Abstract
This paper describes the ‘ordinary science intelligence’ scale (OSI_2.0). Designed for use in the empirical study of risk perception and science communication, OSI_2.0 comprises items intended to measure a latent capacity to recognize and make use of valid scientific evidence in everyday decision-making. The derivation of the items, the relationship of them to the knowledge and skills OSI requires, and the psychometric properties of the scale are examined. Evidence of the external validity of OSI_2.0 is also presented. Finally, the utility of OSI_2.0 is briefly illustrated by its use to assess standard survey items on evolution and global warming: when administered to members of a US general population sample, these items are more convincingly viewed as indicators of one or another latent cultural identity than as indicators of science comprehension.
1. Introduction: OSI_2.0
This paper (more a technical research note, actually) furnishes information on the ‘ordinary science intelligence’ scale (OSI). OSI is a tool to facilitate empirical investigation of how individual differences in science comprehension contribute to public perceptions of risk and like facts. The paper describes the motivation for OSI, the process used to develop it, and the scale’s essential psychometric properties. It also presents evidence of the external validity of the scale as a predictor of scientific reasoning proficiency. Finally, it illustrates the utility of OSI for testing hypotheses on the relationship between public science comprehension and controversial applications of science.
The current version of OSI is the successor to the science comprehension instrument used in Kahan et al. (Citation2012) and was featured in a study reported in Kahan (Citation2015a). Additional refinements, including creation of a short form, are anticipated. To distinguish it from previous and likely future versions, the scale described in this paper will be referred to as ‘OSI_2.0.’
2. What and why?
The validity of any science-comprehension instrument must be evaluated in relation to its purpose. The quality of the decisions ordinary individuals make in myriad ordinary roles – from consumer to business owner or employee, from parent to citizen – will depend on their ability to recognize and give proper effect to all manner of valid scientific information (Baron Citation1993; Dewey Citation1910). It is variance in this form of science comprehension – and not variance in the forms or levels of comprehension distinctive of trained scientists, or the aptitudes of prospective science students – that OSI_2.0 is intended to measure.
This capacity will certainly entail knowledge of certain basic scientific facts or principles. But it will demand as well various forms of mental acuity essential to the acquisition and effective use of additional scientific information. A public science-comprehension instrument cannot be expected to discern proficiency in any one of these reasoning skills with the precision of an instrument dedicated specifically to measuring that particular form of cognition. It must be capable, however, of assessing the facility with which these skills and dispositions are used in combination to enable individuals to successfully incorporate valid scientific knowledge into their everyday decisions.
A valid and reliable measure of such a disposition could be expected to contribute to the advancement of knowledge in numerous ways. For one thing, it would facilitate evaluation of science education across societies and within particular ones over time (National Science Board Citation2014). It would also enable scholars of public risk perception and science communication to more confidently test competing conjectures about the relevance of public science comprehension to variance in – indeed, persistent conflict over – contested risks, such as climate change (Hamilton Citation2011; Hamilton, Cutler, and Schaefer Citation2012), and controversial science issues such as human evolution (Miller, Scott, and Okamoto Citation2006). Such a measure would also promote ongoing examination of how science comprehension influences public attitudes toward science more generally, including confidence in scientific institutions and support for governmental funding of basic science research (e.g. Allum et al. Citation2008; Gauchat Citation2011). These results, in turn, would enable more critical assessments of the sorts of science competencies that are genuinely essential to successful everyday decision-making in various domains – personal, professional, and civic (Toumey Citation2011).
In fact, it has long been recognized that a valid and reliable public science-comprehension instrument would secure all of these benefits. The motivation for the research reported in this paper is widespread doubt among scholars that prevailing measures of public ‘science literacy’ possess the properties of reliability and validity necessary to attain these ends (e.g. Pardo & Calvo Citation2004; Guterbock et al. 2011; Roos Citation2012; Stocklmayer and Bryant Citation2012). OSI_2.0 was developed to remedy these defects.
The goal of this paper is not only to apprise researchers of OSI_2.0’s desirable characteristics in relation to other measures typically featured in studies of risk and science communication. It is also to stimulate these researchers and others to adapt and refine OSI_2.0, or simply devise a superior alternative from scratch, so that researchers studying how risk perception and science communication interact with science comprehension can ultimately obtain the benefit of a scale more distinctively suited to their substantive interests than are existing ones.Footnote1
3. Item derivation and scale development
OSI_2.0 is a latent variable measurement instrument. Responses to the items that the scale comprises are not understood to certify familiarity with any canonical set of facts or principles. Rather, they are conceptualized as observable (or manifest) indicators of an unobservable (latent) cognitive capacity that enables individuals to acquire and use scientific knowledge. The cognitive capacity is deemed to cause responses to the items, which individually can be seen as imperfect or noisy proxies for the unobserved capacity. When responses to multiple items are appropriately aggregated, their covariance furnishes an even more discerning (reliable) measure of the latent variable insofar as each item’s random variance – that part of the variance unrelated to the latent variable – is offset by the same in the others (DeVellis Citation2012).
OSI_1.0 – the scale used in Kahan et al. (Citation2012) – consisted of eight items from the National Science Foundation Science Indicators (National Science Board Citation2014) battery and fourteen numeracy scale items (Lipkus, Samsa, and Rimer Citation2001; Peters et al. Citation2006). The goal of combining these measures was to measure a latent science comprehension disposition indicated jointly by knowledge of various basic scientific facts and principles and by quantitative reasoning dispositions essential to giving empirical information proper effect.
Drawn from a more diverse range of sources, and evaluated over multiple different data collections, the 18 items that make up OSI_2.0 reflect the same basic strategy. Although intended to measure a unitary cognitive capacity, the items can for expository purposes be divided into four sets (Table ).
Table 1. OSI_2.0 items.
3.1. Scientific ‘fact’ items
The first set of items – six in total – relate to knowledge of certain basic scientific facts. There is indeed no reason for thinking that knowledge of these particular facts is particularly germane to the capacity to recognize and use valid scientific knowledge in everyday life. Nevertheless, they are ones that it is reasonable to believe a person who has acquired such a capacity will be familiar with.
The primary source was the NSF Indicators battery (National Science Board Citation2014), which is dominated by true–false items relating to basic propositions in the physical and biological sciences (e.g. ‘Antibiotics kill viruses as well as bacteria’; ‘Electrons are smaller than atoms’). The Indicators’ ‘basic fact’ items are known to furnish an extremely undemanding test (Pardo & Calvo Citation2004). When the battery is administered to a general US population sample, the median number of correct responses to the battery’s nine ‘fact’ questions is six (National Science Board Citation2014). Over the course of the development of OSI_2.0, certain NSF items (e.g. ‘The center of the Earth is very hot’; ‘It is the father’s gene that decides whether the baby is a boy or a girl’) were discarded as too likely to be answered correctly to furnish information useful for assessing differences in science comprehension.
To supplement the Indicators' 'basic fact' items, comparable ones from the Pew ‘Science and Technology’ battery (Citation2013) were assessed as well. One item, a multiple-choice question (‘which gas makes up most of the Earth’s atmosphere – hydrogen, nitrogen, carbon dioxide, oxygen’), was included in the scale.
3.2. Scientific ‘methods’ items
OSI_2.0 also includes three NSF Indicator items relating to ‘understanding of how science generates and assesses evidence, rather than knowledge of particular facts’ (National Science Board Citation2014, 7–23). One of these items tests recognition of the contribution that a control condition makes to drawing causal inferences in experiments. Two others assess familiarity with rudimentary principles of probability.
3.3. Quantitative reasoning
Six OSI_2.0 items come from the Lipkus/Peters Numeracy battery (Lipkus, Samsa, and Rimer Citation2001; Weller et al. Citation2012). Numeracy items measure proficiency in reasoning with quantitative information. Only modest math skill is needed to answer the problems that the items set forth; solving them depends, first, on fluency with quantitative representations of information (probabilities, percentages, and odds, e.g.) and, second, on consistent recognition of which quantitative reasoning skills (ones involving conditional probabilities, e.g.) are necessary to draw valid inferences from such information.
3.4. Cognitive reflection items
Finally, OSI_2.0 includes the three items that make up the Cognitive Reflection Test (Frederick Citation2005). The CRT items present word problems, the solution to which requires respondents to resist crediting an answer that is immediately and intuitively appealing but that can be shown by logical analysis to be unsound. Responses to the items are understood to indicate a disposition to critically interrogate existing beliefs on the basis of available information. CRT scores have been shown to predict resistance to cognitive biases associated with over-reliance on heuristic reasoning (Hoppe and Kusterer Citation2011; Toplak, West, and Stanovich Citation2011), particularly when used in conjunction with numeracy items (Liberali et al. Citation2011; Reyna et al. Citation2009).
4. Psychometric properties
This section reports the psychometric properties of OSI_2.0. The analyses described are based on the responses of a sample of 2000 US adults recruited by YouGov, Inc., a public opinion research firm that conducts online surveys and experiments on behalf of academic and governmental researchers and commercial customers (including political campaigns). The firm’s general population recruitment and stratification methods have been validated in studies comparing the results of YouGov surveys with those conducted for the American National Election Studies (Ansolabehere & Rivers Citation2013). The sample in this study was 55% female, and the average age of the subjects was 49 years. Seventy-two percent of the subjects were white, and 12% African-American. The median education level was ‘some college’; the median annual income was $40,000–$49,000. The study was administered between 24 April and 5 May 2014.
4.1. Covariance structure
Although it includes a combination of knowledge, skills, and dispositions, ‘OSI’ is posited to be a unitary cognitive capacity. Accordingly, OSI_2.0 should display the properties characteristic of a reliable measure of a single latent variable.
Factor analysis results were consistent with treating it as such (Table , I). The analysis, which employed principal factor extraction without rotation, disclosed that a single factor accounted for 87 percent of the variance in OSI_2.0’s 18 items. That factor had an eigenvalue of 7.5, over 12 times as large as the second largest factor (0.6), which explained only 7% of the variance. With the exception of ELECTRON (β = 0.43), every item had a factor-loading coefficient greater than 0.50. Under conventional standards, these results support treating the items as measuring a single latent disposition (DeVellis Citation2012; Morizot, Ainsworth, and Reise Citation2007).
Table 2. Factor analyses.
The Cronbach’s α was 0.83 for the scale as a whole. This score suggests OSI_2.0 can be expected to furnish a highly reliable measure of the posited ‘OSI’ disposition. But as will be discussed next, the use of item response theory to score responses enables a more informative assessment of scale reliability across the range of the entire latent OSI disposition.
4.2. Item response theory
A two-parameter item response theory model was used to score OSI_2.0 (Table ). In such a model, the item ‘difficulty’ parameter reflects the level of the latent disposition at which a respondent’s probability of a correct answer is 0.5; the ‘slope’ parameter reflects how dramatically the probability approaches 0 or 1 as the respondent’s disposition level either falls short of or exceeds that level (Figure ). Weighting items consistently with these parameters enables the measurement precision of the scale (its reliability) to be assessed across the range of the continuous latent variable. This property makes IRT desirable for constructing and scoring scales that a researcher can be confident discriminate among levels of the underlying disposition either uniformly across the range of that disposition or with greater precision within particular ranges of interest (DeMars Citation2010; Embretson Citation2010; Embretson and Reise Citation2000).
Table 3. IRT item parameters.
Figure 1. Illustrative item response curves.
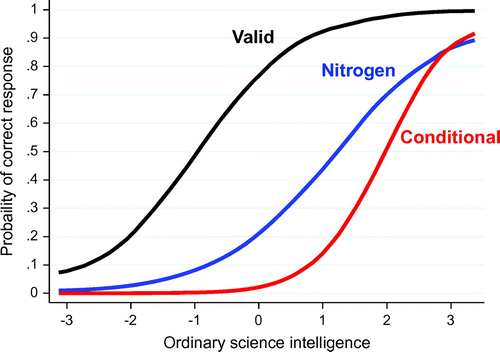
Fitting such a model to OSI_2.0 suggests a scoring hierarchy among the different sets of items. The ‘method’ and ‘fact’ items are the easiest, and thus function primarily to rank respondents lowest in ‘OSI.’ Although the most difficult item is CONDITIONAL, a member of the Numeracy set, the CRT items generally are the hardest and thus contribute the most to the ranking of the highest levels of OSI. As a set, the Numeracy items are middling in difficulty, but in fact they contribute to discrimination across the entire OSI range (Table and Figure ).
Figure 2. Test information functions and reliability.
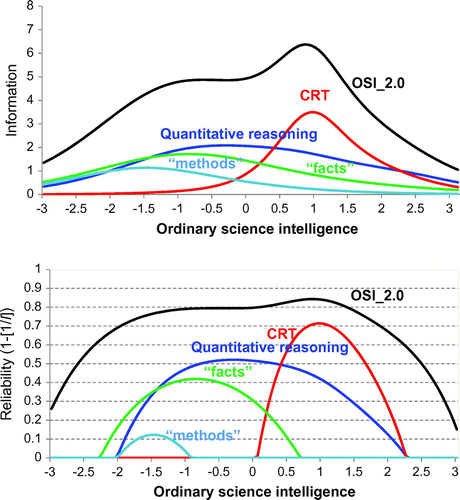
The subcomponents of OSI_2.0 combine to form a unitary scale that has high reliability across the entire range of the OSI disposition. The variable IRT reliability coefficient is highest (0.84) at +1 SD but remains above 0.70 even at −2 and +2 SDs.
The reliability of the individual subcomponents, in contrast, can be seen to be concentrated over much smaller portions of the OSI disposition. For example, CRT, the reliability of which peaks at 0.71 at +1 SD, drops below 0.50 at +1.7 SDs. Because of the extreme difficulty of the test, the reliability of CRT drops to 0.0 at the mean, rendering it unable on its own to distinguish varying levels of OSI for approximately half the members of the general population.
5. External validity
Dishearteningly, there is scant research validating the NSF Science Indictor battery or like ‘science literacy’ measures featured in the study of risk perception and science communication (Pardo & Calvo Citation2004). The dearth of such scholarship furnishes much of the motivation for the development of OSI_2.0.
Evidence of the external validity of OSI_2.0 consists in part in the extensive research examining the power of the items that form its quantitative reasoning and cognitive reflection subcomponents to predict reasoning proficiencies and dispositions essential to recognizing valid scientific knowledge and giving it proper effect. To supplement this evidence, OSI_2.0 scores were used to assess study subjects’ performance on a standard ‘covariance detection’ problem.
A ‘covariance detection’ problem requires respondents to analyze the results of a fictional experiment (Arkes and Harkness Citation1983). To correctly answer the problem, respondents must contrast the ratio of positive to negative outcomes in a treatment condition with the ratio of such outcomes observed in a control condition, information summarized in a 2 × 2 contingency table or equivalent. Respondents will get the wrong answer if they use either of two common heuristic substitutes for such reasoning: a comparison of the number of positive outcomes to negative outcomes in the treatment condition; or a comparison of the number of positive outcomes in the treatment to the number of positive outcomes in the control (Figure ). The problem tests not only individuals’ motivation and ability but also their spontaneous recognition of the need to perform an analytical task essential to making valid causal inferences (Stanovich Citation2009, 2011).
Figure 3. Covariance detection problem.
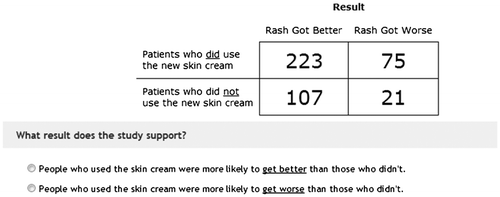
The task that the covariance problem features is integral to identifying and giving proper effect to valid scientific information. If OSI_2.0 successfully measures OSI, then it ought to be a strong predictor of performance on this problem.
This turns out to be so. Individuals who score low on the scale are highly unlikely to answer the question correctly. They become progressively more likely to give the right response as their scores progress, and at about the 90th percentile of OSI_2.0 there is approximately a 70% probability a respondent will answer the covariance problem correctly (Figure ). This relationship furnishes evidence of the external validity of OSI_2.0.
Figure 4. Predictive power of OSI_2.0 for performance on the covariance detection problem.
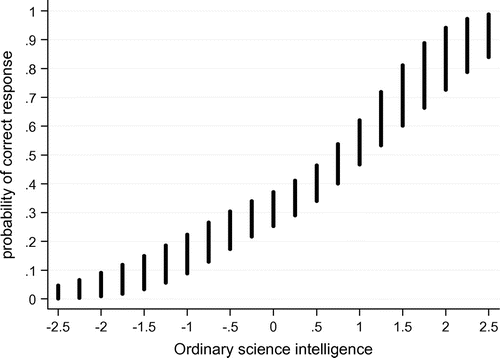
The same is true for the relationship between OSI_2.0 and other measures one would expect to play a role in OSI. Unsurprisingly, there is a modest positive correlation (r = 0.40, p < 0.01) between OSI_2.0 scores and level of education. The same degree of association (r = 0.40, p < 0.01) exists between OSI_2.0 and scores on Baron’s ‘actively open minded thinking’ (‘AOT’) scale, which measures the disposition to seek out and fairly evaluate evidence contrary to one’s existing beliefs (Haron, Ritov & Mellers Citation2013; Baron 2008).
AOT and education are correlated (r = 0.21, p < 0.01), too, but less strongly than either is with OSI_2.0. In addition, neither AOT nor education is as strong a predictor as OSI_2.0 of performance on the covariance detection problem (Figure ).
Figure 5. Predictive power of education and AOT for performance on the covariance detection problem.
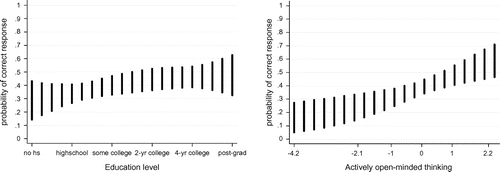
All these relationships supply additional grounds for confidence in the external validity of OSI_2.0. While education and actively open-minded thinking are both integral to OSI, OSI posits a science comprehension capacity that is more specific than, and that does not simply reduce to, either education or AOT. The modest size of the correlations between both education and AOT, on the one hand, and OSI_2.0, on the other, show that the former are failing to account fully for the latent combination of knowledge, skills, and motivations that is being measured by the latter. Further, the superiority of OSI_2.0 in predicting covariance detection supports the inference that the capacities uniquely accounted for by OSI_2.0 are exactly the ones that figure in proficiencies distinctive of OSI.
6. Covariates
Normally distributed in the study sample (Figure ), OSI_2.0 scores had a small correlation (r = 0.08, p < 0.01) with being male, and only a slightly larger one (r = 0.18, p < 0.01) with being white. The signs of the correlations are consistent with relationships between gender and race on the one hand, and performance on standardized measures of scientific literacy, mathematical skill, and performance on critical reasoning measures, on the other. The size of the effects, however, is smaller than ones typically observed (e.g. Frederick Citation2005; Kahan Citation2013; Roth et al. Citation2001).
Figure 6. Distribution of OSI_2.0 scores.
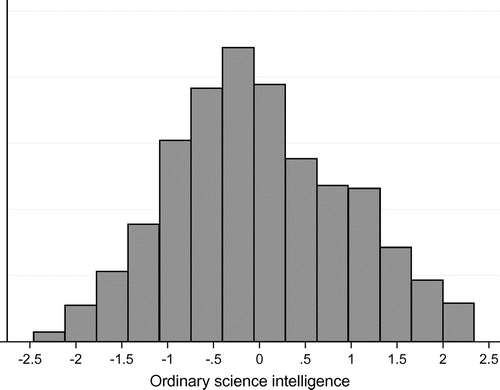
There was no meaningful correlation (r = 0.01, p = 0.59) between OSI_2.0 and political orientation as measured by a scale (α = 0.78) that combines self-reported liberal–conservative ideology and partisan self-identification. This is consistent with findings that show that objective measures of reasoning proficiency, as opposed to various self-report ones that are in fact weaker predictors of forms of behavior associated with critical reasoning (Liberali et al. Citation2011; Toplak, West, and Stanovich Citation2011), do not meaningfully vary in relation to political outlooks in general population studies (Baron Citation2015; Kahan Citation2013; Kahan et al. Citation2013).
7. Relationship to ‘belief in evolution’ and ‘belief in the big bang’
The value of a valid and reliable public science comprehension measure ultimately turns on what one can do with it. The relationship of acceptance or non-acceptance of evolution to public science comprehension is an issue of considerable scholarly interest (e.g. Miller, Scott, and Okamoto Citation2006). Use of OSI_2.0 suggests that for members of a general public sample in the US, at least, variance in ‘belief in’ evolution does not validly convey information about science comprehension. On the contrary, OSI_2.0 reveals that items assessing acceptance of evolution and related facts are biased measures of that disposition.
Among the NSF Indicators ‘basic facts’ items are ones relating to the role of evolution in the natural history of human beings and another relating to a popular rendering of the inflationary theory of the universe in cosmology:
EVOLUTION. Human beings, as we know them today, developed from earlier species of animals (True/false).
BIGBANG. The universe began with a huge explosion (True/false).
‘Differential item function’ is an IRT technique used to assesses whether indicators of a latent variable display the same measurement properties across distinct subpopulations. It is used in the field of standardized testing to identify questions that are ‘culturally biased,’ which refers not to group animus on the part of the test designers or administrators but rather to the systematic misestimation of the aptitude of a group of test takers in whom responses to those questions do not bear the requisite relationship to the aptitude or skill being assessed (Osterlind and Everson Citation2009).
DIF analysis shows that the Indicators’ EVOLUTION and BIGBANG items are both biased with respect to individuals who display a relatively high degree of religiosity (Figure , top two panels). As the form of science comprehension measured by OSI_2.0 increased, the probability of a correct response to the items increased substantially more for relatively non-religious individuals than for relatively religious ones. The impact was especially dramatic for EVOLUTION: in relation to that question, increasing OSI_2.0 scores had no effect on the probability of answering the question correctly for individuals of modestly high religiosity. In other words, for such individuals, the item is simply an invalid indicator of OSI. On BIGBANG, the increasing probability of a correct response that one expects to see in such an indicator was present for both relatively religious and non-religious individuals. Nevertheless, the slope was substantially steeper for the latter (Figure ).
Figure 7. Differential item function analysis for NSF Indicator EVOLUTION and BIGBANG items.
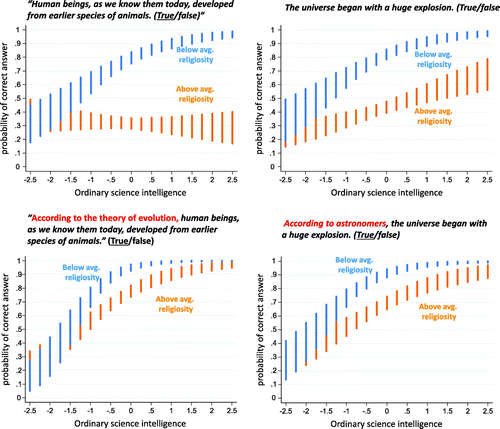
It bears emphasis that this differential is not a consequence of any relationship between religiosity and science comprehension. There is in fact a small negative correlation (r = −0.18, p < 0.01) between OSI_2.0 and religiosity, which was measured with a scale that combined self-reported church attendance, frequency of prayer, and declared ‘importance’ of religion (α = 0.86). But the DIF analysis examines the discrepancy in the probability of responding correctly among religious and non-religious test takers who have the same OSI level. Accordingly, an otherwise valid science-comprehension test containing these items would systematically underestimate the OSI of non-religious respondents relative to religious ones of the same OSI capacity.
A similar analysis was performed on variants of EVOLUTION and BIGBANG. The alternative items contained introductory clauses that expressly identified the asserted proposition with scientific understandings of the natural history of human beings and the expansionary theory of the universe (National Science Board Citation2014). One might expect these variants to mitigate the tension between affirmative responses and religious beliefs inconsistent with the asserted proposition. In fact, for both items, the differential between religious and non-religious respondents was substantially reduced (Figure ).
The conclusion that EVOLUTION and BIGBANG do not validly measure OSI but rather some aspect of personal identity relating to religiosity was also supported by common factor analysis. As indicated in Section 4.1, the covariance structure for the 18 OSI_2.0 items suggests that the scale is reasonably understood as measuring a single, unidimensional latent variable (Table , I). When the standard NSF Indicator EVOLUTION and BIGBANG items were included, however, factor analysis suggested that the resulting covariance structure was better explained by positing two factors: one consisting of the 18 OSI_2.0 items, and the other of EVOLUTION and BIGBANG (Table , II). In an additional analysis, EVOLUTION and BIGBANG loaded along with the religiosity items on a factor that was separate from the factor explaining the covariance structure of the OSI_2.0 items (Table , III).
This analysis suggests that, for US respondents at least, the standard EVOLUTION and BIGBANG items could be viewed along with the religiosity items as indicators of a latent religiosity variable, albeit one with a reliability score (α = 0.85) not materially different from the one formed by the three religiosity items alone.
This result bolsters the conclusion of Roos (Citation2012), who reports that the EVOLUTION and BIGBANG items, along with another on continental drift and another on literal belief in the Bible, cohered as a factor separate from the other NSF Indicator items in a structural equation model. They are likewise consistent with Rissler, Duncan, and Caruso (Citation2014), who report finding that religiosity measures better predict ‘acceptance of evolution’ among university students than did measures of educational attainment.
Similar analyses were performed substituting the alternative EVOLUTION and BIGBANG items. In a factor analysis, the alternative EVOLUTION and BIGBANG variants still loaded more heavily on a second factor distinct from the one formed by the 18 OSI_2.0 item (Table , IV). But when the three religiosity items were added (Table , V), the variants displayed only modest loading coefficients on the second, religiosity factor (β = 0.30 for EVOLUTION; β = 0.36 for BIGBANG), and the aggregate religiosity scale including these items displayed lower reliability (α = 0.80) than did the three-item scale formed by the church-attendance, frequency-of-prayer, and importance-of-religion items alone. This result supports the conclusion that the reworded items are not valid indicators of either science comprehension or religiosity, although they might well function as indicators of the former if administered to subpopulations of relatively nonreligious individuals only.
The validity of the EVOLUTION and BIGBANG items as indicators of science comprehension when administered to members of the general population in the US has provoked considerable controversy (Mooney Citation2010). The analysis of these items in relation to OSI_2.0 supports the NSF’s recent decision to exclude these items when assessing performance on the Indicators’ science literacy battery over time and across societies (National Science Board Citation2014). The OSI_2.0 scale similarly omits them so as to avoid the bias that their administration to a US general population sample would entail.
The proportion of ‘false’ responses to EVOLUTION and BIGBANG is lower in European samples (Miller, Scott, and Okamoto Citation2006). An appropriately cross-culturally validated version of OSI_2.0 could be used to determine whether these items are in fact free of cultural bias when administered to such samples, and whether they otherwise make a sufficient contribution to discernment of science comprehension to justify including them in the scale when it is administered to residents of those countries. A cross-culturally valid OSI_2.0 could also be used to assure that other items bear the same relationship to the latent science comprehension disposition across and within national samples, an issue that has not been meaningfully investigated in connection with current science-literacy assessments (Pardo and Calvo Citation2004; Solano-Flores and Nelson-Barber Citation2001).
8. Relationship to global warming beliefs and risk perceptions
There is also considerable interest in whether political conflict over human-caused global warming is attributable to deficiencies in public science literacy (Miller Citation2004) or some related form of critical reasoning (Weber Citation2006). Performance on OSI_2.0 suggests the answer, at least in the US, is No. Professions of belief in human-caused climate change and perceptions of the risks it poses are more convincingly viewed as indicators of an aspect of personal identity associated with individuals’ political outlooks than as indicators of science comprehension.
When study subjects were asked whether human activity is creating global warming, the probability that they would respond affirmatively displayed only a weak relationship to their OSI_2.0 scores. The probability of such a response is 44% (±4%, 0.95 LC) for an individual with a mean OSI_2.0 score. That probability dips down only modestly to 40% (±5%, 0.95 LC), and rises only modestly to 52% (±4%, 0.95 LC), for individuals with scores one standard deviation above and below the mean, respectively (Figure ). This relative insensitivity to differences in OSI_2.0 scores contrasts dramatically with the sensitivity displayed by valid OSI_2.0 indicators (Figure ).
Figure 8. Item-response (and DIF) for ‘belief in’ human-caused global warming.
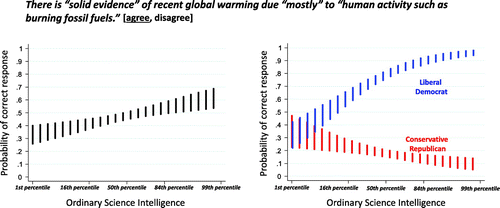
More importantly, this item bears a radically different relationship to OSI for individuals of opposing political outlooks. Whereas the probability of belief in human-caused global warming increases slightly for relatively left-leaning individuals, the probability is unaffected for right-leaning ones as OSI_2.0 scores increase. As a result, consistent with previous studies of the relationship between science comprehension and global warming risk perceptions (Hamilton, Cutler, and Schaefer Citation2012; Kahan et al. Citation2012), higher OSI_2.0 scores magnify polarization.
Factor analysis also supports the inference that global warming beliefs and risk perceptions are more convincingly treated as indicators of a latent identity that features a left–right political orientation. When the ‘belief in’ climate change item, a global warming risk-rating item, and the two political outlook measures (liberal–conservative ideology and partisan self-identification) are analyzed along with the 18 OSI_2.0 items, the covariance structure is best explained by positing two factors: one consisting of the political outlook measures and the global-warming items, and the other by the OSI_2.0 items (Table , VI). Consistent with this analysis, aggregating the global warming items with the political outlook measures forms an even more reliable scale of subjects’ latent political orientation (α = 0.84) than do the two political outlook measures by themselves. In sum, how members of the US general public respond to items assessing their acceptance of human-caused global warming, like ones assessing their acceptance of human evolution, are more convincingly viewed as indicators of who they are, culturally speaking, than of what they know about science.
This conclusion is of obvious significance for assessing what sorts of science communication are likely to be efficacious for dispelling political conflict over climate change in the US (Kahan Citation2015a). Because the ordinary citizens most able to comprehend scientific evidence are the most politically polarized, it makes little sense to believe disseminating more information will promote convergence of understanding; what is needed are forms of communication, and forms of political deliberation, that disentangle positions on climate change from the cultural meanings that motivate individuals with competing identities to credit such information in opposing patterns (Kahan Citation2015b). Evidence that similar dynamics of cultural cognition account for conflict over evidence of climate change in other democratic societies (Aasen Citation2015; Shi, Visschers, and Siegrist Citation2015) suggests the value of using OSI_2.0 to investigate whether differences in science comprehension are as inconsequential to political conflict in those societies as they are in the US.
9. What next?
The scale-development process that generated OSI_2.0 remains ongoing. Future research will include additional forms of behavioral validation, including assessments of the scale’s power to predict individuals’ capacity to recognize and make appropriate use of valid science in various real-world domains.
Efforts to develop a short-form version of OSI_2.0 and to cross-culturally validate the scale are also anticipated. Item response theory, a scale-development and scoring tool that has been oddly neglected in the study of public science comprehension, is well suited to both of these tasks. The information IRT supplies on the relative contribution that individual items make to measurement precision at various levels of a latent trait can be sued both to economize on items (Weller et al. Citation2012) and to test for measurement commensurability across different test populations (Richardson and Coates Citation2014).
10. Conclusion: incremental progress dominates standing still
The scale development exercise that generated OSI_2.0 is offered as an admittedly modest contribution to an objective of grand dimensions. How ordinary citizens come to know what is collectively known by science is simultaneously a mystery that excites deep scholarly curiosity and a practical problem that motivates urgent attention by those charged with assuring democratic societies make effective use of the collective knowledge at their disposal. An appropriately discerning and focused instrument for measuring individual differences in the cognitive capacities essential to recognizing what is known to science is essential to progress in these convergent inquiries.
The claim made on behalf of OSI_2.0 is not that it fully satisfies this need. It is presented instead to show the large degree of progress that can be made toward creating such an instrument, and the likely advances in insight that can be realized in the interim, if scholars studying risk perception and science communication make adapting and refining admittedly imperfect existing measures, rather than passively employing them as they are, a routine component of their ongoing explorations.
Disclosure statement
No potential conflict of interest was reported by the author.
Notes
1. To facilitate such research, the data reported in this study, along with codebook and user guide, can be downloaded from http://www.culturalcognition.net/osi_2/.
References
- Aasen, M. 2015. “The Polarization of Public Concern about Climate Change in Norway.” Climate Policy advance on-line publication. doi:10.1080/14693062.2015.1094727.
- Allum, N., P. Sturgis, D. Tabourazi, and I. Brunton-Smith. 2008. “Science Knowledge and Attitudes across Cultures: A Meta-analysis.” Public Understanding of Science 17: 35–54.10.1177/0963662506070159
- Ansolabehere, Stephen, and Douglas Rivers. 2013. “Cooperative Survey Research.” Annual Review of Political Science 16 (1): 307–29.
- Arkes, H. R., and A. R. Harkness. 1983. “Estimates of Contingency between Two Dichotomous Variables.” Journal of Experimental Psychology: General 112: 117–135.10.1037/0096-3445.112.1.117
- Baron, J. 1993. “Why Teach Thinking? An Essay.” Applied Psychology 42: 191–214.10.1111/apps.1993.42.issue-3
- Baron, J. 2015. “Supplement to Deppe et al. (2015).” Judgment and Decision Making 10 (4): 1–2.
- Bartholomew, David J., M. Knott, and Irini Moustaki. 2011. Latent Variable Models and Factor Analysis: a Unified Approach. 3rd ed, Wiley series in probability and statistics. Chichester, West Sussex: Wiley.
- DeMars, C. 2010. Item Response Theory. Oxford: Oxford University Press.10.1093/acprof:oso/9780195377033.001.0001
- DeVellis, R. F. 2012. Scale Development: Theory and Applications. Thousand Oaks, CA: Sage.
- Dewey, J. 1910. “Science as Subject-matter and as Method.” Science 31: 121–127.10.1126/science.31.787.121
- Embretson, S. E. 2010. Measuring Psychological Constructs: Advances in Model-based Approaches. Washington, DC: American Psychological Association.10.1037/12074-000
- Embretson, S. E., and S. P. Reise. 2000. Item Response Theory for Psychologists. Mahwah, NJ: L. Erlbaum Associates.
- Frederick, S. 2005. “Cognitive Reflection and Decision Making.” Journal of Economic Perspectives 19: 25–42.10.1257/089533005775196732
- Gauchat, G. 2011. “The Cultural Authority of Science: Public Trust and Acceptance of Organized Science.” Public Understanding of Science 20: 751–770.10.1177/0963662510365246
- Guterbock, Thomas M, Scott Keeter Holbrook, Susan Losh, Jeff Mondak, Bryce Reeve, Deborah Rexrode, David Sikkink, Sally Stares, Roger Tourangeau, and Chris Toumey. 2011. Measurement and Operationalization of the ‘Science in the Service of Citizens and Consumers' Framework. Center for Survey Research, University of Virginia, Charlottesville, VA. Available at http://www.coopercenter.org/sites/default/files/publications/Instrumentation%20Workshop%20Final%20Report.2011-01-31.pdf
- Hamilton, L. C. 2011. “Education, Politics and Opinions about Climate Change Evidence for Interaction Effects.” Climatic Change 104: 231–242.10.1007/s10584-010-9957-8
- Hamilton, L. C., M. J. Cutler, and A. Schaefer. 2012. “Public Knowledge and Concern about Polar-region Warming.” Polar Geography 35: 155–168.10.1080/1088937X.2012.684155
- Haran, Uriel, Ilana Ritov, and Barbara A. Mellers. 2013. “The Role of Actively Open-minded Thinking in Information Acquisition, Accuracy, and Calibration.” Judgment and Decision Making 8: 188–201.
- Hoppe, E. I., and D. J. Kusterer. 2011. “Behavioral Biases and Cognitive Reflection.” Economics Letters 110: 97–100.10.1016/j.econlet.2010.11.015
- Kahan, D. M. 2013. “Ideology, Motivated Reasoning, and Cognitive Reflection.” Judgment and Decision Making 8: 407–424.
- Kahan, D. M. 2015a. “Climate-Science Communication and the Measurement Problem.” Political Psychology 36: 1–43.10.1111/pops.v36.S1
- Kahan, D. M. 2015b. “What is the “Science of Science Communication”?” Journal of Science Communication 14: 1–12.
- Kahan, D. M., E. Peters, M. Wittlin, P. Slovic, L. L. Ouellette, D. Braman, and G. Mandel. 2012. “The Polarizing Impact of Science Literacy and Numeracy on Perceived Climate Change Risks.” Nature Climate Change 2: 732–735.10.1038/nclimate1547
- Kahan, D. M., E. Peters, E. Dawson, and P. Slovic. 2013. “Motivated Numeracy and Enlightened Self Government.” Cultural Cognition Project Working Paper No. 116, Available at http://papers.ssrn.com/sol3/papers.cfm?abstract_id=2319992.
- Liberali, J. M., V. F. Reyna, S. Furlan, L. M. Stein, and S. T. Pardo. 2011. “Individual Differences in Numeracy and Cognitive Reflection, with Implications for Biases and Fallacies in Probability Judgment.” Journal of Behavioral Decision Making 25:361–381.
- Lipkus, I. M., G. Samsa, and B. K. Rimer. 2001. “General Performance on a Numeracy Scale among Highly Educated Samples.” Medical Decision Making 21: 37–44.10.1177/0272989X0102100105
- Miller, J. D. 2004. “Public Understanding of, and Attitudes toward, Scientific Research: What We Know and What We Need to Know.” Public Understanding of Science 13: 273–294.10.1177/0963662504044908
- Miller, J. D., E. C. Scott, and S. Okamoto. 2006. “Science Communication: Public Acceptance of Evolution.” Science 313: 765–766.10.1126/science.1126746
- Mooney, C. 2010. “Why Did NSF Cut Evolution and the Big Bang from the 2010 Science and Engineering Indicators?” Discover, April 9. http://tinyurl.com/nmhfv5p.
- Morizot, J., A. T. Ainsworth, and S. P. Reise. 2007. “Toward Modern Psychometrics: Application of Item Response Theory Models in Personality Research.” In Handbook of Research Methods in Personality Psychology, edited by R. W. Robins, R. C. Fraley, and R. F. Krueger, 407–423. New York, NY: Guilford.
- National Science Board. 2014. Science and Engineering Indicators. Arlington, VA: National Science Foundation. 2010.
- Osterlind, S. J., and H. T. Everson. 2009. Differential Item Functioning. Thousand Oaks, CA: Sage.10.4135/9781412993913
- Pardo, R., and F. Calvo. 2004. “The Cognitive Dimension of Public Perceptions of Science: Methodological Issues.” Public Understanding of Science 13: 203–227.10.1177/0963662504045002
- Peters, E., D. Vastfjall, P. Slovic, C. K. Mertz, K. Mazzocco, and S. Dickert. 2006. “Numeracy and Decision Making.” Psychological Science 17: 407–413.10.1111/j.1467-9280.2006.01720.x
- Pew Research Center for the People & the Press. 2013. Public’s Knowledge of Science and Technology. Washington, DC: Pew Research Center.
- Reyna, Valerie F., Wendy L. Nelson, Paul K. Han, and Nathan F. Dieckmann. 2009. “How Numeracy Influences Risk Comprehension and Medical Decision Making.” Pscyh. Bulletin 135: 943–973.10.1016/j.lindif.2007.03.011
- Richardson, S., and H. Coates. 2014. “Essential Foundations for Establishing Equivalence in Cross-National Higher Education Assessment.” Higher Education 68: 825–836.10.1007/s10734-014-9746-9
- Rissler, L. J., S. I. Duncan, and N. M. Caruso. 2014. “The Relative Importance of Religion and Education on University Students’ Views of Evolution in the Deep South and State Science Standards across the United States.” Evolution Education Outreach 7: 1–17.
- Roos, J. M. 2012. “Measuring Science or Religion? A Measurement Analysis of the National Science Foundation Sponsored Science Literacy Scale 2006–2010.” Public Understanding of Science 23: 797–813.
- Roth, P. L., C. A. Bevier, P. Bobko, F. S. Switzer, and P. Tyler. 2001. “Ethnic Group Differences in Cognitive Ability in Employment and Educational Settings: A Meta-analysis.” Personnel Psychology 54: 297–330.10.1111/peps.2001.54.issue-2
- Shi, J., V. H. M. Visschers, and M. Siegrist. 2015. Risk Analysis 35: 2183–2201.
- Solano-Flores, G., and S. Nelson-Barber. 2001. “On the Cultural Validity of Science Assessments.” Journal of Research in Science Teaching 38: 553–573.10.1002/(ISSN)1098-2736
- Stanovich, K. E. 2009. What Intelligence Tests Miss: The Psychology of Rational Thought. New Haven, CT: Yale University Press.
- Stanovich, K. E. 2011. Rationality and the Reflective Mind. New York, NY: Oxford University Press.
- Stocklmayer, S. M., and C. Bryant. 2012. “Science and the Public – What Should People Know?” International Journal of Science Education, Part B 2 (1): 81–101.10.1080/09500693.2010.543186
- Toplak, M., R. West, and K. Stanovich. 2011. “The Cognitive Reflection Test as a Predictor of Performance on Heuristics-and-Biases Tasks.” Memory & Cognition 39: 1275–1289.
- Toumey, C. 2011. “Science in the Service of Citizens and Consumers.” Nature Nanotechnology 6: 3–4.10.1038/nnano.2010.263
- Weber, E. 2006. “Experience-Based and Description-Based Perceptions of Long-Term Risk: Why Global Warming Does Not Scare Us (Yet).” Climatic Change 77: 103–120.10.1007/s10584-006-9060-3
- Weller, J. A., N. F. Dieckmann, M. Tusler, C. Mertz, W. J. Burns, and E. Peters. 2012. “Development and Testing of an Abbreviated Numeracy Scale: A Rasch Analysis Approach.” Journal of Behavioral Decision Making 26: 198–212.