed January 2022 http://arxiv.org/abs/0906.0485
- Howison, J., E. Deelman, M. J. McLennan, R. Ferreira da Silva, and J. D. Herbsleb. 2015. “Understanding the Scientific Software Ecosystem and Its Impact: Current and Future Measures.” Research Evaluation 24 (4): 454–470. doi:https://doi.org/10.1093/reseval/rvv014.
- Järvenpää, S. L., and M. L. Markus. 2018. “Data Perspective in Digital Platforms: Three Tales of Genetic Platforms.” Proceedings of the 51st Hawaii International Conference on System Sciences, Hawaii.
- Kahn, R. E., and V. G. Cerf. 1988. “An Open Architecture for a Digital Library System and a Plan for Its Development.” In The Digital Library Project Vol. 1: The World of Knowbots. Reston, VA: Corporation for National Research Initiatives. Accessed January 2022 https://citeseerx.ist.psu.edu/viewdoc/download?doi=10.1.1.475.2209&rep=rep1&type=pdf.
- Kallinikos, J., and J. D. Constantiou. 2015. “Big Data Revisited: A Rejoinder.” Journal of Information Technology 30 (1): 70–74. doi:https://doi.org/10.1057/jit.2014.36.
- Kaplan, F. 2015. “A Map for Big Data Research in Digital Humanities.” Frontiers in Digital Humanities 1 (7): 1.
- Kellogg, K. C., W. J. Orlikowski, and J. Yates. 2006. “Life in the Trading Zone: Structuring Coordination across Boundaries in Postbureaucratic Organizations.” Organization Science 17 (1): 22–44. doi:https://doi.org/10.1287/orsc.1050.0157.
- King, G. 2007. “An Introduction to the Dataverse Network as an Infrastructure for Data Sharing.” Sociological Methods & Research 36 (2): 173–199. doi:https://doi.org/10.1177/0049124107306660.
- Knorr Cetina, K. 1999. Epistemic Cultures: How the Sciences Make Knowledge. Cambridge, Mass: Harvard University Press.
- Knorr Cetina, K. K. 2007. “Culture in Global Knowledge Societies: Knowledge Cultures and Epistemic Cultures.” Interdisciplinary Science Reviews 32 (4): 361–375. doi:https://doi.org/10.1179/030801807X163571.
- Kruskal, W. H., and W. Allen Wallis. 1952. “Use of Ranks in One-Criterion Variance Analysis.” Journal of the American Statistical Association 47 (260): 583–621. doi:https://doi.org/10.1080/01621459.1952.10483441.
- Kuhn, T. S. 1962. The Structure of Scientific Revolutions. Chicago: University of Chicago Press, 176–177.
- Kupferschmidt, K. 2018. “Researcher at the Center of an Epic Fraud Remains an Enigma to Those Who Exposed Him.” Science. Accessed January 2022 https://www.sciencemag.org/news/2018/08/researcher-center-epic-fraud-remains-enigma-those-who-exposed-him.
- Lazer, E. 2009. Resurrecting Pompeii. London: Routledge.
- Lecarpentier, D., P. Wittenburg, W. Elbers, A. Michelini, R. Kanso, P. Coveney, and R. Baxter. 2013. “EUDAT: A New Cross-Disciplinary Data Infrastructure for Science.” International Journal of Digital Curation 8 (1): 279–287. doi:https://doi.org/10.2218/ijdc.v8i1.260.
- Lee, M., E. Almirall, and J. Wareham. 2016. “Open Data and Civic Apps: First-Generation Failures, Second-Generation Improvements.” Communications of the ACM 59 (1): 82–89. doi:https://doi.org/10.1145/2756542.
- Leonelli, S. 2012. “Introduction: Making Sense of Data-Driven Research in the Biological and Biomedical Sciences.” Studies in History and Philosophy of Biological and Biomedical Sciences 43 (1): 1–3. doi:https://doi.org/10.1016/j.shpsc.2011.10.001.
- Lyon, L. 2016. “Transparency: The Emerging Third Dimension of Open Science and Open Data.” LIBER Quarterly 25 (4): 153–171.
- McKight, P. E., and J. Najab. 2010. “Kruskal-Wallis Test.” The Corsini Encyclopedia of Psychology. Wiley Online Library: John Wiley & Sons, Ltd. p.1-1
- Meijer, I., S. Berghmans, H. Cousijn, C. Tatum, G. Deakin, A. Plume, and A. Rushforth, et al. 2017. Open Data: The Researcher Perspective. Netherlands: University of Leiden.
- Merton, R. K. 1973. The Sociology of Science: Theoretical and Empirical Investigations. Chicago: University of Chicago Press.
- Miles, M. B., and A. M. Huberman. 1994. Qualitative Data Analysis: An Expanded Sourcebook. Thousand Oaks, CA: Sage.
- Mîndrilă, D. 2010. “Maximum Likelihood (ML) and Diagonally Weighted Least Squares (DWLS) Estimation Procedures: A Comparison of Estimation Bias with Ordinal and Multivariate Non-Normal Data.” International Journal for Digital Society 1 (1): 60–66. doi:https://doi.org/10.20533/ijds.2040.2570.2010.0010.
- Mittleman, B., G. Neil, and J. Cutcher-Gershenfeld. 2013. “Precompetitive Consortia in Biomedicine—How are We Doing?” Nature Biotechnology 31 (11): 979–985. doi:https://doi.org/10.1038/nbt.2731.
- Monge, P. R., J. Fulk, M. E. Kalman, A. J. Flanagin, C. Parnassa, and S. Rumsey. 1998. “Production of Collective Action in Alliance-Based Interorganizational Communication and Information Systems.” Organization Science 9 (3): 411–433. doi:https://doi.org/10.1287/orsc.9.3.411.
- Mørk, B. E., M. Aanestad, O. Hanseth, and M. Grisot. 2008. “Conflicting Epistemic Cultures and Obstacles for Learning across Communities of Practice.” Knowledge and Process Management 15 (1): 12–23. doi:https://doi.org/10.1002/kpm.295.
- Mukherjee, A., and S. Stern. 2009. “Disclosure or Secrecy? The Dynamics of Open Science.” International Journal of Industrial Organization 27 (3): 449–462. doi:https://doi.org/10.1016/j.ijindorg.2008.11.005.
- Murray, F., and S. O’Mahony. 2007. “Exploring the Foundations of Cumulative Innovation: Implications for Organization Science.” Organization Science 18 (6): 1006–1021. doi:https://doi.org/10.1287/orsc.1070.0325.
- National Science Board. 2005. “Long-Lived Digital Data Collections Enabling Research and Education in the 21st Century.” Accessed January 2022 https://apps.dtic.mil/sti/citations/ADA444393
- NIH. 2003. “NIH Data Sharing Policy and Implementation Guidance.”accessed March 10 2020. https://grants.nih.gov/grants/policy/data_sharing/data_sharing_guidance.htm
- O’Mahony, S., and B. A. Bechky. 2008. “Boundary Organizations: Enabling Collaboration Among Unexpected Allies.” Administrative Science Quarterly 53 (3): 422–459. doi:https://doi.org/10.2189/asqu.53.3.422.
- OECD. 2015. “Making Open Science a Reality.” No. 25 OECD Science, Technology and Industry Policy Papers, Paris: OECD Publishing. Accessed January 2022 https://wiki.lib.sun.ac.za/images/0/02/Open-science-oecd.pdf
- Olson, M. 1965. “The Logic of Collective Action: Public Goods and the Theory of Groups.” In Harvard Economic Studies, 124. Cambridge: Harvard University Press, p.208.
- Open Targets. 2020. “Open Targets Platform: Release 20.02 Is Out.” Accessed January 2022 http://blog.opentargets.org/2020/03/02/open-targets-platform-release-20-02-is-out/
- OpenAire. 2019. “RDM Costs.” OpenAIRE, accessed March 10 2020. https://www.openaire.eu/how-to-comply-to-h2020-mandates-rdm-costs
- Ostrom, E. 1986. “An Agenda for the Study of Institutions.” Public Choice 48 (1): 3–25. doi:https://doi.org/10.1007/BF00239556.
- Ostrom, E. 1990. Governing the Commons: The Evolution of Institutions for Collective Action. Cambridge: Cambridge University Press.
- Pasquetto, I. V., B. M. Randles, and C. L. Borgman. 2017. “On the Reuse of Scientific Data.” Data Science Journal 16: 8. doi:https://doi.org/10.5334/dsj-2017-008.
- Perkmann, M., and H. Schildt. 2015. “Open Data Partnerships between Firms and Universities: The Role of Boundary Organizations.” Research Policy 44 (5): 1133–1143. doi:https://doi.org/10.1016/j.respol.2014.12.006.
- Peters, I., P. Kraker, E. Lex, C. Gumpenberger, and J. I. Gorraiz. 2017. “Zenodo in the Spotlight of Traditional and New Metrics.” Frontiers in Research Metrics and Analytics 2: 13. doi:https://doi.org/10.3389/frma.2017.00013.
- Piwowar, H. A., T. J. Vision, and M. C. Whitlock. 2011. “Data Archiving Is a Good Investment.” Nature 473 (7347): 285. doi:https://doi.org/10.1038/473285a.
- Plantin, J.-C., C. Lagoze, P. N. Edwards, and C. Sandvig. 2018. “Infrastructure Studies Meet Platform Studies in the Age of Google and Facebook.” New Media & Society 20 (1): 293–310. doi:https://doi.org/10.1177/1461444816661553.
- Pujol Priego, L., and J. Wareham. 2018. Open Targets: Open Science Monitor Case Study. European Commission, Directorate-General for Research and Innovation, Publications Office, 2019. http://publications.europa.eu/publication/manifestation_identifier/PUB_KI0518020ENN.
- Pujol Priego, L., and J. Wareham. 2019. REANA: Reproducible Research Data Analysis Platform: Open Science Monitor Case Study. European Commission, Directorate-General for Research and Innovation, Publications Office, 2019. http://publications.europa.eu/publication/manifestation_identifier/PUB_KI0219176ENN.
- Ribes, D., and C. P. Lee. 2010. “Sociotechnical Studies of Cyberinfrastructure and E-Research: Current Themes and Future Trajectories.” Computer Supported Cooperative Work (CSCW) 19 (3–4): 231–244. doi:https://doi.org/10.1007/s10606-010-9120-0.
- Romasanta, A., and J. Wareham. 2021. “Science Mesh: Enabling Seamless Research Collaborations through a Federated Cloud Infrastructure.” ECIS 2021 - 29th European Conference on Information Systems, Marrakech, Morocco.
- Romasanta, A. K., J. Wareham, L. Pujol Priego, P. Garcia Tello, and M. Nordberg. 2021. “Risky Business: How to Capitalize on the Success of Big Science.” Issues in Science and Technology, 23 July 2021 https://issues.org/risky-business-big-science-deep-tech-transfer-commercialization/.
- Rosseel, Y. 2012. “Lavaan: An R Package for Structural Equation Modeling.” Journal of Statistical Software 48 (2). doi:https://doi.org/10.18637/jss.v048.i02.
- Royal Society. 2012. “Science as an Open Enterprise.” Policy Unit, United Kingdom. Accessed January 2022 https://royalsociety.org/~/media/Royal_Society_Content/policy/projects/sape/2012-06-20-SAOE.pdf
- Simko, T., K. Cranmer, M. R. Crusoe, L. Heinrich, A. Khodak, D. Kousidis, and D. Rodriguez 2018. “Search for Computational Workflow Synergies in Reproducible Research Data Analyses in Particle Physics and Life Sciences.” 2018 IEEE 14th International Conference on E-Science ( e-Science), Amsterdam.
- Stephan, P. E. 1996. “The Economics of Science.” Journal of Economic Literature 34 (3): 1199–1235.
- Tenopir, C., E. D. Dalton, S. Allard, M. Frame, I. Pjesivac, B. Birch, D. Pollock, K. Dorsett, and P. van den Besselaar. 2015. “Changes in Data Sharing and Data Reuse Practices and Perceptions among Scientists Worldwide.” PLoS ONE 10 (8): e0134826. doi:https://doi.org/10.1371/journal.pone.0134826.
- Thelwall, M., and K. Kousha. 2016. “Figshare: A Universal Repository for Academic Resource Sharing?” Online Information Review 40 (3): 333–346. doi:https://doi.org/10.1108/OIR-06-2015-0190.
- Vamathevan, J., D. Clark, P. Czodrowski, I. Dunham, E. Ferran, G. Lee, B. Li, et al. 2019. “Applications of Machine Learning in Drug Discovery and Development.” Nature Reviews Drug Discovery 18 (6): 463–477. doi:https://doi.org/10.1038/s41573-019-0024-5.
- Vassilakopoulou, P., S. Espen, and M. Aanestad. 2016. “A Commons Perspective on Genetic Data Governance: The Case of BRCA Data.” Research Papers, Paper 136. Accessed January 2022 http://aisel.aisnet.org/ecis2016_rp/136
- Venkatesh, V., S. A. Brown, and H. Bala. 2013. “Bridging the Qualitative-Quantitative Divide: Guidelines for Conducting Mixed Methods Research in Information Systems.” MIS Quarterly 37 (1): 21–54. doi:https://doi.org/10.25300/MISQ/2013/37.1.02.
- Vertinsky, L. S. 2014. “Patents, Partnerships, and the Pre-Competitive Collaboration Myth in Pharmaceutical Innovation.” U.C. Davis Law Review 48 (4): 1509–1580.
- Vitali, M., L. Mathiassen, and A. Rai. 2018. “The Sustainability of Polycentric Information Commons.” MIS Quarterly 42 (2): 607–631. doi:https://doi.org/10.25300/MISQ/2018/14015.
- Wallis, J. C., E. Rolando, C. L. Borgman, and L. A. Nunes Amaral. 2013. “If We Share Data, Will Anyone Use Them? Data Sharing and Reuse in the Long Tail of Science and Technology.” PLoS ONE 8 (7): e67332. doi:https://doi.org/10.1371/journal.pone.0067332.
- Wareham, J., L. Pujol Priego, A. K. Romasanta, T. Wareham Mathiassen, M. Nordberg, and P. Garcia Tello. 2021. “Systematising Serendipity for Big Science Infrastructures: The ATTRACT Project.” Technovation 102374. Forthcoming. doi:https://doi.org/10.1016/j.technovation.2021.102374.
- Weill, P., and J. W. Ross 2004. “IT Governance on One Page,” SSRN Scholarly Paper No. ID, 664612.
- Weinberg, A. M. 1961. “Impact of Large-Scale Science in the United States.” Science 134 (3473): 161–164. doi:https://doi.org/10.1126/science.134.3473.161.
- Whyte, A., and G. Pryor. 2011. “Open Science in Practice: Researcher Perspectives and Participation.” International Journal of Digital Curation 6 (1): 199–213. doi:https://doi.org/10.2218/ijdc.v6i1.182.
- Wicherts, J. M., M. Bakker, D. Molenaar, and R. E. Tractenberg. 2011. “Willingness to Share Research Data Is Related to the Strength of the Evidence and the Quality of Reporting of Statistical Results.” PLoS ONE 6 (11): e26828. doi:https://doi.org/10.1371/journal.pone.0026828.
- Wilkinson, M. D., M. Dumontier, I. J. Aalbersberg, G. Appleton, M. Axton, A. Baak, N. Blomberg, et al. 2016. “The FAIR Guiding Principles for Scientific Data Management and Stewardship.” Scientific Data 3 (1): 160018. doi:https://doi.org/10.1038/sdata.2016.18.