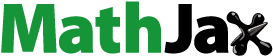
Abstract
Objective: To investigate preferences for fertility treatment from the Australian general population with the aims of calculating the willingness to pay in tax contribution for attributes (characteristics) that make up treatment and for an “ideal” fertility treatment program. We also assessed whether willingness-to-pay varies by the relationship status or sexual orientation of the patient.
Methods: A stated preference discrete choice experiment was administered to a panel of 801 individuals representative of the Australian general population. Seven attributes of fertility treatment under three broad categories were included: outcome, process, and cost. Attributes were identified through published literature, focus group discussions, expert knowledge, and a pilot study. A Bayesian fractional experimental design was used, and data analysis was performed using a generalized multinomial logit model. Further analyses included interaction terms and latent class modeling.
Results: Six of the seven attributes influenced the choice of a treatment program. Under process attributes, individuals preferred: continuity of care of clinic staff, where patients are seen by the same doctor but different nurses at each visit; “alternative” treatments being offered to all patients; and onsite clinic counseling and peer-support groups. Personalization and tailoring of the treatment journey were not important. Among outcome attributes, the improved success rate of having a baby per cycle and significant side-effects were considered important. Cost of treatment also influenced the choice of treatment program. Individual preferences for fertility treatment were not associated with patients’ relationship status or sexual orientation. Latent class modeling revealed sub-groups with distinct fertility treatment preferences.
Conclusion: This study provides important insights into the attributes that influence the preferences of fertility treatment in Australia. It also estimates socially-inclusive willingness-to-pay values in tax contributions for an “ideal” package of treatment. The results can inform economic evaluations of fertility treatment programs.
Background
Infertility affects ∼15% of couples at any one time, causing significant personal suffering to an estimated 50 million couples worldwideCitation1. The treatment for infertility has been revolutionized over the last generation with the introduction of assisted reproductive technologies (ART), including in vitro fertilization (IVF), whereby oocytes (eggs) are fertilized outside of the body using partner or donor sperm and transferred into a woman’s uterus with the aim of achieving a pregnancy. At least 2.4 million ART cycles are performed each year globallyCitation2. Other fertility treatments include artificial insemination and ovulation induction. Artificial insemination involves inserting male sperm (partner or donor) into the woman’s uterus close to the time of ovulation. Ovulation induction involves taking hormonal tablets or sometimes injections to induce ovulation by encouraging eggs to develop in the ovaries and be released. Often ovulation induction and stimulation are also used during artificial insemination to increase the chance of pregnancy.
Public and third-party funding for fertility treatment varies extensively internationally. Australia has a relatively supportive funding environment for fertility treatment, with almost all ART and other forms of fertility treatments attracting a rebate through the universal health insurance scheme, Medicare. There are no restrictions to access or reimbursement based on female age or number of treatment cycles, which are criteria used to ration funding in most other countriesCitation3. For example, most European Union countries provide government support to fund a limited number of fertility treatment cycles with restrictions on eligibility based on number of cycles, age, sexual orientation, and relationship statusCitation4. In contrast, Japan provides income means-tested funding for a limited number of cycles, while no public funding for fertility treatment exists in the US, South America, and most developing countries. However, in recent years an increasing number of states in the US have introduced insurance mandates to cover the provision of fertility treatmentsCitation5–7.
The cost of a fresh embryo ART cycle in Australia ranges between $9,000 and $11,000 AUD with ∼ $6,000–$7,000 reimbursed to the patient through Medicare. Similarly the cost of an artificial insemination cycle in Australia is between $2,000 and $3,000 with ∼ $1,000–$2,000 reimbursed through MedicareCitation6. The total cost to taxpayers of funding fertility treatment in Australia is estimated to be $400 million AUD per year, or ∼ $30 per average Australian taxpayer. Whether this represents good value for money from a general population perspective is largely unknown, as is the societal preferences for funding fertility treatment.
A stated preference discrete choice experiment (SPDCE) approach is a survey-based technique which examines expected behavior and is a particularly well-suited methodology for exploring individuals’ preferences for fertility treatment, which has both substantial health and non-health outcomesCitation8–10. In a SPDCE, individuals are asked to consider a number of attributes (characteristics) of a healthcare intervention or treatment program and make trade-offs when making a choice between alternatives based on different combinations of attributes and levels. “Levels” in the context of a SPDCE are the different values that can be assigned to an attribute. The choices made reflect the opportunity cost which is fundamental in determining the value of an interventionCitation11. The SPDCE approach is based on random utility theory (RUT), which assumes that individuals make choices which give them the highest level of satisfactionCitation12, and the theory of economic value, which posits that individuals value any non-market priced commodity based on a bundle of its attributes as opposed to its whole consumption per seCitation13. When a cost or a proxy attribute for price is included as part of the model, it is possible to indirectly estimate willingness-to-pay (WTP) values for positively or negatively preferred attributes or their combination, through the marginal rate of substitution (MRS)Citation14–16. The MRS is calculated as the ratio of the statistically significant coefficients of the attributes of interest to the negative of the coefficient of the cost attributeCitation17.
A small number of studies in ART have used SPDCE to elicit preferences for various aspects of fertility treatmentCitation18,Citation19, but rarely has this approach been extended to estimate WTP for fertility treatment programs in its entirety. The aim of this study was to elicit the Australian general population’s preferences and WTP for fertility treatment, specifically to estimate WTP values for attributes that make up fertility treatments; to determine WTP for an “ideal” package of treatment to be offered to Australians based on the current level of public insurance expenditure on fertility treatment; and to determine if WTP varies by the relationship status (single or couple) or sexual orientation of the patient receiving treatment.
Methods
Attributes and levels
Seven fertility treatment attributes, each with three possible levels, were identified through qualitative workCitation20–23. The methods for the qualitative work have been described previouslyCitation24. In summary, it included reviewing relevant published literature, two focus group discussions (FGDs) with members of the general population (n = 17). A third FGD was conducted with fertility patients attending a large fertility clinic in Sydney, Australia (n = 4).
Published literature revealed three broad categories of attributes for fertility treatment: outcome attributes related to the quality of treatment as determined by its effectiveness and safetyCitation25–32; process attributes concerned with the delivery of treatment which include, among other things, patient-centeredness, provision of information on treatment, continuity and transition of care, patient’s involvement in decision-making about treatmentCitation25,Citation26,Citation28,Citation32–37; and cost attribute related to how fertility treatment is fundedCitation38–41. These findings were supported by information provided from the FGDs and expert consultations.
All FGD participants were recruited through poster advertisement on notice boards in public places (for the general public) and on the fertility clinic’s notice board (for patients). Participants in the FGDs were required to be aged 18 years or older, Australian citizens or residents, and able to speak English. For the IVF clinic FGD, participants had to also be considering, receiving or had previously received fertility treatment. Interested individuals responded to the FGD advertisements by contacting the researchers through a dedicated email address and were screened for eligibility. FGDs used the nominal group techniqueCitation42 to identify and rank attributes of fertility treatment. FGDs lasted ∼1 h, with discussions audio recorded for later transcription without identifying information. The transcribed data were analyzed thematically into the three broad categories of fertility treatment attributes using NVivo software version 11Citation43. Subsequently, the results from the FGDs were consolidated and supplemented by expert opinion through interviews with two fertility doctors, one fertility nurse and one women’s health academic on what they perceived as key characteristics of fertility treatment.
The appropriateness of the identified list of attributes and levels was further validated through an online pilot study with members of the general population (n = 52) recruited by a commercial survey company, Survey Sampling International, SSI (https://www.surveysampling.com/). SSI used members of its general population panels of 100,000 members following the same inclusion criteria as with the FGDs of the general population. The commercial survey panels provided a convenient and engaged sample that mirrored the demographics of the Australian general population in terms of age and sex, Australian Bureau of Statistics (ABS) 2016 Census demographic distribution, as shown in , for the final survey.
Table 1. Socio-demographic characteristics of respondents participating in the SPDCE.
The identified attributes from the different qualitative components were iteratively revised for clarity, until the researchers reached consensus on their framing and wording. The attributes with their associated levels are presented in . The attribute-levels were designated based on good practice principles of SPDCE designCitation44,Citation45.
Table 2. Identified attributes and levels of a fertility treatment program.
The derivation of WTP values used the cost attribute which was framed as the additional amount of tax that a taxpayer would have to pay to fund fertility treatment for all patients in a year, reflecting the way the general population funds fertility treatment in Australia. Currently, the total Medicare Benefit Scheme (MBS) and Pharmaceutical Benefits Scheme (PBS) expenditure for ART is ∼ $250,000,000 and $150,000,000 AUD per year, respectively. With an estimated 14,000,000 taxpayers, a taxpayer contributes on average ∼ $30 AUD per year as part of the Medicare levy towards fertility treatment.
Experimental design
The seven attributes, each with three levels, resulted in 2,187 choice scenarios (= 37) in the full factorial experimental design. This number of choice scenarios would be cumbersome and not feasible to be presented to respondents in a surveyCitation17,Citation46,Citation47. Hence, we followed the recommended practice of using a statistically reduced experimental design that is still capable of estimating the main effect in the SPDCE modelCitation48–50. A Bayesian fractional experimental design in Ngene softwareCitation51 was used, which generated 12 choice scenarios based on the priors from a pilot study which used a near-orthogonal fractional designCitation52.
Questionnaire construction and data collection
The output of the experimental design was used to construct the SPDCE questionnaire. Given our sample population, we framed the preference elicitation questions following the conceptual framework of Dolan et al.Citation53, which resulted in obtaining socially-inclusive preferences for fertility treatment from an ex-ante context. is an example of the choice set and its preference elicitation question.
As with the pilot study, an online SPDCE was administered to a sample of the Australian population (n = 801) by a commercial survey company, Survey Sampling International (SSI). Respondents were English speaking Australian citizens or residents. The sample was drawn from SSI’s general population panel members and was selected to reflect at least the gender, age, and marital status distribution of the Australian general population aged 18 years and older, as presented in .
Sample size calculations for a SPDCE is a developing field of research in healthcare. There is no consensus or guidance on the appropriate sample size for a SPDCE. Several factors, such as the format of the questionnaire, the complexity of the choice tasks, the desired precision of results, and the need for sub-group analysis determine the minimum sample size to be usedCitation54. Recently, de Bekker-Grob et al.Citation55 proposed an approach to calculating the sample size for a SPDCE but it requires prior information or knowledge about some parameters, such as expectations of the relative sizes of the preference weights to be estimated, for which we had no information. Previous studies have shown that sample sizes of 40–100 respondents may be enough for reliable statistical analysisCitation56. OrmeCitation57 proposes a “rule of thumb” of using 300 respondents or more for robust results, and a minimum of 200 per group for sub-group analysisCitation54,Citation58. Researchers commonly use Orme’sCitation57 “rule of thumb”, such that we considered a sample size of above 800 to yield enough observations for robust results for our study on the basis that it is considerably larger than the recommended guidance.
Respondents were provided with background information about fertility treatments and how they are funded in Australia, and then asked the following:
Thinking about the characteristics of fertility treatment programs described, which of the following programs—Treatment A or Treatment B—would you prefer others, or yourself, had access to should it be needed to help have a baby. You may also select “Neither” of the options if you would not choose either.
Each participant completed 12 hypothetical choice scenarios constructed from the experimental design where a fertility treatment program varied on attribute-levels. A series of socio-demographic questions was also included, together with a practice question to familiarize the participants with the survey formatCitation9,Citation16,Citation59,Citation60. With the cost of fertility treatment attribute included, attribute trade-offs reveal how much individuals are willing to pay for marginal changes in the attributesCitation14–16,Citation61,Citation62. The experimental design allowed some attribute-levels to be the same in the scenarios being compared (attribute-level overlap) which reduced respondents’ task complexity and facilitated trading-off of attributesCitation63,Citation64. This study is part of a wider project that has been previously describedCitation24 for which ethical approval was obtained from the University of New South Wales, Sydney (HC17255).
Econometric model
Within the random utility theory (RUT) framework, the utility that an individual i derives from choosing alternative j (Treatment A, B, or Neither) in choice set t is given by:
where
is the observed utility
is a vector of variables representing attributes of alternative j, and
is a vector of coefficients.
is the deterministic component, assumed to be linear and additive,
is the random componentCitation17,Citation65,
is the number of respondents, and
is the number of choice scenarios. In this study, the deterministic component is represented as:
where
to
are preference parameters or marginal utilities of individuals for given levels of attributes with reference to the base level. A choice outcome variable was created as a dichotomous dependent variable to signify the choice decision made for each fertility treatment program scenario, with the independent variables being the combinations of each attribute-level. Effects coding was used for all categorical attributes to allow effect of each attribute to be uncorrelated with the constant, while continuous attributes assumed their valuesCitation66. Coding of “Neither” option was the same throughout choice sets.
We progressed from Model 1, the classical conditional logit (clogit) model, also known as multinomial logit (MNL) (see Supplementary Appendix), which estimated fixed effects with the assumption of homogeneous preferences across respondentsCitation67,Citation68 to Model 2a, a generalized multinomial logit (GMNL) modelCitation69–71. The GMNL model is an extension of the commonly used mixed logit (MXL) model which accounts for both taste and scale heterogeneity. Taste heterogeneity implies that individuals’ preferences for observed attributes are different, while scale heterogeneity is the non-consistency of individuals’ choices between attributes. In the GMNL model, all parameters were specified as random and normally distributed except cost parameter, which was assumed to be fixed. This specification avoids undefined moments and heavily skewed distributions when calculating WTP values through marginal rate of substitution (MRS)Citation72–74. An additional model (Model 2b) was estimated where Model 2a was extended to include an interaction which estimated fixed effects of responses to the question on whether fertility treatment should be available to same-sex or single individuals and all the attributes. Latent class (LC) analysis (Model 3) with discrete distribution of parameters across individuals was also undertaken to identify classes with similar preferences, but different across classesCitation17,Citation65. The optimal number of model classes is theoretically unknown and was determined through the estimation of a series of models with an increasing number of classes from 2 to 10Citation72. An iterative procedure was used to retain four classes based on comparisons of the Log-likelihood (LL), Akaike, Consistent Akaike, and Bayesian Information Criteria (AIC,CAIC and BIC) after which an additional class did not significantly improve the model fitCitation75. Moving from the base MNL model to more advanced GMNL and LC models resulted in an improved model fit, as shown by the LL, AIC, and BIC scores in the lower section of Citation76.
Table 3. GMNL and LC model results.
This SPDCE was unlabeled, hence we included an alternative specific constant for choosing treatment program A as ASCa and the “Neither” option as ASCn. These parameters were specified as random with normal distribution and allowed us to capture the average effect on utility for a systematic tendency of choosing treatment program A and “Neither” option, respectivelyCitation73.
Data analysis
All analyses were performed in Stata 14Citation77. The estimated coefficients and their statistical significance indicate individuals’ relative strengths of preferences for the different fertility treatment attribute-levels, where more preferred attribute-levels have higher preference weights. Changes in the preference weights between the most and least preferred levels of each attribute can be interpreted as the importance of an attribute relative to that of all other attributes, conditional on the ranges for each attributeCitation78. A positive coefficient implies that the change in the attribute-level increases the utility derived and the converse for the negative coefficient.
Subsequently, WTP for attributes of fertility treatment were indirectly calculated using the MRS. The positive/negative WTP estimate indicates individual’s willingness to pay/to be compensated for a change in a given attribute level, all factors being held constant. The 95% confidence intervals (CIs) for WTP values were derived using the delta methodCitation79, and the WTP values are reported in 2018 Australian dollars.
Results
Survey respondents
A total of 801 respondents completed the SPDCE survey in June 2018, which resulted in 28,836 observations. The average time spent to complete the questionnaire was 9.46 min. A total of 27% chose the “Neither” option, while 73% made at least a choice of a treatment program across all choice scenarios. Those who did not want to have children in future, were not on full-time work, and those without private insurance were more likely to choose none of the treatment programs presented to them in the choice scenarios. Most respondents (83%) indicated that the SPDCE questionnaire was very easy, easy, or OK to complete. There were slightly more females (51%) than males, and 45% were aged between 18 and 40 years. Most respondents had children (56%). presents the socio-demographic characteristics of the respondents.
SPDCE results
reports estimates for the GMNL model (Model 2a), an extension of the GMNL to include interaction effects (Model 2b) and the latent class model (Model 3). The results of the preliminary MNL model 1 are presented in the Supplementary Appendix. The estimated coefficients in the models are log-odds associated with each attribute and can be interpreted as relative individual preference weights. The size and sign of the coefficients indicate the extent of individual preference for the attributes.
In Model 1, the ASCa was statistically insignificant, suggesting that, holding all other factors constant, individual choices of a treatment program were not influenced by the systematic tendency to choose only the first alternative (Treatment Program A). The ASCn was positive and statistically significant, which implies that respondents derived utility in choosing the “Neither” option. At least one level of all seven attributes was statistically significant at ρ < 0.001 which implies that they were considered important when choosing a treatment program.
Of interest are the results in Models 2a, 2b, and 3. The ASCa coefficients were statistically insignificant for these models. The ASCn coefficients related to choosing “Neither” option were also insignificant, except for class 1, 2, and 3 of Model 3. The positive and statistically significant ASCn suggest utility derivation from the “Neither” option and the converse for the negative significant ASCn, all other factors being the same.
In Model 2a, at least one level of only six attributes influenced individuals’ choice of a fertility treatment program. Examining process attributes first, continuity of care of clinic staff where patients are “seen by the same doctor but different nurses” at each visit, relative to “seen by different doctors and nurses” each visit, was preferred the most. This was followed by a treatment program that offers “alternative” treatments to all patients compared to a treatment program that offered none, and onsite clinic counseling and peer-support groups compared to non-provision of information, in that order. The extent that the treatment journey is personalized and tailored for each patient did not influence the treatment program choice decision. Improved success rate (likelihood) of having a baby per cycle was considered most important. Both moderate and significant side-effects had a strong negative association with the decision to choose a treatment program.
Cost of treatment was also an important consideration. The scale parameters from Models 2a and 2b were statistically significant (Model 2a = 0.7079, ρ < 0.001 and Model 2b = 0.6929, ρ < 0.001), indicating the presence of scale heterogeneity. Holding all other attributes constant in Model 2a (GMNL model), the ratio of treatment preferences to cost of treatment (marginal rate of substitution) revealed that respondents were willing to pay an additional annual tax contribution over and above what they pay now of:
$10.66 (95% CI = $6.60-$14.73) for continuity of care of clinic staff, where patients are seen by the same doctor but different nurses at each visit;
$10.12 (95% CI = $5.51–$14.73) for a treatment program that offers “alternative” treatments to all patients;
$4.42 (95% CI = $1.87–$6.98) for onsite clinic counseling and peer-support groups; and
$2.23 (95% CI = $1.64–$2.82) for a 1% improvement in the success rate per cycle of having a baby.
Respondents had a considerable disutility for a treatment program with significant side-effects which cause major problems performing usual activities’ relative to negligible side-effects, such that they were willing to pay an additional annual tax of $55.38 (95% CI = $39.64–$71.13) to avoid significant side-effects.
There was little evidence to suggest that the general population’s preferences for fertility treatment differed by patients’ sexuality or relationship status.
Latent class modeling (Model 3) revealed similar preference class shares for fertility treatment that were different across classes of 18% (class 1), 18% (class 2), 48% (class 3), and 16% (class 4). There was no systematic tendency of choosing treatment program A in all the four classes. Individuals in class 1 derived utility in choosing “Neither” option compared to a treatment program. On the other hand, classes 2 and 3 preferred choosing at least a treatment program, while class 4 individuals appear not to have cared about the “Neither” option. Individuals in classes 1–3 were influenced by outcome attributes. They exhibited a dislike for a treatment program with significant side-effects relative to negligible side-effects, and considered improved success rate per cycle of having a baby as important. Individuals had varied preferences related to process attributes. Class 1 preferred a treatment program with continuity of care of clinic staff, where patients are seen by the same team of fertility doctors and nurses each visit compared to being seen by different doctors and nurses each visit, and a treatment program that offers “alternative” treatments to all patients compared to one that does not. Only continuity of care of clinic staff where patients are seen by the same doctor but different nurses at each visit relative to having different doctors and nurses each visit was considered important by class 2 individuals. Class 3 preferred continuity of care of clinic staff where patients are seen by the same team of fertility doctors and nurses each visit compared to different doctors and nurses each visit and a treatment journey with some level of personalized treatment based on upfront tests compared to having the same treatment pathway for all patients. Only class 2 individuals cared about cost of treatment. On the other hand, individuals in class 4 (16%) did not consider any of the attributes important, and perhaps did not care about fertility treatment in general. presents overall preferences for a fertility treatment program from results of latent class analysis. The standard deviations relative to the coefficients of ASCn, moderate, and significant side-effects, and success rate per treatment cycle for Models 2a and 2b were statistically significant, implying significant variability in preferences for these attributes.
Table 4. Results of latent class analysis.
Discussion
This study found that societal preferences for attributes of a fertility treatment program and subsequently their WTP are influenced mostly by process and outcome attributes. For process attributes the level of continuity of care of clinic staff, whether “alternative”/experimental treatments are offered, and whether there is counseling and peer-support groups were the most important, in that order. For outcome attributes, improved success rate (likelihood) of having a baby per cycle and side-effects were considered important. An increase in the cost of treatment would result in disutility for a treatment program. This study has also found that those who did not want to have children in the future, were not in full-time work, and those without private insurance were more likely to choose none of the configuration of the treatment program scenarios presented to them.
This study has produced WTP estimates of the attributes of a fertility treatment program from a socially-inclusive Australian general population perspective and an ex-ante context. These WTP estimates can be used in an economic evaluation of fertility treatment to assess value for money for the public investment in fertility treatment. For example, a cost-benefit analysis framework where the marginal WTP estimates or the total of the marginal WTP estimates for the preferred attributes or most preferred configuration of fertility treatment program could be compared with the cost of delivering those attributes, if knownCitation80–82. The total of the marginal WTP estimates can be derived using the compensating variation (CV) approach which accounts for the probability of making a choice, hence consistent with the RUT framework and has a solid basis in welfare theoryCitation83,Citation84.
The common assumption used in healthcare when applying the CV approach to an unlabeled SPDCE is that individuals have a preference for one treatment over another, which would be attributed solely to differences in one or more attributes of that treatmentCitation68,Citation85. Hence, there is certainty in choosing the treatment, with the probability of making a choice being one. In this case, the CV approach reduces to the sum of marginal WTP for each attributeCitation80.
On this basis, this study has found that a representative sample drawn from the Australian general population, as shown in (at least by gender, age distribution, marital status), is willing to pay an additional $27.43 in annual tax contribution, to fund the preferred configuration of a fertility treatment program for everyone who might benefit from treatment in Australia. Currently, in Australia, on an average $30 per year per taxpayer from the Medicare levy subsidizes fertility treatment with undefined attributes for everyone who needs it. The total cost to Australian taxpayers is around $400 million. The societal WTP of $27.43 as an additional annual average tax contribution to fund the “ideal” fertility treatment program translates to about a 90% increase from the current level. Given that respondents were told that the average Australian taxpayer contributes about $30 per year to Medicare towards providing fertility treatment to all Australian citizens and residents, these WTP estimates indicate the current level of funding for fertility treatment is viewed favorably, and that society would be willing to pay more in tax for an “ideal” package of treatment to be offered to Australians.
Individuals preferred a fertility treatment program with the following process attributes: continuity of care of clinic staff where patients are seen by the same doctor but different nurses at each visit compared to having different doctors and nurses each visit, “alternative” treatments offered to all patients rather than none, onsite clinic counseling and peer-support groups compared to non-provision of information counseling and peer-support, and with the following outcomes attributes: improved success rate of having a baby per cycle, and without significant side-effects relative to negligible side-effects. Cost of treatment also influenced the choice of a treatment program such that the marginal utility of increases in cost was negative. There was little evidence to suggest that individual preferences for fertility treatment vary according to relationship status (single or couple) or sexual orientation of the patient being treated. The results of the latent class analysis revealed that the general population inevitably consists of sub-groups with different preferences, which are different across the groups.
This research makes a considerable contribution to informing policy and resource allocation decisions related to the provision of fertility treatment. However, as is the case with all SPDCEs, there are methodological limitations such that the results should be interpreted with caution. It is impractical and cognitively not plausible to include all relevant attributes and levels for fertility treatment in the SPDCE; however, the qualitative and pilot stages of the study were used to identify the most relevant attributes for inclusion. In addition, this study was undertaken in Australia, where funding for fertility treatment is subsidised by government through Medicare, hence the absolute results are not directly transferable to other settings. It is further acknowledged that the SPDCE approach has inherent hypothetical biasCitation86. In this regard, effort was made to mitigate hypothetical bias by reminding respondents to make choices between the hypothetical scenarios of fertility treatment presented to them as they would in real life. The use of a market research panel sample of a survey company may also be a drawback because they are rewarded for taking part. However, it is argued that professional respondents of a panel are more attentive and responsive, hence engage with the survey, than volunteersCitation87.
Conclusion
To our knowledge, this is the first study that has attempted to elicit preferences and subsequently calculated WTP values for attributes of a package of fertility treatment. This study provides important insights into the attributes that influence the choice of a fertility treatment program from the perspective of the Australian general population. It further produces socially-inclusive WTP estimates for preferred fertility treatment attributes and treatment programs which can be used in economic evaluations, such as cost-benefit analyses to assess the value for money of fertility treatment programs. The results will contribute to the development of an evidence-based policy framework for the provision of cost-effective and patient-centered fertility treatment in Australia.
Transparency
Declaration of funding
This paper is part of the study being funded by the Australian National Health and Medical Research Council (NHMRC) project grant AP1104543 and has been approved by the University of New South Wales Human Research Ethics Committee (HEC 17255).
Declaration of financial/other interests
WB, ND, MS, RN, and GMC declare that they have no conflicts of interest. JME peer reviewers on this manuscript have no relevant financial or other relationships to disclose.
Supplemental Material
Download MS Word (23.9 KB)Acknowledgments
We wish to thank members of the public and patients who agreed to participate in our focus group discussions which informed the design of this study. We also thank Professor Denzel Fiebig of the University of New South Wales, whose comments on the first draft assisted greatly in the revision of this paper.
References
- Mascarenhas MN, Flaxman SR, Boerma T, et al. National, regional, and global trends in infertility prevalence since 1990: a systematic analysis of 277 health surveys. PLoS Med 2012;9(12):e1001356
- Adamson GD, de Mouzon J, Chambers GM, et al. International Committee for Monitoring Assisted Reproductive Technologies. World report on assisted reproductive technologies, 2011. Fertil Steril 2018;110(6):1067–1080
- Chambers GM, Sullivan EA, Ishihara O, et al. The economic impact of assisted reproductive technology: a review of selected developed countries. Fertil Steril 2009;91(6):2281–94
- Berg Brigham K, Cadier B, Chevreul K. The diversity of regulation and public financing of IVF in Europe and its impact on utilization. Hum Reprod 2013;28(3):666–75
- Connolly MP, Hoorens S, Chambers GM. The costs and consequences of assisted reproductive technology: an economic perspective. Hum Reprod Update 2010;16:603–13
- Chambers GM, Zhu R, Hoang V, et al. A reduction in public funding for fertility treatment - an econometric analysis of access to treatment and savings to government. BMC Health Serv Res 2012;12:142
- Resolve. US National Infertility Association. Insurance Coverage. 2018. Available at: https://resolve.org/what-are-my-options/insurance-coverage/infertility-coverage-state/ [Last accessed October 2018]
- Devlin N, Parkin D. Funding fertility: issues in the allocation and distribution of resources to assisted reproduction technologies. Hum Fertil 2003;6(supp 2):S2–S6
- de Bekker-Grob EW, Ryan M, Gerard K. Discrete choice experiments in health economics: a review of the literature. Health Econ 2012;21:145–72
- Settumba SN, Shanahan M, Botha W, et al. Reliability and validity of the contingent valuation method for estimating willingness to pay: a case of in vitro fertilisation. Appl Health Econ Health Policy 2018
- Briggs A. A view from the bridge: health economic evaluation—a value-based framework? Health Econ 2016;25:1499–502
- McFadden D. Conditional logit analysis of qualitative choice behavior. in Frontiers in econometrics, P. Zarembka, (Ed.). 1974, Academic Press. p. 105–142
- Lancaster KJ. A new approach to consumer theory. J Polit Econ 1966;74:132–57
- Ryan M, Gerard K, Amaya-Amaya M. Discrete choice experiments in a nutshell, in using discrete choice experiments to value health and health care. Dordrecht: Springer Netherlands; 2008. P. 13–46
- McIntosh E, Clarke P, Frew E, et al. Applied methods of cost-benefit analysis in health care. Oxford: Oxford University Press; 2010
- Ryan M, Kolstad J, Rockers P, et al. How to conduct a discrete choice experiment for health workforce recruitment and retention in remote and rural areas: a user guide with case studies, in CapacityPlus. Geneva: World Bank and World Health Organization; 2012
- Hensher D, Rose J, Greene W. Applied choice analysis analysis. 2nd ed. Cambridge: Cambridge University Press; 2015
- van Empel IW, Dancet EA, Koolman XH, et al. Physicians underestimate the importance of patient-centredness to patients: a discrete choice experiment in fertility care. Human Reprod 2011;26:584–93
- Van den Wijngaard L, Rodijk I, van der Veen F, et al. Patient preference for a long-acting recombinant FSH product in ovarian hyperstimulation in IVF: a discrete choice experiment. Human Reprod 2014;30:331–7
- Coast J, Al-Janabi H, Sutton EJ, et al. Using qualitative methods for attribute development for discrete choice experiments: issues and recommendations. Health Econ 2012;21:730–41
- Vass C, Rigby D, Payne K. The role of qualitative research methods in discrete choice experiments. Med Decis Making 2017:37(3):298–313
- Ikenwilo D, Heidenreich S, Ryan M, et al. The best of both worlds: an example mixed methods approach to understand men’s preferences for the treatment of lower urinary tract symptoms. Patient 2018;11(1):55–67
- Katz DA, Stewart KR, Paez M, et al. Development of a discrete choice experiment (DCE) questionnaire to understand veterans’ preferences for tobacco treatment in primary care. Patient 2018;11(6):649–663
- Botha W, Donnolley N, Shanahan M, et al. Assessment of the societal and individual preferences for fertility treatment in Australia: study protocol for stated preference discrete choice experiments. BMJ Open 2018;8:e020509
- Aarts JWM, Huppelschoten AG, van Empel IWH, et al. How patient-centred care relates to patients' quality of life and distress: a study in 427 women experiencing infertility. Hum Reprod 2012;27:488–95
- van Empel IWH, Aarts JWM, Cohlen BJ, et al. Measuring patient-centredness, the neglected outcome in fertility care: a random multicentre validation study. Hum Reprod 2010;25:2516–26
- van Empel IWH, Dancet EAF, Koolman XHE, et al. Physicians underestimate the importance of patient-centredness to patients: a discrete choice experiment in fertility care. Hum Reprod 2011;26:584–93
- Dancet EAF, Nelen WLDM, Sermeus W, et al. The patients’ perspective on fertility care: a systematic review. Hum Reprod Update 2010;16:467–87
- Palumbo A, De La Fuente P, Rodríguez M, et al. Willingness to pay and conjoint analysis to determine women’s preferences for ovarian stimulating hormones in the treatment of infertility in Spain. Hum Reprod 2011;26:1790–8
- Porter R, Kissel C, Saunders H, et al. Patient and nurse evaluation of recombinant human follicle-stimulating hormone administration methods: comparison of two follitropin injection pens. Curr Med Res Opin 2008;24:727–35
- Dancet EAF, D'Hooghe TM, Spiessens C, et al. Quality indicators for all dimensions of infertility care quality: consensus between professionals and patients. Hum Reprod 2013;28:1584–97
- Mourad SM, Nelen WLDM, Akkermans RP, et al. Determinants of patients’ experiences and satisfaction with fertility care. Fertil Steril 2010;94:1254–60
- Cousineau TM, Green TC, Corsini E, et al. Online psychoeducational support for infertile women: a randomized controlled trial. Hum Reprod 2008;23:554–66
- van Empel IWH, Nelen WLDM, Tepe ET, et al. Weaknesses, strengths and needs in fertility care according to patients. Hum Reprod 2010;25:142–9
- Silva S, Barros H. Perspectives on access to in vitro fertilization in Portugal. Rev Saude Publica 2012;46:344–50
- Dancet EAF, D'Hooghe TM, Sermeus W, et al. Patients from across Europe have similar views on patient-centred care: an international multilingual qualitative study in infertility care. Hum Reprod 2012;27:1702–11
- Malin M, Hemminki E, Räikkönen O, et al. What do women want? Women’s experiences of infertility treatment. Soc Sci Med 2001;53:123–33
- Van Hoof W, Provoost V, Pennings G. Reflections of Dutch patients on IVF treatment in Belgium: a qualitative analysis of internet forums. Hum Reprod 2013;28:1013–22
- Iaconelli A, Jr, Setti AS, Braga DPAF, et al. Main concerns regarding in vitro fertilization techniques: results of a website survey. Fertil Steril 2013;100:S66
- Chambers GM, Adamson GD, Eijkemans MJ. Acceptable cost for the patient and society. Fertil Steril 2013;100:319–27
- Klitzman R. How much is a child worth? Providers’ and patients’ views and responses concerning ethical and policy challenges in paying for ART. PloS One 2017;12:e0171939
- Hiligsmann M, van Durme C, Geusens P, et al. Nominal group technique to select attributes for discrete choice experiments: an example for drug treatment choice in osteoporosis. Patient Prefer Adher 2013;7:133
- QSR International Pty Ltd, NVivo qualitative data analysis Software version 11. Melbourne, Australia; 2012
- Kløjgaard ME, Bech M, Søgaard R. Designing a stated choice experiment: the value of a qualitative process. J Choice Model 2012;5:1–18
- Ratcliffe J, Longworth L. Investigating the structural reliability of a discrete choice experiment within health technology assessment. Int J Tech Assess Health Care 2002;18:139–44
- Viney R, Savage E, Louviere J. Empirical investigation of experimental design properties of discrete choice experiments in health care. Health Econ 2005;14:349–62
- Lancsar E, Louviere J. Conducting discrete choice experiments to inform healthcare decision making. Pharmacoeconomics 2008;26:661–77
- Rose JM, Bliemer MC. Constructing efficient stated choice experimental designs. Transport Rev 2009;29:587–617
- Pfarr C, Schmid A, Schneider U. Using discrete choice experiments to understand preferences in health care. Health Care Provision and Patient Mobility 2014;12:27–48
- Louviere JJ, Hensher DA, Swait JD. Stated choice methods: analysis and applications. Cambridge: Cambridge University Press; 2000
- Choicemetrics. Ngene 1.2: User manual and reference guide. Sydney, Australia: Choice Metrics Pty Ltd.; 2018
- Hensher DA. Stated preference analysis of travel choices: the state of practice. Transportation 1994;21:107–33
- Dolan P, Olsen JA, Menzel P, et al. An inquiry into the different perspectives that can be used when eliciting preferences in health. Health Econ 2003;12:545–51
- Marshall D, Bridges JP, Hauber B, et al. Conjoint analysis applications in health—how are studies being designed and reported? Patient 2010;3:249–56
- de Bekker-Grob EW, Donkers B, Jonker MF, et al. Sample size requirements for discrete-choice experiments in healthcare: a practical guide. Patient 2015;8:373–84
- de Bekker-Grob E, Bliemer M, Donkers B, et al. Patients’ and urologists’ preferences for prostate cancer treatment: a discrete choice experiment. Br J Cancer 2013;109:633–40
- Orme B. Getting started with conjoint analysis: strategies for product design and pricing research. Madison, WI: Research Publishers; 2006
- Rose J, Bliemer MJ. Sample size requirements for stated choice experiments. Transportation 2013;40:1021–41
- Bennett J. Some fundamentals of environmental choice modelling. Research report no.11. Australia: School of Economics and Management, University of New South Wales; 1999
- Ryan M, Gerard K. Using discrete choice experiments to value health care programmes: current practice and future research reflections. Appl Health Econ Health Policy 2003;2:55–64
- Mentzakis E, Ryan M, McNamee P. Using discrete choice experiments to value informal care tasks: exploring preference heterogeneity. Health Econ 2011;20:930–44
- Kjaer T. A review of the discrete choice experiment-with emphasis on its application in health care. Denmark: Syddansk Universitet; 2005
- Flynn TN, Bilger M, Malhotra C, et al. Are efficient designs used in discrete choice experiments too difficult for some respondents? A case study eliciting preferences for end-of-life care. PharmacoEconomics 2016;1–12
- Flynn TN. Where next for discrete choice health valuation exercises? Soc Sci Res Network 2016. Available at: https://papers.ssrn.com/sol3/papers.cfm?abstract_id=2810444 [Last accessed 2 September 2018].
- Train KE. Discrete choice methods with simulation. Cambridge: Cambridge University Press; 2009
- Bech M, Gyrd‐Hansen D. Effects coding in discrete choice experiments. Health Econ 2005;14:1079–83
- Hauber AB, González JM, Groothuis-Oudshoorn CG, et al. Statistical methods for the analysis of discrete choice experiments: a report of the ISPOR conjoint analysis good research practices task force. Value Health 2016
- Lancsar E, Fiebig DG, Hole AR. Discrete choice experiments: a guide to model specification, estimation and software. PharmacoEconomics 2017;35:697–716
- Fiebig DG, Keane MP, Louviere J, et al. The generalized multinomial logit model: accounting for scale and coefficient heterogeneity. Market Sci 2010;29:393–421
- Gu Y, Hole AR, Knox S. Fitting the generalized multinomial logit model in Stata. Stata J 2013;13:382–97
- Vass CM, Wright S, Burton M, et al. Scale heterogeneity in healthcare discrete choice experiments: a primer. Patient 2018;11:167–73
- Hole AR. Discrete choice methods in health economics, in health econometrics. Bingley, United Kingdom: Emerald Publishing Limited; 2018. p. 85–99
- Hole AR, Kolstad JR. Mixed logit estimation of willingness to pay distributions: a comparison of models in preference and WTP space using data from a health-related choice experiment. Empir Econ 2012;42:445–69
- Revelt D, Train K. Mixed logit with repeated choices: households’ choices of appliance efficiency level. Rev Econ Stat 1998;80:647–57
- Goossens LM, Utens CM., Smeenk FW, et al. Should I stay or should I go home? A latent class analysis of a discrete choice experiment on hospital-at-home. Value Health 2014;17:588–96
- Hole AR. Modelling heterogeneity in patients’ preferences for the attributes of a general practitioner appointment. J Health Econ 2008;27:1078–94
- StataCorp, L. Stata data analysis and statistical Software. Release. 2015. 14
- González JM, Doan J, Gebben DJ, et al. Comparing the relative importance of attributes of metastatic renal cell carcinoma treatments to patients and physicians in the United States: a discrete-choice experiment. PharmacoEconomics 2018:1–14
- Hole AR. A comparison of approaches to estimating confidence intervals for willingness to pay measures. Health Econ 2007;16:827–40
- Carlsson F. Non-market valuation: stated preference methods. Oxford: Oxford University Press; 2011
- Drummond MF, Sculpher MJ, Claxton K, et al. Methods for the economic evaluation of health care programmes. Oxford: Oxford University Press; 2015
- van der Pol M, McKenzie L. Costs and benefits of tele-endoscopy clinics in a remote location. J Telemed Telecare 2010;16:89–94
- Lancsar E, Savage E. Deriving welfare measures from discrete choice experiments: inconsistency between current methods and random utility and welfare theory. Health Econ 2004;13:901–7.
- Karlström A. Appraisal. In: Hess S, Daly A, editors. Handbook of choice modelling. Cheltenham, United Kingdom: Edward Elgar Publishing; 2014. p 601–26
- Ryan M. Deriving welfare measures in discrete choice experiments: a comment to Lancsar and Savage (1). Health Econ 2004;13:909–12
- Fifer S, Rose J. Hypothetical bias in stated preference experiments: is it a problem? And if so, how do we deal with it? Transportation research part A: policy and practice 2014;61:164–177
- Ben-Akiva M, McFadden D, Train K. Foundations of stated preference elicitation consumer behavior and choice-based conjoint analysis. in Society for economic measurement annual conference. Paris, France; 2016. Available at: http://eml.berkeley.edu/∼train/foundations.pdf 97 Discrete Choice Methods in Health Economics [Last accessed 1 September 2018]