Abstract
Aim
In a randomized control trial mid-regional proadrenomedullin (MR-proADM)-guided decision-making has been proven to safely reduce hospital admissions based on an accurate assessment of disease severity in the Emergency Department (ED). This study aimed to assess the impact of a MR-proADM-Guided Triage (MR-GT) versus standard Hospital Triage (HT) on clinical and economic outcomes in ED patients with suspected infection in Spain, Italy, Germany, and the UK, using Patient-Level Data (PLD) from two observational studies.
Methods
PLD was collected from patients enrolled at a Spanish hospital during two observational studies. Logistic regression was used to identify predictors of hospitalization. A patient-level simulation model was subsequently developed to evaluate the clinical and economic impact of MR-GT versus HT using results from the statistical analysis and country-specific cost data from the published literature. Probabilistic and deterministic sensitivity analyses were carried out.
Results
Four hundred seventy-three patients were enrolled in this study. MR-proADM had the strongest association with hospital admission, followed by age and National Early Warning Score (NEWS). In the simulation model, MR-GT was associated with an overall reduction in hospitalization relative to HT, equal to 22.6 percentage points (40.9 vs. 63.5%). In addition, the use of MR-GT would reduce the total hospital cost per patient presenting to the ED with suspected infection by roughly 30%, with a mean cost saving per patient of €626, €1,484, €1,154, and £1,113 in Spain, Italy, Germany, and the UK, respectively. The robustness of these findings was confirmed by sensitivity analyses.
Limitations
The statistical analyses were not performed on the same population simulated in the model. Clinical input parameters were assumed to be the same for all countries.
Conclusions
MR-proADM showed to be the main predictor of hospitalization. An MR-proADM decision algorithm provides cost savings in Spain, Italy, Germany, and the UK.
Introduction
Emergency Department (ED) crowding represents a major global healthcare challenge, especially for acute infections even before the COVID-19 pandemicCitation1. The number of ED visits has increased dramatically in recent years, also due to having to manage patients with minor complaints that could be treated in an outpatient settingCitation2,Citation3. Along with limited resources, including the lack of hospital beds and staff shortages, an overcrowded ED impedes proper patient managementCitation4,Citation5. The literature also outlines many consequences of this phenomenon, such as a soaring clinical workload, which potentially leads to delayed diagnoses, late treatment, and prolonged hospitalizationCitation6–8. ED crowding is also associated with increasing healthcare costs exacerbating hospital resource allocation, especially due to patients’ boarding (i.e. length of stay in the ED after admission due to lack of hospital beds)Citation9. A retrospective study, carried out by Krochmal et al. in a US hospital, disclosed the soaring costs associated with inpatients remaining in the ED after admission. These patients were also found to have had a greater average length of stay compared to those who were promptly transferred to inpatient units, leading to an increase in costs of $6.8 million over 3 yearsCitation10.
Low Respiratory Tract Infections (LRTIs), Urinary Tract Infections (UTIs), Intra-Abdominal Infections (IAIs), and Skin and Soft Tissue Infections (SSTIs) are among the main causes of ED admissionsCitation11–14. In infected patients, a prompt assessment of the severity and the likelihood of further progression is crucial to providing adequate therapeutic strategies and avoiding more severe stages of the diseaseCitation15. If poorly managed, these infections can potentially lead to life-threatening conditions, sepsis being the most commonCitation16,Citation17. As highlighted in a study conducted by Paoli et al. in the US, costs per patient also increased with the worsening of disease and varied depending on whether sepsis was present or not at the time of admissionCitation18. Nonetheless, the same study disclosed higher total cost in the lowest severity cohort population, due to higher prevalence of milder forms of sepsis. Therefore, methods for early recognition of septicemia are pivotal to reducing the overall burden of sepsisCitation18. Although EDs are crucial for identifying septic patients, they lack objective diagnostic tests, both in terms of tissue diagnostics and serological testsCitation19,Citation20. Different scores, such as the Sequential Organ Failure Assessment (SOFA), and the quick SOFA (qSOFA) have been developed in this regard and are commonly used in the Hospital Triage (HT). While SOFA is characterized by being a cumbersome and complex score, its simplified version qSOFA has been shown to have significant sensitivity limitationCitation15, which could lead to delayed therapeutic response, especially in initially-low severity patientsCitation21,Citation22. Conversely, the unnecessary hospitalization of not-at-risk patients would lead to an increase in hospital occupancy and healthcare expenditure, as well as a higher risk of inappropriate therapy in high-severity patientsCitation23,Citation24. Thus, there is a clear public need for improving the diagnostic and prognostic approach to infections in the ED, to make safe ED discharge decisions, and to early detect potentially at-risk patientsCitation12,Citation19,Citation25–27.
The mid-regional fragment of proadrenomedullin (MR-proADM) has been proven to be an accurate biomarker in the prognosis of patients with a suspicion of infectionCitation28. As shown in the IDEAL pilot study, an MR-proADM-Guided Triage (MR-GT) improves patient workflow at EDs, especially in overcrowded conditions, reducing hospital admissions with no increase in mortalityCitation28. Therefore, the MR-GT would allow safe discharges, leading to a reduced economic burden and clinical workload of the hospitalsCitation29. In addition, it outperforms the established clinical scores in predicting 28-day mortality in septic patients, adding value in assessing the risk of disease progressionCitation15,Citation29,Citation30.
In this study, we analyzed Patient-Level Data (PLD) from two observational studies to investigate predictors of hospitalization in patients presenting to the ED with a suspected infection. In doing so, we also aimed to assess the clinical and economic benefits of an MR-GT in comparison with a standard HT in Spain, Italy, Germany, and the UK.
Methods
This study presents a multistage approach, comprised of a statistical analysis and an economic evaluation, to assess the impact of MR-GT versus standard HT on clinical outcomes and costs in patients presenting to the ED with suspected infections.
PLD analysis
Population
We retrospectively analyzed data from patients enrolled during two observational studiesCitation8,Citation15. The objective of these studies was to investigate the performance of blood biomarkers in patients presenting to the ED with suspected infection to assess potential disease progression and hospitalization decisionsCitation8,Citation15. The cohorts analyzed in the present study consist of all adult patients who presented to the ED of a Spanish hospital (Hospital Clínico San Carlos, Madrid) with clinical signs of infection and underwent standard ED assessment (standard HT, including routine laboratory and microbiology tests) during two time periods: from August 2016 to July 2017, and from May to July 2018. Collected data included demographic characteristics, comorbidities, biomarker levels, severity scores, hospitalization, and costs related to the enrolled patients. This dataset is referred to as the Spanish database in this manuscript. The research was performed in line with the principles of the Declaration of Helsinki: informed consent was obtained for all patients, and ethical approval was granted from the relevant governance bodies. For more details on the study design, inclusion and exclusion criteria, and data collection, we refer to the original papersCitation8,Citation15.
Study endpoints
The study endpoint is the assessment of predictors of hospitalization risk, defined as hospital admission with a stay of >24 h. In addition, for the subsequent development of the economic model, the following outcomes were also analyzed: Length of hospital Stay (LOS) for hospitalized patients—length of time from arrival in the ED to discharge; cost for hospitalization and ED discharge—full hospital expense including medical attention, ED cost, hospitalization resources, outpatient tariffs, consumables, and maintenance.
Statistical analysis
For the descriptive statistics, categorical variables were presented as absolute and relative frequencies while continuous variables were expressed as median and interquartile range (Q1–Q3). For normally distributed variables, mean and standard deviation (SD) were reported.
Univariate and multivariate analyses were used to identify the key predictors of hospitalization, LOS, and costs. A logistic regression analysis assessed the association of patients’ characteristics with the risk of hospitalization, whereas gamma regressions evaluated the association with the length of stay (identity link) and costs (log link). For each outcome, a series of univariate regression models were performed and significant covariates (p-value threshold assumed equal to .20) were introduced by a stepwise selection approach into a multivariate model. To increase the analysis stability, a logarithmic transformation was applied to biomarker values, whereas the other continuous variables have been normalized by taking their z-score. Demographic characteristics (age, sex, and infection source) were included in all the analyses as adjustment factors. The measures of association were expressed as Odds Ratio (OR) in case of logistic regression and as Multiplicative Factors (MF) or delta in case of gamma regression models (log and identity link, respectively). A p-value <.05 was considered statistically significant. All statistical analyses were performed with the statistical software R (version 4.1.2).
Economic evaluation
Overview
An economic evaluation was carried out to estimate the cost-effectiveness of MR-proADM-guided decision-making for patients presenting to the ED with suspected infection. To this purpose, we developed a patient-level simulation model in Microsoft Excel that compares two treatment alternatives: a conventional HT, which simulates routine clinical assessment, and an MR-GT, in which the hospitalization is determined exclusively by the MR-proADM level. The model was populated with real-world evidence and data from the published literature. In particular, the PLD from the two observational studies was used to inform the model development and to estimate its outcomes, including the hospitalization rate (in the HT) and the total costCitation8,Citation15. Given the high severity of the patients enrolled in these two studies, the model was subsequently calibrated to replicate the mid-low severity cohort with suspected infections enrolled in the IDEAL pilot study that demonstrated the clinical utility of an MR-GT in the EDCitation28. In this setting, where the decision to hospitalize is more challenging, MR-proADM establishes its highest potential.
Model structure
Ten thousand simulated patients, whose characteristics match those of the target population, were sent simultaneously through both arms which differed solely in the hospital admission criterion. In the MR-GT arm, the hospitalization was determined by the simulated MR-proADM level: with a value >0.87 nmol/L the patient is hospitalized, otherwise, the patient is discharged. In the HT arm, to mimic real-life hospitalization decision, the risk of hospitalization was estimated, for each patient, by adjusting the baseline risk for the demographic and clinical risk factors identified in the PLD analysis (Table S3).
The patient’s pathway is shown in : individuals are either admitted to the hospital or treated in the outpatient setting. In addition, patients initially discharged from the ED may subsequently be readmitted to the hospital (within 14 days). Since data on hospital readmission was not available in the PLD, the mean readmission rates were taken from Del Castillo et al. (7.1 and 10% in HT and MR-GT, respectively)Citation28 and sampled, in the simulation, from a Bernoulli distribution.
Clinical inputs
Modeled patients were assigned a set of baseline characteristics, sampled from their distributions, allowing the representation of individual‐level heterogeneity. To simulate a realistic cohort, the patient population was stratified by sex and infection source, and sampling in each stratum was performed according to the correlation structure identified in the Spanish database. In particular, copulas were used to model the dependence among continuous variablesCitation31. Statistics per strata and correlation analysis, related to the population used to generate the simulated cohort, are reported in Table S1 and Figure S1, respectively. This study focused on a cohort of mid-low severity patients; thus, input data were subsequently re-adjusted to mimic the characteristics of the target population, reproportioning the parameters of each stratum accordingly, based on the IDEAL pilot studyCitation28 (Table S2).
Costs
The economic evaluation was conducted in four European (EU) countries (Spain, Italy, Germany, and the UK) from the perspective of the hospital, associating a cost to each model state (hospitalization and outpatient treatment) that is independent of the treatment arm. Costs included in the analysis are those related to ED visits and hospitalizations, while costs related to the MR-proADM test and changes in the triage pathway were not considered in this study.
The cost of the ED visit (discharged patients) was retrieved from outpatient tariffs and the published literatureCitation32–35.
Hospitalization cost was calculated as the product of the length of stay and the ward cost per day. A multivariate gamma regression model, performed on the PLD (hospitalized patients), was employed to estimate the patient-specific LOS. The results of the statistical procedure are presented in Table S4. The Intensive Care Unit (ICU) cost was not included in this analysis, compatibly with the mid-low severity of the patient cohortCitation28. The average hospital cost per day was taken from a retrospective cost analysis for Spain, from the report of the National Agency for Regional Health Services for Italy, and from previous economic evaluations for Germany and the UKCitation36–39.
Unit costs, summarized in , were updated to 2022 using the Harmonized Indices of Consumer Price (HICP) for healthCitation40.
Table 1. Economic inputs.
In case of hospital readmission, both the costs of hospitalization and ED visits were considered, under the assumption that the variables affecting the length of stay do not change over time. Finally, for Spain, the total cost obtained in the two arms was split according to the distribution of cost breakdown, available for 26 Spanish patients (Figure S2).
Model validation and sensitivity analyses
Based on ISPOR Good Research PracticesCitation41, an external validation was performed by comparing the hospital admission rates observed in the IDEAL pilot trialCitation28 with those predicted by our model (as the baseline characteristics simulated in the model mimic the population enrolled in the trial). Additionally, an alternative cost calculation method was implemented to validate the economic results in the Spanish case. In this scenario, outpatient and hospital costs were directly estimated according to the cost data available in the Spanish database and updated to 2022 (Table S5). As such, the length of stay was sampled from a gamma distribution (mean 6.3 days, SD 4.7)Citation28.
Furthermore, a Probabilistic Sensitivity Analysis (PSA) was undertaken to assess the effect of parameter uncertainty on model outcomes. The patient-level simulation was repeated 1,000 times, with input parameters randomly drawn from their respective distributions every time. In the absence of reliable data regarding uncertainty, we assumed a standard deviation of 10% of their mean value.
A Deterministic Sensitivity Analysis (DSA) was also performed to test the effect of parameter variations and identify key determinants of results. Input parameters were individually varied by ±20% of their base-case values.
The parameters for the base case and sensitivity analyses are presented in Table S6.
Results
A total of 473 patients were enrolled and analyzed in this study. The median age was 76 years (Q1–Q3: 61–84 years), and 257 (54.3%) patients were male. Respiratory (45.5%) and urogenital (27.5%) infections were the most common infection source. The median value of MR-proADM was 1.31 nmol/L, the median National Early Warning Score (NEWS) was 5 points, and 405 (85.6%) patients were hospitalized. Baseline characteristics are summarized in .
Table 2. Baseline characteristics (PLD).
Univariate logistic regression found that MR-proADM had the strongest association in predicting hospitalization (Table S3). In multivariate analysis, the baseline risk of hospitalization was 78.2% (95% CI: 60.6–89.9%), with the association for MR-proADM maintained (OR [95% CI]: 2.19 [1.36–3.67]). Age and NEWS were also found to be predictors of hospital admission ().
Figure 2. Logistic regression results—hospitalization. Abbreviations. CI, confidence interval; MR-proADM, mid-regional proadrenomedullin; OR, odds ratio; NEWS. national early warning score.
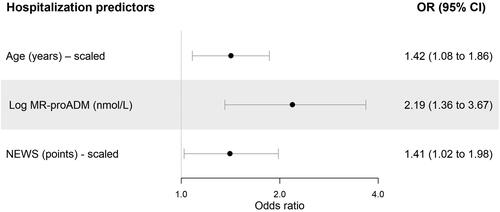
shows the base-case results for Spain obtained from the simulation run on 10,000 patients. MR-GT is associated with an overall reduction in hospital admission rate relative to HT (22.6 percentage points; 40.9% MR-GT vs. 63.5% HT), which is barely offset by the higher number of 14 days readmission (6.1 vs. 2.8%). As a consequence, an MR-proADM decision algorithm is expected to reduce the expense by roughly 30% of the total, with a mean cost saving per patient of €626, €1,484, €1,154, and £1,113 in Spain, Italy, Germany, and the UK, respectively (). Thus, an MR-GT results as more effective and less costly than the standard HT in all evaluated settings.
Figure 3. Economic results in Spain, Italy, Germany, and the UK (per patient). Abbreviations. MR-GT, MR-proADM-guided triage; HT, hospital triage; UK, United Kingdom.
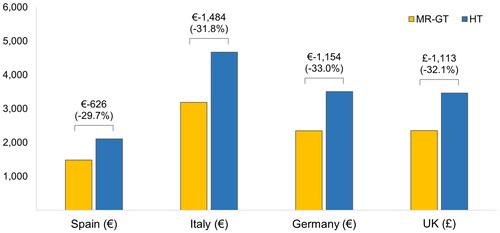
Table 3. Model results—Spanish setting.
The hospitalization rate obtained from the model is well aligned with the published data from which the input parameters were derived, both in terms of mean values and confidence intervals (Figure S3). The results of the alternative scenario analysis carried out on the Spanish cost data are presented in Table S7. Overall, the validation results indicated that our model produced outputs that were consistent both with the existing literature and the outcomes of scenario analysis.
Model robustness was confirmed by PSA results. As depicted in (for Spain), more than 97% of simulation points fell in the south-east quadrant around the base case, indicating the reliability of both clinical and economic estimates. The cost-effectiveness plane also shows a characteristic of this model: cost and hospitalization savings are highly correlated, which is predictable, as the reduction in hospital admission is the main driver of cost savings. The DSA reveals that the results are mostly affected by MR-proADM cut-off (0.87 nmol/L), MR-proADM level, and age (). Costs are also sensitive to changes in the hospital cost per day. Nonetheless, for all the variations tested, the main conclusions are consistent with the base case, confirming the clinical and economic superiority of the MR-GT strategy. Sensitivity analysis results for Italy, Germany, and the UK are presented in Figures S4 and S5.
Discussion
In mid-to-low risk infection patients, proper severity assessment during the diagnosis phase is critical in guiding optimal treatment decision-making in the ED, to cope with ED crowding, and to reduce the resource consumption and the economic burden due to unnecessary hospitalizations. Previous studies showed that MR-proADM is a precise prognostic biomarker to assess disease severity and safely reduce unnecessary hospital admissionsCitation8,Citation15.
The present study identifies predictors of hospitalization in patients presenting to the ED with suspected infection and subsequently evaluates the clinical and economic benefits of the entrenchment of MR-proADM testing into an early infection management protocol in the ED. Age, MR-proADM, and NEWS resulted as risk factors for hospitalization, as consistent with previous findingsCitation8,Citation15. The analysis also shows that in a mid-to-low severity cohort of patients with suspected infection MR-proADM guidance in the ED has the potential to significantly reduce unneeded hospital admissions, thus leading to cost savings in Spain, Italy, Germany, and the UK. On average, 226 hospitalizations per 1,000 patients presenting to the ED could be avoided with MR-GT, saving roughly 30% of total hospital expenditures.
The simulated cohort was built using Spanish PLD and subsequently adjusted to reflect the characteristics of low-risk infection patients enrolled in the IDEAL pilot trialCitation28. The selected target population is the most representative for the use of MR-proADM as a predictor of hospitalization in the ED. The role of the biomarker is indeed of particular importance in patients with few pathophysiological symptoms, where there are the highest uncertainties regarding the hospitalization decisionCitation15. In addition, input data re-calibration allowed for internal model validation. In terms of the hospitalization rate, model predictions compare well with the published data from which the input parameters were derivedCitation28. Nonetheless, if a high-severity cohort had been considered, MR-GT would still have been the most advantageous strategy, with a mean cost saving of roughly €150 per patient due to a reduction in hospital admission of 4.6%.
In this study, hospitalization costs were determined by the length of the hospital stay and the hospital cost per day, allowing the model to be generalized to more EU countries. Nevertheless, an alternative scenario was implemented for the Spanish setting, where patient-specific costs were estimated from regression models performed on the total cost available in the PLD. In this hypothesis, the simulated costs (and related cost savings in percentage) were fairly similar to the base-case results: €1,298 (vs. €1,478) for MR-GT and €1,755 (vs. €2,104) for HT, with a percentage saving of 26% (vs. 30%).
The cost of the MR-proADM test was omitted in this model for several reasons. First, the price could not be determined precisely as it would have to include the combined costs of the reagent, equipment usage, and service components. Lastly, the reagent cost may vary between settings. Even though the cost of the test was not included in the analysis, it will not exceed the projected savings, thus confirming the MR-GT cost-effectiveness.
This model presents a conservative estimate of the cost savings attributable to an MR-proADM-based decision algorithm. In the MR-GT arm, the avoided costs of not performing HT-related diagnostics exams were neglected. In addition, the MR-GT readmission rate was assumed to be slightly higher than HT as reported by Del Castillo et al. despite no significant differences between the two arms in the IDEAL pilot trialCitation28. Finally, the potential MR-GT impact on LOS was not considered in the present model, where savings are determined solely by the reduction in hospitalization. However, MR-proADM has been shown to identify patients with a high risk of further disease progression more accurately than more commonly used biomarkersCitation8,Citation15, reducing the risk of delayed treatment and prolonged hospitalization. Therefore, it can be assumed that the economic benefits of MR-GT are even higher than shown here. Based on these findings, future interventional studies are needed to further explore the economic impact of MR-GT in the ED, as well as the related changes in the triage pathway. To the best of our knowledge, this is the first health economic analysis of the incorporation of MR-proADM in ED decision-making.
A few limitations should be highlighted. The statistical analyses were not performed on the same population simulated in the model; thus, the identified predictors of hospitalization may differ when considering a cohort of lower severity. Moreover, in the absence of additional information, outpatient and hospital costs were supposed to be independent of the treatment arm. As such, costs estimated for the HT were also applied in the MR-GT arm. Therefore, further real-world analyses where an MR-GT is routinely used should confirm the results of the present study (including the test cost and related changes in the triage pathway). Finally, clinical input parameters were assumed to be the same for all countries in scope, not accounting for regional variability among clinical practices. Consequently, sensitivity analyses were undertaken to confirm the clinical and economic superiority of MR-GT in different national healthcare systems.
Conclusions
MR-proADM is found to be a strong predictor of hospitalization. By reducing the number of unneeded hospital admissions, an MR-proADM decision algorithm provides cost savings in Spain, Italy, Germany, and the UK.
Transparency
Declaration of funding
This study was funded by Thermo Fisher Scientific (Germany).
Declaration of financial/other relationships
TS and SK are employees of BRAHMS GmbH. MPi, HH, and FM are employed by DiaSorin SpA. LP is the co-owner and an employee of AdRes, which has received project funding from Thermo Fisher Scientific. CP and MPo are employees of Adres. JGC has received consulting honoraria from Thermo Fisher Scientific.
Author contributions
MPi and SK secured the funding. LP and MPo conceived the study. MPi, TS, and SK collaborated on data acquisition. CP performed the statistical analysis and developed the model. CP, HH, and FM reviewed the literature and drafted the manuscript. JDC, LP, MPi, MPo, and TS participated in the design of the study and critically revised the manuscript. All authors read and approved the final manuscript.
Reviewer disclosures
Peer reviewers on this manuscript have received an honorarium from JME for their review work but have no other relevant financial relationships to disclose.
Ethical approval
The study was conducted according to the guidelines of the Declaration of Helsinki (1964) and the study protocol was approved by the ethics board of each hospital where necessary. Informed consent to participate in the study was obtained for all patients enrolled in the study.
Consent for publication
No individual participant data is reported that would require consent to publish from the participant (or legal parent or guardian for children).
Supplemental Material
Download MS Word (1.4 MB)Acknowledgements
The authors are grateful to the ED staff of Hospital Clínico San Carlos (Madrid, Spain) and especially to Carlota Clemente-Callejo, co-investigator of MR-proADM studies in this hospital. The authors also wish to thank Sonia Allibardi for her contribution to this paper.
Data availability statement
The data that support the findings of this study are available from the corresponding author, CP, upon reasonable request.
References
- Kutz A, Florin J, Hausfater P, et al. Predictors for delayed emergency department care in medical patients with acute infections – an international prospective observational study. PLOS One. 2016;11(5):e0155363. doi: 10.1371/journal.pone.0155363.
- Chmiel C, Wang M, Sidler P, et al. Implementation of a hospital-integrated general practice–a successful way to reduce the burden of inappropriate emergency-department use. Swiss Med Wkly. 2016;146:w14284. doi: 10.4414/smw.2016.14284.
- Murtas R, Tunesi S, Andreano A, et al. Time-series cohort study to forecast emergency department visits in the city of Milan and predict high demand: a 2-day warning system. BMJ Open. 2022;12(4):e056017. doi: 10.1136/bmjopen-2021-056017.
- Jayaprakash N, O'Sullivan R, Bey T, et al. Crowding and delivery of healthcare in emergency departments: the European perspective. West J Emerg Med. 2009;10(4):233–239.
- Di Somma S, Paladino L, Vaughan L, et al. Overcrowding in emergency department: an international issue. Intern Emerg Med. 2015;10(2):171–175. doi: 10.1007/s11739-014-1154-8.
- Mohiuddin S, Busby J, Savović J, et al. Patient flow within UK emergency departments: a systematic review of the use of computer simulation modelling methods. BMJ Open. 2017;7(5):e015007. doi: 10.1136/bmjopen-2016-015007.
- Savioli G, Ceresa IF, Gri N, et al. Emergency department overcrowding: understanding the factors to find corresponding solutions. J Pers Med. 2022;12(2):279.
- Gonzalez Del Castillo J, Wilson DC, Clemente-Callejo C, et al. Biomarkers and clinical scores to identify patient populations at risk of delayed antibiotic administration or intensive care admission. Crit Care. 2019;23(1):335. doi: 10.1186/s13054-019-2613-4.
- Kenny JF, Chang BC, Hemmert KC. Factors affecting emergency department crowding. Emerg Med Clin North Am. 2020;38(3):573–587. doi: 10.1016/j.emc.2020.04.001.
- Krochmal P, Riley TA. Increased health care costs associated with ED overcrowding. Am J Emerg Med. 1994;12(3):265–266. doi: 10.1016/0735-6757(94)90135-x.
- Tatar D, Senol G, Anar C, et al. Markers of lower respiratory tract infections in emergency departments. Multidiscip Respir Med. 2013;8(1):20. doi: 10.1186/2049-6958-8-20.
- Shallcross LJ, Rockenschaub P, McNulty D, et al. Diagnostic uncertainty and urinary tract infection in the emergency department: a cohort study from a UK hospital. BMC Emerg Med. 2020;20(1):40. doi: 10.1186/s12873-020-00333-y.
- Sartelli M, Chichom-Mefire A, Labricciosa FM, et al. The management of intra-abdominal infections from a global perspective: 2017 WSES guidelines for management of intra-abdominal infections. World J Emerg Surg. 2017;12(1):29. doi: 10.1186/s13017-017-0141-6.
- Kamath RS, Sudhakar D, Gardner JG, et al. Guidelines vs actual management of skin and soft tissue infections in the emergency department. Open Forum Infect Dis. 2018;5(1):ofx188.
- Saeed K, Wilson DC, Bloos F, et al. The early identification of disease progression in patients with suspected infection presenting to the emergency department: a multi-centre derivation and validation study. Crit Care. 2019;23(1):40. doi: 10.1186/s13054-019-2329-5.
- Chen YS, Liao TY, Hsu TC, et al. Temporal trend and survival impact of infection source among patients with sepsis: a nationwide study. Crit Care Resusc. 2020;22(2):126–132. doi: 10.51893/2020.2.oa2.
- Fleischmann-Struzek C, Mikolajetz A, Schwarzkopf D, et al. Challenges in assessing the burden of sepsis and understanding the inequalities of sepsis outcomes between national health systems: secular trends in sepsis and infection incidence and mortality in Germany. Intensive Care Med. 2018;44(11):1826–1835. doi: 10.1007/s00134-018-5377-4.
- Paoli CJ, Reynolds MA, Sinha M, et al. Epidemiology and costs of sepsis in the United States–an analysis based on timing of diagnosis and severity level. Crit Care Med. 2018;46(12):1889–1897. doi: 10.1097/CCM.0000000000003342.
- Singer M, Deutschman CS, Seymour CW, et al. The third international consensus definitions for sepsis and septic shock (sepsis-3). JAMA. 2016;315(8):801–810. doi: 10.1001/jama.2016.0287.
- Macdonald SP, Williams JM, Shetty A, et al. Review article: sepsis in the emergency department – part 1: definitions and outcomes. Emerg Med Australas. 2017;29(6):619–625. doi: 10.1111/1742-6723.12886.
- Yébenes JC. Early detection of sepsis: to adapt or to die. Med Clin. 2017;149(5):209–210. doi: 10.1016/j.medcle.2017.07.025.
- Bermejo-Martin JF, Martín-Fernandez M, Almansa R. Pre-sepsis: a necessary concept to complete the SEPSIS-3 picture? J Crit Care. 2018;44:148. doi: 10.1016/j.jcrc.2017.10.041.
- Kumar A, Roberts D, Wood KE, et al. Duration of hypotension before initiation of effective antimicrobial therapy is the critical determinant of survival in human septic shock. Crit Care Med. 2006;34(6):1589–1596. doi: 10.1097/01.CCM.0000217961.75225.E9.
- Leisman DE, Goldman C, Doerfler ME, et al. Patterns and outcomes associated with timeliness of initial crystalloid resuscitation in a prospective sepsis and septic shock cohort. Crit Care Med. 2017;45(10):1596–1606. doi: 10.1097/CCM.0000000000002574.
- Chiarella F, González-Del Castillo J, Candel FJ, et al. Key issues in the infected patient care in the emergency department. Rev Esp Quimioter. 2016;29(6):318–327.
- Francis NA, Cals JW, Butler CC, et al. Severity assessment for lower respiratory tract infections: potential use and validity of the CRB-65 in primary care. Prim Care Respir J. 2012;21(1):65–70. doi: 10.4104/pcrj.2011.00083.
- Zilberberg MD, Nathanson BH, Sulham K, et al. Descriptive epidemiology and outcomes of emergency department visits with complicated urinary tract infections in the United States, 2016–2018. J Am Coll Emerg Physicians Open. 2022;3(2):e12694. doi: 10.1002/emp2.12694.
- Gonzalez del Castillo J, Clemente-Callejo C, Llopis F, et al. Midregional proadrenomedullin safely reduces hospitalization in a low severity cohort with infections in the ED: a randomized controlled multi-centre interventional pilot study. Eur J Intern Med. 2021;88:104–113. doi: 10.1016/j.ejim.2021.03.041.
- Elke G, Bloos F, Wilson DC, et al. The use of mid-regional proadrenomedullin to identify disease severity and treatment response to sepsis – a secondary analysis of a large randomised controlled trial. Crit Care. 2018;22(1):79. doi: 10.1186/s13054-018-2001-5.
- Montrucchio G, Sales G, Rumbolo F, et al. Effectiveness of mid-regional pro-adrenomedullin (MR-proADM) as prognostic marker in COVID-19 critically ill patients: an observational prospective study. PLOS One. 2021;16(2):e0246771. doi: 10.1371/journal.pone.0246771.
- Schmidt T. Coping with copulas. Copulas – from theory to application in finance; 2007.
- Orden de 17/11/2014, de la Consejería de Sanidad Y Asuntos Sociales. Por la Que se Establecen Los Precios Públicos de la Asistencia Sanitaria Y de los Servicios Prestados en la Red de Centros Sanitarios Dependientes Del Servicio de Salud de Castilla-La Mancha [cited 2023 Feb 2]. Available from: https://noticias.juridicas.com/base_datos/CCAA/539877-orden-sanidad-y-asuntos-sociales-17-nov-2014-ca-castilla-la-mancha-precios.html
- Potluri R, Ranjan S, Bhandari H, et al. Healthcare cost comparison analysis of nivolumab in combination with ipilimumab versus nivolumab monotherapy and ipilimumab monotherapy in advanced melanoma. Exp Hematol Oncol. 2019;8:14. doi: 10.1186/s40164-019-0138-9.
- OBI Regional Tariffs (Campania ER, FVG, Lazio, Liguria, Bolzano, Veneto) weighted for resident population (ISTAT 2020); 2020 [cited 2023 Feb 2]. Available from: https://www.salute.gov.it/imgs/C_17_pubblicazioni_3142_allegato.pdf
- National Cost Collection for the NHS [cited 2023 Feb 4]. Available from: https://www.england.nhs.uk/costing-in-the-nhs/national-cost-collection/
- Mayordomo JI, López A, Viñolas N, et al. Retrospective cost analysis of management of febrile neutropenia in cancer patients in Spain. Curr Med Res Opin. 2009;25(10):2533–2542. doi: 10.1185/03007990903209563.
- Kim PJ, Lookess S, Bongards C, et al. Economic model to estimate cost of negative pressure wound therapy with instillation vs control therapies for hospitalised patients in the United States, Germany, and United Kingdom. Int Wound J. 2022;19(4):888–894. doi: 10.1111/iwj.13689.
- Guest JF, Keating T, Gould D, et al. Modelling the annual NHS costs and outcomes attributable to healthcare-associated infections in England. BMJ Open. 2020;10(1):e033367. doi: 10.1136/bmjopen-2019-033367.
- AGENAS – Agenzia Nazionale per i Servizi Sanitari [cited 2023 Feb 3]. Available from: https://www.agenas.gov.it/
- Eurostat – Harmonised Index of Consumer Prices (HICP) [cited 2023 Feb 3]. Available from: https://ec.europa.eu/eurostat/web/hicp
- ISPOR – The Professional Society for Health Economics and Outcomes Research [cited 2023 May 29]. Available from: https://www.ispor.org/heor-resources/good-practices/article/model-transparency-and-validation