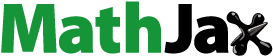
ABSTRACT
Software is at the core of digitalisation and is often claimed to play a central role in innovation and in shaping competition across industries and firms. There are yet few studies of the extent and nature of software development across firms. We employ a unique firm-level survey comprising 3,929 firms across Sweden to analyse the distribution and characteristics of firms that invest in software development and the orientation of their investments. The results confirm that software development activities are present in most industries, but heterogeneously distributed across firms. Internal software development is associated with innovation-oriented large firms in high-tech and knowledge-intensive industries, and is often affiliated with MNEs. The results suggest that software development is comparable to R&D investments and constitutes an example of digital innovation. This strengthens the value of studying software development activities to understand how firms invest in and build competitive advantage in the digitalised economy.
1. Introduction
In 2011, Netscape founder and Silicon Valley profile Marc Andreessen coined the phrase ‘software is eating the world’ (Andreessen Citation2011). He was describing how traditional business models, such as that of physical bookstores, were increasingly being challenged by software-based businesses like Amazon. Software-based businesses allowed customers not only to do their shopping online but also to easily browse and be recommended a much larger variety of titles than would have been possible walking from shelf to shelf in a physical shop.
Andreessen’s main point, that business models using the software would become commonplace and outcompete those that do not, has indeed been vindicated and lies at the core of the ongoing digitalisation. The development has been compared to a new industrial revolution (Schwab Citation2017). Intangible assets – mostly software-dependent – are growing in importance and outweigh the value of physical capital in a growing number of businesses (Haskel and Westlake Citation2018). Corrado et al. (Citation2009) estimate that investments in intangibles amount to 800 billion USD in omitted contributions to measures of US GDP as of 2003 and show that adding this to capital in growth statistics results in increased growth measured as output per worker stemming largely from capital deepening.
Several studies point to a software-biased shift in innovation in the sense that innovation is increasingly associated with technological intangibles, in particular software development (Evans and Schmalensee Citation2016; Branstetter, Drev, and Kwon Citation2019). Andersson, Kusetogullari, and Wernberg (Citation2021) study Swedish firms and find that those who engage in software development are more likely to introduce new innovations and report a higher degree of innovation-related sales than other firms in the manufacturing and service industries. Software is also at the very foundation of technologies like big data analytics, machine learning, cyber security, and cloud computing, to mention a few.
Taken together, this speaks to the increasingly central role software and software development play both in innovation and shaping competition across industries. Yet, as numerous studies of the diffusion of technology and innovation throughout history have shown (Griliches Citation1957; Utterback Citation1974; Pavitt Citation1984; Geroski Citation2000; Hall Citation2004), a shift towards an increasing role of software development is likely to neither be instant nor evenly distributed across types of firms and industries. This raises the question of what characterises the firms that not only use but invest in developing their own software.
Formulating a theoretical backdrop to the distribution of software development in the economy, we take stock of two key aspects: 1) digital technologies are general-purpose technologies, 2) neither software nor the digital infrastructure on which it depends are new to the economy.
There is a wide variety of theoretical approaches to the diffusion of new technologies and innovation. While early neoclassical models built on the assumption that new technologies and innovations spread instantly across the economy, later contributions provide a more nuanced perspective on how spatial as well as technological distance, sectoral differences, and firm-level capabilities influence the diffusion of new technologies and innovations (Pavitt Citation1984, Cohen and Levinthal Citation1990; Agarwal and Gort Citation2002; Ahmad Citation2003; Cantwell and Iammarino Citation2005; Frenz and Ietto‐Gillies Citation2007; Andreessen Citation2011; Haskel and Westlake Citation2018; Andersson, Kusetogullari, and Wernberg Citation2021).
One important aspect is that new technologies are far from homogeneous with regards to the manner in which they spread and diffuse in the economy, not least because their scope of application varies. Some technologies affect specific sectors while others have more profound effects on the entire economy (Nelson and Winter Citation1982). The latter tend to be technologies that can be adapted and employed for a variety of purposes – they are general-purpose technologies (GPTs) like the steam engine or electricity (Bresnahan and Trajtenberg Citation1995). GPTs do not only add to existing production, but also change the conditions for production across sectors, resulting in the disruption of business models and long-term structural change. Arthur (Citation2009) distinguishes between technologies that add to an existing system (structural deepening) and technologies that disrupt and replace parts of that system (domain shifting). Digitalisation constitutes an economy-wide domain shift and software is what makes digital computers a general-purpose technology in the first place.
This holds several important implications for how we understand the current distribution of software development in the economy. First, software development should not be expected to be limited to a specific sector of the economy. Digitalisation emerged with the growth of the IT sector but is no longer limited to it. Software development is not a new activity as such, but with the advancement of digital transformation, it is spreading and becoming increasingly heterogeneous precisely because it spreads and spans across sectors. Digitalisation comprises not only technology adoption but also the search, adaptation and complementary innovations required to draw productivity benefits from the new technologies (Brynjolfsson and Hitt Citation2000). Software development – programming digital technologies for a specific purpose – is coupled with complementary innovation aimed at leveraging technologies in different sectors under different conditions.
Furthermore, an overt focus on (static) sectoral boundaries may prove misleading when looking at digital transformation. Castellacci (Citation2008) has shown that the transformation to software-based business models has led firms to shift from manufacturing to service sectors (in terms of statistical categorisation). A recent example of this is the Swedish telecom company Ericsson, one of Sweden’s largest firms, which shifted from manufacturing to services in 2017. This speaks to the need for an economy-wide approach to the analysis of software development.
A second implication is that because it is a GPT, sector- and firm-level differences in diffusion are more likely to determine when than if firms adopt software-based technologies. In fact, most, if not all, parts of the economy already use software-based technologies to some degree. According to Statistics Sweden, 94 per cent of all firms with more than ten employees had a fixed broadband connection, and 91 per cent maintained a website in 2021. Twenty-four per cent reported taking purchase orders online in 2020. Thus, the distribution of software development is not likely to be solely determined by the diffusion and adoption of software-based technologies. In fact, we should probably not expect every firm to eventually engage in software development. Instead, we can think about the decision to invest in software development as a type of ‘make or buy’ decision intimately coupled with digital transformation – buying standardised software comes at a lower initial cost which may make it more easily available, but developing your own could potentially result in a unique competitive advantage, or failure.Footnote1 These are essentially two different approaches on digital transformation, and the latter is characterised by a higher degree of uncertainty and, arguably, risk-taking. Investments in software development may be motivated by both necessity (specific demands that are not met by standardised software or it is too expensive) and opportunity (potential for innovation), but these firms set themselves apart by not being subject to the same conditions – for better or for worse – provided by standardised software.
The growing ubiquity of software-based businesses in the economy as a whole is well-documented (e.g. Ensmenger Citation2012; Brynjolfsson and McAfee Citation2014; McAfee and Brynjolfsson Citation2017; Auerswald Citation2017), and there are numerous studies on how firms implement software-based tools in their organisations and processes (e.g. Quinn, Baruch, and Zien Citation1996; Yoo, Henfridsson, and Lyytinen Citation2010; Ensmenger Citation2012; Nambisan et al. Citation2017; Kim, Lee, and Gopal Citation2019; Niebel, Rasel, and Viete Citation2019). However, there is a lack of firm-level studies that explore the middle-ground between these two bodies of research, i.e. the distribution and orientation of software development in the economy as a whole and the characteristics of software-developing firms. Put differently, software-based business is increasingly pervasive in the economy and firms reorient their business to implement software-based solutions, but the understanding of which firms invest in software development, either to sell software to others or to develop a competitive advantage for other products and services they sell, is still very limited. Yet, these firms likely play a key role not only in their respective industries but also in the digital competitiveness of the economy.
This paper contributes to filling the gap between macro-level descriptions of digital transformation as a homogenous – albeit unevenly advanced – phenomenon and micro-level case studies of software development in individual firms. It is well-known from that software development has spread beyond the IT industry, but there is no comprehensive overview of how it is distributed across other parts of the economy or what characterises the firms across sectors that invest in developing their own software to a lesser or larger degree. Adding to this picture, we hope to contribute to a better understanding not only of decisions pertaining to firm-level digital transformation but also of the digital shift in the economy as a whole. We are not looking for a homogenous software sector, but for the heterogeneities of software development across sectors and the common characteristics that set software-developing firms apart from their competitors.
We employ unique firm-level survey data comprising 3,929 firms across the Swedish economy to analyse the distribution and characteristics of firms that invest in software development as well as the orientation of their investments. The analyses provide a snapshot of the distribution and direction of software development across firms in Sweden, i.e. an advanced and digitally mature OECD economy, and also provide an overview of investments in intangible assets related to software development.
Software is everywhere and software development is nothing new, but with the digital transition of the economy it is becoming a key instrument in the transformation of businesses to leverage digital technologies. Against this background, we propose that firms that engage in software development are in some respect at the forefront of the digital transformation in their respective industries. While there may be firms that pioneer the use of digital technologies and software-based business models without developing software, firms that do develop software are highly unlikely to be digital laggards because of the investment and risk-taking it requires. This warrants further investigation of these firms.
Firms develop software for different purposes. Some firms may use software development to produce new products or services, while others use software to increase the efficiency of their existing business. We take this into consideration by distinguishing between five primary orientations of software development: (1) as an independent service or product, (2) software developed to be embedded in a physical product, (3) for distribution or sales of a product or service, (4) for own internal operations and efficiency and (5) sales of software development services to others.
The results confirm that software development activities are present in most industries but heterogeneously distributed across firms and in orientation. Most of the results concerning the types of firms and their market contexts that invest in software development are in line with the notion that software development is comparable to R&D investments and constitutes an example of digital innovation. This in turn strengthens the value of studying software development activities to better understand how firms invest in and build competitive advantage in the digitalised economy.
2. General-purpose technologies, digitalisation and software development
2.1. A new general-purpose technology
Economic analysis has long considered technological change as a core driver of economic growth (Romer Citation1990; Solow Citation1957). At the same time, many standard economic models have been criticised on the grounds that they either assume that technologies arrive exogenously or that they are assumed to spread instantaneously, as if the economy was a ‘flat market’. However, technologies are different in terms of the pervasiveness of their diffusion as well as in terms of their impacts on different segments of the economy. To understand technology-driven economic change, one must account for its uneven and heterogeneous impact.
Inspired by the way economic historians highlight the key role played by specific technologies, such as the steam engine during the industrial revolution, Bresnahan and Trajtenberg (Citation1995) introduce the concept of general-purpose technologies (GPTs). GPTs are enabling technologies with considerable innovative complementarities, or to paraphrase a more recent attempt at a consensus definition, they are ‘pervasive, improving over time and able to spawn new innovations’ (Brynjolfsson and McAfee Citation2014, p. 76). In other words, a general-purpose technology provides a common technological base, upon which specific applications and innovations can be developed, diffused, or recombined across sectors or markets.
The ongoing digitalisation constitutes the introduction of a new general-purpose technology comparable to the steam engine and electricity before it (Castells Citation1996; Varian Citation2003; Lipsey, Carlaw, and Bekar Citation2005; Grajek Citation2012; Brynjolfsson and McAfee Citation2014; McAfee and Brynjolfsson Citation2017).
Digital technology deploys such architectural paradigms as Cyber-Physical Systems (CPS). As the internet has transformed the way how humans interact with each other, CPS transforms the way how humans interact with the physical world (Rajkumar et al. Citation2010). In CPS, information from the physical and cyberspace is closely monitored, coordinated and synchronised, which facilitates the creation of a hyperconnected world (Bordel et al. Citation2017; Monostori et al. Citation2016). The pervasiveness and combination of computational and physical spheres spawn new innovations in various areas such as robotics, automotive and healthcare systems. These systems become significant in various aspects of the industry, as virtual simulations and testing become more obtainable.
The interaction between the physical and digital dimensions is the core of CPS, where data analytics plays a key role. Being integrated into data analytics, software is used to process, filter and store data from the physical world and codify it for cyberspace.
CPS is one of the trends that transform many different industries as well as firms. It is used in intelligent houses, smart electricity networks and intelligent transport systems. The pervasiveness of digital transformation also drives the development of new ways for manufacturing firms to produce products. For instance, digitisation drives the development of ‘digital twins’ which provide the foundations for the product life-cycle management (Grieves and Vickers Citation2017). The concept of ‘digital twin’ can be defined as a virtual representation of a physical object that contains all information about its physical characteristics and the environment within which the physical product exists. Digital twins are beneficial for manufacturers as they open prospects for virtual simulation, modelling and evaluation of product performance (Grieves and Vickers Citation2017).
The integration between the cyber and physical settings on different scales creates multiple applications for firms to obtain a competitive advantage and exploit the full potential of digital transformation across industries.
Taken together, digitalisation combines (i) computational capacity that makes it possible to process anything that can be quantified (e.g. Arthur Citation2009), (ii) distributed networks that connect people, firms and machines and generate large amounts of data from their interactions (e.g. Benkler Citation2006), and (iii) software (e.g. Ensmenger Citation2012). It is software that makes it possible to program the computational power and direct the use of the networked resources to develop a wide variety of different applications. One could argue that software is what makes digital networked computers a general-purpose technology.
2.2. Software’s role in digitalisation
As digitalisation progresses, so too is software development spreading across different sectors of the economy. The decision to invest in software development, as opposed to buying standardised software ‘off the shelf’ can be framed in two ways: First, it can be understood as calculated risk-taking with the aim to secure a competitive edge, either out of necessity because there is no suitable standardised software product corresponding to the needs of the firm, or out of opportunity because the investing firm believes it can develop a software-based solution that is superior to existing ones in the market. Even if software development starts out as a means to an end within an existing business model, it may also develop into a new business opportunity where the firm can sell software as an independent product or service.
Second, for a firm to engage in software development can also be understood as a part of its digital transformation. In attempting to leverage productivity benefits from new technologies, firms must engage in an experimental search for complementary innovations, i.e. to adapt organisation and work to the new tools available (Brynjolfsson and Hitt Citation2000). Developing software is a way of adapting the new tools to the specific needs of the individual firm.
Software development can be thought of as utilising an ‘ever-expanding set of lego bricks’ of software-based functions (Branstetter, Drev, and Kwon Citation2019, p. 543) with increasing combinatorial innovation potential. It enables a variety of new industries including video games, social media and multisided platforms (e.g. Evans and Schmalensee Citation2016; Marchand and Hennig-Thurau Citation2013; Williams Citation2002). Furthermore, embedded software provides ample evidence of how software can be used to leverage computational capacity in traditional industries such as car manufacturing and aircraft design (Lee Citation2002, Citation2000). For example, modern military aircraft design is made inherently unstable to improve manoeuvrability, but this would be impossible unless software balanced the aircraft in real-time (Arthur Citation2009, p. 73). In addition, software and software development are at the heart of machine learning and artificial intelligence (e.g. Domingos Citation2015). The software also makes up an ‘invisible infrastructure’ that is becoming increasingly pervasive in and integrated into the economy as digitalisation progresses.
Pineiro (Citation2003), who studies individual-based variations in software programming, points out that software is literally everywhere, and a growing share of society is in different ways becoming dependent on different types of software. Along this vein, Ensmenger (Citation2012) argues that, as software is becoming ubiquitous, software programmers are becoming de facto planners of the digital society. Both of these observations echo the legal scholar Lawrence Lessig (Citation2000), who has made the argument that because software code regulates digital space much like legal code regulates physical space, code is the law.
Software also takes the centre stage in the ongoing shift or rebalancing from tangible to intangible assets in the economy. Haskel and Westlake (Citation2018) point out that not only is it becoming harder to estimate value without including intangible assets, but intangibles also exhibit characteristics that separate them from physical capital. Specifically, they formulate four characteristics of intangible assets: 1) they constitute sunk costs, 2) they generate spillovers, 3) they tend to be scalable, and 4) they have synergies (or complementarities). Because of this, the authors conclude, ‘we might reasonably expect an economy [increasingly] dominated by intangibles to behave differently’ (ibid p. 10).
Even so, the nature and characteristics of software and software development have oftentimes been overlooked or left out in macroeconomic analyses of the digital shift in the economy, perhaps because it falls in between traditional measures of technology uptake, organisational factors like human capital, and more recent indicators focusing on data.
2.3. Software development as an R&D investment linked to digital innovation
Since the economy is not ‘flat’ with respect to the introduction of digital technologies, and by extension the shift towards intangible assets, the distribution of software development across industries and firms provides an important indicator both how different industries and firms relate to technological change and how this is reflected in the economy. While software development is no new phenomenon and it is common knowledge that firms outside of the IT industry engage in their own software development, there is no coherent overview of how software development activities are distributed across the economy or what characterises the firms that invest in developing their own software across sectors.
Most firms in a modern economy like Sweden use software-based tools to some degree in their business. At the same time, there are likely to be considerable differences between the least and the most advanced uses of the software. There is a wide variation in the type of software-based applications that firms employ, ranging from email and smartphones to Enterprise Resource Planning (ERP) or Customer Relationship Management (CRM) systems and to big data analytics, machine learning and cyber security. Small and medium-sized enterprises, which constitutes most firms in all economies, often exhibit a low digital maturity in terms of the applications they have implemented into their businesses (Tillväxtanalys Citation2018; Wernberg Citation2020).
While most of these firms buy their software ‘off the shelf’, there is also a small group that invests in their own software development. These firms stand out because they are making a larger investment into tailoring software-based applications than their peers, but also because they are taking a considerable risk. Firms that buy standardised software products potentially gain the same competitive advantage from their investment as every other firm that bought the same product, provided it is successfully implemented into their business. Software-developing firms, on the other hand, stand to gain a unique competitive edge if they manage to successfully build software that is better aligned to their needs. They also risk netting a loss if that investment fails.
All firms should not be expected to engage in software development because of their digital transformation. On the contrary, because of the associated costs and risks, it is reasonable to believe that the number of software-developing firms will remain relatively small in comparison with firms that buy their software off the shelf. Thus, firm-level statistics about software development in the economy should not be interpreted as a comprehensive measure of progress. Instead, we propose to use these data to provide a snapshot of the distribution and orientation of investments in software-based intangible assets. When firms invest in software development it is coupled with their digital transformation, and as such the distribution of software development activities across sectors provides an important overview of the digital transition of the economy.
This transition is not homogeneous across industries as firms are expected to have different outputs. provides statistics across the purpose and nature of software development for manufacturing and service industries. The table shows the distributions of firms across different software development processes. It indicates all firms in the sample, only service and then only manufacturing firms and shows the percentage distribution across the purpose of the software.
Table 1. Statistics for in-house, external and mixed software development over the purpose of software.
There are some considerable differences between service and manufacturing firms. The share of firms investing in software development is larger in services industries than in manufacturing across the board, except for embedded software where manufacturing firms heavily outweigh service firms. Manufacturing firms favour in-house developers most heavily in work related to embedded software, while the same is true for service firms when it comes to selling software development as a service or building independent software products and services. In general, the table suggests that the more a firm’s service or product is tied to software development, the more likely that the firm develops software internally. Moreover, the nature of the product differs across the industries. Manufacturing firms choose to develop software and use it along with their physical products, while service firms develop software and sell it as a standalone service/intangible product.
There are clear differences between in-house and external developers with respect to the orientation of the software being developed. Firms that develop software as an independent product, work with embedded software or sell software development as a service predominantly use in-house developers, while firms that develop software to improve their operations or expand their distribution and sales rely more heavily on external developers. We expect that firms developing software to improve their internal processes or logistics choose not to invest in internal development as it requires competence and substantial changes in the organisation. This reflects not only a difference in the value of internalising the software development work, but also in the degree to which firms change their organisation to leverage software-based solutions.
Taken together, the discussion above suggests that while most firms in developed economies employ software-based tools to some degree, there is a considerable span between the least and the most advanced uses of the software. The approach taken in this paper builds on the presumption there should be a significant difference between firms that buy software ‘off the shelf’ and those that develop their own. Firms that buy standardised software products gain the same potential competitive advantage as any other firm that buys the same software.Footnote2 Furthermore, these users benefit from the economies of scale associated with updating and developing standardised software products.
Developing your own software is more akin to an R&D investment: it may succeed and generate a competitive advantage that is unique to the developing firm and separates it from competitors, but it may also fail and net a considerable loss to the firm. Software development, as compared to buying and implementing third party software products, is associated with more risk-taking and experimentation. Also, software development goes beyond mere technology adoption and arguably gives an indication that firms are attempting to adapt their business to leverage the benefits of digital technologies in a way that gives them a competitive edge. In this respect, software development is like R&D investments or investments in other types of digital innovation. This is the main proposition that this paper aims to test.
To this end, we employ a unique data set to study the distribution and orientation of software development among firms in the Swedish economy as well as the characteristics of software-developing firms with the purpose of contributing to a better understanding of 1) what characterises firms that invest in software development to gain a competitive advantage in the digital transformation, 2) what areas are most software-development-intensive in the Swedish economy and, by extension, in what areas are firms investing in this type of intangible assets.
Sweden provides a particularly interesting empirical case because of its high level of technological penetration and uptake. Early political initiatives aimed at expanding physical broadband infrastructure and promoting household investments in personal computers set the means that basic conditions for leveraging digital technologies are widely spread and accessible to most, if not all, businesses. A majority of ICT investment carried out by firms in Sweden is spent on software (Andersson and Lööf Citation2009). Investments in both in-house and external software development is high, and Sweden is holding a leading position amongst OECD countries. According to Ahmad (Citation2003), Sweden has spent 2.7% of its GDP share on software, placing the United States second. Accordingly, any differences in technology diffusion of investments in software development are unlikely to be the consequence of lacking or varying conditions. Instead, distributional patterns and characteristics reflect a larger degree of differences in priorities and firm-level capabilities. Taken together, this provides a tangible picture of what could be described as Sweden’s digital competitiveness.
3. Data, empirical strategy and descriptive statistics
3.1. Data
We employ data based on a unique firm-level survey about software development (SWD) that took place during 2019 among firms in Sweden. Firms that took part in the survey have also been identified in the Community Innovation Survey (CIS) data as well as firm-level register data. This allows us to combine information on firms’ SWD activities with information on innovation types as well as firm-specific information about the structure of employees, ownership, industry, size, and internationalisation.
The SWD survey focuses on whether firms develop software, if the software is developed by in-house employees, external consultants or whether the firms use both strategies. The survey also answers the question about the primary function of the software that a firm develops.Footnote3 For this, firms that develop software were asked to choose only one option that characterises their software. In the empirical analysis, we consistently separate between firms that have in-house, only external or mixed software development strategies as they represent different degrees by which software development is integrated with firms’ business operations.
It also includes questions related to the firm’s own perception of the market situation, specifically the degree of competition and whether it is a new or established market segment. The design of survey questions, as well as the population frame, was developed in collaboration with SWEDSOFT and Statistics Sweden (SCB), who also conducted the survey and validated the results.Footnote4
The frame population for the survey included Swedish companies. It was drawn from various industries: (i) Manufacturing, (ii) Energy, (iii) Trade, (iv) Transportation and Warehousing, (v) Information and Communication, (vi) Finance and Insurance, (vii) Law, Economics, Science and Technology. Survey questions were sent out to 9,425 firms in Sweden, and 4,598 firms submitted their response, which implies a response rate of 49 %. The person who responded to the SWD survey had to be part of the firm’s management board, corresponding to the chief technology officer or CEO.
A unique feature of the data is that the firms that completed the survey are identified in the firm-level register data. These register data include the Firm and Establishment Dynamics database (FEK), Foreign Trade data and the individual-level data from the Longitudinal Individual Level database (LISA). This implies that we can obtain information that refers to the year 2017 and covers (i) balance sheet statistics, (ii) employment composition, (iii) internationalisation activities and (iv) ownership structure.
These large-scale data have been paired with the latest Community Innovation Survey (CIS 2018), which allows us to correlate survey answers intimately with R&D activities and product strategies of firms. The CIS 2018 referred to the years 2016–208 and the SWD survey to 2019.Footnote5
After merging all datasets and dropping missing observations for variables of interest, we have 3,929 firms. This allows us both to map the development of software across industries and firm traits and to estimate which firm traits are most likely to correlate with software development in different industries. All data are accessed through the Microdata Online Access (MONA) service provided by SCB.Footnote6 The merged data allow us to develop a dataset with detailed information on software development as well as various background characteristics of the firms, such as size, age and education of employees, technical infrastructure, industry affiliation, export activity, multinationality, R&D activities and innovation types.
3.2. Empirical strategy and variables
3.2.1. Multinomial logit models (MNL)
The aim of the empirical analysis is to assess the characteristics of firms that develop software and how these firms differ depending on the orientation of the software they develop. Our main proposition is that software development is like R&D investments or investments in other types of digital innovation.
To this end, we use probability models to analyse how the probability of developing software is related to firm-level characteristics. We undertake two separate data analyses with two multinomial logit models (MNL). First, we examine companies focusing on the link between in-house, externally sourced and mixed software development and firms’ characteristics. Second, we assess how different purpose of the software that firms develop correlate with firm-level attributes.
To estimate the relationship for the first model, we set up an MNL where the dependent variable is a dummy which is one if the firm reports that they develop software in-house, two if it develops software using external service providers, three if it uses a mixture of both and zero if a firm is not involved in software development. For this model, the choice of ‘no software development’ is a base category. The firms were allowed to choose only one of these options.
For the second model, we analyse the relationship between the use of the software a firm develops and the attributes of the firm. Firms than develop software were asked to choose only one option that best corresponds to the main purpose of this software. In the analysis, the dependent variable is the dummy that displays the order of alternative characteristics of developed software, such as (1) software as an independent service or product, (2) value of the product is created by included software, (3) software for distribution or sale of the product, (4) for own operations, don’t sell anything software-based and (5) software development service (as a consultant). Firms that do not develop software were excluded from this analysis as they were not asked this question. We set the ‘software development service’ as a base category in the second model.
In our econometric analysis, predicted probability coefficients are interpreted with respect to the base category. The predicted probability for the MNL model is given by (Greene Citation2003):
where j ∈ ; j ∈ {1,2,3,4,5} and i = 1, …,1260 for the second MNL. EquationEquation 1
(1)
(1) provides a set of probabilities for different choices of software development
. In these models,
is the set of regression coefficients associated with outcome j, and vector
is the set of explanatory variables associated with observation
. After the estimation, we compute the average marginal effects which are the slope of the prediction function at a given value of the explanatory variable. It allows us to further examine the relative influence of our results and define the direction and magnitude of the relation between software development of any kind and firms’ attributes.
3.3. Control variables – firm characteristics related to the probability of SWD
We assess how several different characteristics of firms and their market position are related to the probability to develop software, including size, available infrastructure, innovation and R&D, education level of employees, firms’ own assessment of their market as well as ownership and internationalisation.
We assess the relationship between software development and firm size. The issue of technology adoption relating to company size has been examined widely in the literature (Cohen Citation2010; Kaplinsky Citation1983; Schumpeter Citation1942) with inconclusive findings. Drawing from Schumpeterian theory, larger firms have an advantage over small companies as they have access to technological resources and greater capabilities to invest capital into equipment and competence that are needed for software development. On the other hand, although large companies accumulate resources needed for software development, they may be less flexible than smaller firms and suffer from bureaucracy. Small firms can adapt to technological change quicker and account for a large share of product innovation, while large firms may prevail in the process innovation (Acs and Audretsch Citation1988; Yin and Zuscovitch Citation1998). One argument is that large firms are better apt to introduce in-house and external software development as they have greater access to resources, as well as to develop software that helps to improve the production and service processes, such as software for their own operations and distribution. However, small firms may be more likely to involve in software development as a product. To test the influence of firm size on software development, we compute firm size as dummies that reflect the number of workers employed at small (10–49), medium (50–249) and large (250+) firms. Information on the number of employees is based on the firm-level register data.
Software development may depend on whether the firm is able to absorb specific knowledge required to foster the implementation of new technologies. We draw on information from the SWD survey and include a dummy variable for whether firms own all technical infrastructure which can be data storage and capacity to handle data as well as having all technical infrastructure or most of the technical infrastructure is supplied by third parties.
We also include variables that reflect the age and education level of employees. These variables are computed from information on individual employees in the LISA database. The literature suggests that older employees may be less inclined towards the usage of new technologies while younger workers are more associated with the adoption and adaption of technological advancements or they join firms with greater innovation prospects (Ouimet and Zarutskie Citation2014; Pfeifer and Wagner Citation2014; Schubert and Andersson Citation2015). The rationale for this variable is that firms with younger workers may have higher propensities to develop software in-house. The average age of employees is intended to capture the link between the adoption and adaption of new technologies and the age of workers.
Firms’ capabilities to adapt to technological shift is also affected by the employees’ human capital, their abilities and knowledge (Vinding Citation2006). Our expectation is that firms with large share of highly skilled employees have greater inclination to engage in software development. Thus, we establish variables about education level of employees and develop two step measures of human capital. First, we identify employees with a long university education that is at least three years.Footnote7 In LISA database, codes for education level follow the SUN2000 (Swedish education) nomenclature. Then, we distinguished between the types of training. The education level of employees is reflected by two variables that are expressed as the proportion of a firm’s employees with long university education in STEMFootnote8 (science, technology, engineering, and mathematics) and the proportion of employees with a long university education in fields other than STEM. By distinguishing between STEM and non-STEM educated employees, we evaluate software development relative to education background.
We relate the characteristics of the markets in which firms operate that concern competitive pressure to introduce new products or develop software. The relationship between market competition and new technologies has been extensively discussed in the literature since Schumpeter’s distinction between Mark I and Mark II (Schumpeter Citation1942, Citation1934). As Mark I suggests, high market competition and low entry barriers foster innovation in smaller firms. On the other hand, established markets with high entry barriers and large firms operating in these markets could be the main drivers of technological developments according to Mark II (Malerba and Orsenigo Citation1996). Software development may stimulate the creation of new niche markets whereas high competition may stimulate firms to adopt new technologies and develop software of various kinds. To control for this, we include variables that reflect the self-assessed characteristics of the market and the degree of competition (both domestic and international). Expressed as dummy variables in the analysis, firms were asked to choose the option that best corresponds to the firm’s market conditions. Firms had to select whether they operate in a new or established market and identify the degree of competition.
As discussed before, several studies document a software-biased shift in innovation in the sense that innovation is increasingly associated with software development (Branstetter, Drev, and Kwon Citation2019; Andersson, Kusetogullari, and Wernberg Citation2021). Industry studies of R&D-performing firms also show that a significant fraction of the R&D staff in many firms indeed develop software.Footnote9 Firms that engage in R&D can be expected to have better prospects to develop new products and services, as they can adopt and adapt to the new technologies and absorb knowledge (Cohen and Levinthal Citation1990). To test for these arguments, our model includes a variable reflecting whether a firm has permanently employed R&D workers, i.e. if a firm is engaged in persistent R&D. It allows us to see how much the firm’s operations are potentially in line with the adoption of digital technologies and how it may be correlated with software development. Another aspect that we consider in the model is the firms’ innovation processes. We aim to better understand whether diverse ways of software development extend the firm’s likelihood to innovate and what is the main type of innovation outcome. The software can be used by firms not only to improve processes but to innovate in products and services. We also control for the degree of innovation by including the dummy on radical innovation, which indicates whether a firm has introduced a new product for the market. All these variables are expressed as dummies in the analysis and drawn from the CIS 2018 database.
Finally, we also consider whether a firm is engaged in export to foreign markets and whether it is affiliated with a multinational enterprise (MNE). Firms may have better prospects to develop new products if they are exposed to international markets and thus be more inclined towards software development. Exporting firms may use the interaction with foreign customers as a source of ideas for new products and services and also be subject to stronger competition, forcing them to be in the frontline of the digitalisation (Andersson and Lööf Citation2009; Fassio Citation2018). Firms that are affiliated with an MNE can have better access to technology and internal resources within MNEs, which opens prospects for product and process innovation (Cantwell and Iammarino Citation2005; Frenz and Ietto‐Gillies Citation2007). We expect that firms that are part of an MNE are disposed towards software development and have enough resources and knowledge to develop software in-house. The variables are drawn from an audited register firm-level data on exports and ownership structure and expressed as dummies.
4. Results
4.1. Frequency and orientation of software development
present descriptive statistics of the variables used in the empirical analyses. From we see that 12.14% of the firms in the sample have in-house software development while 11.05% of the firms develop software only externally and 8.88% use a mixture of internal and external development. Significantly less than half of the firms in the sample thus develop software (32.07%). Software development of any kind is more common for firms in the electronics, IT services and printing/publishing sectors. The majority of firms in law (82.2%) don’t develop software. In the industries, the fraction of firms that are engaged in internal software development is larger for machinery, electronics and publishing services. In the IT-services and technical engineering sectors majority of software is developed internally or as a mixture of both internal and external ways. External software development is however most frequent in energy/oil (21.21%).
Table 2. Industry distribution over the sample.
Table 3. Summary statistics: estimation sample.
According to Pavitt (Citation1984), firms can be classified according to their use and development of innovation. First, supplier-dominated firms such as firms from the textiles sector depend on innovation from suppliers. In line with Pavitt (Citation1984), most firms (80%) in the textiles and clothing industries do not develop software. Second, production-intensive firms (such as machinery and engineering) exist in symbiosis with large manufacturers and vehicles firms that consume their innovation. We see a similar pattern in , as most vehicles firms develop their software externally (18.9%). However, around 12% of vehicles sector firms have chosen to invest in software development in-house, which may be due to the changing nature of machinery and vehicles products.
The general pattern is thus that internal software development is common across industries, and that software development is more frequent in the electronics and telecommunications sectors.
presents summary statistics of all variables in the analysis. In our estimation sample, most firms are small (60%) and medium-sized (31%). Roughly half of all firms had their own technical infrastructure, and the average age of employees was around 41 years with 69 years as a maximum and 21 years at a minimum value. The average proportion of employees with a long university education in STEM was 19% whereas the proportion of employees educated in fields other than STEM reached 9%. With respect to market and competition variables, we see that majority of firms perceived the market conditions to be described as a market with high competition (80%) and only 4% defined their market as new. It is also clear that the most common reason to engage in software development is to improve the efficiency of their existing business (12% of the full sample), followed by those who develop their distribution and sales channels using software, working with embedded software, developing software as an independent product, and selling software development as a service. Looking at innovation variables, we see that a large share of firms (51%) is focused on process innovation and only 9.8% invest in persistent R&D. Roughly half of the firms in the sample report owning their own technical infrastructure, while the other half rents it from a third-party provider. This speaks to the notion that while most firms surely use the software in different capacities, there is a considerable difference between the least and the most advanced users.
4.2. Determinants of software development
The following section provides the estimation results from the MNL models. To determine the effect of firm characteristics on the probability scale, we computed average marginal effects over the estimation sample. For this model, we split the results into three alternative models: using the full sample and separating out service and manufacturing sectors, respectively. presents the estimation of the MNL model analysing the relation between software development and the firms’ characteristics.
Table 4. Determinants of software development in firms.
A first observation is that the probability of in-house software development exhibits a much clearer relationship with specific firm characteristics than external development. This indicates that in-house development is more concentrated to a few parts of the economy, perhaps because it requires more substantial long-term investments than it does to hire external developers.
Looking at the full sample, firm size clearly plays into the distribution of software development activities. First, firm size is strongly positively associated with the probability of in-house, external and mixed development across all three models. This indicates that overall software development is heavily defined by the investments made by the largest firms. While there are small firms and start-ups that have made considerable contributions to software development and software-related innovations, they are a clear minority among small and medium-sized firms. Most of these firms on the contrary exhibit low levels of digital adoption (Wernberg Citation2020). The result also shows that large firms are more likely to be engaged in software development. There is also a strong positive correlation between firm size and external development, which is in line with the notion that larger firms have more resources to invest in new projects and bring in consultants.
There is a strong, significant and positive connection between the probability of in-house software development activities and the share of employees with STEM educational background across all three models. This relationship is also positive for mixed software development. Furthermore, employees with STEM education also exhibit a negative and significant association with external software development. Firms with higher shares of STEM skills among employees tend to internalise their software development, whereas those with lower shares of STEM skills hire external developers. One explanation is of course that by internalising their software development, firms hire developers that add to the share of STEM skills. Yet, taken together with the negative correlation between STEM employees and external software development, these results may also lend themselves to interpretations suggesting that firms with higher shares of STEM skills (including individuals that are not developers themselves) have a higher absorptive capacity to internalise and adapt to new technologies. In line with this type of argument, firms that report owning and operating their own technical infrastructure (as opposed to buying it as a service from a third party) also exhibit a positive and significant correlation with the probability of in-house development across all three models.
Firms operating in a new market show a significant and positive probability of developing software internally. However, this result appear to be derived from a heterogeneous group of service firms in the full sample, because it vanish in the model where manufacturing firms are studied in isolation.
Exporting firms exhibit a positive and significant correlation with software development activities across all three models, except for external development in manufacturing firms. Related to this, firms that are part of a multinational enterprise (MNE) are disproportionately engaged in in-house software development for the full sample. This is consistent with the fact that MNEs typically have the resources to invest in new technology and R&D at an early stage. A distinguishing feature of MNEs is that they have ratios of R&D relative to sales, a large number of scientific, technical and other ‘white-collar’ workers as a percentage of their workforce, significant intangible assets and large product differentiation efforts, such as high advertising to sales ratios (Agarwal and Gort Citation2002).
Finally, firms that are involved in product and service innovation are more likely to develop software in-house. Moreover, firms that invest in persistent R&D are more likely to be engaged in software development activities across all models except manufacturing firms. These results are in line with the empirical results indicating a software-biased turn in innovation (Andersson, Kusetogullari, and Wernberg Citation2021). The process innovation variable shows a positive and significant correlation with external software development across all three models, which indicates that firms tend to outsource software development services to improve their internal processes.
4.3. Who develops software for what?
While the last section reported results for overall software development activities, we now separate different purposes of software development to test if and how they are related to different firm-level characteristics (). For this estimation, we use a slightly different approach and include only the manufacturing dummy rather than industry fixed effects. The primary motivation behind this is that we do not estimate this model for each sector specification and focus on the variations in the nature of software development. In the following model, we include only firms that develop software.
Table 5. Purpose of software: multinomial logit model (all sample).
There is a considerable variation in scope among the firms that engage in software development activities. Software development geared towards selling software-based products and services or working with embedded software is predominantly conducted in small firms with persistent R&D activity and firms with a larger share of STEM-educated employees. This speaks to a strong and positive correlation between knowledge-intensity and these two categories of software development, which were also biased towards in-house development (see descriptive statistics). Innovation variables follow a logical pattern, showing a strong relationship between embedded software and product innovation and software development as independent product and service innovation. Respectively, firms that develop software for their operations engage in process innovation.
Looking at the firm size, software development activities tilt towards the improvement of internal operations in medium-sized and large firms. Software development in firms that are part of multinational enterprises (MNEs) is more likely to be oriented towards on the one hand embedded software and on the other hand distribution and sales channels. Exporting firms exhibit a variation in software development activities with positive and significant conditional correlations with embedded software. Finally, we see that manufacturing firms are more likely to develop software to improve their own operations or to embed it into their main product while service companies are more inclined to develop software for their distribution operations or sell it as an independent product. This result is in line with our discussion on the intangible nature of the service industry’s output and the dissimilarity in the purpose of software development between manufacturing and services.
5. Summary and conclusions
This paper has analysed the characteristics of firms that are engaged in software development and the orientation of firms’ software development activities. Software is at the core of digitalisation as it is software that makes it possible to program and use computational power and direct the use of the network resources to develop a wide variety of different applications.
The main findings of this paper offer valuable insights on the relationship between software development and firms’ attributes. We find that larger firms, exporting firms, and firms that are part of MNEs all exhibit a higher degree of software development activities. These firms have the necessary resources to invest in new technologies and internalise the human capital required to leverage these technologies. This indicates that the largest firms make up an important part of the competitiveness in Swedish software development. While there are certainly technology-driven start-ups that have made considerable breakthroughs in software development, they are very much a minority among Swedish SMEs. This result indicates that in the interaction between small digital start-ups and large established firms, the larger firms are not just on the demand side, and the start-ups are not the only innovators.
Our next finding shows that in-house software development is positively and significantly correlated with firms that have higher shares of STEM-educated employees. These software development activities are primarily geared towards building new software-based products and services or working with embedded software. Taken together, these firms are investing heavily in software-related digital innovation and building a competitive edge in digital transformation. This comparatively small group of firms are also among the ones that will have the highest demand for technical (digital) expertise in the future. A better understanding of their needs could provide tangible inputs for higher education and vocational training.
Software development related to distribution, sales, and internal operations is more associated with external development. Accordingly, firms that develop these kinds of software externally do not internalise the relevant skills associated with software development. It is highly likely that these firms are to a greater degree customers of knowledge-intensive firms that sell software development as a service. Firms that choose to develop software externally are also investing in building a competitive edge using software – much more so than firms that simply buy their software off the shelf – but in a different way than their knowledge-intensive peers that tie software development more strongly to their core business. While both internal and external software development is represented among service firms, manufacturing firms exhibit a much stronger tilt towards software activities related to embedded software products.
Moreover, software development is positively and significantly correlated with persistent R&D activities in both the manufacturing and service industries. Taken together, software development can and should be treated as an investment comparable but complementary to R&D or, more broadly speaking, digital innovation. While software development activities vary across industries and firms, they make up an important intangible asset that is strongly associated not only with innovation but also with competitiveness in the digitalised economy. Thus, there should be a strong case for tracking and improving our understanding of software development in the economy.
Against this backdrop, we arrive at two broad concluding remarks that also point to potential directions for future research. First, the results show diversity across firms in terms of the application of developed software, which suggests that the demand for software development skills tends to be more heterogeneous than what is oftentimes assumed. Educational institutions and policymakers can create training programmes to address specific demands for skills needed for various types of software. Yet, our results suggest that there may be no easy one-size-fits-all answer as to what digital skills firms will demand in the future comparable to the education of engineers following the last industrial revolution.
Finally, our results support the idea that software development is akin to R&D investments for many firms. This, in turn, holds two possible implications for how we think of R&D as an economic indicator. First, R&D may be turning more software-intensive, as suggested by the correlation between R&D-intensive firms and investments in software development. This is also in line with our previous findings that innovation in Swedish firms is becoming increasingly software-biased (Andersson, Kusetogullari, and Wernberg Citation2021). Second, software development could potentially lower the barrier to R&D activities. It requires little or no physical capital, given that even demanding computation can be conducted through cloud services. While investments in human capital still provide a tangible barrier to engage in software development, the conditions are arguably shifting towards more accessible innovation activities. Specifically, the barrier to experiment with digital technologies has been steadily lowered over the last decades and with the introduction of machine learning and other AI services that contribute to automating some standard programming techniques, we may see new variations of research, development, design and innovation emerging in the future. Taken together, these two implications suggest that we may need to revisit issues of the measure, organisation and distribution of R&D in the economy.
Disclosure statement
No potential conflict of interest was reported by the author(s).
Data availability statement
The data that support the findings of this study is accessed through the Microdata Online Access (MONA) service provided by Statistics Sweden. Restrictions apply to the availability of these data, which were used under license for this study. Data are available at https://www.scb.se/MONA with the permission of Statistics Sweden.
Notes
1 In addition, the decision to invest in software development is not only a result of internal factors, but also of changing external conditions. Because software-based applications are part of a GPT, each firm’s environment is not only shaped by its immediate competitors but also by ripple effects of the structural change happening in other parts of the economy. For example, the wide spread of smartphones with internet connection has significantly changed the demand for software-based services far beyond the IT and telecommunications sector.
2 Granted, some firms may be better equipped than others to leverage a specific standardised software-based technology.
3 The complete set of survey questions are available from the authors upon request.
4 Information about SWEDSOFT is available here: https://www.swedsoft.se/en/.
5 In our empirical context the discrepancy in timing between the CIS and the SWD survey is not an issue. First, we do not aim to conduct a strict causal analysis between software development and R&D activities, but focused on the overall relationship between those two. Second, software development is a continuous event that involves refinements and testing rather than a sole occasion. The survey was designed to assess whether software is integrated into firms’ business operations rather than an one-off event that took part in the particular year.
7 Long university education is defined as employees with any of the following codes: 53 – three years; 54 – four years; 55 – five or longer. Doctorate education: 64 – PhD; 62 – licentiate.
8 Code 4 – Biology and environmental science; physics, chemistry and geoscience; mathematics and natural science; computer science. Code 5 – Engineering.
9 A survey of the 39 largest R&D-firms in Sweden conducted in 2016 showed that four out of 10 R&D employees, i.e. 40 %, are involved in software development, (see https://www.nyteknik.se/innovation/4-av-10-fou-anstallda-utvecklar-programvara-6578226)
References
- Acs, Z. J., and D. B. Audretsch. 1988. “Innovation in Large and Small Firms: An Empirical Analysis.” Am. Econ. Rev 78: 678–690.
- Agarwal, R., and M. Gort. 2002. “Firm and Product Life Cycles and Firm Survival.” American Economic Review 92 (2): 184–190. doi:10.1257/000282802320189221.
- Ahmad, N., 2003. “Measuring Investments in Software, OECD Directorate for Science, Technology and Industry Working.” Paper 2003/6, OECD, Paris, available at www.oecd.org/sti/working-papers
- Andersson, M., A. Kusetogullari, and J. Wernberg. 2021. “Software Development and Innovation: Exploring the Software Shift in Innovation in Swedish Firms.” Technological Forecasting and Social Change 167: 120695. doi:10.1016/j.techfore.2021.120695.
- Andersson, M., and H. Lööf. 2009. “Learning-by-Exporting Revisited: The Role of Intensity and Persistence*.” The Scandinavian Journal of Economics 111 (4): 893–916. doi:10.1111/j.1467-9442.2009.01585.x.
- Andreessen, M. 2011. “Why Software Is Eating The World.” Wall Str. J .
- Arthur, W. B. 2009. The Nature of Technology: What It Is and How It Evolves. New York: Simon and Schuster.
- Auerswald, P. E. 2017. The Code Economy: A forty-thousand Year History. Oxford: Oxford University Press.
- Benkler, Y. 2006. The Wealth of Networks: How Social Production Transforms Markets and Freedom. New Haven [Conn: Yale University Press.
- Bordel, B., R. Alcarria, T. Robles, and D. Martín. 2017. “Cyber–physical Systems: Extending Pervasive Sensing from Control Theory to the Internet of Things.” Pervasive Mob. Comput 40: 156–184. doi:10.1016/j.pmcj.2017.06.011.
- Branstetter, L. G., M. Drev, and N. Kwon. 2019. “Get with the Program: Software-driven Innovation in Traditional Manufacturing.” Management Science 65 (2): 541–558. doi:10.1287/mnsc.2017.2960.
- Bresnahan, T. F., and M. Trajtenberg. 1995. “General Purpose Technologies ‘Engines of Growth’?” Journal of Econometrics 65 (1): 83–108. doi:10.1016/0304-4076(94)01598-T.
- Brynjolfsson, E., and L. M. Hitt. 2000. “Beyond Computation: Information Technology, Organizational Transformation and Business Performance.” Journal of Economic Perspectives 14 (4): 23–48. doi:10.1257/jep.14.4.23.
- Brynjolfsson, E., and A. McAfee. 2014. The Second Machine Age: Work, Progress, and Prosperity in a Time of Brilliant Technologies. New York: WW Norton & Company.
- Cantwell, J., and S. Iammarino. 2005. “The Technological Innovation of Multinational Corporations in the French Regions.” Revue d’économie industrielle 109 (1): 9–28. doi:10.3406/rei.2005.3062.
- Castellacci F. (2008). Technological paradigms, regimes and trajectories: Manufacturing and service industries in a new taxonomy of sectoral patterns of innovation. Research Policy, 37(6–7), 978–994. 10.1016/j.respol.2008.03.011
- Castells, M. 1996. The Rise of the Network Society. Hoboken: Blackwell Publishing.
- Cohen, W. M. 2010. Fifty Years of Empirical Studies of Innovative Activity and Performance, 129–213. Amsterdam: Elsevier. doi:10.1016/S0169-7218(10)01004-X. https://www.sciencedirect.com/science/article/pii/S016972181001004X
- Cohen, W. M., and D. A. Levinthal. 1990. “Absorptive Capacity: A New Perspective on Learning and Innovation.” Administrative Science Quarterly 35 (1): 128–152. doi:10.2307/2393553.
- Corrado C, Hulten C and Sichel D. (2009). INTANGIBLE CAPITAL AND U.S. ECONOMIC GROWTH. Review of Income and Wealth, 55 (3): 661–685. 10.1111/j.1475-4991.2009.00343.x
- Domingos, P. 2015. The Master Algorithm: How the Quest for the Ultimate Learning Machine Will Remake Our World. 1st edition. ed. New York: Basic Books.
- Ensmenger, N. L. 2012. The Computer Boys Take Over: Computers, Programmers, and the Politics of Technical Expertise. Cambridge: MIT Press.
- Evans, D. S., and R. Schmalensee. 2016. Matchmakers: The New Economics of Multisided Platforms. Brighton: Harvard Business Review Press.
- Fassio, C. 2018. “Export-led Innovation: The Role of Export Destinations.” Industrial and Corporate Change 27 (1): 149–171. doi:10.1093/icc/dtx028.
- Frenz, M., and G. Ietto‐Gillies. 2007. “Does Multinationality Affect the Propensity to Innovate? an Analysis of the Third UK Community Innovation Survey.” International Review of Applied Economics 21 (1): 99–117. doi:10.1080/02692170601035033.
- Geroski, P. A. 2000. “Models of Technology Diffusion.” Research Policy 29 (4–5): 603–625. doi:10.1016/S0048-7333(99)00092-X.
- Grajek, M. 2012. ICT for Growth: A Targeted Approach 2012/10 . Brussels: Bruegel Policy Contribution. No. 2012/10.
- Greene, W. H. 2003. Econometric Analysis. 5th edition. ed. Upper Saddle River, N.J: Prentice Hall.
- Grieves, M., and J. Vickers. 2017. “Digital Twin: Mitigating Unpredictable, Undesirable Emergent Behavior in Complex Systems.” In Transdisciplinary Perspectives on Complex Systems: New Findings and Approaches, edited by F.-J. Kahlen, S. Flumerfelt, and A. Alves, 85–113. Cham: Springer International Publishing. doi:10.1007/978-3-319-38756-7_4.
- Griliches, Z. 1957. “Hybrid Corn: An Exploration in the Economics of Technological Change.” Econometrica, Journal of the Econometric Society 25 (4): 501–522. doi:10.2307/1905380.
- Hall, B. H. 2004. Innovation and Diffusion. Cambridge: National Bureau of Economic Research. No. w10212.
- Haskel, J., and S. Westlake. 2018. Capitalism without Capital: The Rise of the Intangible Economy. Princeton: Princeton University Press.
- Kaplinsky, R. 1983. “Firm Size and Technical Change in a Dynamic Context.” The Journal of Industrial Economics 32 (1): 39–59. doi:10.2307/2097985.
- Kim, K., J. Lee, and A. Gopal. 2019. “Software-based Innovation, Product Market Competition, and Value Creation in the IT Hardware Industry.” Academy of Management Proceedings 2019: 1. Accessed 18 November 2019.
- Lee, E. A. 2000. “What’s Ahead for Embedded Software?” Computer 33 (7): 18–26. doi:10.1109/2.868693.
- Lee, E. A. 2002. “Embedded Software.” In Advances in Computers, edited by M. V. Zelkowitz, 55–95. Cambridge: Elsevier. doi:10.1016/S0065-2458(02)80004-3.
- Lessig, L., 2000. “Code Is Law [WWW Document].” Harv Mag. https://harvardmagazine.com/2000/01/code-is-law-html ( accessed 4.13.21).
- Lipsey, R. G., K. I. Carlaw, and C. T. Bekar. 2005. Economic Transformations: General Purpose Technologies and long-term Economic Growth. New York: OUP Oxford.
- Malerba, F., and L. Orsenigo. 1996. “Schumpeterian Patterns of Innovation are technology-specific.” Research Policy 25 (3): 451–478. doi:10.1016/0048-7333(95)00840-3.
- Marchand, A., and T. Hennig-Thurau. 2013. “Value Creation in the Video Game Industry: Industry Economics.” Consumer Benefits, and Research Opportunities. J. Interact. Mark 27: 141–157. doi:10.1016/j.intmar.2013.05.001.
- McAfee, A., and E. Brynjolfsson. 2017. Machine, Platform, Crowd: Harnessing Our Digital Future. New York: W. W. Norton & Company.
- Monostori, L., B. Kádár, T. Bauernhansl, S. Kondoh, S. Kumara, G. Reinhart, O. Sauer, G. Schuh, W. Sihn, and K. Ueda. 2016. “Cyber-physical Systems in Manufacturing.” CIRP Ann 65: 621–641. doi:10.1016/j.cirp.2016.06.005.
- Nambisan, S., K. Lyytinen, A. Majchrzak, and M. Song. 2017. “Digital Innovation Management: Reinventing Innovation Management Research in a Digital World.” Mis Quarterly 41 (1): 223–238.
- Nelson, R. R., and S. G. Winter. 1982. An Evolutionary Theory of Economic Change. Cambridge, MA: Harvard University Press.
- Niebel, T., F. Rasel, and S. Viete. 2019. “BIG data–BIG Gains? Understanding the Link between BIG Data Analytics and Innovation.” Economics of Innovation and New Technology 28 (3): 296–316.
- Ouimet, P., and R. Zarutskie. 2014. “Who Works for Startups? the Relation between Firm Age, Employee Age, and Growth.” J. Financ. Econ 112: 386–407. doi:10.1016/j.jfineco.2014.03.003.
- Pavitt, K. 1984. “Sectoral Patterns of Technical Change: Towards a Taxonomy and a Theory.” Res. Policy 13 (6): 343–373. doi:10.1016/0048-7333(84)90018-0.
- Pfeifer, C., and J. Wagner. 2014. “Is Innovative Firm Behavior Correlated with Age and Gender Composition of the Workforce? Evidence from a New Type of Data for German Enterprises.” J. Labour Mark. Res 47: 223–231. doi:10.1007/s12651-013-0137-y.
- Pineiro, E. 2003. “The Aesthetics of Code : On Excellence in Instrumental Action.“ Stockholm: Industriell ekonomi och organisation. https://www.diva-portal.org/smash/record.jsf?pid=diva2%3A9481&dswid=-6023
- Quinn, J. B., J. J. Baruch, and K. A. Zien. 1996. “Software-based Innovation.” The McKinsey Quarterly 4: 94.
- Rajkumar, R., I. Lee, L. Sha, and J. Stankovic, 2010. “Cyber-physical Systems: The Next Computing Revolution.” in: Design Automation Conference. Presented at the Design Automation Conference, Anaheim, CA, USA, pp. 731–736. 10.1145/1837274.1837461.
- Romer, P. M. 1990. “Endogenous Technological Change.” J. Polit. Econ 98: S71–S102.
- Schubert, T., and M. Andersson. 2015. “Old Is Gold? the Effects of Employee Age on Innovation and the Moderating Effects of Employment Turnover.” Econ. Innov. New Technol 24: 95–113. doi:10.1080/10438599.2014.897858.
- Schumpeter, J. A. 1934. The Theory of Economic Development —. Cambridge, MA: Harvard University Press.
- Schumpeter, J. A. 1942. Capitalism, Socialism and Democracy. London: Harper & Brothers.
- Schwab, K. 2017. The Fourth Industrial Revolution, 192. New York: Crown.
- Solow, R. M. 1957. “Technical Change and the Aggregate Production Function.” Rev. Econ. Stat 39: 312–320. doi:10.2307/1926047.
- Tillväxtanalys (2018). https://www.tillvaxtanalys.se/publikationer/pm/pm/2019-09-16-foretagens-digitala-mognad-2018.html
- Utterback, J. M. 1974. “Innovation in Industry and the Diffusion of Technology.” Science 183 (4125): 620–626.
- Varian, H. R. 2003. Innovation, Components and Complements. Berkeley: University of California. October.
- Vinding, A. L. 2006. “Absorptive Capacity and Innovative Performance: A Human Capital Approach.” Econ. Innov. New Technol 15: 507–517. doi:10.1080/10438590500513057.
- Wernberg, J. (2020). “Små och medelstora.” https://entreprenorskapsforum.se/wp-content/uploads/2020/10/Rapport_Digital_omstallning_web.pdf
- Williams, D. 2002. “Structure and Competition in the U.S. Home Video Game Industry.” Int. J. Media Manag 4: 41–54. doi:10.1080/14241270209389979.
- Yin, X., and E. Zuscovitch. 1998. “Is Firm Size Conducive to R&D Choice? A Strategic Analysis of Product and Process Innovations.” J. Econ. Behav. Organ 35: 243–262. doi:10.1016/S0167-2681(98)00057-2.
- Yoo, Y., O. Henfridsson, and K. Lyytinen. 2010. “Research commentary—the New Organizing Logic of Digital Innovation: An Agenda for Information Systems Research.” Information Systems Research 21 (4): 724–735.