ABSTRACT
Artificial intelligence (AI) in business coaching, as in other human resource development professional service roles, opens up the possibility of multiple chances, such as cost- and time-effective gains. However, as AI can act autonomously and may surpass human performance, it can both lead to unforeseen risks as well as create a threat for professional service workers, including business coaches. Using a within-subject threat manipulation design, the present research investigated whether business coaches (N = 436; from over 50 different countries) respond to the topic of AI in coaching with heightened threat-related affective states and how this change affects their attitude towards the topic. Expectedly, the topic evoked higher behavioral inhibition and lower behavioral activation threat-related affective states, leading to lower curiosity in and a more negative opinion of AI in coaching. Theoretical and practical implications are addressed to lower the coaches’ threat-related affective states towards AI application in professional services. A hybrid approach between responsible AI and an ethically skilled and professionally trained coach is recommended.
Introduction
AI is a new and expanding technology that can be applied in various markets and offers opportunities for growth and competitive advantage for organisations. In 2022, the global total investment in AI reached over US$ 90 billion and AI’s estimated market value is US$184 billion for 2024, rising to an estimated US$ 826 billion by 2030 with an estimated annual growth rate of 37% (Grand View Research, Citation2022; Statista, Citation2024). In other words, AI is developing at a rapid pace, with new iterations and models appearing monthly, and being able to perform at least as good as a human being in nearly every aspect but is also capable of interacting with the physical world through embodiment (Thompson, Citation2024). Thus, AI has the potential over the cover decade to greatly impact the international economy, changing the work environment, replacing work roles and forcing both individuals and organisations to adapt (Bughin et al., Citation2018; Pareira et al., Citation2022). This impact is already visible in human resource development (HRD) practices: Human-aligned conversational explanation systems are being created (Dazeley et al., Citation2021) and AI is used in HRD-related fields, such as the healthcare industry or in fields of psychology (Brown et al., Citation2019; Nitiéma, Citation2023; Sharma et al., Citation2023). In addition, AI is increasingly used in several HRD areas, including talent acquisition and performance management, but also talent development and learning (Ekuma, Citation2024). Human-aligned conversational explanation systems are being created (Dazeley et al., Citation2021), and the first studies have been published on AI and its application in consulting, coaching, and training (e.g. Allemand & Flückiger, Citation2022; Allemand et al., Citation2020; Kettunen et al., Citation2022; Mirjam et al., Citation2021; Terblanche et al., Citation2022).
While this change towards AI provides new capabilities, it also comes with its challenges. Human-AI interaction situations are still a challenge for the well-being of all stakeholders, including HRD practitioners and their clients (Sajtos et al., Citation2024). Additionally, AI might not only transform but also replace certain job roles (Frey & Osborne, Citation2017; Grace et al., Citation2018). AI in theory can outperform human intelligence and performance, creating an existential threat to existing industries (Khandii, Citation2019; Moczuk & Płoszajczak, Citation2020; Nath & Sahu, Citation2020). For instance, Goldman (Citation2023) estimated that 25% of knowledge workers’ roles could be automated by AI by 2030. In addition to the threat of AI as a potential replacement of the human coaching, AI in its current developmental state itself poses threats, as it is still difficult to control for ethical mismanagement or AI diversity biases (Diller,Citation2024; Passmore & Tee, Citation2023a). In other words, AI is still capable of failing with dangerous consequences for all stakeholders (Xiang, Citation2023). Particularly in helping professions where the client is co-dependent, such as coaching,, these failures are dangerous. For instance, when the AI coach does not recognise the client’s negative emotions or shows a bias towards Western centric, white, male learning (Diller, Citation2024). These failures not only have dangerous consequences but further raise the question of who is responsible for the harm the AI causes (Nath & Sahu, Citation2020). In sum, AI may be perceived as a threat to both one’s job and one’s control due to the ethical risks of AI.
Thus, while AI performs slightly better than humans in certain HRD tasks, the use of AI applications is not yet widely accepted (Böhmer & Schinnenburg, Citation2023). However, failing to adapt or develop a proactive response to these new technologies can have significant implications not only for the organisational growth but also for the practitioners themselves. While a proactive response could help mitigate potential challenges (Kim, Citation2022), failing to respond could risk being outdated and ineffective in the new emerging organisational landscape (Evans, Citation2019). In other words, it is essential to stay curious and positive towards such new technologies (Barrett & Christian Rose, Citation2020). If coaches have a positive and curious opinion towards AI, AI coaching has the potential to become a valuable resource for them and their clients (Passmore & Tee, Citation2023a). In addition, a positive and curious attitude leads to exploration, personal growth, autonomy, positive social relationships, and various aspects of psychological well-being (Kashdan et al., Citation2013; Vogl et al., Citation2020). For instance, curiosity fosters workplace learning, task focus, work thriving, and job performance (Reio & Wiswell, Citation2000; Usman et al., Citation2023). Curiosity can be described as a motivational force to explore information about a topic, deriving from an inner need to reduce incongruity and increase knowledge. This cognitive motivation can either be a temporal psychological state that only lasts until the information gap is closed or can be a trait-like inquisitiveness similar to the Big Five-factor openness to experience (Schmidt & Rotgans, Citation2021). Furthermore, showing positive attitudes and openness towards new technologies enriches education and development practices (Štemberger & Čotar Konrad, Citation2021) as well as fosters the use of these new technologies (Kwak et al., Citation2022).
The present research explores whether AI increases the coaches’ threat-related affective states and whether this may influence their curiosity in and opinion of AI coaching. The scarcity of literature on attitudes and responses towards AI (Castagno & Khalifa, Citation2020; Yu et al., Citation2023) as well as on measures like curiosity and opinion in work-related contexts (Torraco & Lundgren, Citation2020) highlights the need to investigate how AI is perceived by professional service workers such as coaches. Given the emergence of AI and its likely transformation of the world of work over the coming decade (e.g. Bughin et al., Citation2018), understanding human reactions to AI is essential to its successful implementation in organisations and its adoption and integration into professional roles by professional service workers such as coaches, as well as managers.
AI coaching as an HRD intervention
AI refers to the development of computer systems that can perform tasks that previously typically required human intelligence, such as cognitive tasks like reasoning, decision-making, and problem-solving (Rai et al., Citation2019). This can be divided into narrow AI with a level of weak artificial intelligence, general AI as equal to human intelligence, and super-intelligence as surpassing humans (Gurkaynak et al., Citation2016). In other words, AI is a machine-assisted, systematic process. This process can be used in human resource development (HRD), such as in coaching (Graßmann & Schermuly, Citation2020; Passmore et al., Citation2024). This technology therefore differs from traditional technological revolutions, as it is not only a complementary work tool but further able to carry out human-specific tasks, sometimes even with greater efficiency and at lower costs (Su et al., Citation2020). Thus, AI reshapes HRD practices by enhancing processes and functions as well as creating jobs, changing work, or even replacing labour. Workers will be faced by the consequences of AI, which can range from minor adaptations in roles to the complete disappearance of roles (Su et al., Citation2020; Tambe et al., Citation2019). For example, low-qualified workers equipped with AI could carry out tasks that were previously reserved for highly skilled workers. In addition, specialist, high demand roles become knowledge engineers, leveraging AI technologies (Su et al., Citation2020). This, however, is just a start. As AI continues to improve at a faster rate than humans, HRD will increasingly be faced by the paradoxes and uncertainties introduced by AI technologies (Charlwood & Guenole, Citation2022).
As one HRD approach, coaching has become a recognised intervention for most enterprise organisation HR strategies. This recognition further comes from several meta-analyses and reviews that underline the benefits of coaching for both the individual (e.g. client’s job satisfaction, goal attainment and well-being) and the organisation (e.g. team satisfaction and organisational performance) (De Haan & Nilsson, Citation2023; Grover et al., Citation2016). Coaching can be defined as a process to empower clients to work with and attain their self-valued and value-congruent goals (Diller et al., Citation2020). While coaching has been described as a human-to-human interaction in the past, the new development of AI has created a new form of coaching, AI coaching, where the human coach is replaced in full or part by an AI bot (Diller & Passmore, Citation2023). This emergence of AI coaching is further underlined by first effectiveness studies: For instance, coaching-related AI applications were tested and found to support clients with their self-coaching, self-control, exercise motivation, or behavioural change (Allemand et al., Citation2020; Kettunen et al., Citation2022; Mai et al., Citation2022; Mirjam et al., Citation2021). In addition, a comparison study on an AI and human coaching processes revealed that the university students as clients reached similar levels of goal attainment as a comparison group with their human coaches (Terblanche et al., Citation2022). Given the application of AI coaching in the market, it is essential to explore both the potential opportunities and challenges as one example of how these technologies may impact roles of HRD professionals.
The opportunities available from AI coaching
AI coaching services can have several opportunities for innovation, both enhancing and replacing the traditional coaching intervention by introducing a service with (1) high levels of accessibility with regard to costs, time, and place, (2) just-in-time-delivery, (3) feelings of anonymity and nonjudgement, as well as (4) innovative methods available through connecting different AI processes including data collection and analysis across the HR experience or offering intersessional activities and interactions.
Firstly, AI coaching services are highly accessible due to their online and cost-effective service, making coach more available to many more managers and organisational users (Graßmann & Schermuly, Citation2020). Global average hourly rates are typically around US$300 per hour where AI tools can be delivered at a fraction of the cost or even for free (Passmore et al., Citation2024; Passmore et al., Citation2024). In addition, clients from geographically remote locations, or those working unsocial shifts, can potentially access AI coaching services when a human coach may be unavailable (Diller & Passmore, Citation2023). Like a ‘pocket coach’, AI coaching can be accessed independently of time and place on a smart device, available in the pocket whenever it is needed. This high accessibility independent of financials, time, and place arguably makes AI coaching more available and inclusive than human coaching (Diller, Citation2024). This advantage was found to notably already reduce barriers in the healthcare sector, such as in health coaching, where remote digital interventions have demonstrated effectiveness in managing health conditions (Mao et al., Citation2017) and chronically ill people were able to access support during the transition of care from hospital to home (Tornivuori et al., Citation2020). Further evidence in mental health has found that text-based applications were accessible and suitable option for addressing conditions like anxiety and depression (Shih et al., Citation2022).
Secondly, due to its ability to learn through experience and independence of human guidance, AI coaching services can offer just-in-time personal adaptive, which arguable can provide a more personalized service (Rowe & Lester, Citation2020). In human resource management, the introduction of AI applications could be beneficial for identifying skill-gaps, recommending development and tracking progression not simply towards completion of learning activities but also in skills acquisition (Budhwar et al., Citation2022). As technology further develops, AI gives the opportunity to incorporate psychoeducational impulses, feedback, or evaluations. For example, Gratch (Citation2014, p. 25) introduced ‘the conflict resolution agent’ as a way of using AI to teach negotiation skills, and Ovida (an AI software tool) enables users to collect data on high trust conversations and get feedback, both on individual sessions and overtime. Similarly in coaching, Passmore and Tee (Citation2023b) argue that AI could provide effective and objective feedback informed by a data-driven approach.
Thirdly, AI services may lead to greater openness and more truthful information from the client’s side than in a human coaching conversation. AI services are perceived to be more trustful and safe by their clients, as these feel less linked to impression management and fear of evaluation (Ellis-Brush, Citation2021; Gratch, Citation2014; Stai et al., Citation2020). These findings align with the online disinhibition effect (J. R. Suler, Citation2004), which suggests that people feel less inhibited and are more open in cyberspace than in the real world leading them to behave differently (J. Suler, Citation2015). In addition, the AI setting can offer a higher level of client anonymity, which can promote greater openness in discussions about difficult topics (Stein & Brooks, Citation2017). This openness and truthfulness can benefit the client for their self-valued and value-congruent goal attainment and therefore contribute to more effective outcomes (Schiemann et al., Citation2019).
Finally, AI can go beyond the traditional coaching process with its enhancements. For example, AI can offer intersessional support, helping clients to make better progress towards their goals (Ellis-Brush, Citation2020; Hassoon et al., Citation2021; Holstein et al., Citation2020; Stephens et al., Citation2019; Tropea et al., Citation2019). These intersessional interactions may not only increase the level of goal attainment but further lead to a lower number of sessions needed with a collaborating human coach. In addition, AI can support the coach: From support them in their sales process, finding new clients, to analysing data for their own coach skill development, AI can be an easily accessed tool of assistance (Diller & Passmore, Citation2023; Luo et al., Citation2020).
The risks and challenges of AI coaching
While AI coaching may have its benefits, it is important to acknowledge the potential limitations and risks of AI services in coaching, as AI still faces notable challenges in HRD (Tambe et al., Citation2019). For instance, AI might increase the digital divide between industries, companies, and countries which leverage AI technologies and have the technological knowledge and those that do not (Bughin et al., Citation2018; Statista, Citation2024). In addition, while AI comes with the opportunity to streamline people development and an almost blind trust among the users, several ethical issues arise and may even hinder personal or organisational development (Diller, Citation2024; Pandya & Wang, Citation2024). Three ethical risks include (1) the lack of empathic accuracy and empathy in situations of need, (2) non-responsible and biased decision-making, and (3) confidentiality and data security (Kinnula et al., Citation2021; Passmore & Tee, Citation2023a).
While AI may be able to model empathic behaviour in terms of paraphrasing and verbalising others' emotions, AI services still lack the ability of empathic accurately identifying the client’s emotions and understanding the underlying motivations of the human client. This can lead to difficulties in identifying a client’s core problem, evaluating goals, and providing feedback on the chosen goals. This risk of low empathic accuracy is of particular importance in identifying and managing self-harming thoughts and emotions (Passmore & Tee, Citation2023b). Moreover, showing empathy besides perspective-taking behaviour remains difficult in a virtual environment, where the context is limited with regard to physical gestures (e.g. handing a tissue, providing a warm beverage, putting a hand on one’s should for comfort). This limitation of reacting empathically is mainly risky in difficult situations where, for example, a person is having strong negative emotions like anxiety, sadness or anger (Diller, Citation2024).
Furthermore, using AI in helping services can contribute to several ethical risks, as it is making autonomous decisions and errors that could fail ethical guidelines, raising questions about accountability and transparency (Etzioni & Etzioni, Citation2016; Hermansyah et al., Citation2023; Passmore & Tee, Citation2023a). For instance, a coachbot could prioritise the client’s goal attainment over everything else, thereby increasing the risk of wider harm to others, the organisation, or society (Passmore & Tee, Citation2023a). To give another example, AI could detect legal loopholes that enable legally acceptable decision-making while ignoring ethical contradictions just to get ahead in the competition (Brendel et al., Citation2021). Moreover, non-responsible AI can promote deviant and inappropriate client behaviour (toxic online disinhibition effect; Suler, Citation2015). This issue of acting ethically responsibly is of particular importance when it comes to sensitive and personal data as well as client and organisational safety (Hermansyah et al., Citation2023). One big issue that AI hereby faces is diversity bias and discrimination (Hermansyah et al., Citation2023; Michael, Citation2019). AI services have been found to inherit biases, such as gender and racial biases, favouring white heterosexual men, based on the biased data and biased demographics of AI product developers (e.g. Köchling et al., Citation2021; Nuseir et al., Citation2021; Singh et al., Citation2020).
One last concern addresses confidentiality and data security. AI is learning from each conversation and thus gaining access to commercially sensitive and personal confidential data, which is subsequently stored and used in future answers towards other users (Passmore et al., Citation2024). In addition, data can be hacked and leaked, which has happened in the past and is a high risk when it comes to confidential information that is exchanged in coaching conversations (Diller, Citation2024). One good example might be the Ashley Madison data leak, where 37 million cheaters and their emails were leaked after a hacker attack (Linshi, Citation2015).
In sum, AI coaching services carry risks that can be difficult to predict and manage, due to the complexity of organisational life, global and organisational diversity and the generative nature of AI. A series of reports on AI misconduct from multiple renowned organisations has sparked a surge in public awareness, prompting calls for more responsible and sustainable use of AI (Chang & Ke, Citation2024). The proposed risks further show that using AI can come with challenges that are not in the control of the coach.
AI coaching as a threat to coaches
Due to their high system autonomy and low system transparency, AI usage can come with risks outside of the coaches’ control (Diller, Citation2024; Ulfert et al., Citation2024). This loss of control can lead to a perceived control threat in situations where individuals have a need for control and safety (Mirisola et al., Citation2013). In addition to the control threat, AI is found to be an occupational identity threat, as individuals perceive AI as potentially adaptating or even replacing their job (Fast & Horvitz, Citation2017; Milad et al., Citation2021; Wissing & Reinhard, Citation2018). For instance, AI systems were perceived as a threat to the professional identity of some medical roles, creating resistance amongst professionals (Jussupow et al., Citation2022). Moreover, if coaches may perceive themselves as a group, AI can further be an intergroup threat, meaning that AI can be a threat to the rights, resources, belief systems, and welfare of the respective group (Zhou et al., Citation2022). In summary, AI can be perceived as a threat by coaches in terms of their control, occupational identity and group.
Based on the General Model of Threat and Defense (Jonas et al., Citation2014), when individuals are faced with a threatening stimulus, their Behavioural Inhibition System (BIS) is activated, and their Behavioural Activation System (BAS) is de-activated. These two motivational systems BIS and BAS play a crucial role in affective responses. While BIS is associated with negative affect, avoidance, and anxious inhibition, BAS is related to positive affect, relaxation, goal-attainment behaviour, and reward seeking (Carver & White, Citation1994). In other words, a threatening topic leads to an increase in BIS and decrease in BAS affective states among coaches. For instance, coaches confronted with their past Dark Triad client increased their BIS and decreased their BAS affective states, meaning that coaches felt more anxious inhibition and less relaxed approach-orientation (Diller et al., Citation2019). The consequences of BIS activation and BAS deactivation can extend beyond affective states to impact various aspects of functioning. For instance, long-term avoidance behaviours associated with BIS activation can impair physical functioning, weaken individuals, and contribute to negative mood states that may contribute towards depression (Petrini & Arendt-Nielsen, Citation2020). For example, if the state of anxious inhibition is prolonged, distress, helplessness, and hopelessness can increase (S. Diller et al., Citation2019). Conversely, BAS deactivation can result in reduced engagement in physical and social activities, potentially exacerbating feelings of isolation and low mood (Hirsh et al., Citation2011). In other words, while positive affective states can enhance motivation and engagement (Watson et al., Citation1988), threat-related affective states can elicit distress and helplessness for the individual.
With regard to the present research, the negative consequences of higher BIS and lower BAS affective states may include the coaches’ curiosity and their opinion towards AI in coaching. Previous research hereby showed that threat-related affective states led to showing disinterest towards or even avoiding the topic (Deng et al., Citation2018; Diller et al., Citation2023). Furthermore, the threat-related affective states increased peoples’ reactance, anger, and negative opinion towards the topic (Mühlberger et al., Citation2020; Mustafaj et al., Citation2021; Pérez-Fuentes et al., Citation2020). Thus, higher BIS and lower BAS affective states may egatively influence coaches’ curiosity and opinion towards AI in coaching. Yet, having a low curiosity and a negative opinion towards new technologies can have severe long-term consequences for coaches in their professional work. Low curiosity can diminish engagement in learning and exploring new topics, intellectual development, personal growth, and (future) performance (Hartung et al., Citation2022; Nakamura et al., Citation2022). Negative opinions about new technologies can contribute towards greater inequalities based on the inability to adapt and to engage with these technologies (Barrett & Christian Rose, Citation2020; Ellis-Brush, Citation2021). Thus, it is essential for coaches to stay curios and open towards new technological developments, such as AI in coaching.
The present research
Although AI as a new type of technological change can significantly change HRD and coaching, psychological perspectives in technology implementation are rarely considered (Ulfert et al., Citation2024). In this study, we investigated whether AI in coaching as a topic increases threat-related affective states. Drawing on recent literature, AI might pose risks that are perceived to be outside the coaches’ control (Diller, Citation2024; Ulfert et al., Citation2024) and further holds the potential to replace human coaches altogether, which might lead coaches to perceive AI as a threat (Fast & Horvitz, Citation2017; Wissing & Reinhard, Citation2018). Based on threat research and in line with the General Model of Threat and Defense (Jonas et al., Citation2014) a threatening stimulus increases BIS and decreases BAS affective states, consequently we hypothesised that thinking about AI coaching evoked threat-related affective states by increasing BIS and decreasing BAS:
Hypothesis 1a:
BIS was higher after the AI coaching manipulation than before.
Hypothesis 1b:
BAS was lower after the AI coaching manipulation than before.
Furthermore, threat-related affective states can lead to reactance, disinterest, and a more negative opinion towards the threatening stimulus. Additionally, if threat-related affective states can lead to avoiding a topic and can even foster the intentions to leave a situation (Deng et al., Citation2018; Diller et al., Citation2023), information seeking behaviour might be less likely to come to display and the opinion of the AI coaching could deteriorate. Therefore, we hypothesised that this change in threat-related affective states affects the coaches’ epistemic curiosity in and opinion of AI coaching:
Hypothesis 2a:
More evoked threat-related affective states negatively predict the epistemic curiosity in AI coaching.
Hypothesis 2b:
More evoked threat-related affective states negatively predict the epistemic curiosity in AI coaching.
To investigate these hypotheses with a valid sample, we aimed for a large, diverse and global sample of coaches in our study to enhance its impact. We further decided on a within-subject threat manipulation design, as this design has been used in previous threat research (Diller et al., Citation2023; Jonas et al., Citation2014; Poppelaars et al., Citation2020), enables the precise measurement of variables related to threats (e.g. effective states) (Riek et al., Citation2006; Toni et al., Citation2008), and establishes a causal relationship between the threat and variable changes, ensuring a higher validity (Shapiro et al., Citation2013).
Materials and methods
Sample
Business coaches from 50 different countries were recruited online through coach training school alumni networks, universities, professional bodies, and large coaching providers. The final sample consisted of 436 workplace coaches (306 females, 127 males, 1 non-binary, 1 who self-identified as a ‘human being’, and 1 who did not define a gender). The respective coaches were between 18 and 78 years old (M = 53.86, SD = 9.39) and worked mainly as external coaches (85.1%; internal: 9.4%; volunteer coaches within their organisation: 5.5%)Footnote1. 388 coaches identified as heterosexual, 17 as gay/lesbian, 8 as bisexual, 2 as queer, 1 as pansexual, 1 as all sexual orientations, 1 as asexual, and 18 coaches preferred not to say. 349 coaches described themselves as White, 28 as Asian, 10 as black, 9 as Middle Eastern or North African, 8 as Hispanic or Latinx, 8 as mixed race, 4 as First nation/Natives, 1 as Mediterranean, 1 as European, and 2 as human beings (14 coaches did not answer). 25% of the coaches worked as associates for coaching providers and 29% worked as associates using a digital coaching platform. Regarding the digitalisation of their coaching, all 436 coaches had used digital coaching, 65 coaches also deliver coaching via the phone, 51 coaches used instant messaging, 16 coaches used specialist coaching software, and 5 coaches had delivered coaching via VR (virtual reality).
Design
A within-subject manipulation design was adopted. The survey was created in collaboration with the EMCC as part of the EMCC Global Coach Survey using Qualtix and was piloted in May 2023 with revisions being made based on the feedback from the initial sample of 20 business coaches. Ethical approval was received from a university Ethics Committee at Henley Business School. The survey was reviewed by a professional body and participation in the study was voluntary, without any advantages or disadvantages, and based on informed consent. The survey was anonymous, and no personal identifying information was required. The final English survey was posted online for 6 weeks in Summer 2023, with invitations distributed by over 30 partner organisations to enhance accessibility and engagement from diverse participants.
Procedure
At first, the coaches were asked about their demographics and affective states. Then, they were asked questions about their coaching practice. Subsequently, they were confronted with thinking about AI in coaching (manipulation), followed by the same measures of affective states. After this, the coach’s epistemic curiosity towards and their opinion on AI coaching was measured. In the end, the coaches were asked about other coaching topics not related to this study (e.g. questions on their coaching practice, on ethical dilemmas and digital usage). These additional data were used for publication as the EMCC Executive Report (Passmore et al., Citation2024). The present data is split from this report with complete independence of the sample selection, research questions, and methodological approach in line with regulations by APA (Citation2011) and JOB (Citation2020). displays the descriptive statistics and correlations for the study variables.
Table 1. Descriptive statistics and correlations for study variables.
Measures
AI coaching threat manipulation
To let coaches reflect on AI coaching, we asked several questions on AI coaching. Asking questions about a threat to induce it is a widely used procedure (Poppelaars et al., Citation2020). Thus, we asked, (1) whether AI chatbots can deliver coaching, (2) to what extent the coaches thought the next 5 years of coaching would be influenced by AI, and (3) how they would define AI coaching.
Threat-related affective states
Drawing on previous threat research on new work developments (e.g. organisational change as a threat; Reiss et al., Citation2019), the BIS scale consisted of five items, namely inhibited, anxious, nervous, restless, and worried (α = .81–.88), and the activated BAS scale of seven items, namely energized, powerful, competent, goal-oriented, determined, relaxed, and calmFootnote2 (α = .79–.85). Hereby, the business coaches were asked to answer 12 items on how they currently felt using a Likert scale from 0 (not at all) to 6 (completely). These items were measured at the beginning of the survey as well as directly after AI coaching as the possible threat.
Curiosity about AI coaching
Curiosity was measured via six statements by the Epistemic Curiosity Scale (Schmidt & Rotgans, Citation2021) adapted to the topic of AI (original item example: I would like to explore this topic in depth; adapted item example: I would like to explore AI coaching chatbots in depth), ranging on a Likert scale from 0 (strongly disagree) to 6 (strongly agree) (α = .94).
Opinion of AI coaching
The opinion of AI coaching was measured with four self-developed items on the coaches positive opinion (‘I will use AI coaching coachbots in my coaching’ and ‘I see how AI coaching coachbots can benefit coaching’) and a negative opinion on AI coaching (‘I have a negative opinion on AI coaching coachbots’ and ‘I would prohibit AI coaching coachbots it I could’), ranging on a Likert scale from 0 (not at all) to 6 (completely) (α = .81). A Principal Component Analysis (PCA) showed only one factor with eigenvalue ≥ 1 accounting for 64% of the total variance. Examination of Kaiser’s criteria and the scree-plot yielded empirical justification for this finding.
Data analysis
Firstly, the open question on the AI definition was qualitatively analysed, using the QCAmap software and the qualitative inductive approach (www.qcamap.org; Fenzl & Mayring, Citation2017). This analysis was done as a reading and manipulation check to secure that the coaches have read, thought about, and dealt with the questions on AI coaching. All further quantitative data were analysed using IBM SPSS statistics 28.0. The demographic data, such as gender and age, were descriptively analysed and normal distributions as well as reliability were calculated. In addition, the quantitative descriptive questions from the AI manipulation were descriptively analysed to serve as a further reading and manipulation check. Hypothesis 1 (the change of BIS and BAS) was tested, using paired t-tests with a significance level of .05. We further explored this change with repeated measures ANOVA to control for demographic variables. Hypothesis 2 (the effect of threat-related affect change on epistemic curiosity and opinion) was tested, performing multiple regression analysis with BIS and BAS as predictors and with a significance level of .05. The quantitative and qualitative data are publicly available via the CC-By Attribution 4.0 International license under osf.io/u83sb.
Results
Reading and manipulation check
As a reading and manipulation check, the business coaches had to answer the questions on AI coaching (all coaches answered). Responses of the coaches depict a rather negative connotation of AI by using words like ‘trustless’, ‘generic’, or ‘unqualified’ as well as 57% of coaches not considering AI as being able to deliver coaching (19% did see AI delivering coaching, 24% were not sure, and 1% preferred not to answer). However, 45% of the coaches expect that AI will be used to augment the coaching practice and 35% of coaches even expect that at least 20% of coaches will be replaced by AI. Independently of this content, it can be said that the coaches have read, thought about, and dealt with AI coaching as a topic.
Hypotheses 1a (H1a) and 1b (H1b): AI evoking threat-related affective states
The paired t-tests showed that participants showed higher threat-related affective states after the questions on AI coaching compared to before: Participants displayed higher BIS (H1a) (before: M = 1.16, SD = 1.06; after: M = 1.99, SD = 1.43), t(435) = 12,633, p < .001, 95%CI[0.71,0.97], Cohen’s d = .61, and lower BAS (H1b) (before: M = 4.35, SD = 0.83; after: M = 3.11, SD = 1.30), t(435) = −19.45, p < .001, 95%CI[−1.37, −1.12], Cohen’s d = .93, compared to before (see ). These findings support H1a and H1b and present a medium to large effect.
Hypothesis 2a and 2b: The effect of BIS and BAS on curiosity (H2a) and opinion (H2b)
Regarding H2a, a multiple linear regression performance depicted how a higher increase of threat-related affective states lead to less curiosity in AI coaching, R2adj. = .11, F(2,433) = 27.09, p < .001. On a closer look, this result derives from the change in BAS affective states, βBAS = .34, pBAS < .001, showing a medium effect, but not the change in BIS affective states, βBIS = .02, pBIS = .672. Regarding H2b, a second multiple linear regression performance displayed how a higher increase of threat-related affective states led to a more negative opinion of AI coaching, stemming from a medium effect from both predictors, βBIS = −.21, pBIS < .001, βBAS = .31, pBAS < .001, R2adj. = .18, F(2,433) = 47.99, p < .001 (see ). These findings support H2a and H2b and present a medium effect.
Figure 2. The effect of threat-related affective states change on curiosity and opinion.
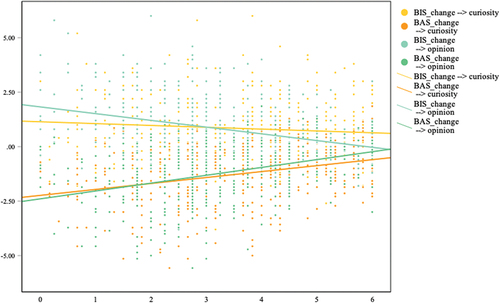
Discussion
The present research investigated whether AI technologies evoke threat-related affective states among business coaches and whether this response affects the coaches’ attitude towards AI in coaching, more precisely their curiosity and opinion. In line with Hypothesis 1, the coaches’ threat-related affective states increased after being confronted with the topic of AI coaching compared to before, depicted by an increase in BIS and decrease in BAS affective states. This finding aligns with recent threat research on AI in other domains as both an identity and intergroup threat (Jussupow et al., Citation2022; Milad et al., Citation2021; Zhou et al., Citation2022). In other words, even if technology such as AI is implemented in a specific area to support employees or professionals, it can also induce negative affective states (Brendel et al., Citation2021). In line with Hypothesis 2, this change in threat-related affective states negatively impacted the coaches’ attitude towards AI coaching, leading to less curiosity in and a more negative opinion of AI coaching. The data indicates that the decrease in curiosity can primarily be explained by the decrease in BAS affective states, while the decrease of the coaches’ opinion towards AI coaching can be attributed to the changes in both BIS and BAS activation. Similarly, previous studies have found that threat-related affective states evoke avoidance motivation, disinterest, prejudice, reactance, and a negative opinion towards the threatening topic (Liang & Xue, Citation2010; Mühlberger et al., Citation2020; Mustafaj et al., Citation2021; Onraet & Van Hiel, Citation2013; Pérez-Fuentes et al., Citation2020). In summary, coaches responded towards AI coaching as a topic with elevated threat-related affective states, leading to a lower curiosity in and a more negative opinion of AI coaching.
Limitations
The present study has two main limitations. First, the survey included additional topics, covering topics on coaching practices, fees, and digital coaching (Passmore et al., Citation2024). This multi-study survey ensured a large sample of coach participation and was designed to avoid the risk of socially desirable or otherwise biased answers towards AI, as AI in coaching was just one of many topics in the survey. However, due to the addition of other topics, the length of the survey may have led to a higher non-completion rate than in a short 10-item questionnaire and also risked less diligent responses. To minimise the risk of non-diligence, we contained the survey’s length to ten minutes and added an open-ended question on AI coaching as not only a manipulation but also reading check. The second main limitation is that we had no randomised control group design, meaning that there was no control condition with a randomised assignment to one of the two conditions. This design was chosen based on previous threat study designs (e.g. Diller et al., Citation2023) and an ethical decision to give all coaches the possibility to reflect on AI as a new and emerging topic for their profession.
Theoretical implications
The present study’s results are a first step towards research in the area of AI coaching as a threat. Future research is needed to (1) better identify the threat it poses, (2) compare the findings to a randomized control group design study, and (3) investigate differences between the anticipated threat in this study to an actual AI coaching threat. The latter differentiation is essential as affective measures differ when it comes to an actual threat, such as coaches being actually confronted with working with or being replaced by AI in their coaching (Franchina et al., Citation2023). This confrontation could either unlock affective states related to a fight/flight/freeze/fright/faint response (Bracha et al., Citation2004), could heighten affective states of worry and concern (Spence et al., Citation2011), or could even reduce the perceived level of threat due to familiarity (Grahlow et al., Citation2022; Oliveira et al., Citation2013). In addition to the named topics, further exploration is needed on how this effect on coaches might influence their clients. This idea is based on the theory of social interdependence and recent studies that show this kind of influence (Diller et al., Citation2021; Schiemann et al., Citation2019; Terblanche & Cilliers, Citation2020). Furthermore, it can be essential to explore interventions how people get back into their approach-orientation (e.g. Diller et al., Citation2023). To sum up, more research on AI coaching threat dynamics is needed – as an outlook, it could even be expanded to other HRD areas, such as consulting, mentoring, or training.
Practical implications
As AI is an emerging and inevitable new technology, practical implications are discussed on how business coaches can shift their focus from AI being a threat to adapting to AI in coaching or even seeing AI as a possibility to improve their coaching. Five main practical implications can hereby be drawn from this study: (1) Reduce AI-evoked threat-related affective states, (2) increase the feeling of control through education and knowledge exchange, (3) work with instead of against AI in coaching, (4) provide an innovative learning environment as an organisation, and (5) most importantly design AI so that it is responsible and not a control threat.
Reduce AI threat-related affective states: A first practical implication is to reduce threat-related affective states due to the topic of AI coaching. One way to reduce BIS as well as increase serenity and relaxation can be mindfulness (Diller et al., Citation2024; Park & Pyszczynski, Citation2019). Such mindfulness interventions can be Mindful Breathing or Walking, a Body Scan or a Loving-Kindness meditation (Passmore & Oades, Citation2014, Citation2015; Sparacio et al., Citation2024). Another way is an affirmation intervention, such as self-affirmation, value affirmation, or agency affirmation (Bayly & Bumpus, Citation2019; SimanTov-Nachlieli et al., Citation2018; Shuman et al., Citation2022). Affirmations can lead to an approach orientation towards a threat instead of avoiding it. Moreover, they help people focusing on their psychosocial resources when confronted with a threat and view the threat in a greater context, thereby affirmations can buffer against a threat and reduce the emergence of defensiveness (Cohen & Sherman, Citation2014). Hereby, the importance is to find the right affirmation that matches the type of threat (SimanTov-Nachlieli et al., Citation2018).
Increase the feeling of control through education and knowledge exchange: A second practical implication is to support coaches with their perceived control, as this can help people dealing with distress, as well as promote adjustment and well-being (Frazier et al., Citation2011). Hereby, education plays a significant role: By equipping individuals with technological knowledge and improving their digital competencies, they can feel more in control, increasing their openness and motivation towards new technologies (König et al., Citation2022; Passmore & Woodward, Citation2023; Syeda et al., Citation2021). Conversations on the application and implementation of new technologies as well as its benefits and challenges can further help professionals to excel in their careers and help their organisations to thrive in the new world of work (Okunlaya et al., Citation2022; Querci et al., Citation2022; Syeda et al., Citation2021; The Economist, Citation2023).
Working with, instead of against AI, in coaching: A third practical implication focusses on the collaboration between AI and human coaches as a solution to reduce intergroup and occupational threat. AI technology itself may not be the best way to go and ways to maximise the benefits of this complementarity need to be explored (Budhwar et al., Citation2022; Herrmann & Pfeiffer, Citation2023). Human-AI collaborations offer great potential in decision-making and problem-solving, due to the complementary nature of the capabilities humans and AI systems offer (Caldwell Sabrina et al., Citation2022; Graßmann & Schermuly, Citation2020; Raisch & Fomina, Citation2024). While AI can expand the human coaches’ scope of action and thereby improve the effectiveness of the human coaches’ work, the coaches’ knowledge, intuition, and social interaction skills can be a profound addition as well (Graßmann & Schermuly, Citation2020). Moreover, a hybrid approach more likely ensures ethical safety, if responsible AI is combined with an ethically aware and ethically trained professional coach (Diller, Citation2024).
Provide an innovative learning environment as an organization: A fourth practical implication is the organisational responsibility to support a positive response to AI technologies in coaching, as the organisational culture and structure can affect the perception of and willingness to use AI (Johnson et al., Citation2022). Simply implementing AI can already help: Using AI led to more effective, efficient, and transparent outcomes, motivating individuals to embrace AI technologies (Kawakami et al., Citation2022; Lukkien et al., Citation2021; Price et al., Citation2019). In other words, using these technologies can help people understand their potential benefits and drawbacks (Kashefi et al., Citation2015; Tyre & Orlikowski, Citation1994) as well as socially influence them towards AI application (Terblanche et al., Citation2022). However, when implementing AI, organisations have the responsibility to ensure that AI systems are designed to serve human needs and are accountable, lawful, and ethical in order to create sustainable and human-centric AI (Watkins & Human, Citation2022). For instance, while employees adhere significantly less to unethical instructions of an AI supervisor compared to a human supervisor, they still would still adhere an unethical instruction to some extent (Lanz et al., Citation2023). Action by the European Union has started the process of legislating to protect citizens and workers (European Parliament, Citation2023), and in the coaching sector, professional bodies such as the International Coaching Federation and EMCC Global have published voluntary standards for AI and digital (EMCC Global, Citation2023; ICF, Citation2021).
Designing responsible AI: The acceptance of coaching chatbots and therefore the success of the coachbot-client or coachbot-coach-client work alliance are tied to the impeccable implementation of responsible AI (Mai et al., Citation2022). When implementing AI chatbots in international HRD, there is a high need for fairness, autonomy and non-discrimination due to inherent biases in AI systems and the linguistic, cultural and accessibility issues (Andreas, Citation2024). Wang and Pashmforoosh (Citation2024) hereby delineate an ethical framework based on nine human-centric principles and propose six HRD interventions to promote responsible AI practices and effective human-machine interactions. In addition, Chang and Ke (Citation2024) propose a model with practical implications to implement socially responsible AI.
Conclusion
Implementing AI technology in coaching as an HRD intervention can have several benefits but can be perceived as a control, occupational, and intergroup threat to coaches. The present study found that coaches from 50 different countries responded to the topic of AI coaching with increased threat-related affective states, leading to lower epistemic curiosity and a more negative opinion towards AI coaching. While further research on these threat dynamics, including its consequences for the clients, is needed, these results lead to essential practical implications that should support coaches with their perception towards AI on an affective and cognitive level in order to foster coach-AI collaboration.
Author contributions
The study design and first draft of the manuscript were crafted by Sandra. Data was mainly collected by Jonathan with the support of the co-authors as well as others (see Acknowledgements). Data was mainly analysed by Loana under the supervision of Sandra. All authors reviewed further versions as well as approved the final manuscript.
Acknowledgements
We want to thank the EMCC Global, as well as digital coaching providers including EZRA and coach training organisations for their support of this research by distributing the survey through their networks.
Disclosure statement
No potential conflict of interest was reported by the authors.
Notes
1. We excluded 50 participants who described their main identity in the coaching profession either as being a client, a coaching researcher/writer, or a manager.
2. We did not use the English translation of ‘friedlich’ (peaceful) as the eighth BAS item. While it made sense in the context of conflict and justice (e.g. Reiss et al., Citation2019), it is not used in newer threat research (e.g. S. Diller et al., Citation2023; Franchina et al., Citation2023).
References
- Allemand, M., & Flückiger, C. (2022). Personality change through digital-coaching interventions. Current Directions in Psychological Science, 31(1), 41–48. https://doi.org/10.1177/09637214211067782
- Allemand, M., Keller, L., Gmür, B., Gehriger, V., Oberholzer, T., & Stieger, M. (2020). MindHike, a digital coaching application to promote self-control: Rationale, content, and study protocol. Frontiers in Psychiatry, 11, 575101. https://doi.org/10.3389/fpsyt.2020.575101
- Andreas, N. B. (2024). Ethics in international HRD: Examining conversational AI and HR chatbots. Strategic HR Review, 23(3), 121–125. https://doi.org/10.1108/SHR-03-2024-0018
- APA. (2011). Journal of Applied Psychology: Data Transparency Appendix Examples. American Psychological Association. Retrieved March 24, 2024, from http://www.apa.org/pubs/journals/apl/data-transparency-appendix-example.aspx
- Barrett, H., & Christian Rose, D. (2020). Perceptions of the fourth agricultural revolution: What’s In, what’s out, and what consequences are anticipated? Sociologia Ruralis, 62(2), 162–189. https://doi.org/10.1111/soru.12324
- Bayly, B. L., & Bumpus, M. F. (2019). An exploration of engagement and effectiveness of an online values affirmation. Educational Research & Evaluation, 25(5–6), 248–269. https://doi.org/10.1080/13803611.2020.1717542
- Böhmer, N., & Schinnenburg, H. (2023). Critical Exploration of AI-driven HRM to Build up Organizational Capabilities. Employee Relations, 45(5), 1057–1082. https://doi.org/10.1108/er-04-2022-0202
- Bracha, H. S., Ralston, T. C., Matsukawa, J. M., Williams, A. E., & Bracha, A. S. (2004). Does ’Fight or Flight’ need updating? Psychosomatics, 45(5), 448–449. https://doi.org/10.1176/appi.psy.45.5.448
- Brendel, A. B., Mirbabaie, M., Lembcke, T.-B., & Hofeditz, L. (2021). Ethical management of artificial intelligence. Sustainability, 13(4), 1974. https://doi.org/10.3390/su13041974
- Brown, C., Story, G. W., Mourao-Miranda, J., & Baker, J. T. (2019). Will artificial intelligence eventually replace psychiatrists? British Journal of Psychiatry, 218(3), 131–134. https://doi.org/10.1192/bjp.2019.245
- Budhwar, P., Malik, A., Silva, M. T. T. D., & Thevisuthan, P. (2022). Artificial intelligence – challenges and opportunities for international hrm: a review and research Agenda. The International Journal of Human Resource Management, 33(6), 1065–1097. https://doi.org/10.1080/09585192.2022.2035161
- Bughin, J., Seong, J., Manyika, J., Chui, M., & Joshi, R. (2018). Notes from the AI frontier: Modeling the impact of AI on the world economy. McKinsey Global Institute, 4, 1–62. https://www.mckinsey.com/~/media/mckinsey/featured%20insights/artificial%20intelligence/notes%20from%20the%20frontier%20modeling%20the%20impact%20of%20ai%20on%20the%20world%20economy/mgi-notes-from-the-ai-frontier-modeling-the-impact-of-ai-on-the-world-economy-september-2018.pdf
- Caldwell Sabrina, P. S., O’Donnell, N., Knight, M. J., Aitchison, M., Gegeon, T., & Conroy, D. (2022). An agile new research framework for hybrid human-AI Teaming: Trust, transparency, and transferability. ACM Transactions on Interactive Intelligent Systems (ThiS), 12(3), 1–36. https://doi.org/10.1145/3514257
- Carver, C. S., & White, T. L. (1994). Behavioral inhibition, behavioral activation, and affective responses to impending reward and punishment: The BIS/BAS scales. Journal of Personality & Social Psychology, 67(2), 319–333. https://doi.org/10.1037/0022-3514.67.2.319
- Castagno, S., & Khalifa, M. (2020). Perceptions of artificial intelligence among healthcare staff: A qualitative survey study. Frontiers in Artificial Intelligence, 3. https://doi.org/10.3389/frai.2020.578983
- Chang, Y.-L., & Ke, J. (2024). Socially responsible artificial intelligence empowered people analytics: A novel framework towards sustainability. Human Resource Development Review, 23(1), 88–120. https://doi.org/10.1177/15344843231200930
- Charlwood, A., & Guenole, N. (2022). Can HR adapt to the paradoxes of artificial intelligence? Human Resource Management Journal, 32(4), 729–742. https://doi.org/10.1111/1748-8583.12433
- Cohen, G. L., & Sherman, D. K. (2014). The psychology of change: Self-affirmation and social psychological intervention. Annual Review of Psychology, 65, 333–371. https://doi.org/10.1146/annurev-psych-010213-115137
- Dazeley, R., Vamplew, P., Foale, C., Young, C., Aryal, S., & Cruz, F. (2021). Levels of explainable artificial intelligence for human-aligned conversational explanations. Artificial Intelligence, 299, 103525. https://doi.org/10.1016/j.artint.2021.103525
- De Haan, E., & Nilsson, V. O. (2023). What can we know about the effectiveness of coaching? A meta-analysis based only on randomized controlled trials. Academy of Management Learning & Education, 1–21. https://doi.org/10.5465/amle.2022.0107
- Deng, M., Zheng, M., & Guinote, A. (2018). When does power trigger approach motivation? Threats and the role of perceived control in the power domain. Social and Personality Psychology Compass, 12(5), e12390. https://doi.org/10.1111/spc3.12390
- Diller, S. J. (2024). Ethics in digital and AI coaching. Human Resource Development International, 1–13. https://doi.org/10.1080/13678868.2024.2315928
- Diller, S. J., Frey, D., & Jonas, E. (2019). Coach me if you can! dark triad clients, their effect on coaches, and how coaches deal with them. Coaching: An International Journal of Theory, Research & Practice, 14(2), 110–126. https://doi.org/10.1080/17521882.2020.1784973
- Diller, S. J., Hämpke, J., Lo Coco, G., & Jonas, E. (2023). Unveiling the influence of the Italian Mafia as a dark triad threat on individuals’ affective states and the power of defense mechanisms. Scientific Reports, 13, 11986. https://doi.org/10.1038/s41598-023-38597-6
- Diller, S. J., Muehlberger, C., Braumandl, I., & Jonas, E. (2020). Supporting students with coaching or training depending on their basic psychological needs. International Journal of Mentoring & Coaching in Education, 10(1), 84–100. https://doi.org/10.1108/IJMCE-08-2020-0050
- Diller, S. J., Mühlberger, C., Loehlau, N., & Jonas, E. (2021). How to show empathy as a coach: The effects of coaches’ imagine-self versus imagine-other empathy on the client’s self-change and coaching outcome. ” Current Psychology, 42(14), 11917–11935. https://doi.org/10.1007/s12144-021-02430-y
- Diller S. J. & Passmore J. (2023). Defining digital coaching: a qualitative inductive approach. Front. Psychol., 14 10.3389/fpsyg.2023.1148243
- Diller, S. J., Stollberg, J., & Jonas, E. (2024). Don’t be scared – Be Mindful! Mindfulness Intervention Alleviates Anxiety Following Individual Threat.” Manuscript under review.
- The Economist. (2023). How artificial intelligence can revolutionise science. The Economist. Retrieved March 24, 2024, from https://www.economist.com/leaders/2023/09/14/how-artificial-intelligence-can-revolutionise-science
- Ekuma, K. (2024). Artificial intelligence and automation in human resource development: A systematic review. Human Resource Development Review, 23(2), 199–229. https://doi.org/10.1177/15344843231224009
- Ellis-Brush, K. (2020). Coaching in a Digital Age: Can a Working Alliance Form Between Coachee and Coaching App?” Doctoral diss. Oxford Brookes University. Retrieved March 24, 2024, from https://radar.brookes.ac.uk/radar/file/b03b0fb5-05ba-49ce-a9ef-8d82b64c0005/1/Ellis-Brush2020CoachingDigital.pdf
- Ellis-Brush, K. (2021). Augmenting coaching practice through digital methods. International Journal of Evidence Based Coaching & Mentoring, 15, 187–197. https://doi.org/10.24384/er2p-4857
- EMCC Global. (2023). Ethics guidance for providing coaching, mentoring and supervision using technology and AI. Retrieved March 24, 2024, from https://emccdrive.emccglobal.org/api/file/download/u7xOUK5c7JhpjZinckAUW6OoX4kG1Hcq8FSwVnR5
- Etzioni, A., & Etzioni, O. (2016). AI assisted ethics. Ethics and Information Technology, 2(18), 149–156. https://doi.org/10.1007/s10676-016-9400-6
- European Parliament. (2023). Artificial Intelligence Act: Deal on comprehensive rules for trustworthy AI. European Parliament. Retrieved March 24, 2024, from https://www.europarl.europa.eu/news/en/press-room/20231206IPR15699/artificial-intelligence-act-deal-on-comprehensive-rules-for-trustworthy-ai
- Evans, P. (2019). Making an HRD domain: Identity work in an online professional community. Human Resource Development International, 22(2), 116–139. https://doi.org/10.1080/13678868.2018.1564514
- Fast, E., & Horvitz, E. (2017). Long-term trends in the public perception of Artificial Intelligence. Proceedings of the Aaai Conference on Artificial Intelligence (Vol. 1. pp. 31). https://doi.org/10.1609/aaai.v31i1.10635
- Fenzl, T., & Mayring, P. (2017). QCAmap: Eine Interaktive Webapplikation für Qualitative Inhaltsanalyse.” [QCAmap: An Interactive Webapplication for Qualitative Content Analysis.] https://www.qcamap.org/ui/de/home
- Franchina, V., Klackl, J., & Jonas, E. (2023). The reinforcement sensitivity theory affects the questionnaire (RST-AQ). A validation study of a new scale targeting affects related to anxiety, approach motivation, and fear. Current Psychology, 43(6), 5193–5205. https://doi.org/10.1007/s12144-023-04623-z
- Frazier, P., Keenan, N., Anders, S., Perera, S., Shallcross, S., & Hintz, S. (2011). Perceived past, present, and future control and adjustment to stressful life events. Journal of Personality & Social Psychology, 100(4), 749–765. https://doi.org/10.1037/a0022405
- Frey, C. B., & Osborne, M. A. (2017). The future of employment: How susceptible are jobs to computerisation? Technological Forecasting & Social Change, 114, 254–280. https://doi.org/10.1016/j.techfore.2016.08.019
- Goldman, S. (2023). Navigating the AI Era: How can companies unlock long-term strategic value. Goldman Sachs. March 24, 2024. https://www.goldmansachs.com/what-we-do/investment-banking/navigating-the-ai-era/multimedia/report.pdf
- Grace, K., Salvatier, J., Dafoe, A., Zhang, B., & Evans, O. (2018). Viewpoint: When will ai exceed human performance? evidence from AI experts. The Journal of Artificial Intelligence Research, 62, 729–754. https://doi.org/10.1613/jair.1.11222
- Grahlow, M., Rupp, C., Derntl, B., & Suslow, T. (2022). The impact of face masks on emotion recognition performance and perception of threat. PLOS ONE, 17(2), e0262840. https://doi.org/10.1371/journal.pone.0262840
- Grand View Research. (2022). Artificial Intelligence market size, share & trends analysis report by solution, by technology (deep learning, machine learning, natural language processing, machine vision), by end use, by region, and segment forecasts 2022 - 2030. Retrieved March 24, 2024, from https://www.grandviewresearch.com/industry-analysis/artificial-intelligence-ai-market
- Graßmann, C., & Schermuly, C. C. (2020). Coaching with Artificial Intelligence: Concepts and capabilities. Human Resource Development Review, 20(1), 106–126. https://doi.org/10.1177/1534484320982891
- Gratch, J. (2014). Virtual humans for interpersonal processes and skills training. AI Matters, 1(2), 24–25. https://doi.org/10.1145/2685328.2685336
- Grover, S., Furnham, A., & Aidman, E. V. (2016). Coaching as a developmental intervention in organisations: A systematic review of its effectiveness and the mechanisms underlying it. PLOS ONE, 11(7), e0159137. https://doi.org/10.1371/journal.pone.0159137
- Gurkaynak, G., Yilmaz, I., & Haksever, G. (2016). Stifling Artificial Intelligence: Human perils. Computer Law & Security Review, 32(5), 749–758.
- Hartung, F.-M., Thieme, P., Wild-Wall, N., & Hell, B. (2022). Being snoopy and smart. Journal of Individual Differences, 43(4), 194–205. https://doi.org/10.1027/1614-0001/a000372
- Hassoon, A., Braig, Y., Naiman, D. Q., Celentano, D. D., Lansey, D., Stearns, V., & Coresh, J., (2021). Randomized trial of two artificial intelligence coaching interventions to increase physical activity in cancer survivors. NPJ Digital Medicine, 4, 168. https://doi.org/10.1038/s41746-021-00539-9
- Hermansyah, M., Najib, A., Farida, A., Sacipto, R., & Rintyarna, B. (2023). Artificial intelligence and ethics: Building an Artificial Intelligence system that ensures privacy and social justice. International Journal of Science and Society, 1(5), 154–168. https://doi.org/10.54783/ijsoc.v5i1.644
- Herrmann, T., & Pfeiffer, S. (2023). Keeping the organization in the loop: A socio-technical extension of human-centered artificial intelligence. AI & Society, 38(4), 1523–1542. https://doi.org/10.1007/s00146-022-01391-5
- Hirsh, J. B., Galinsky, A. D., & Zhong, C.-B. (2011). Drunk, Powerful, and in the Dark. Perspectives on Psychological Science, 6(5), 415–427. https://doi.org/10.1177/1745691611416992
- Holstein, K., Aleven, V., & Rummel, N. (2020). A Conceptual Framework for human–AI hybrid adaptivity in education. Artificial Intelligence in Education: 21st International ConferenceProceedings, July 6-10, 2020, Ifrane, Morocco (pp. 240–254). Springer International Publishing. https://doi.org/10.1007/978-3-030-52237-7
- ICF. (2021). The value of artificial intelligence coaching standards. International Coaching Federation. Retrieved March 24, 2024, from https://coachingfederation.org/app/uploads/2021/08/The-Value-of-Artificial-Intelligence-Coaching-Standards_Whitepaper.pdf
- JOB. (2020). Journal of Organizational Behavior: Author Guidelines. Retrieved March 24, 2024, from http://onlinelibrary.wiley.com/journal/10.1002/(ISSN)1099-1379/homepage/ForAuthors.html
- Johnson, B. A. M., Coggburn, J. D., & James Llorens, J. (2022). Artificial intelligence and public human resource management: Questions for research and practice. Public Personnel Management, 51(4), 538–562. https://doi.org/10.1177/0091026022112649
- Jonas, E., McGregor, I., Klackl, J., Agroskin, D., Fritsche, I., Holbrook, C., Nash, K., Proulx, T., & Quirin, M. (2014). Threat and defense: From anxiety to approach. In Advances in Experimental Social Psychology (Vol. 49, pp. 219–286). Elsevier Academic Press. https://doi.org/10.1016/B978-0-12-800052-6.00004-4
- Jussupow, E., Spohrer, K., & Heinzl, A. (2022). Identity threats as a reason for resistance to artificial intelligence: Survey study with medical students and professionals. JMIR Formative Research, 6(3), e28750. https://doi.org/10.2196/28750
- Kashdan, T. B., Sherman, R. A., Yarbro, J., & Funder, D. C. (2013). How are curious people viewed and how do they behave in social situations? From the perspectives of self, friends, parents, and unacquainted observers. Journal of Personality, 81(2), 142–154. https://doi.org/10.1111/j.1467-6494.2012.00796.x
- Kashefi, A., Abbott, P., & Ayoung, A. (2015). User it adaptation behaviors: what have we learned and why does it matter?. AMCIS 2015 Proceedings (pp. 12). Retrieved March 24, 2024, from https://aisel.aisnet.org/amcis2015/EndUser/GeneralPresentations/12
- Kawakami, A., Sivaraman, V., Cheng, H.-F., Stapleton, L., Cheng, Y., Qing, D., & Perer, A., et al. (2022). Improving human-AI partnerships in child welfare: Understanding worker practices, challenges, and desires for algorithmic decision support. Proceedings of the 2022 CHI Conference on Human Factors in Computing Systems (CHI’22). Association for Computing Machinery (Vol. 52. pp. 1–18, New York, NY, USA. https://doi.org/10.1145/3491102.3517439
- Kettunen, E., Kari, T., & Frank, L. (2022). Digital coaching motivating young elderly people towards physical activity. Sustainability, 14(13), 7718. https://doi.org/10.3390/su14137718
- Khandii, O. (2019). Social threats in the digitalization of economy and society. SHS web of conferences, Fifteenth Scientific and Practical International Conference “International Transport Infrastructure, Industrial Centers and Corporate Logistics” (NTI-UkrSURT 2019), 6-8 June 2019 Kharkiv, Ukraine (Vol. 67). EDP Sciences. https://doi.org/10.1051/shsconf/20196706023
- Kim, S. (2022). Working with robots: Human resource development considerations in human–robot interaction. Human Resource Development Review, 21(1), 48–74. https://doi.org/10.1177/15344843211068810
- Kinnula, M., Iivari, N., Sharma, S., Eden, G., Turunen, M., Achuthan, K., Nedungadi, P., Avellan, T., Thankachan, B., & Tulaskar, R. (2021). Researchers’ toolbox for the future: Understanding and designing accessible and inclusive artificial intelligence (AIAI). Proceedings of the 24th International Academic Mindtrek Conference (Academic Mindtrek ’24). Association for Computing Machinery (pp. 1–4 New York, NY, USA. https://doi.org/10.1145/3464327.3464965
- Köchling, A., Riazy, S., Claus Wehner, M., & Simbeck, K. (2021). Highly accurate, but still discriminatory: A fairness evaluation of algorithmic video analysis in the recruitment context. Business & Information Systems Engineering, 63(1), 39–54. https://doi.org/10.1007/s12599-020-00673-w
- König, A., Laura, A., & Tally, H. (2022). The impact of subjective technology adaptivity on the willingness of persons with disabilities to use emerging assistive technologies: a european perspective. In K. Miesenberger, G. Kouroupetroglou, K. Mavrou, R. Manduchi, M. Covarrubias Rodriguez, & P. Penáz (Eds.), Computers helping people with special needs. icchp-aaate 2022. lecture notes in computer science (Vol. 13341, pp. 207–214). Springer. https://doi.org/10.1007/978-3-031-08648-9_24
- Kwak, Y., Ahn, J.-W., & Hee Seo, Y. (2022). Influence of AI Ethics Awareness, Attitude, Anxiety, and Self-Efficacy on Nursing Students’ Behavioral Intentions. BMC Nursing, 21, 1. https://doi.org/10.1186/s12912-022-01048-0
- Lanz, L., Briker, R., & Gerpott, F. H. (2023). Employees adhere more to unethical instructions from human than AI supervisors: Complementing experimental evidence with machine learning. Journal of Business Ethics, 189(3), 625–646. https://doi.org/10.1007/s10551-023-05393-1
- Liang, H., & Xue, Y. (2010). Understanding security behaviors in personal computer usage: A Threat avoidance perspective. Journal of the Association for Information Systems, 11(7), 394–413. https://doi.org/10.17705/1jais.00232
- Linshi, J. (2015). Ashley madison data leak exposes reckless work email use. Times. Retrieved June 24, 2024, from https://time.com/4003135/ashley-madison-email-hack/
- Lukkien, D., Herman Nap, H., Buimer, H., Peine, A., Boon, W., Ket, H., & Minkman, M., (2021). Toward responsible artificial intelligence in long-term care: A scoping review on practical approaches. The Gerontologist, 63(1), 155–168. https://doi.org/10.1093/geront/gnab180
- Luo, X., Shaojun Qin, M., Fang, Z., & Qu, Z. (2020). Artificial Intelligence coaches for sales agents: caveats and solutions. Journal of Marketing, 85(2), 14–32. https://doi.org/10.1177/0022242920956676
- Mai, V., Neef, C., & Richert, A. (2022). ’Clicking vs. Writing’—the impact of a chatbot’s interaction method on the working alliance in ai-based coaching. Coaching Theorie Und Praxis, 8(1), 15–31. https://doi.org/10.1365/s40896-021-00063-3
- Mao, A. Y., Chen, C., Magaña, C., Caballero Barajas, K., & Nwando Olayiwola, J. (2017). A mobile phone-based health coaching intervention for weight loss and blood pressure reduction in a national payer population: A retrospective study. JMIR mHealth and uHealth, 5(6), e80. https://doi.org/10.2196/mhealth.7591
- Michael, L. (2019). Machine coaching. Proceedings of the IJCAI 2019 Workshop on Explainable Artificial Intelligence (XAI), 11 August 2019, Macau, China (pp. 80–86).
- Milad, M., Brünker, F., Nicholas, R. J., Möllmann Frick, N., & Stieglitz, S. (2021). The rise of artificial intelligence – understanding the AI identity threat at the workplace. Electronic Markets, 32(1), 73–99. https://doi.org/10.1007/s12525-021-00496-x
- Mirisola, A., Roccato, M., Russo, S., Spagna, G., & Vieno, A. (2013). Societal threat to safety, compensatory control, and right-wing authoritarianism. Political Psychology, 35(6), 795–812. https://doi.org/10.1111/pops.12048
- Mirjam, S., Flückiger, C., Rüegger, D., Kowatsch, T., Roberts, B. W., & Allemand, M. (2021). Changing personality traits with the help of a digital personality change intervention. Proceedings of the National Academy of Sciences, 118(8), e2017548118. https://doi.org/10.1073/pnas.2017548118
- Moczuk, E., & Płoszajczak, B. (2020). Artificial Intelligence – benefits and threats for society. Humanities and Social Sciences Quarterly, 27(2), 133–139. https://doi.org/10.7862/rz.2020.hss.22
- Mühlberger, C., Klackl, J., Sittenthaler, S., & Jonas, E. (2020). The approach-motivational nature of reactance—evidence from asymmetrical frontal cortical activation. Motivation Science, 6(3), 203–220. https://doi.org/10.1037/mot0000152
- Mustafaj, M., Madrigal, G., Roden, J., & Ploger, G. (2021). Physiological threat sensitivity predicts anti-immigrant attitudes. Politics and the Life Sciences, 41(1), 1–13. https://doi.org/10.1017/pls.2021.11
- Nakamura, S., Darasawang, P., & Reinders, H. (2022). A classroom-based study on the antecedents of epistemic curiosity in l2 learning. Journal of Psycholinguistic Research, 51, 293–308. https://doi.org/10.1007/s10936-022-09839-x
- Nath, R., & Sahu, V. (2020). The problem of machine ethics in artificial intelligence. AI & Amp; Society, 35(1), 103–111. https://doi.org/10.1007/s00146-017-0768-6
- Nitiéma, P. (2023). Artificial Intelligence in medicine: Text mining of health care workers’ opinions. Journal of Medical Internet Research, 25, e41138. https://doi.org/10.2196/41138
- Nuseir, M. T., Al Kurdi, B. H., Alshurideh, M. T., & Alzoubi, H. M. (2021). Gender discrimination at workplace: Do Artificial Intelligence (AI) and Machine Learning (ML) have opinions about it. Proceedings of the International Conference on Artificial Intelligence and Computer Vision (AICV2021) (pp. 301–316). Springer International Publishing. https://doi.org/10.1007/978-3-030-76346-6_28, Eds. A. E. Hassanien, A. Haqiq, P. J. Tonellato, L. Bellatreche, S. Goundar, A. T. Azar, E. Sabir, & D. Bouzidi.
- Okunlaya, R. O., Syed Abdullah, N., & Alinda Alias, R. (2022). Artificial Intelligence (AI) library services innovative conceptual framework for the digital transformation of University education. Library Hi Tech, 40(6), 1869–1892. https://doi.org/10.1108/lht-07-2021-0242
- Oliveira, G., Uceda, S., Oliveira, T., Fernandes, A., Garcia-Marques, T., & Oliveira, R. (2013). Threat perception and familiarity moderate the androgen response to competition in women. Frontiers in Psychology, 4, 4. https://doi.org/10.3389/fpsyg.2013.00389
- Onraet, E., & Van Hiel, A. (2013). When threat to society becomes a threat to oneself: implications for right-wing attitudes and ethnic prejudice. International Journal of Psychology, 48(1), 25–34. https://doi.org/10.1080/00207594.2012.701747
- Pandya, S. S., & Wang, J. (2024). Artificial Intelligence in Career Development: A Scoping Review. Human Resource Development International, 27(3), 324–344. https://doi.org/10.1080/13678868.2024.2336881
- Pareira, U., Giovana, W. D. L. M., & de Oliveira, M. Z. (2022). Organizational learning culture in industry 4.0: Relationships with work engagement and turnover intention. Human Resource Development International, 25(5), 557–577. https://doi.org/10.1080/13678868.2021.1976020
- Park, Y. C., & Pyszczynski, T. (2019). Reducing defensive responses to thoughts of death: Meditation, mindfulness, and Buddhism. Journal of Personality & Social Psychology, 116(1), 101–118. https://doi.org/10.1037/pspp0000163
- Passmore, J., Diller, S. J., Isaacson, S., & Brantl, M. (2024). The digital & AI coaches’ handbook: The complete guide to the use of online, AI, and technology in coaching. Routledge.
- Passmore, J., & Oades, L. (2014). Positive psychology coaching: A model for coaching practice. The Coaching Psychologist, 10(2), 68–70. https://doi.org/10.1002/9781119835714.ch46
- Passmore, J., & Oades, L. (2015). Positive psychology coaching techniques: random acts of kindness, consistent acts of kindness & empathy. The Coaching Psychologist, 11(2), 90–92. https://doi.org/10.1002/9781119835714.ch49
- Passmore, J., Saraeva, A., Money, K., & Diller, S. J. (2024). Trends in digital and ai coaching: executive report”. Henley Business School and EMCC International. Retrieved March 24, 2024, from https://www.jonathanpassmore.com/technical-reports/future-trends-in-digital-ai-coaching-executive-report
- Passmore, J., & Tee, D. (2023a). Can chatbots replace human coaches: Issues and dilemmas for the coaching profession, coaching clients and for organisations. The Coaching Psychologist, 19(1), 47–54. https://doi.org/10.53841/bpstcp.2023.19.1.47
- Passmore, J., & Tee, D. (2023b). The library of babel: Assessing the powers of artificial intelligence in coaching conversations and knowledge synthesis. Journal of Work Applied Management, 16(1), 4–18. https://doi.org/10.1108/JWAM-06-2023-0057
- Passmore, J., & Woodward, W. (2023). Coaching education: Wake up to the new digital and AI coaching revolution! International Coaching Psychology Review, 18(1), 58–72. https://doi.org/10.53841/bpsicpr.2023.18.1.58
- Pérez-Fuentes, M. D. C., Del Mar Molero Jurado, M., Martínez, Á., Linares, J., & Moran, J. M. (2020). Threat of Covid-19 and emotional state during Quarantine: positive and negative affect as mediators in a cross-sectional study of the spanish population. PLOS ONE, 15(6), e0235305. https://doi.org/10.1371/journal.pone.0235305
- Petrini, L., & Arendt-Nielsen, L. (2020). Understanding pain catastrophizing: putting pieces together. Frontiers in Psychology, 11. https://doi.org/10.3389/fpsyg.2020.603420
- Poppelaars, E. S., Klackl, J., Scheepers, D. T., Mühlberger, C., & Jonas, E. (2020). Reflecting on existential threats elicits self-reported negative affect but no physiological arousal. Frontiers in Psychology, 11, 962. https://doi.org/10.3389/fpsyg.2020.00962
- Price, W. N., Gerke, S., & Cohen, I. G. (2019). Potential liability for physicians using Artificial Intelligence. JAMA, 322(18), 1765. https://doi.org/10.1001/jama.2019.15064
- Querci, I., Barbarossa, C., Romani, S., & Ricotta, F. (2022). Explaining how algorithms work reduces consumers’ concerns regarding the collection of personal data and promotes AI technology adoption. Psychology & Marketing, 39(10), 1888–1901. https://doi.org/10.1002/mar.21705
- Rai, A., Constantinides, P., & Sarker, S. (2019). Next-generation digital platforms: Toward human-AI hybrids. Management Information System Quarterly, 43(1), iii–ix.
- Raisch, S., & Fomina, K. (2024). Combining human and artificial intelligence: Hybrid problem-solving in organizations. Academy of Management Review. https://doi.org/10.5465/amr.2021.0421
- Reio, T. G., Jr., & Wiswell, A. (2000). Field investigation of the relationship among adult curiosity, workplace learning, and job performance. Human Resource Development Quarterly, 11(1), 5–30.
- Reiss, S., Prentice, L., Schulte-Cloos, C., & Jonas, E. (2019). Organizational change as threat – from implicit anxiety to approach through procedural justice. Gruppe Interaktion Organisation Zeitschrift für Angewandte Organisationspsychologie (GIO), 50(2), 145–161. https://doi.org/10.1007/s11612-019-00469-x
- Riek, B. M., Mania, E. W., & Gaertner, S. L. (2006). Intergroup threat and outgroup attitudes: A meta-analytic review. Personality and Social Psychology Review, 4(10), 336–353. https://doi.org/10.1207/s15327957pspr1004_4
- Rowe, J. P., & Lester, J. C. (2020). Artificial Intelligence for personalized preventive adolescent healthcare. Journal of Adolescent Health, 67(2), 52–58. https://doi.org/10.1016/j.jadohealth.2020.02.021
- Sajtos, L., Wang, S., Roy, S., & Flavián, C. (2024). Guest editorial: Collaborating and sharing with AI: A research agenda. Journal of Service Theory & Practice, 34(1), 1–6. https://doi.org/10.1108/JSTP-01-2024-324
- Schiemann, S. J., Mühlberger, C., Schoorman, D. F., & Jonas, E. (2019). Trust me, i am a caring coach: The benefits of establishing trustworthiness during coaching by communicating benevolence. Journal of Trust Research, 9(2), 164–184. https://doi.org/10.1080/21515581.2019.1650751
- Schmidt, H. G., & Rotgans, J. I. (2021). Epistemic curiosity and situational interest: Distant cousins or identical twins? Educational Psychology Review, 33(1), 325–352. https://doi.org/10.1007/s10648-020-09539-9
- Shapiro, J. R., Williams, A. M., & Hambarchyan, M. (2013). Are all interventions created equal? A multi-threat approach to tailoring stereotype threat interventions. Journal of Personality & Social Psychology, 104(2), 277–288. https://doi.org/10.1037/a0030461
- Sharma, M., Rani Chaudhary, P., Mudgal, A., Nautiyal, A., & Tangri, S. (2023). Review on Artificial Intelligence in medicine. Journal of Young Pharmacists, 15(1), 01–06. https://doi.org/10.5530/097515050514
- Shih, E., Aylward, B. S., Kunkle, S., & Graziani, G. (2022). Association between care modality and use with treatment response among members accessing virtual mental health services: Real-world observational study. JMIR Formative Research, 6(7), e36956. https://doi.org/10.2196/36956
- Shuman, E., Hebel-Sela, S., Zipris, I., Hasson, Y., Hameiri, B., & Halperin, E. (2022). Advancing support for intergroup equality via a self-affirmation campaign. Group Processes and Intergroup Relations, 26(8), 1888–1908. https://doi.org/10.1177/13684302221128505
- SimanTov-Nachlieli, I., Shnabel, N., Lisa Aydin, A., & Ullrich, J. (2018). Agents of prosociality: Agency affirmation promotes mutual prosocial tendencies and behavior among conflicting groups. Political Psychology, 39(2), 445–463. https://doi.org/10.1111/pops.12418
- Singh, R., Agarwal, A., Singh, M., Nagpal, S., & Vatsa, M. (2020). On the robustness of face recognition algorithms against attacks and bias. Proceedings of the AAAI Conference on Artificial Intelligence, 34(9), 13583–13589. https://doi.org/10.1609/aaai.v34i09.7085
- Sparacio A., IJzerman, H., Ropovik, I., Giorgini F., Spiessens, C., Uchino, B. N., Landvatter, J., Tacana, T., Diller, SJ., Derrick, JL., Segundo, J., Pierce, JD., Ross, RM., Francis, Z., LaBoucane, A., Ma-Kellams, C., Ford, MB., Schmidt, K., Wong, CC., … Jiga-Boy, GM. (2024). Self-administered mindfulness interventions reduce stress in a large, randomized controlled multi-site study. Nature Human Behavior. https://doi.org/10.1038/s41562-024-01907-7
- Spence, A., Poortinga, W., & Pidgeon, N. (2011). The psychological distance of climate change. Risk Analysis, 32(6), 957–972. https://doi.org/10.1111/j.1539-6924.2011.01695.x
- Stai, B., Heller, N., McSweeney, S., Rickman, J., Blake, P., Vasdev, R., & Edgerton, Z., (2020). Public perceptions of Artificial Intelligence and robotics in medicine. Journal of Endourology, 10(34), 1041–1048. https://doi.org/10.1089/end.2020.0137
- Statista. (2024). Artificial Intelligence (AI) worldwide – statistics & facts. Retrieved June 22, 2024, from https://www.statista.com/topics/3104/artificial-intelligence-ai-worldwide/#statisticChapter
- Stein, N., & Brooks, K. (2017). A fully automated conversational artificial intelligence for weight loss: Longitudinal observational study among overweight and obese adults. JMIR Diabetes, 2(2), e28–e28. https://doi.org/10.2196/diabetes.8590
- Štemberger, T., & Čotar Konrad, S. (2021). Attitudes Towards using digital technologies in education as an important factor in developing digital competence: The case of slovenian student teachers. International Journal of Emerging Technologies in Learning (Ijet), 16(14), 83. https://doi.org/10.3991/ijet.v16i14.22649
- Stephens, T. N., Joerin, A., Rauws, M., & Werk, L. N. (2019). Feasibility of pediatric obesity and prediabetes treatment support through tess, the AI behavioral coaching chatbot. Translational Behavioral Medicine, 9(3), 440–447. https://doi.org/10.1093/tbm/ibz043
- Su, Z., Togay, G., & Côté, A.-M. (2020). Artificial Intelligence: A destructive and yet creative force in the skilled labour market. Human Resource Development International, 24(3), 341–352. https://doi.org/10.1080/13678868.2020.1818513
- Suler, J. (2015). The disinhibited self. In Psychology of the Digital Age: Humans Become Electric (pp. 95–111). Cambridge University Press. https://doi.org/10.1017/CBO9781316424070.007
- Suler, J. R. (2004). The online disinhibition effect. CyberPsychology & Behavior, 7(1), 321–326. https://doi.org/10.1089/1094931041291295
- Syeda, H. B., Syed, M., Sexton, K. W., Syed, S., Begum, S., Syed, F., & Prior, F., (2021). Role of machine learning techniques to tackle the Covid-19 Crisis: Systematic review. JMIR Medical Informatics, 9(1), e23811. https://doi.org/10.2196/23811
- Tambe, P., Cappelli, P., & Yakubovich, V. (2019). Artificial intelligence in human resources management: Challenges and a path forward. California Management Review, 61(4), 15–42. https://doi.org/10.1177/0008125619867910
- Terblanche, N., & Cilliers, D. (2020). Factors that influence users’ adoption of being coached by an artificial intelligence coach. Philosophy of Coaching: An International Journal, 5. https://doi.org/10.22316/poc/05.1.06
- Terblanche, N., Molyn, J., Haan, E., & Nilsson, V. O. (2022). Comparing artificial intelligence and human coaching goal attainment efficacy. PLOS ONE, 17(6), e0270255. https://doi.org/10.1371/journal.pone.0270255
- Thompson, A. D. (2024). Alan’s conservative countdown to AGI. LifeArchitect.ai. Retrieved May 4, 2024, from https://lifearchitect.ai/agi/
- Toni, S., Johns, M., & Forbes, C. E. (2008). An integrated process model of stereotype threat effects on performance. Psychological Review, 115(2), 336–356. https://doi.org/10.1037/0033-295x.115.2.336
- Tornivuori, A., Tuominen, O., Salanterä, S., & Kosola, S. (2020). A systematic review on randomized controlled trials: Coaching elements of digital services to support chronically ill adolescents during transition of care. Journal of Advanced Nursing, 76(6), 1293–1306. https://doi.org/10.1111/jan.14323
- Torraco, R. J., & Lundgren, H. (2020). What HRD is doing—what HRD should be doing: The case for transforming HRD. Human Resource Development Review, 19(1), 39–65.
- Tropea, P., Schlieter, H., Sterpi, I., Judica, E., Gand, K., Caprino, M., & Gabilondo, I., (2019). Rehabilitation, the great absentee of virtual coaching in medical care: Scoping review. Journal of Medical Internet Research, 21(10), e12805. https://doi.org/10.2196/12805
- Tyre, M. J., & Orlikowski, W. J. (1994). Windows of opportunity: Temporal patterns of technological adaptation in organizations. Organization Science, 1) 5, 98–118. https://doi.org/10.1287/orsc.5.1.98
- Ulfert, A.-S., Le Blanc, P., González-Romá, V., Grote, G., & Langer, M. (2024). Are we ahead of the trend or just following? The role of work and organizational psychology in shaping emerging technologies at work. European Journal of Work & Organizational Psychology, 33(2), 120–129. https://doi.org/10.1080/1359432X.2024.2324934
- Usman, M., Liu, Y., Mehmood, Q., & Ghani, U. (2023). Does employee work-related curiosity predict workplace thriving? The moderating role of core-self evaluations. Evidence-Based HRM, 11(4), 611–627. https://doi.org/10.1108/EBHRM-07-2022-0164
- Vogl, E., Pekrun, R., Murayama, K., & Loderer, K. (2020). Surprised–curious–confused: Epistemic emotions and knowledge exploration. Emotion, 20(4), 625–641. https://doi.org/10.1037/emo0000578
- Wang, J., & Pashmforoosh, R. (2024). A new framework for ethical Artificial Intelligence: Keeping HRD in the loop. Human Resource Development International, 27(3), 428–451. https://doi.org/10.1080/13678868.2024.2346492
- Watkins, R., & Human, S. (2022). Needs-aware Artificial Intelligence: AI that ‘serves [Human] Needs. AI and Ethics, 3(1), 49–52. https://doi.org/10.1007/s43681-022-00181-5
- Watson, D., Anna Clark, L., & Tellegen, A. (1988). Development and validation of brief measures of positive and negative affect: The panas scales. Journal of Personality & Social Psychology, 54(6), 1063–1070. https://doi.org/10.1037/0022-3514.54.6.1063
- Wissing, B. G., & Reinhard, M.-A. (2018). Individual differences in risk perception of Artificial Intelligence. Swiss Journal of Psychology, 4(77), 149–157. https://doi.org/10.1024/1421-0185/a000214
- Xiang, C. (2023). Man dies by suicide after talking with ai chatbot widow says. Vice. Retrieved March 24, 2024, from https://www.vice.com/en/article/pkadgm/man-dies-by-suicide-after-talking-with-ai-chatbot-widow-says
- Yu, L., Li, Y., & Fan, F. (2023). Employees’ appraisals and trust of artificial intelligences’ transparency and opacity. Behavioral Sciences, 13(4), 344. https://doi.org/10.3390/bs13040344
- Zhou, Y., Shi, Y., Lu, W., & Wan, F. (2022). Did Artificial Intelligence invade humans? The study on the mechanism of patients’ willingness to accept artificial intelligence medical care: From the Perspective of Intergroup Threat Theory. Frontiers in Psychology, 13. https://doi.org/10.3389/fpsyg.2022.866124