ABSTRACT
Anecdotal evidence suggests that harsh social conditions in the road haulage industry are having an impact on transport crime. This paper analyses transport crime, and demonstrates how to use a combination of official statistics and crowdsourced data in the process. A hierarchical regression analysis was applied to investigate the relations among different factors in order to predict transport crime threats. A secondary data set on transport crime from the Swedish Police was combined with primary crowdsourced data from volunteer observations of trucks in Sweden from both high-wage and low-wage countries. The findings imply that transportation is more vulnerable to antagonistic threats in geographical areas where the low-wage hauliers operate more frequently. For policymakers and practitioners, these findings provide useful guidance for the planning of security measures. To the authors’ knowledge, this paper is the first exploratory study of its kind that uses a combination of official statistics and crowdsourced data.
Introduction
On a November night in 2012 a group of perpetrators stole diesel in Västerås, Sweden. The thieves were caught in the act and an investigation was initiated. It revealed that the Romanian haulage company that employed the drivers was contracted to drive at a rate that did not even cover the cost of diesel – much less salaries and other expenses. (Swedish Television, ‘Uppdrag granskning’ [‘Mission Investigate’], March 2013)
This anecdote addresses two timely topics in contemporary logistics management: transport crime and the social sustainability of supply chains. Despite over two decades of research on supply chain security risks, Zailani et al. (Citation2015, 653) state: ‘Although many companies are devoting increased resources and attention to security efforts, little guidance is available to firms seeking to minimise their exposure to unexpected and potentially damaging or disruptive occurrences affecting their supply chains.’ Urciuoli and Hintsa (Citation2017) confirm that previous research lacks managerial guidance on how to deal with security issues. In almost every supply chain, road haulage (in particular parking) is the most vulnerable link to antagonistic threats (Ekwall and Lantz Citation2013) and socially unsustainable practices (Hilal Citation2008; European Commission Citation2014). Huq, Chowdhury, and Klassen (Citation2016, 20) define social sustainability as considering ‘the health and wellbeing of people in the supply chain and impact on society’. Following the recent liberalisation of the European road transport market (European Commission Citation2014), social sustainability in supply chains, particularly in road haulage, is gaining increased attention from scholars, policymakers and practitioners (Hilal Citation2008; Kummer, Dieplinger, and Fürst Citation2014; Piecyk and Björklund Citation2015).
The security and safety of road hauliers and truck drivers have also gained significant attention from practitioners (i.e. IRU Citation2008; Nilsson Citation2013). A closely related issue is diesel theft. It strikes the road hauliers in two ways: Firstly, the truck from which the diesel is stolen needs to be repaired and refilled; secondly, it alters the rules of fair competition in the industry (EUROPOL Citation2010). Diesel theft is the most common transport crime and has become a plague for the entire road haulage industry (Lindén Citation2013).
The European media, haulage associations, unions and to some extent academic and industry research are increasingly reporting on truck drivers who are facing very harsh conditions (Hilal Citation2008; Steen Jensen et al. Citation2014; COWI Citation2015). Some hauliers, based on ‘gut feeling’ or experience, prohibit their drivers from resting at big truck stops with large numbers of international trucks. While previous research has focused on analysing official statistics (e.g. Ekwall and Lantz Citation2013), policymakers have been promoting ‘secure parking’ areas (European Commission Citation2011). The statistics used have been from historical data on thefts, which provide limited guidance for practitioners and policymakers. The social sustainability of road transportation has received scant research attention, and the only input to the debate is limited to anecdotal evidence and wage statistics, the latter of which are frequently uncertain in determining whether the salaries surveyed are before or after taxes, or whether they are with or without allowances (e.g. COWI Citation2015).
Is the lack of social sustainability in logistics, exemplified by poor driver conditions (Hilal Citation2008; COWI Citation2015), related to transport crime? The answer would potentially yield important implications for transport and logistics managers, policymakers and research on both supply chain management and criminology. For this, statistics on various types of road hauliers are needed. The issue of limited statistics on road haulage has been frequently discussed (McKinnon and Leonardi Citation2009; Zmud et al. Citation2013; van den Engel, Francke, and Burgess Citation2014). Zmud et al. (Citation2013) and Bonnel et al. (Citation2009) outline several methods to collect data, but the existing research does not address crowdsourcing as a data-gathering method that can complement the limited existing statistics. Saxton, Oh, and Kishore (Citation2013) define crowdsourcing ‘as a type of sourcing model that lies at the intersection of outsourcing and sophisticated internet technologies’ (2). Crowdsourcing has gained considerable attention in information systems research in recent years (Schlagwein and Bjørn-Andersen Citation2014; Aitamurto, Landemore, and Galli Citation2017). In addition to various other application areas, crowdsourcing enables researchers to gain access to new data sets of previously inaccessible richness.
Given the lack of guidance for managers, the lack of insights into transport crime, and the lack of statistics that can aid policymakers, the purpose of this paper is twofold: (1) to complement official statistics with crowdsourced data collection in logistics research in order to facilitate effective policy-making, and (2) to explore the incidents of transport crime using crowdsourced data on truck positions. The aim is to contribute to theory that can aid practitioners and policymakers (Zailani et al. Citation2015; Urciuoli and Hintsa Citation2017). Criminologists have been using census data to predict crime patterns for decades (e.g. Block Citation1979; Cohen and Felson Citation1979). The originality of the present study is rooted in its innovative crowdsourcing approach to obtain a new type of data that together with data from a more traditional source (i.e. police statistics) would enable prediction.
The rest of this introductory section explains transport crime and reflects upon the ethical considerations of the study. As the study involves human beings (the drivers in the observed trucks), analysing how this relates to crime is a sensitive matter not to be taken lightly. A framework is presented that establishes the constructs of the paper, followed by the proposed hypotheses. The data and methodology section includes an explanation of the data sources and the analysis. The results of the statistical analysis are then presented followed by a discussion and conclusions.
Transport crime
Transport crimes can be divided into three distinctively different categories, as suggested by the Swedish Police (Persson Citation2015):
Diesel theft,
Theft from trucks or trailers,
Theft of trucks or trailers.
Diesel theft, as stated in the introduction, is by far the most common transport crime. It is a rather easy crime to commit, with a minimum of tools necessary and a product – diesel – which is both easy to sell and beneficial to use (many owner-operators and drivers get paid per kilometre driven, including compensation to pay for diesel). It should be noted that diesel is stolen from an array of different actors, including hauliers, farmers and municipalities and that the official records do not specify the category of victim.
Theft from the contents of trucks or trailers is easy to commit, even though it may be slightly more complicated than diesel theft. Many products have limited protection as many trailers only have soft covers.
Theft of trucks and trailers is a rather advanced crime in comparison with the other two. There are a few cases where a truck is stolen after being left with the keys in the ignition, but the rapid technological development with immobilisers and similar devices has made it difficult or even impossible for the less advanced thief to steal the truck itself in most cases (see e.g. van Ours and Vollaard Citation2016). Thus, in general terms, it requires careful planning and advanced equipment to steal a truck and move it and/or the trailer to a market (e.g. Africa or Asia) where it can be sold.
Ethical considerations
In short, a crime occurs when a motivated perpetrator finds an opportunity (Felson and Clarke Citation1998). Causality is a main concern of criminologists, but establishing casual pathways is a complicated process. As criminals are difficult to study per se, controlled experiments are impossible for most types of crimes. Hence, studying past events to predict and prevent future crimes is inevitable. As the transport industry has a hard time protecting all disparate vehicles on the roads and on parking lots, small- and medium-sized enterprises (SMEs) (road hauliers) in particular are suffering from transport crime. Thus, small pieces of this complex picture need to be added together to shed light on if social conditions, in particular, can be linked to crimes.
Independent of nationality, most drivers are satisfied with their jobs in general (Prockl et al. Citation2017). Yet, previous research and recent investigations show that the international road haulage industry does have serious social sustainability issues (Hilal Citation2008; COWI Citation2015; BBC Citation2017).
In this paper the authors aim to achieve a balance between the importance of social sustainability, the impact of transport crime, and the ethical concerns of comparing the observed presence of trucks associated with relatively high salaries (such as Danish or Swedish trucks) and trucks registered in low-wage countries (such as Bulgaria or Romania). Hence, the authors would like to emphasise that the purpose is by no means to stigmatise a group of drivers, some of which are already facing harsh conditions, but rather to put the spotlight on the negative externalities of freight transportation as well as the poor social conditions.
In transport, the opportunity for a motivated perpetrator to commit a crime is more or less always there due to the distributed nature of the industry, which further motivates the study undertaken.
Framework
As alluded to in the introduction, one of the gaps in the transport literature is that a theoretical framework has yet to be articulated that explains why the lack of social sustainability of hauliers should affect transport crimes. While scholars have noted that drivers suffer when insufficient social regulations are in place (Belzer Citation2000; Hilal Citation2008), industry magazines have pointed towards hauliers from low-wage countries (Nilsson Citation2013). This anecdotal evidence has yet to be empirically examined.
Social sustainability
Triggered by accidents and bad press, many companies are paying increasing attention to social sustainability, focusing on aspects such as the societal impact of operations and human and labour rights (Marshall et al. Citation2016). The social sustainability review of supply chain and logistics literature by Abbasi (Citation2017) indicates that the governance complexity of social sustainability in SMEs is a challenge when attempting to achieve social sustainability in supply chains. Another review by Nakamba, Chan and Sharmina (Citation2017), found that few studies are considering the social practices, particularly at the micro level and in the context of small and medium-sized enterprises. They also found that previous literature has focused on the buyers’ perspective rather than on the supply side (Nakamba, Chan, and Sharmina Citation2017).
Though many reports have looked at the working conditions of drivers, the supply chain management and logistics literature has mainly focused on supply chain workers in production or at the point of origin (e.g. Huq, Chowdhury, and Klassen Citation2016). Studies on driver conditions are predominantly based on US drivers (Belzer Citation2000; Belman, Lafontaine, and Monaco Citation2005), with few studies investigating drivers in Europe (Prockl et al. Citation2017). The social challenges of the sector, though, seem to be international phenomena that have been investigated and debated for decades (Burnewicz Citation1998; Belzer Citation2000; Belman, Lafontaine, and Monaco Citation2005; Hilal Citation2008).
Road haulage is a commodity and hauliers in Europe are generally SMEs, often loosely coupled with the shippers or interacting only with an intermediary such as a logistics service provider (LSP) (Nilsson, Sternberg, and Klaas-Wissing Citation2017). The lack of information on the social conditions of road haulage was recently studied by Nilsson, Sternberg, and Klaas-Wissing (Citation2017). They found that driver conditions are in many cases unknown even to the LSP contracting the road haulier.
EU driver working conditions
The European domestic road haulage markets have gradually been deregulated, allowing haulers to operate (under conditions stipulated in the directives Citation92/Citation106/EEC and (EC) Citation1072/Citation2009) in other markets than their country of origin/registration. The ‘new’ EU member states (EU12) have significantly lower labour costs than the ‘old’ EU member states (EU15). As shown in , the salary difference between Sweden and Bulgaria, for example, is almost tenfold and despite salary increases in the EU12 countries, the absolute gap between the EU12 and EU15 remains. This gap has triggered a massive flagging out of trucks from the EU15 to EU12 countries. Kummer, Dieplinger, and Fürst (Citation2014) found that 50% of Austrian truck fleets were flagged out over a 10-year period, at a cost of fiscal losses of €29,702 per driver and €6183 per truck. A clear majority of the foreign trucks in most EU15 countries, in particular Scandinavia, are registered in EU12 countries.
Table 1. Average hourly labour costs in € in Europe for selected countries (Eurostat Citation2017).
Several surveys of EU12 drivers reveal long shifts in the truck. COWI (Citation2015) questioned 168 foreign drivers in Denmark and found that the average driver had been home 6.8 weeks ago, with many drivers being on the road much longer.
On the contrary, Scandinavian drivers are relatively very well paid. Collective agreements ensure that the majority of the road hauliers provide their employees with salaries and benefits (such as insurance, retirement plans and protective clothing) according to the agreements. It should be noted, however, that an unknown portion of the drivers in Scandinavian trucks are employed outside the collective agreements. Anecdotal evidence (published in the local Scandinavian languages) suggests that this unknown portion of drivers come from EU12 countries and are paid on the same level as drivers in those countries (Hultén Citation2017). As can be seen in , a significant difference exists between the EU15 (high wage) and the EU12 (low wage) countries.
A more granular approach than comparing country to country, would be to compare regions in countries. This is not possible, however, as many countries do not issue licence plates that differ from region to region. Yet another more granular approach would be carry out an analysis based on the company level instead of the country level. Again, this is not possible because many trucks lack appropriate company names and/or just display the name of the LSP. Hence, ‘low average labour costs’ is used here as a proxy to categorise the groups that contain drivers facing harsh conditions.
Factors behind crime
As stated in the introduction, establishing causality in criminology is a complicated process. Several theories and models describing causes of delinquency (Hirschi Citation1969) have been proposed over the past century. Cohen and Felson (Citation1979) propose routine activity theory. It shows that the presence of crime can be explained by: (1) a potential offender, (2) a vulnerable target, and (3) the absence of a suitable guardian. Capable guardianship is important in this context because the lack of guardianship directly contributes to opportunities for theft. For example, the likelihood of burglary is predicted to be lower when homeowners are routinely at home most of the time, and vice versa. According to this theory, we also know that: (a) areas where people are not present some hours of the day or night (such as SME haulier parking lots) are vulnerable targets, and (b) the mobility and toolkit of the offender predicts crime rates (i.e. the type of crime the offender is capable of carrying out).
Social bond theory (Hirschi Citation1969) suggests that individuals who have low levels of societal controls are more likely to engage in crime. Similarly, those with a low stake in conformity are expected to engage in more crime (Toby Citation1957). Low-wage drivers are frequently both alienated (alone without a social context in a foreign country for durations of 2 to 6 months) (COWI Citation2015), and live under harsh social conditions (relatively very low wages and rough living conditions in small trucks) (Hilal Citation2008).
The effect of social factors on crime rates are debated in criminology (Kubrin and Weitzer Citation2003).
Social disorganization theory focuses on the effects of ‘kinds of places’ or different types of neighborhoods in creating conditions favorable or unfavorable to crime and delinquency. Social disorganization refers to the inability of a community to realize common goals and solve chronic problems. According to the theory, poverty, residential mobility, ethnic heterogeneity, and weak social networks decrease a neighborhood’s capacity to control the behavior of people in public, and increase the likelihood of crime. (Kubrin and Weitzer Citation2003, 374)
Hypotheses
This paper draws on previous literature on social sustainability and criminology theory to hypothesise on the relation between transport crime and the social sustainability of drivers. Sweden is used as the context and the unit of analysis is the municipality (Sweden has 290 municipalities).
Given the harsh social conditions foreign truck drivers are facing (Hilal Citation2008; COWI Citation2015), we posit these hypotheses that are in line with routine activity theory (Cohen and Felson Citation1979) and to some extent, social disorganisation theory (Kubrin and Weitzer Citation2003):
H1a: The presence of trucks from low-wage countries is positively related to the prevalence of thefts from trucks or trailers.
H1b: The presence of trucks from low-wage countries is positively related to the prevalence of diesel thefts.
Stealing trucks or trailers is a complicated crime, demanding careful planning and advanced logistics (van Ours and Vollaard Citation2016). We posit that low-wage truck drivers largely lack the tools and network needed to have an effect on the prevalence of thefts of trucks or trailers and thus posit that:
H1c: The relation between the presence of trucks from low-wage countries and the prevalence of thefts of (entire) trucks or trailers is weaker than the relation between the presence of trucks from low-wage countries and the prevalence of diesel thefts and the prevalence of thefts from trucks or trailers.
Scandinavian drivers, and most EU15 drivers in general, get to go home frequently, are paid relatively high wages and work in a social context where they have a sense of belonging. According to social bond theory (Toby Citation1957; Hirschi Citation1969), they would be less inclined to engage in theft from vehicles and trailers and diesel thefts. We posit that:
H2: The relation between the presence of trucks with drivers from high-wage countries and the prevalence of transport crimes is weaker than the relation between the presence of trucks with drivers from low-wage countries and the prevalence of transport crimes.
Based on the three different types of transport crime discussed above, H2 is subdivided into three hypotheses:
H2a: The relation between the presence of trucks with drivers from high-wage countries and the prevalence of thefts from trucks or trailers is weaker than the relation between the presence of trucks with drivers from low-wage countries and the prevalence of thefts from trucks or trailers.
H2b: The relation between the presence of trucks with drivers from high-wage countries and the prevalence of diesel thefts is weaker than the relation between the presence of trucks with drivers from low-wage countries and the prevalence of diesel thefts.
H2c: The relation between the presence of trucks with drivers from high-wage countries and the prevalence of thefts of trucks or trailers is weaker than the relation between the presence of trucks with drivers from low-wage countries and the prevalence of thefts of trucks or trailers.
Data and methodology
The approach to this research is mainly quantitative, combining secondary data on diesel thefts from the Swedish Police with a primary crowdsourced data set on truck observations. The usage of secondary data sources has been encouraged by Rabinovich and Cheon (Citation2011), whereas the approach that combines primary crowdsourced data and secondary data in the transport and logistics context is previously unexplored.
Sweden is a sparsely populated country with long trucking distances and strong export dependency, situated in the northern corner of Europe (relatively little amount of transit traffic). It also has extensive social security for its inhabitants, very little poverty and very small income differences, with the median income of all regions in Sweden being between €24,033 and €30,240 (SCB Citation2018). These factors, together with access to data, make Sweden an ideal case to analyse.
Crowdsourcing – ‘The Cabotage study’
What initially started in the spring of 2013 as an initiative to investigate the environmental effects of the European freight deregulation, quickly became one of the largest crowdsourced research studies collecting observations on truck positions at that time – the Cabotagestudien (‘Cabotage study’). The interface of the smartphone app that was used is presented in . Over 4000 volunteers collected data on vehicle positions for a total of 12 weeks (Sternberg et al. Citation2015) divided into 3 periods: spring 2013, autumn 2013, spring 2015. In the final period (spring 2015), both Swedish and foreign trucks observations were collected.
Figure 1. The app, named after the study (Cabotagestudien, available at the App Store and Google Play). Volunteers download the app, register licence plate numbers and add comments on nationality, for example.
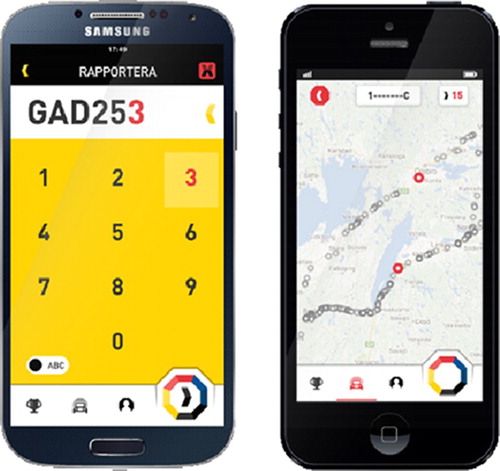
In short, whenever observing, the volunteer drivers would enter the licence plate numbers of either foreign or Swedish trucks (depending on the stage of the study) in the smartphone app. They were instructed not to use the app while driving. Roughly 70% were professional drivers, whereas the remaining 30% represented various other groups. In total, over 485,679 observations of 128,756 trucks were made. In the research presented in this paper, 363,305 observations (made in Sweden) were analysed.
Based on the combination of characters and numbers the volunteer enters, the app suggests a nationality, which was optional to add to an observation. 286,927 of the observations of trucks included nationalities entered by the volunteers, but for 76,378 plates, no nationality was entered. The four most common foreign nationalities in Sweden were Bulgaria (2354 trucks, 39,360 observations), Poland (5022 trucks, 35,312 observations), Estonia (2142 trucks, 28,395 observations) and Lithuania (2557 trucks, 17,940 observations). EU15 countries with more than a handful of registrations, were Germany (2427 trucks, 15,307 observations), Finland (1140 trucks, 6841 observations), Denmark (822 trucks, 4969 observations) and the Netherlands (583 trucks, 4,030 trucks). On the spot-market, the lowest price wins, which is why cabotage (2009) (i.e. domestic transportation by foreign hauliers) is mainly carried out by the hauliers with the lowest cost. Bulgarian trucks typically stay for longer periods and are frequently observed in the weekends, while Danish or German trucks that mainly carry out international transportation, for example, stay for shorter durations.
The validation process (carried out through access to the GPS systems of 500 trucks belonging to 6 different hauliers from Bulgaria, Romania, Germany, Denmark and Sweden) can be found in Sternberg et al. (Citation2015) and the graphical representation of the data set can be found at www.cabotagestudien.se. presents a sample of an observed truck that was also part of the validation set.
Figure 2. The left image shows the movement pattern of a truck, based on volunteer observations. The right image shows the actual movement pattern of the same truck, based on the GPS of the fleet management system of the haulier (Sternberg et al. Citation2015).
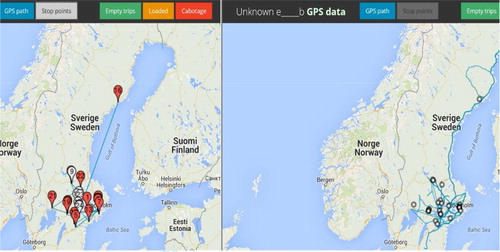
For years, road haulage associations and unions have maintained that foreign hauliers would engage in illegal traffic. In the first weeks of data collection in Sweden, volunteers already were observing many Bulgarian and Romanian trucks on a daily basis. That these trucks carried out illegal traffic was validated later on by in-company data sets, police information and media coverage (Radio Sweden Citation2014; Sternberg et al. Citation2015).
Official statistics
This paper uses two sources of official statistics: the Swedish Police crime statistics related to freight transportation, and the population sizes (inhabitants) of Swedish municipalities from Statistics Swedish (Statistiska centralbyrån). The population size calculation used is that of 31 December 2014.
The official crime statistics are primarily based on victims’ reports of crimes to the police or police reports from investigations. Some crimes are not reported for various reasons. We used data from the police statistics for the 12 weeks corresponding to the crowdsourced data collection periods. The data selected were on the reported thefts of trucks or trailers (crime type 9803), thefts from trucks or trailers (crime type 9804), and diesel thefts (crime type 9821) in Swedish municipalities. The population size was used as a control variable, based on the theory that municipality population size is likely to correlate positively with crime frequency as well as with truck observation frequency.
Statistical analysis
Based on the above outline, six different variables were used in this study:
Population (POP): municipality population size,
X9821: number of reported diesel thefts (Police code),
X9803: number of reported thefts of trucks or trailers (Police code),
X9804: number of reported thefts from trucks or trailers (Police code),
CS_LOW: truck observation frequency from the Cabotage study – all known plates except Swedish, Danish, German and Dutch (i.e. low-wage countries),
CS_HIGH: truck observation frequency from the Cabotage study – Swedish, Finnish, Danish, German and Dutch plates (i.e. high-wage countries)
Hierarchical regression analyses were used to examine the relation between reported thefts and observed trucks while controlling for municipality population size. Hence, the three regression models used in this study were:
The H1c, H2a, H2b and H2c hypotheses were tested by the procedure outlined by Paternoster et al. (Citation1998).
Results
presents the descriptive statistics for the data. All six variables exhibit substantial kurtosis as well as positive skewness. Consequently, the square root was used for all six variables in the regression analyses (Field Citation2013). Most, but not all skewness and excess kurtosis were eliminated by the square root transformation. One reason for this phenomenon is that the theft frequencies are somewhat zero-inflated. However, recent methodological research shows that traditional OLS (Ordinary least squares) methods actually are robust to more extreme normality violations than previously explored, and that such methods work well even if the outcomes are leptokurtic and zero-inflated (Judkins and Porter Citation2016). Hence, the analysis proceeds applying the OLS methodology.
Table 2. Descriptive statistics.
displays the results of a hierarchical regression analysis where the number of reported thefts of trucks or trailers initially was modelled as a function of population size only, and finally as a function of population size and truck observation frequencies. The explained variation increases from 0.417 to 0.500 when the truck observation frequencies are included. Further, CS_HIGH is significantly positively related to X9803 (B = 0.008, p = .013 but there is no significant relation between CS_LOW and X9803. However, the regression coefficients for CS_LOW and CS_ HIGH are not significantly different (Z = 0.263, p = .792).
Table 3. Hierarchical regression – thefts of trucks or trailers.
Hence, hypothesis H2c is not supported.
displays the results of a hierarchical regression analysis where the number of reported thefts from trucks or trailers initially was modelled as a function of population size only, and finally as a function of population size and truck observation frequencies. The explained variation increases from 0.504 to 0.589 when the truck observation frequencies are included. Further, CS_LOW is significantly positively related to X9804 (B = 0.028, p = .006), but there is no significant relation between CS_ HIGH and X9804. In addition, the regression coefficients for CS_LOW and CS_ HIGH are significantly different (Z = 2.057, p = .039). Hence, the hypotheses H1a and H2a are supported:
H1a: The presence of trucks from low-wage countries is positively related to the prevalence of thefts from trucks or trailers.
H2a: The relation between the presence of trucks with drivers from high-wage countries and the prevalence of thefts from trucks or trailers is weaker than the relation between the presence of trucks with drivers from low-wage countries and the prevalence of thefts from trucks or trailers.
Table 4. Hierarchical regression – thefts from trucks or trailers.
displays the results of a hierarchical regression analysis where the number of reported diesel thefts was initially modelled as a function of population size only, and finally as a function of population size and truck observation frequencies. The explained variation increases from 0.463 to 0.512 when the truck observation frequencies are included. Further, CS_LOW is significantly positively related to X9821 (B = 0.041, p = .009), but there is no significant relation between CS_ HIGH and X9821. In addition, the regression coefficients for CS_LOW and CS_ HIGH are significantly different (Z = 2.348, p = .019). Hence, the hypotheses H1b and H2b are supported.
Table 5. Hierarchical regression – diesel thefts.
Hence, hypotheses H1b and H2b are supported:
H1b: The presence of trucks from low-wage countries is positively related to the prevalence of diesel thefts.
H2b: The relation between the presence of trucks with drivers from high-wage countries and the prevalence of diesel thefts is weaker than the relation between the presence of trucks with drivers from low-wage countries and the prevalence of diesel thefts.
Based on the information in , the regression coefficient for CS_LOW in was found marginally significantly different from the regression coefficient for CS_LOW in (Z = 1.802, p = .071) and significantly different from the regression coefficient for CS_LOW in (Z = 2.004, p = .045). Hence, hypothesis H1c is supported.
H1c: The relation between the presence of trucks from low-wage countries and the prevalence of thefts of (entire) trucks or trailers is weaker than the relation between the presence of trucks from low-wage countries and the prevalence of diesel thefts and the prevalence of thefts from trucks or trailers.
displays the correlation coefficients (Pearson’s) between the explanatory variables. As one might expect, they correlate substantially, but the variance inflation factors were all below 10; hence, the multicollinearity in the regression models used here should not be regarded as high (Kutner, Nachtsheim, and Neter Citation2004). Further, the Durbin-Watson statistics were all close to 2, indicating no autocorrelation issues in the above analyses. Even so, the regression results should probably be interpreted with some caution because of the substantial correlation.
Table 6. Correlation coefficients.
Discussion
In a 2012 interview, the CEO of a large European road haulier stated to the authors: ‘Some drivers told us flat out that “If you pay us this low salary we are forced to steal diesel to manage”.’ According to social disorganisation theory (Kubrin and Weitzer Citation2003) and routine activity theory (Cohen and Felson Citation1979), the lower pay and the weak social networks decrease the ‘trucking neighbourhood’s’ capacity to control the behaviour of drivers and increase the likelihood of crime.
Not all foreign drivers have poor working conditions. On the contrary, many international hauliers from EU12 countries care extensively about their drivers, pay decent salaries, ensure proper working conditions, insurance, equipment and ensure that the drivers can return home on a frequent basis (Prockl et al. Citation2017). For these reasons, those trucks are less frequently seen on weekends, particularly when compared to other trucks that are observed over extensive periods in Sweden. Hence, this latter set of observed ‘low-wage drivers’ becomes representative of the foreign hauliers with trucks operating long-term on the domestic market, that is, the less socially sustainable foreign hauliers. The purpose of this paper is by no means to stigmatise EU12 drivers, many already being under severe pressure due to poor working conditions, but rather to highlight the effect of the actual unsustainable practices being the norm for many hauliers (please refer to the ethical considerations in the Introduction).
The results show that easier crimes, such as thefts from trucks and trailers and diesel thefts, are related to the presence of trucks from low-wage countries but not to the presence of trucks from high-wage countries (H2b, H2c, H1a, H1b). This is not surprising considering the major differences between low-wage and high-wage drivers, not only in terms of salary but also in terms of family and social context.
On the contrary, regarding the prevalence of thefts of entire trucks or trailers, there is no significant difference between the presence of trucks from low-wage countries and the presence of trucks from high-wage countries. This is also not surprising, given the lack of motivation and the complexity of the crime. From a routine activity theory viewpoint, the drivers observed neither have the tools necessary to overcome technical barriers (such as immobilisers) nor the logistics capacity to sell entire vehicles.
In every country, police statistics are flawed. Unless a victim of a crime ends up in a hospital, there is always a risk of non-reporting. ‘Though official data severely underestimate crime, they at least provide a rough indicator of trends over time in the volume of several major felonies’ according to Cohen and Felson (Citation1979, 588). In this study, the authors have not tried to estimate non-reporting. A large share of petty crime is not reported, but the non-reporting is assumed in this paper to be evenly distributed throughout Swedish municipalities.
Future work
The scope of the analysis in this paper has been limited to Sweden. Testing of the hypotheses presented in other geographical contexts can yield interesting results. Scandinavia, Benelux and France represent unique markets where domestic hauliers pay salaries multiple times higher than the salaries paid by Bulgarian and Romanian hauliers, for example. Hence, testing of the hypotheses in a country with relatively smaller salary differences would complement the analysis presented in this paper.
Unsustainable practices are widespread in the road haulage industry. Future research into sustainable supply chain management would benefit from acknowledging this. Policymakers and practitioners alike are looking for guidance on how to minimise unsustainable behaviour in the market. This paper has provided several references to ‘grey literature’ and industry magazines that address unsustainable practices. As social media becomes increasingly widespread, so does transparency and Marshall et al. (Citation2016) outline the need for companies to be prepared for sustainability disclosure. Effective routines and tools are needed to gain more sustainability information in outsourced logistics, in particular when it comes to the social sustainability of drivers.
This paper explores the link between poor driver conditions (Hilal Citation2008) and transport crimes. Petty thefts often lead to supply chain disturbances and extensive costs to repair and replace stolen diesel or goods, but that is outside the scope of this paper. Examining these costs using social disorganisation theory may yield interesting insights into the factors that predict transport-related crimes.
According to Cohen and Felson (Citation1979), crime occurs in the absence of a capable guardian. Considering not only the results of research on haulier trucking conditions, but also the recent terror attacks in Europe that used trucks, future research on monitoring of both the truck and driver status and conditions could yield needed policy implications.
Crowdsourcing is a promising approach to data collection in freight transportation because it generates data not available through other approaches. However, several questions remain as to the opportunities and barriers. The generalisability of the approach presented in this paper of combining crowdsourcing and crime records to other contexts remains to be investigated.
Conclusions
Logistics managers and policymakers benefit from the awareness of socially unsustainable practices that are common in the road haulage sector and the indirect costs of such unsustainable practices. Road haulage is vulnerable to motivated offenders due to its decentralised nature; and policymakers and managers lack guidance (Urciuoli and Hintsa Citation2017; Zailani et al. Citation2015). The results of this study have implications for both research and practice. Routine activity theory (Cohen and Felson Citation1979) emphasises thinking of ways to increase guardianship, be that from the police, other truck drivers, or truck stop/farm/hotel/business owners.
Firstly, by analysing a combination of primary and secondary data, the analysis indicates that the presence of low-wage drivers to some extent might predict transport-related crimes in Sweden. This finding confirms and contributes to previous criminological theories (e.g. Cohen and Felson Citation1979), by demonstrating their applicability to road haulage. Many hauliers already have practices in place to avoid large concentrations of trucks from low-wage countries. The empirical analysis of this paper confirms the validity of such practices being able to contribute to security (Urciuoli and Hintsa Citation2017). If the social sustainability of the road haulage sector is allowed to further deteriorate (as the continuous stream of anecdotal evidence suggests), the building of safe parking lots and increased surveillance (capable guardian) will become increasingly important. As the analysis shows, the theft of entire vehicles and/or trailers is unrelated to the presence of trucks that may have drivers who face tough working conditions. That links this research to work in the field of crime economics by van Ours and Vollard (Citation2016), for example. To the authors’ best knowledge, the economics of transport crime in freight transportation has received no attention and is a field that could potentially yield further interesting insights for theory and supply chain management/transport practice.
Secondly, this research is the very first attempt to combine official statistics (secondary data) with primary crowdsourced data. Crowdsourcing represents an opportunity of unprecedented empirical data access and potentially, massive industry involvement as in the case of the ‘Cabotage study’. The results indicate that combining primary crowdsourced data with secondary data is a viable path forward that can enhance future policy-making and managerial practice (Zailani et al. Citation2015). Further research is needed on crowdsourcing methods in logistics and transportation that can address in-depth data collection and crowd management in the logistics and transport area.
Thirdly, this study contributes to the limited body of research on the social sustainability of supply chain, SME suppliers (Nakamba, Chan, and Sharmina Citation2017). Social sustainability in road haulage is increasingly becoming an issue and the trend of flagging out truck fleets to low-cost countries may further accelerate the sustainability problems (Hilal Citation2008, Kummer, Dieplinger, and Fürst Citation2014). The fragmented structure of the industry and the lack of transparency makes monitoring difficult (Nilsson, Sternberg, and Klaas-Wissing Citation2017). This research highlights the relation between negative externalities and unsustainable supply chain practices, calling for further study of transparency and disclosure in the road haulage industry (Marshall et al. Citation2016).
Acknowledgements
The authors would like to thank the three anonymous reviewers for their insightful comments and suggestions for significantly improving the manuscript. We also offer our gratitude to the changes suggested by Prof. Meltem Denizel at Iowa State University. The authors are grateful to all the volunteers and organisations (e.g. 3F and DTL in Denmark) that in different ways supported this study. Particular gratitude goes to the Foundation of Charles and Elin Lindley for financing the programming of the system. We are grateful for all the help and data we have received from the Swedish Police. Finally, we would like to thank our programmer and data scientist, Michal Filipiak (Lund University) for development and data management.
Disclosure statement
No potential conflict of interest was reported by the authors.
References
- Abbasi, Maisam. 2017. “Towards Socially Sustainable Supply Chains – Themes and Challenges.” European Business Review 29 (3): 261–303. doi: 10.1108/EBR-03-2016-0045
- Aitamurto, Tanja, Hélène Landemore, and Jorge Saldivar Galli. 2017. “Unmasking the Crowd: Participants” Motivation Factors, Expectations, and Profile in a Crowdsourced Law Reform.” Information, Communication & Society 20 (8): 1239–1260. doi:10.1080/1369118X.2016.1228993.
- BBC. 2017. “Ikea Drivers Living in Trucks for Months.” Accessed 15 March. http://www.bbc.com/news/business-39196056.
- Belman, Dale, Francine Lafontaine, and Kristen A. Monaco. 2005. “Truck Drivers in the Age of Information: Transformation Without Gain.” In Trucking in the Age of Information, edited by Dale Belman, and Chelsea C. White, 183–212. Burlington, VT: Ashgate.
- Belzer, Michael H. 2000. Sweatshops on Wheels: Winners and Losers in Trucking Deregulation. New York: Oxford University Press.
- Block, Richard. 1979. “Community, Environment, and Violent Crime.” Criminology; An Interdisciplinary Journal 17 (1): 46–57.
- Bonnel, Patrick, Martin Lee-Gosselin, Johanna Zmud, and Jean-Loup Madre. 2009. “Keeping Up with a Changing World: Challenges in the Design of Transport Survey Methods.” In Transport Survey Methods: Keeping Up with a Changing World, edited by Johanna Zmud, Martin Lee-Gosselin, Marcela Munizaga, and Juan Antonio Carrasco, 3–14. Bingley: Emerald.
- Burnewicz, J. 1998. “Social Aspects of Road Transport: Introduction to the Analysis of Issues.” Social Aspects of Road Transport. Paris, France.
- Cohen, Lawrence E., and Marcus Felson. 1979. “Social Change and Crime Rate Trends: A Routine Activity Approach.” American Sociological Review 44 (4): 588–608. doi: 10.2307/2094589
- Council Directive (EC) 92/106/EEC of the Council of European Communities of 7th December 1992 on common rules for certain types of combined transport of goods between Member States. L 368/38. European Union, http://eur-lex.europa.eu/LexUriServ/LexUriServ.do?uri=OJ:L:2009:300:0072:0087:EN:PDF.
- COWI. 2015. Sidveje i Dansk Transport. Copenhagen: DTL.
- Ekwall, Daniel, and Björn Lantz. 2013. “Seasonality of Cargo Theft at Transport Chain Locations.” International Journal of Physical Distribution and Logistics Management 43 (9): 728–746. doi: 10.1108/IJPDLM-06-2012-0175
- European Commission. 2011. “Intelligent Transport Systems.” http://ec.europa.eu/transport/its/index_en.htm. Accessed 6 May.
- European Commission. 2014. Report from the Commission to the European Parliament and the Council on the State of the Union Road Transport Market. In COM(2014). Brussels: European Commission.
- EUROPOL. 2010. “Organised Crime & Energy Supply.” EUROPOL Public Information. The Hague: EUROPOL.
- Eurostat. 2017. “Hourly Labour Costs.”. http://ec.europa.eu/eurostat/statistics-explained/index.php/Hourly_labour_costs. Accessed 5 January 2018.
- Felson, Marcus, and Ronald V. Clarke. 1998. “Opportunity Makes the Thief – Practical Theory for Crime Prevention.” In Police Research Series, edited by Barry Webb. London: Policing and Reducing Crime Unit Research, Development and Statistics Directorate.
- Field, Andy P. 2013. Discovering Statistics Using IBM SPSS Statistics. London: Sage.
- Hilal, Nadia. 2008. “Unintended Effects of Deregulation in the European Union: The Case of Road Freight Transport.” Sociologie du travail 50 (S): e19–e29. doi: 10.1016/j.soctra.2008.07.002
- Hirschi, T. 1969. Causes of Delinquency. Berkeley: University of California Press.
- Hultén, Hilda. 2017. Accessed 5 January 2018. http://intelligentlogistik.com/nyhetsflode/arbetsmarknad/kampar-mot-slaveriet-i-akeribranschen/ Published 19 December 2017.
- Huq, F. A., I. N. Chowdhury, and R. D. Klassen. 2016. “Social Management Capabilities of Multinational Buying Firms and Their Emerging Market Suppliers: An Exploratory Study of the Clothing Industry.” Journal of Operations Management 46: 19–37. doi: 10.1016/j.jom.2016.07.005
- IRU. 2008. Attacks on Drivers of International Heavy Goods Vehicles. Geneva: IRU.
- Judkins, David R., and Kristin E. Porter. E. 2016. “Robustness of Ordinary Least Squares in Randomized Clinical Trials.” Statistics in Medicine 35 (11): 1763–1773. doi:10.1002/sim.6839.
- Kubrin, Charis, and Ronald Weitzer. 2003. “New Directions in Social Disorganization Theory.” Journal of Research in Crime and Delinquency 40 (4): 374–402. doi: 10.1177/0022427803256238
- Kummer, Sebastian, Maria Dieplinger, and Elmar Fürst. 2014. “Flagging Out in Road Freight Transport: A Strategy to Reduce Corporate Costs in a Competitive Environment: Results from a Longitudinal Study in Austria.” Journal of Transport Geography 36 (1): 141–150. doi: 10.1016/j.jtrangeo.2014.03.006
- Kutner, Michael, Christopher Nachtsheim, and John Neter. 2004. Applied Linear Regression Models. 4th ed. New York: McGraw-Hill.
- Lindén, Tommy. 2013. “Stolen Diesel Stays in the Country (Stulen diesel stannar i landet).” http://sverigesradio.se/sida/artikel.aspx?programid=101&artikel=5618591.
- Marshall, Donna, Lucy McCarthy, Paul McGrath, and Fiona Harrigan. 2016. “What’s Your Strategy for Supply Chain Disclosure?” MIT Sloan Management Review 57 (2): 36–45.
- McKinnon, Allan, and Jacques Leonardi. 2009. “The Collection of Long-distance Road Freight Data in Europe.” In Transport Survey Methods: Keeping up with a Changing World, edited by Patrick Bonnel, Martin Lee-Gosselin, Johanna Zmud, and Jean-Loup Madre, 295–310. Bingley: Emerald Group.
- Nakamba, C. C., P. W. Chan, and M. Sharmina. 2017. “How Does Social Sustainability Feature in Studies of Supply Chain Management? A Review and Research Agenda.” Supply Chain Management: An International Journal 22 (6): 522–541. doi: 10.1108/SCM-12-2016-0436
- Nilsson, Fredrik, Henrik Sternberg, and Thorsten Klaas-Wissing. 2017. “Who Controls Carbon Emissions from Transport and Who Cares? Investigating the Monitoring of Environmental Sustainability from a Logistics Service Provider’s Perspective.” International Journal of Logistics Management 28 (3): 798–820. doi: 10.1108/IJLM-11-2015-0197
- Nilsson, Per-Arne. 2013. “Vem stjäl er diesel?” Tidningen Proffs:nil. http://www.tidningenproffs.se/kronika/2013/06/Vem-stjal-er-diesel/. Accessed 15 January.
- Paternoster, Ray, Robert Brame, Paul Mazerolle, and Alex R. Piquero. 1998. “Using the Correct Statistical Test for Equality of Regression Coefficients.” Criminology; An Interdisciplinary Journal 36 (4): 859–866.
- Persson, Anders. 2015. “Transport- och dieselstölder 2007–2014”, Dnr. 481 A059.919/2015, Swedish Police, National Group of Transport Security, Police Region West, Gothenburg, Sweden.
- Piecyk, Maja, and Maria Björklund. 2015. “Logistics Service Providers and Corporate Social Responsibility: Sustainability Reporting in the Logistics Industry.” International Journal of Physical Distribution and Logistics Management 45 (5): 459–485. doi: 10.1108/IJPDLM-08-2013-0228
- Prockl, Günter, Christoph Teller, Herbert Kotzab, and Rob Angell. 2017. “Antecedents of Truck Drivers’ Job Satisfaction and Retention Proneness.” Journal of Business Logistics 38 (3): 184–196. doi: 10.1111/jbl.12156
- Rabinovich, E., and S. Cheon. 2011. “Expanding Horizons and Deepening Understanding via the Use of Secondary Data Sources.” Journal of Business Logistics 32 (4): 303–316. doi: 10.1111/j.0000-0000.2011.01026.x
- Radio Sweden. 2014. “Thousands Support Truck-driver Protest in Sweden.” Radio Sweden. http://sverigesradio.se/sida/artikel.aspx?programid=2054&artikel=6026038. Accessed 12 December.
- Regulation (EC) No 1072/2009 of the European Parliament and of the Council of 21 October 2009 on Common Rules for Access to the International Road Haulage Market. L 300/72. European Union, http://eur-lex.europa.eu/LexUriServ/LexUriServ.do?uri=OJ:L:2009:300:0072:0087:EN:PDF.
- Santos, Rachel B., and Roberto G. Santos. 2015. “Examination of Police Dosage in Residential Burglary and Residential Theft from Vehicle Micro-time Hot Spots.” Crime Science 4 (27): 113–112. doi:10.1186/s40163-015-0041-6.
- Saxton, Gregory D., Onook Oh, and Rajiv Kishore. 2013. “Rules of Crowdsourcing: Models, Issues, and Systems of Control.” Information Systems Management 30 (1): 2–20. doi: 10.1080/10580530.2013.739883
- SCB (Statistics Sweden). Accessed 1 May 2018. https://www.scb.se/hitta-statistik/statistik-efter-amne/hushallens-ekonomi/inkomster-och-inkomstfordelning/inkomster-och-skatter/pong/tabell-och-diagram/inkomster--individer-lankommun/sammanraknad-forvarvsinkomst-per-kommun/.
- Schlagwein, Daniel, and Niels Bjørn-Andersen. 2014. “Organizational Learning with Crowdsourcing: The Revelatory Case of LEGO.” Journal of the Association for Information Systems 15 (11): 754–778.
- Steen Jensen, Ragnhild, Mona Bråten, Bård Jordfald, Merethe Dotterud Leiren, Tor-Olav Nævestad, Kåre H. Skollerud, Henrik Sternberg, and Tommy Tranvik. 2014. “Arbeitsforhold i gods og turbil.” Fafo-Rapport. Oslo: Fafo.
- Sternberg, Henrik, Michal Filipiak, Erik Hofmann, and Daniel Hellström. 2015. Cabotagestudien: A Study on Trucking Deregulation in Scandinavia and Beyond. Lund: Lund University.
- Swedish Television, Svenska vägar – utländska chaufförer (“Swedish roads – foreign drivers”). https://www.svt.se/nyheter/granskning/ug/svenska-chaufforer-konkurreras-ut, aired on 20 March 2013, 8:00 PM.
- Toby, J. 1957. “Social Disorganization and Stake in Conformity: Complementary Factors in the Predatory Behavior of Hoodlums.” Journal of Criminal Law, Criminology, and Police Science 48: 12–17. doi: 10.2307/1140161
- Urciuoli, Luca, and Juha Hintsa. 2017. “Adapting Supply Chain Management Strategies to Security – An Analysis of Existing Gaps and Recommendations for Improvement.” International Journal of Logistics: Research and Applications 20 (3): 276–295. doi: 10.1080/13675567.2016.1219703
- van den Engel, Aad, Jan Francke, and Arnaud Burgess. 2014. “Legal or Illegal Cabotage? How Big Data Can Help to Solve This Question.” European transport conference, Frankfurt, Germany.
- van Ours, Jan C., and Ben Vollaard. 2016. “The Engine Immobiliser: A Non-starter for Car Thieves.” The Economic Journal 126 (593): 1264–1291. doi: 10.1111/ecoj.12196
- Zailani, Suhaiza Hanim, Karthigesu Seva Subaramaniam, Mohammad Iranmanesh, and Mohd Rizaimy Shaharudin. 2015. “The Impact of Supply Chain Security Practices on Security Operational Performance among Logistics Service Providers in an Emerging Economy: Security Culture as Moderator.” International Journal of Physical Distribution and Logistics Management 45 (7): 652–673. doi: 10.1108/IJPDLM-12-2013-0286
- Zmud, Johanna, Martin Lee-Gosselin, Marcela Munizaga, and Juan Antonio Carrasco. 2013. “Transport Surveys: Considerations for Decision Makers and Decision Making.” In Transport Survey Methods: Best Practice for Decision Making, edited by Johanna Zmud, Martin Lee-Gosselin, Marcela Munizaga, and Juan Antonio Carrasco, 3–20. Bingley: Emerald.