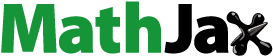
ABSTRACT
Rapid development of the ride-sharing economy has led to a rising need to better understand travellers’ decision making regarding their travel time and cost. The present study conducted a travel choice experiment using smartphone applications, based on data collected from 532 respondents and 2128 stated-preference surveys in China. Based on prospect theory, the experiment utilized a coupon reward policy to analyze how much ride-sharing platforms might influence travellers’ choices in both work and leisure contexts. The results of an ordered logit model revealed that older residents were likely to pay more to reduce waiting time. It was further found that tourists had significantly higher probabilities to take expensive alternatives with shorter queueing time. The tourists’ value of time was higher than that of residents, while the reward policy employed was found to increase the residents’ value of time. Specific theoretical and managerial implications of the findings are discussed.
Highlights
It conducted stated-preference surveys regarding travellers’ ride-sharing choices in China
The choice experiment employed four scenarios: rewarded residents, non-rewarded residents, rewarded tourists, and non-rewarded tourists
The logit model revealed older residents were likely to pay more to reduce waiting time
The tourists’ value of time was higher than that of residents
The reward policy increased the residents’ value of time, but not that of the tourists
1. Introduction
The sharing economy has become increasingly popular. Its popularity has been greatly enhanced due to the rapid development of information communication technologies (ICT), in particular the penetration of smartphone devices (Dickinson et al., Citation2014; Guttentag, Citation2015). Although there is no universal definition, the sharing economy is widely known as a peer-to-peer (P2P) economy, which is based on networks of individuals who share underutilized goods and services (Sigala, Citation2018). In a shared economic system, goods are often of a desirable nature and their use rests on an open access to the resources concerned which are commercially exploited by agents with legal property rights. The quantity of potential users is normally high, and hence the management of such large number cases can more easily be handled by the use of ICT (Liu et al., Citation2016).
The sharing economy is often associated with both the hospitality industry (Chalkiti & Sigala, Citation2008) and the transportation industry (Jacobson & King, Citation2009), as it is utilized by popular platforms such as Airbnb and Uber. Regarding sharing behaviour in transportation, ride-sharing provides opportunities to people who have similar itineraries to share vehicles, in order to improve the utilization of existing transportation modes and to potentially reduce peak period congestion (Brownstone & Small, Citation2005). Given the severe constraints inherent in matching and routing casual ride-sharing, several ride-sharing companies (e.g. Uber and Didi) have developed customer services. These services are largely similar to taxi services, with drivers predominantly not sharing a final trip destination with their passengers. Real-time ride-sharing requires a convenient means of communication between a large set of providers and travellers, and various smartphone applications (apps) have thus been developed to assist commercial ride-sharing platforms (Jacobson & King, Citation2009). In recent years, the boom of commercial ride-sharing platforms has changed the travel modes of both local people in their residential cities and visitors to tourism destinations.
In China, the major ride-sharing business is operated by Didi Chuxing (abbreviated as Didi in this paper) who has monopolized the market since 2016 (Wirtz & Tang, Citation2016). Although transportation-related agents have put large efforts into seeking solutions for traffic optimization, the substantial increase in demand, particularly during peak periods, has resulted in problems with queueing for potential travellers in China (Fang et al., Citation2013). It is thus expected that better price-setting policies and procedures might balance the demand, which could increase user satisfaction and therefore benefit the industry. This research applied theoretical modelling and experimental approaches, in an attempt to provide novel insights into Chinese travellers’ choice patterns and their value of time related to ride-sharing in different contexts.
2. Literature review
2.1. Ride-sharing
Transportation is a critical component of successful tourism development. Although a plethora of studies have examined transportation to destinations, scant research has examined intra-city mobility including travelling around a city, visiting urban attractions, and returning to accommodations (Chen, Zhang, et al., Citation2016). Consequently, Chen, Neuts, et al. (Citation2016) have suggested the importance of understanding tourists’ motivations, preferences, and intentions related to using tourism transportation at destinations.
The rapid growth of tourism at many destinations has exerted pressure on local transportation systems (Khadaroo & Seetanah, Citation2010). Some researchers have highlighted the importance of flexible urban transportation systems to help curb growing tourist demand (Gössling, Citation2017; Riganti & Nijkamp, Citation2008). Thus, efficient and diverse transportation systems have been suggested to have the ability to improve tourists’ satisfaction and their image of the destinations visited (Chen et al., Citation2019; Song, Shi, Chen, Nijkamp, et al., Citation2020). The tourism sector also offers an interesting example of flexible ride-sharing, since transportation congestion (particularly waiting in a queue to use vehicles) is often caused by excess demand and seasonality (Riganti & Nijkamp, Citation2008).
Literature on the ride-sharing economy has become increasingly popular (Cheng, Citation2016), including both theoretical (see e.g. Hall & Krueger, Citation2017) and applied empirical studies (see Cervero et al., Citation2007). It has been suggested that a financial policy (e.g. positive or negative incentives to travellers or carriers) could solve congestion and bottlenecks in urban transportation, and improve travellers’ utility (Rouwendal et al., Citation2012). However, operational studies considering the price-setting of ride-sharing platforms and the decision-making process of travellers appear to be scant.
The current commercial ride-sharing economy platform typically provides free device usage and reward to travellers, while charging ride-sharing providers with membership and transaction fees (e.g. 15–30% of the fare). Due to the large demands for transportation in China, queueing time for transportation services can be extensively long. This is usually much worse during public holidays at popular destinations, when tourists are more likely to need transportation. In queuing situations, the loss of time can lead to considerable physical and psychological costs to tourists, who might be willing to pay more (i.e. increase their WTP) to reduce their waiting time (Saveriades, Citation2000).
2.2. Value of time
Value of time has been defined as the opportunity cost of the time travellers spend on their journeys, which has been found to differ greatly depending on whether one is travelling for work or leisure purposes (Beesley, Citation1965). In a transport context, the maximum cost travellers are willing to pay (WTP) to arrive their destinations has been referred to as their value of time (Johnston et al., Citation1999). Although the value of time has been a widely-developed concept for analyzing work environments in transport economics (Brownstone & Small, Citation2005), only a few studies have applied it to tourism settings (Fezzi et al., Citation2014). Neuts et al. (Citation2016) have stated that tourists’ perceptions of value should be considered when setting prices for tourism transportation related products. Therefore, it is likely that assessing travellers’ WTP to reduce queueing time would assist in planning and developing new transportation modes (e.g. the emerging commercial ride-sharing) and may also be important for price setting and policy making (Dantan et al., Citation2017).
Multiple theories have guided research related to travellers’ value of time, and the majority of existing models are based on the utility maximization assumption and expected utility theory (Von Neumann & Morgenstern, Citation1947). Despite the wide use of expected utility theory, likely the most discussed is prospect theory (Starmer, Citation2000), also known as non-expected utility theory (Kahneman & Tversky, Citation1979). As a descriptive model, prospect theory was essentially developed for simple prospects with monetary outcomes (i.e. gains and losses) and stated probability from utility theory, which is based on stylized assumptions on individuals’ preferences depending on contexts and value maximization (Manski, Citation1977).
Choice modelling utilizes a systematic component to explain relevant exogenous factors and a random unobserved component (Grether & Plott, Citation1979), and discrete choice models (Morley, Citation1994) have been prominent frameworks for examining individual decisions and for empirically estimating WTP. Prospect theory and discrete-choice models have been widely applied in decision making regarding the tourists’ demand and WTP in tourism, hospitality and leisure research (Crouch et al., Citation2007).
It is worth noting that the platform plays a critical role of connecting consumers and suppliers in two-sided market research (Rochet & Tirole, Citation2002, Citation2003). In this study, it is likely that the ride-sharing platform could be a key component for the research framework (see ). Hence, this study aims to provide a better understanding of critical factors in a ride-sharing economy, mainly from the perspective of travellers’ value of time during transportation congestion. More specifically, this research focuses on three major questions: (1) the ride-sharing platform’s pricing; (2) travellers’ decision-making regarding their cost to reduce the queueing time in both work and leisure contexts; and (3) the influence of a reward policy on travellers’ value of time.
3. Choice modelling
3.1. Theory specification
The foundation of a choice experiment approach is to maximize utility in a random-utility model. In the current study, the indirect utility function for each respondent
and choice set
is as follows:
(1)
(1) where
is decomposed into a deterministic function
of the different alternative attributes
in the choice set, and a stochastic component
specifying the unobserved effects.
The probability of a respondent choosing a choice set
is:
(2)
(2)
(3)
(3) where
represents a scale parameter, which is inversely related to the variance of
.
To estimate in model (3),
in equation (1) is written in a linear function,
(4)
(4) where
are coefficients to be estimated;
is used to control for local residents, tourists, rewards or not.
In comparison to the above utility theory, prospect theory further clarifies how people choose between probabilistic alternatives and evaluate gains and losses (Kahneman & Tversky, Citation1979). In prospect theory, the utility of prospect can be defined as:
(5)
(5) where
is a weighted probability function;
is the value function of observed variables
; and £ is a reference point.
Accordingly, prospect theory identifies gains and losses from different options with a neutral reference point (£) for appropriate decision making. It further evaluates the outcome of each alternative and estimates its probabilities through an S-shaped value function. The asymmetric value function is concave for gains and convex for losses, as the theory postulates that losses hurt more than gains help (see ).
Figure 2. The value function of prospect theory (Source: Kahneman & Tversky, Citation1979).
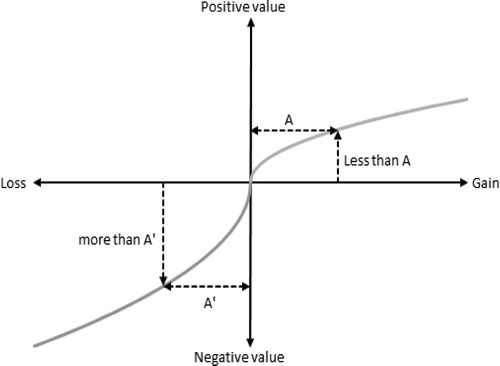
However, in the utility model suggested by prospect theory, the utility and the weighted probability functions () may be nonlinear in real-world situations. Thus, travellers’ value functions are probably not monotonically increasing or decreasing. In order to understand the complex process of travellers’ choices and value of time in the emerging ride-sharing market in China, the current research will thus conduct an experiment with multiple choices.
3.2. Choice experiment
Didi currently has a monopoly on the ride-sharing platform in China. Travellers get access to Didi through Wechat (called Weixin in China), which is the most popular smartphone chat app in China with over 938 million monthly active users (Tencent Interim Report, Citation2016). The choice experiment in this study covered the period from May 27 through 4 June 2017, and was distributed by Wechat to be consistent with the travel behaviours of Didi ride-sharing users.
During the experiment, respondents were contacted by an instant mobile online moment through social networks in China, and each respondent was surveyed with alternative ride-sharing choices in four scenarios. These contexts included: residential city without rewards, residential city with rewards, tourism destination without rewards, and tourism destination with rewards. Each participant was asked to make a choice under all four scenarios (i.e. work commuting in a residential city, leisure travel at a tourism destination, with a reward coupon, and without a reward coupon). The choice experiments are displayed in .
Table 1. The choice experiment on ride-sharing.
3.3. Data description
In general, this research experiment was conducted over all 35 provincial-level administrative units in China including 23 provinces, four municipalities (Beijing, Tianjin, Shanghai, and Chongqing), five autonomous regions (Guangxi, Inner Mongolia, Tibet, Ningxia, and Ningxia), two special administrative regions (Hong Kong and Macau), and Taiwan.
Ride-sharing has not been accessible in some western inland provinces in China (e.g. Gansu, Ningxia, Tibet, and Xinjiang), and this is likely why the number of respondents in these regions was significantly lower than in the eastern coast areas. By contrast, the Zhejiang province is an area with active entrepreneurship, which has seen a large increase in (online) small businesses in recent years (Liu et al., Citation2016) and, as would be expected, had the most respondents (121). Beyond the 35 Chinese prefectures, there were 21 respondents originally from China, who currently live in foreign countries such as the U.S., Canada, the U.K., the Netherlands, and Japan. In total, there were 532 respondents. The corresponding number of responses from each region is displayed in .
Table 2. The residential provinces or municipalities of respondents.
The respondents’ characteristics, including their gender, age, use experience, and use frequency, are presented in . The majority of respondents appeared to be relatively young, with 75% being younger than 40 years old. According to the Chinese Statistics report (Citation2017), this is generally consistent with the typical users of smartphones (67% at 18–45 years) and ride-sharing apps (60% at 26–35 years).
Table 3. The characteristic profile of respondents in using Didi ride-sharing.
4. Empirical results
This research is inspired by two-sided market theory (Chen et al., Citation2017; Rochet & Tirole, Citation2003), with a conceptual research framework regarding the ride-sharing platform. This study is also grounded in prospect theory (Kahneman & Tversky, Citation1979), dealing with travellers’ gains and losses under conditions of risks and uncertainty (Crouch et al., Citation2007). Hence, the empirical models focus on travellers’ decision-making with respect to their travel time and cost via a stated-preference experiment. Considering the ordinal ranking of extra payment and additional queueing time among the four alternatives in the experiment, ordered logit models based on a nonlinear probability function of the independent variables were utilized as suggested by McElevy and Zaviona (Citation1975). Thus, an ordered logit model was applied to understand the choice preference of residents and tourists in different reward contexts.
The results in revealed that older residents were 1.6–2.4 times more likely to choose the choices that needed higher payment to reduce waiting time, compared to younger respondents (18–29 years). It was further found that tourists had significantly higher probabilities (1.5–2.4 times) to choose expensive choices with shorter queueing time. Frequency and experience were not found to significantly (p > 0.1) be related to WTP. Further, gender was not found to affect travellers’ choices significantly. The model also predicted that the alternative 2 (i.e. a combination of paying $1.5 extra and waiting for 15 mins) was the preferred choice with probability of about 40% for residents and 30% for tourists.
Table 4. Odds ratio and probability of the ordered logit model of travellers choices.
In order to better understand the effects of travellers’ age on their choices, the probabilities of the four alternatives were further predicted based on the results of the ordered logit model. reveals that non-rewarded travellers in the young age category had a comparative high probability of about 30% to choose alternative 1 (i.e. the one without extra payment and waiting for 30 mins). This suggests that a reward policy would increase young travellers’ WTP to reduce their queueing time. Tourists over 30 years were likely to choose more expensive alternatives 3 and 4. Yet, it seems that rewards did not affect tourists’ choices significantly, in particular for the those over 40 years old.
Based on the results of the ordered logit model, travellers’ choice frequencies were further used to estimate their average value of time (i.e. the average cost travellers are willing to pay). Because the trade-off between waiting time and additional costs varies per alternative, each alternative has its own (implied) value-of-time interval. For example, the first alternative’s average cost is less than US$6, followed by US$6-9 for the second, US$9-18 for the third, and more than US$18 for the fourth alternative. However, the midpoint cannot be used to estimate the average value of time for the first and fourth unbounded intervals. It has been assumed that value of time has a log-normal distribution and a finite value-of-time could be estimated (Yang et al., Citation2001). The choice frequencies suggest that a fraction chooses alternative 1 (a value of time < US$6/hour) and a fraction
chooses alternative 2 (a value of time US$6–$9/hour). This implies that a fraction
has a cumulative value of time (<US$9/ hour). These cumulative frequencies were used to fit the log-normal distribution by regressing the logarithm of maximum implied value of time on the normalized cumulative frequencies (see the estimated coefficients in ).
Table 5. Parameters of the log-normal distributions.
Based on the parameters displayed in , the maximum cost of travellers’ WTP in the four contexts could be estimated. reveals that the average value of time was about $10/hour for residents without a reward, nearly $12/hour for rewarded residents, and over $13/hour for (non-)rewarded tourists. Therefore, the reward policy increased residents’ value of time by about $2/hour, whereas its influence on tourists was almost negligible. Tourists, especially the non-rewarded tourists, were more likely to have higher values of time (i.e. over $15/hour), as their value-of-time distributions had a larger tail. These findings about travellers’ varied value of time in different contexts may provide ride-sharing platforms with guidelines to implement more effective dynamic pricing.
5. Discussion and conclusions
Ride-sharing has become an important option for both local residents and tourists, but it has rarely attracted academic attention (Heo, Citation2016). Especially in China, large demands during rush hours and at tourism destinations have challenged the price-setting mechanism of providers and have likely made travellers’ decision-making processes more elaborate (Song, Chen, and Chen, Citation2020). The current study compared the differences in decision making between residents and tourists at their residential cities and at tourism destinations in both rewarded and non- rewarded contexts. Through this process, it is believed the current study provides a better understanding of the ride-sharing economy from both residents’ and tourists’ perspectives.
With the use of a choice experiment on ride-sharing in China, the current study used an ordered logit model to analyze travellers’ preferences and found that older people had a higher WTP than younger respondents. Specifically, it was found that tourists aged 30–59 years old had higher WTP than residents, although a reward policy could reduce their WTP in both work and leisure environments. This suggests that ride-sharing platforms should use reward policies carefully, since they might not contribute to travellers’ WTP.
Moreover, the results of the logit model showed a statistically non-significant value (p > 0.1) of ride-sharing for use frequency, and use experience in the four contexts. Thus, a reward policy might not be a viable incentive for ride-sharing users who travel at a higher frequency or have longer use experience. Moreover, this research found that travellers in a leisure context had a comparatively higher WTP, in particular middle-aged tourists (30–59 years), compared to residents in a work environment. This suggests that ride-sharing platforms could segment leisure travellers and regular work commuters to better set prices for target groups with a different WTP. This could be done by distinguishing users’ residential cities through their registered profiles and charge those who are tourists at a higher rate.
However, it was further found that the tourists’ value of time was about $2 to $4/hour higher than that of the residents, which is inconsistent with past research (Walsh et al., Citation1990). For example, Van Ommeren et al. (Citation2000) suggested that time used to travel for work has greater value than time used to travel for leisure users. Yet, the current study found that tourists in China had a higher value of time at tourism destinations than in their residential cities. A plausible explanation is that tourism in China is still a comparatively luxury experience for citizens and that they attach a high value to their travel time at tourism destinations. Thus, there are potential differences in the role time plays in the decision-making process (Rao, Citation2018; Rao et al., Citation2019; Rao & Zhang, Citation2020). It is suggested that this phenomenon warrants further research in the hospitality and tourism fields and more studies in other contexts to better understand travellers’ consumer behaviour. These findings also suggest that tourism related authorities might put more effort into improving local transportation in order to avoid of tourism-related congestion, as it would waste tourists’ valuable time and hurt their experiences at destinations.
6. Implications and limitations
6.1. Theoretical implications
Guided by both utility theory and prospect theory, the present research is believed to be one of the first to attempt to understand the ride-sharing economy from the perspective of travellers’ WTP. With this framework, the current study applied a stated-preference experiment to investigate the decision-making processes both tourists and residents utilize in a ride-sharing context. Based on the foundation of two-sided market theory in a sharing economy, this paper found that the reward policy of ride-sharing platforms may decrease travellers’ WTP, particularly for users in a work environment. Additionally, in accordance with utility theory and prospect theory, results of the choice experiments revealed that travellers in a leisure context felt more loss during queueing and that they had a higher WTP than those in a work environment. Moreover, tourists had a higher value of time and were less sensitive to the reward policy, in contrast to the residents’ value of time in a work environment. These findings have thus generated three main theoretical contributions.
First, this research contributes to the sharing economy literature by providing a better understanding of price-setting mechanisms for ride-sharing platforms. Thus, it is believed this study has further enriched two-sided market theory from a ride-sharing economy perspective and how rewards can affect consumers’ choices, in particular the WTP of both tourists and local residents. In the current ride-sharing economy, commercial platforms typically provide rewards to travellers, while charging ride-sharing providers with membership and transaction fees. In queuing situations, travellers could offer to pay more to reduce the waiting time during peak periods. The pricing framework highlights the roles of three major players and reveals the complexity of the payment relationships among them. It is further believed that it advances the theoretical understanding of how the demand and supply sides interact on ride-sharing platforms in terms of payment and lays ground for future studies on other sharing platforms. It was also found that the ride-sharing platform may decrease its efficiency when using a reward policy, although it has been widely applied in the traditional two-sided markets of credit cards (Chang et al., Citation2005).
Second, this study has shed some light on prospect theory by comparing travellers’ WTP in different contexts by employing a choice experiment approach. It is believed that respondents’ decision-making processes were complex and that tourists had more loss (time) than gains (money) when they chose to wait for ride-sharing. Accordingly, travellers in a leisure context were found to have a higher WTP to reduce the queuing time than those in a work environment. Previous literature has identified that WTP varies by external factors, such as travel time and distance (Li et al., Citation2010). Results of the present research suggest that WTP may vary by internal factors, such as respondents’ age and travel purposes (i.e. work or leisure) as well. It is hence believed these findings have enriched the current knowledge about travellers’ consumer behaviour in different contexts, in particular that they had a higher WTP in a leisure environment than a work one.
Third, it is believed this research contributes to the ride-sharing economy literature by introducing the value of time from transport economics and by empirically examining it under different contexts (e.g. tourists vs. residents, and rewards vs. non-rewards). Value of time is a well-developed concept in transportation studies (Beesley, Citation1965; Van Ommeren et al., Citation2000), yet has not been applied to a ridesharing context for tourists. This research found that tourists had a higher value of time than residents, which revealed that the value of time may vary according to different travel purposes. It is hence believed that the results have led to new insights into the value of time in a tourism setting by examining ride-sharing decisions of both work commuters (residents) and tourists.
6.2. Practical implications
The rapidly growing Chinese economy has generated increasing traffic problems, and has led to an increase in ride-sharing with an excess demand of travellers in recent years (Liu et al., Citation2016). This study applied several empirical approaches to analyze the travellers’ choices related to various ride-sharing contexts. The findings provide a profile of Chinese ride-sharing users, including their WTP and choice preferences under different reward policies. It was found that a reward policy might not be a useful incentive for frequent users or those with more use experience. These results suggest potential implications for ride-sharing platforms, service providers, and transportation authorities.
For transportation service providers, it is believed that the findings have distinct implications during rush hours, on public holidays, and at tourism destinations. It was found that older travellers (over 40) were willing to pay more for ride-sharing services than younger travellers (less than 30 years old). Ride-sharing platforms might thus be able to use this information, together with users’ choice preferences, to differentiate prices for different user segments to improve revenue generated.
It was further found that the WTP of tourists was higher than that of residents, suggesting that prices could be set higher during peak seasons at tourism destinations and during hours when there are increases in tourism traffic. This would likely be particularly important during residents’ commute times to and from work.
The research framework in this study also provides transportation authorities with a general model for understanding the complex payment relationships between the ride-sharing platform, travellers, and ride-sharing drivers. The model further delineated how rewards may influence consumers’ decisions in different contexts. This could be used by the related authorities to make policies to improve industry efficiency (Engida et al., Citation2018, Citation2020; Hurley et al., Citation2014, Citation2016). For example, urban transportation regulators may charge ride-sharing users with rush-hour fees to encourage them to move to other transportation sources. Further, programmes and public transportation should be developed to be more attractive to local residents, particularly during excess demand situations. Meanwhile, the transportation authority at popular tourism destinations could also support the ride-sharing platforms to launch sharing commuter buses during public holidays to avoid tourists’ potential disappointment and the loss of valuable time.
A final implication includes the travellers’ value of time in different contexts. In comparison to local residents, it seems that tourists had higher values of time ($13/hour), but were less sensitive to a reward policy. The coupon reward policy was found to increase residents’ value of time by about $2/hour, but did not affect tourists’ value of time significantly. These findings further suggest that ride-sharing platforms might be better off by charging flexible rush-hour fees rather than providing regular rewards, which has also been widely-applied in public transportation sectors (Saveriades, Citation2000). Given that tourists had a higher value of time, the ride-sharing platform may encourage ride-sharing providers to work during public holidays and to allocate them around tourism destinations to reduce tourists’ queueing time in using vehicles.
6.3. Limitations and future directions
The current study aimed to contribute to the understanding of the ride-sharing economy, travellers’ WTP in work and leisure contexts, and the influences of financial reward policies, to aid transportation regulators in reducing bottlenecks caused by excess demand in China. It should be noted however that the commercial ride-sharing market in China has been monopolized by Didi since 2016 and there has been little to no competition from other platforms. Thus, a limitation of the current study is that travellers’ decision-making may change if an alternative ride-sharing platform becomes available. For example, if there were several ride-sharing agents (e.g. Uber and Lyft), it is likely that the findings might have been different. Given that some imbalanced regional factors (e.g. industry structure and urban-rural difference) might have influenced the empirical results (Pardey et al., Citation2014, Citation2016, Citation2018; Song, Shi, Chen, and Li, Citation2020). Hence, future research should examine the effects of regional factors on WTP for shared economy resources.
Considering that the present paper focused on travellers’ choices in different contexts, it would be beneficial to include detailed users’ profiles (e.g. family status and disposable income) in future studies. Moreover, it would be interesting to conduct another series of choice experiments in a competitive market (Zhang et al., Citation2017), where there are alternative ride-sharing providers and travellers having multiple service providers to choose. Also, future research might seek ways to further extend the current findings to include other transportation choices (e.g. public transportation and the emerging bike-sharing) in urban transportation. Consequently, a smart transportation choice system including different and alternative travel tools for both local residents and tourists could also be an important area for future research. With regard to transportation bottlenecks caused by excess ride-sharing demand in China, it would be meaningful for transportation authorities to adjust the related regulations to develop more sharing platforms and service providers in a long run.
Yet, in conclusion, it is believed the current study provides an operational model for understanding the decision-making processes tourists and residents use related to their WTP for ride-sharing transportation services. It was found that older travellers had a higher WTP in a leisure context than in a work environment and that tourists’ value of time was comparatively higher, but less sensitive than residents. Hence, these results suggest that there is a lot of variation from person to person, and that travellers’ decision-making processes are rather complex.
Disclosure statement
No potential conflict of interest was reported by the author(s).
References
- Beesley, M. E. (1965). The value of time spent in travelling: Some new evidence. Economica, 32(126), 174–185. https://doi.org/10.2307/2552547
- Brownstone, D., & Small, K. A. (2005). Valuing time and reliability: Assessing the evidence from road pricing demonstrations. Transportation Research Part A: Policy and Practice, 39(4), 279–293. https://doi.org/10.1016/j.tra.2004.11.001
- Cervero, R., Golub, A., & Nee, B. (2007). City carshare: Longer-term travel demand and car ownership impacts. Transportation Research Record: Journal of the Transportation Research Board, 1992(9), 70–80. https://doi.org/10.3141/1992-09
- Chalkiti, K., & Sigala, M. (2008). Information sharing and knowledge creation in online forums: The case of the Greek online forum ‘DIALOGOI’. Current Issues in Tourism, 11(5), 381–406. https://doi.org/10.1080/13683500802316006
- Chang, H., Evans, D. S., & Swartz, D. D. G. (2005). The effect of regulatory intervention in two-sided markets: An assessment of interchange-fee capping in Australia. Review of Network Economics, 4(4), 328–358. https://doi.org/10.2202/1446-9022.1080
- Chen, J. M., Lijesen, M. G., & Nijkamp, P. (2017). Interpretation of cruise industry in a two-sided market context: An exploration on Japan. Maritime Policy and Management, 44(6), 790–801. https://doi.org/10.1080/03088839.2017.1335898
- Chen, J. M., Neuts, B., Nijkamp, P., & Liu, J. (2016). Demand determinants of cruise tourists in competitive markets: Motivation, preference and intention. Tourism Economics, 22(2), 227–253. https://doi.org/10.5367/te.2016.0546
- Chen, J. M., Petrick, J. F., Papathanassis, A., & Li, X. (2019). A meta-analysis of the direct economic impacts of cruise tourism on port communities. Tourism Management Perspectives, 31, 209–218. https://doi.org/10.1016/j.tmp.2019.05.005
- Chen, J. M., Zhang, J., & Nijkamp, P. (2016). A regional analysis of willingness-to-pay in Asian cruise markets. Tourism Economics, 22(4), 809–824. https://doi.org/10.1177/1354816616654254
- Cheng, M. (2016). Current sharing economy media discourse in tourism. Annals of Tourism Research, 60(C), 111–114. https://doi.org/10.1016/j.annals.2016.07.001
- Chinese Statistics Report. (2017). https://www.statista.com/statistics/224110/smartphone-users-in-china-by-age/
- Crouch, G. I., Oppewal, H., Huybers, T., Dolnicar, S., Louviere, J. J., & Devinney, T. (2007). Discretionary expenditure and tourism consumption: Insights from a choice experiment. Journal of Travel Research, 45(3), 247–258. https://doi.org/10.1177/0047287506295912
- Dantan, S., Bulteau, J., & Nicolaï, I. (2017). Enhancing sustainable mobility through a multimodal platform: Would travellers pay for it? International Journal of Sustainable Development, 20(1–2), 33–55. https://doi.org/10.1504/IJSD.2017.083501
- Dickinson, J. E., Ghali, K., Cherrett, T., Speed, C., Davies, N., & Norgate, S. (2014). Tourism and the smartphone app: Capabilities, emerging practice and scope in the travel domain. Current Issues in Tourism, 17(1), 84–101. https://doi.org/10.1080/13683500.2012.718323
- Engida, T. G., Rao, X., Berentsen, P. B., & Lansink, A. G. O. (2018). Measuring corporate sustainability performance–the case of European food and beverage companies. Journal of Cleaner Production, 195, 734–743. https://doi.org/10.1016/j.jclepro.2018.05.095
- Engida, T. G., Rao, X., & Lansink, A. G. O. (2020). A dynamic by-production framework to examine inefficiency specific to corporate social responsibility. European Journal of Operational Research. https://doi.org/10.1016/j.ejor.2020.05.022.
- Fang, Z., Juan, Z., & Jia, H. (2013). Examination of staggered shifts impacts on travel behavior: A case study of Beijing, China. Transport, 28(2), 175–185. https://doi.org/10.3846/16484142.2013.803263
- Fezzi, C., Bateman, I. J., & Ferrini, S. (2014). Using revealed preferences to estimate the value of travel time to recreation sites. Journal of Environmental Economics and Management, 67(1), 58–70. https://doi.org/10.1016/j.jeem.2013.10.003
- Gössling, S. (2017). Tourism, information technologies and sustainability: An exploratory review. Journal of Sustainable Tourism, 25(7), 1024–1041. https://doi.org/10.1080/09669582.2015.1122017
- Grether, D. M., & Plott, C. R. (1979). Economic theory of choice and the preference reversal phenomenon. The American Economic Review, 69(4), 623–638. www.jstor.org/stable/1808708.
- Guttentag, D. (2015). Airbnb: Disruptive innovation and the rise of an informal tourism accommodation sector. Current Issues in Tourism, 18(12), 1192–1217. https://doi.org/10.1080/13683500.2013.827159
- Hall, J. V., & Krueger, A. B. (2017). An analysis of the labor market for Uber’s driver-partners in the United States. ILR Review, 77(3), 705–732. https://doi.org/10.1177/0019793917717222.
- Heo, Y. (2016). Sharing economy and prospects in tourism research. Annals of Tourism Research, 58, 166–170.
- Hurley, T. M., Rao, X., & Pardey, P. G. (2014). Re-examining the reported rates of return to food and agricultural research and development. American Journal of Agricultural Economics, 96(5), 1492–1504. https://doi.org/10.1093/ajae/aau047
- Hurley, T. M., Rao, X., & Pardey, P. G. (2016). Re-examining the reported rates of return to food and agricultural research and development: Reply. American Journal of Agricultural Economics, 99(3), 827–836. https://doi.org/10.1093/ajae/aaw079
- Jacobson, S. H., & King, D. M. (2009). Fuel saving and ride-sharing in the US: Motivations, limitations, and opportunities. Transportation Research Part D: Transport and Environment, 14(1), 14–21. https://doi.org/10.1016/j.trd.2008.10.001
- Johnston, R. J., Swallow, S. K., & Weaver, T. F. (1999). Estimating willingness to pay and resource tradeoffs with different payment mechanisms: An evaluation of a funding guarantee for watershed management. Journal of Environmental Economics and Management, 38(1), 97–120. https://doi.org/10.1006/jeem.1999.1077
- Kahneman, D., & Tversky, A. (1979). Prospect theory: An analysis of decision under risk. Econometrica, 47(2), 263–291. https://doi.org/10.2307/1914185
- Khadaroo, A. J., & Seetanah, B. (2010). Transport infrastructure and foreign direct investment. Journal of International Development, 22(1), 103–123. https://doi.org/10.1002/jid.1506
- Li, Z., Hensher, D. A., & Rose, J. M. (2010). Willingness to pay for travel time reliability in passenger transport: A review and some new empirical evidence. Transportation Research Part E: Logistics and Transportation Review, 46(3), 384–403.
- Liu, B., Zhang, X., Liu, N., & Wang, Y. (2016). A function spaces model to express the development trend of electronic information manufacturing industry in Zhejiang province during 1978-2013. Journal of Residuals Science and Technology, 13(8), 1–14. http://www.destechpub.net/2019/JRST-Volume-13-Number-8-2016.pdf.
- Manski, C. (1977). The structure of random utility models. Theory and Decision, 8(3), 229–254. https://doi.org/10.1007/BF00133443
- McElevy, R. D., & Zaviona, W. (1975). A statistical model for the analysis of ordinal level dependent variables. The Journal of Mathematical Sociology, 4(1), 103–120. https://doi.org/10.1080/0022250X.1975.9989847
- Morley, C. L. (1994). Discrete choice analysis of the impact of tourism prices. Journal of Travel Research, 33(2), 8–14. https://doi.org/10.1177/004728759403300202
- Neuts, B., Chen, J. M., & Nijkamp, P. (2016). Assessing customer value in segmented cruise markets: A modelling study on Japan and Taiwan. Academy of Marketing Studies Journal, 20(3), 1–10. https://www.abacademies.org/articles/amsjvol20no32016.pdf.
- Pardey, P. G., Alston, J. M., Chan-Kang, C., Hurley, T. M., Andrade, R. S., Dehmer, S. P., Lee, K., & Rao, X. (2018). The shifting structure of agricultural R&D: Worldwide investment patterns and payoffs. In From agriscience to agribusiness (pp. 13–39). Springer.https://link.springer.com/chapter/10.1007/978-3-319-67958-7_2.
- Pardey, P. G., Andrade, R. S., Hurley, T. M., Rao, X., & Liebenberg, F. G. (2016). Returns to food and agricultural R&D investments in Sub-Saharan Africa, 1975–2014. Food Policy, 65, 1–8. https://doi.org/10.1016/j.foodpol.2016.09.009
- Pardey, P. G., Chan-Kang, C., Dehmer, S., Beddow, J. M., Hurley, T. M., Rao, X., & Alston, J. M. (2014). Investments in and the economic returns to agricultural and food R&D worldwide. In Agriculture and people (pp. 78–97). Elsevier.https://doi.org/10.1016/B978-0-444-52512-3.00045-0.
- Rao, X. (2018). Land fragmentation with double dividends–the case of Tanzanian agriculture. European Review of Agricultural Economics, 46(4), 609–635. https://doi.org/10.1093/erae/jby034
- Rao, X., Hurley, T. M., & Pardey, P. G. (2019). Are agricultural R&D returns declining and development dependent? World Development, 122, 27–37. https://doi.org/10.1016/j.worlddev.2019.05.009
- Rao, X., & Zhang, Y. (2020). Livestock insurance, moral hazard, and farmers’ decisions: A field experiment among hog farms in China. The Geneva Papers on Risk and Insurance-Issues and Practice, 45(1), 134–156. https://doi.org/10.1057/s41288-019-00151-9
- Riganti, P., & Nijkamp, P. (2008). Congestion in popular tourist areas: A multi-attribute experimental choice analysis of willingness-to-wait in Amsterdam. Tourism Economics, 14(1), 25–44. https://doi.org/10.5367/000000008783554785
- Rochet, J.-C., & Tirole, J. (2002). Cooperation among competitors: Some economics of payment card associations. The RAND Journal of Economics, 33(4), 549–570. https://doi.org/10.2307/3087474
- Rochet, J.-C., & Tirole, J. (2003). Platform competition in two-sided markets. Journal of the European Economic Association, 1(4), 990–1029. https://doi.org/10.1162/154247603322493212
- Rouwendal, J., Verhoef, E. T., & Knockaert, J. (2012). Give or take? Rewards versus charges for a congested bottleneck. Regional Science and Urban Economics, 42(1), 166–176. https://doi.org/10.1016/j.regsciurbeco.2011.08.011
- Saveriades, A. (2000). Establishing the social tourism carrying capacity for the tourist resorts of the East coast of the Republic of Cyprus. Tourism Management, 21(2), 147–156. https://doi.org/10.1016/S0261-5177(99)00044-8
- Sigala, M. (2018). Market formation in the sharing economy: Findings and implications from the sub-economies of Airbnb. In Social dynamics in a systems perspective (pp. 159–174). Springer. https://link.springer.com/chapter/10.1007/978-3-319-61967-5_9.
- Song, H., Chen, J. M., & Chen, Y. (2020). Mediating and moderating effects in golf tourism: Evidence from Hainan Island. Tourism Economics, https://doi.org/10.1177/1354816620902331.
- Song, C., Shi, S., Chen, J. M., & Li, X. (2020). Does inward FDI affect outward FDI in tourism in China? Tourism Economics, 26(2), 344–349. https://doi.org/10.1177/1354816619873530
- Song, C., Shi, S., Chen, J. M., Nijkamp, P., & Li, X. (2020). The influence of emigration on tourism outward foreign direct investment: Evidence from China. Journal of Travel Research, 59(4), 631–641. https://doi.org/10.1177/0047287519846430
- Starmer, C. (2000). Developments in non-expected utility theory: The hunt for a descriptive theory of choice under risk. Journal of Economic Literature, 38(2), 332–382. https://doi.org/10.1257/jel.38.2.332
- Tencent Interim Report. (2016). https://www.tencent.com/zhcn/articles/1500061 1495014502.pdf
- Van Ommeren, J., Van den Berg, G. J., & Gorter, C. (2000). Estimating the marginal willingness to pay for commuting. Journal of Regional Science, 40(3), 541–563. https://doi.org/10.1111/0022-4146.00187
- Von Neumann, J., & Morgenstern, O. (1947). Theory of games and economic behavior. Princeton University Press.
- Walsh, R. G., Sanders, L. D., & McKean, J. R. (1990). The consumption value of travel time on recreation trips. Journal of Travel Research, 29(1), 17–24. https://doi.org/10.1177/004728759002900105
- Wirtz, J., & Tang, C. (2016). Uber: Competing as market leader in the US versus being a distant second in China. Service Marketing: People Technology Strategy, 626–632. https://doi.org/10.1142/9781944659028_0019
- Yang, H., Kong, H. Y., & Meng, Q. (2001). Value-of-time distributions and competitive Bus services. Transportation Research Part E: Logistics and Transportation Review, 37(6), 411–424. https://doi.org/10.1016/S1366-5545(01)00009-6
- Zhang, Y., Rao, X., & Wang, H. H. (2017). Organization, technology and management innovations through acquisition in China’s pork value chains: The case of the Smithfield acquisition by Shuanghui. Food Policy, 83, 337–345. https://doi.org/10.1016/j.foodpol.2017.08.004