ABSTRACT
Introduction
Depression has been associated with impairments in different cognitive domains in younger adults, including prospective memory (PM; the ability to plan and execute intended actions in the future). However, it is still not well documented nor understood whether depression is also associated with impaired PM in older adults. The current study aimed to examine the association between depressive symptoms and PM in young-old and old-old adults, and to understand the potential influence of underlying factors, such as age, education, and metamemory representations (one’s belief about their memory abilities).
Method
Data of 394 older adults from the Vivre-Leben-Vivere study were included in the analyses (Mage = 80.10 years, SD = 6.09; range = 70–98 years).
Results
Bayesian ANCOVA revealed a 3-way interaction between depressive symptoms, age, and metamemory representations, indicating that the association between depressive symptoms and PM performance depended on age and metamemory representations. In the lower depressive symptoms group, old-old adults with higher metamemory representations performed as well as young-old adults independently of their metamemory representations. However, in the higher depressive symptoms group, old-old adults with higher metamemory representations performed more poorly than young-old adults with higher metamemory representations.
Conclusion
This study indicates that metamemory representations may buffer the negative effect of age on PM performance only in old-old individuals with low depressive symptoms. Importantly, this result provides new insight into the mechanisms underlying the association between depressive symptoms and PM performance in older adults as well as into potential interventions.
Introduction
The World Health Organization (Citation2017, December) estimates that currently 7% of older adults worldwide live with depression, making it one of the most common psychological disorders in older adulthood. Depression is considered a leading cause for disability as depressive individuals experience multiple impairments in their daily life, such as reduced work-related activity, loneliness, reduced social skills, and somatization leading to reports of physical pain (Enns et al., Citation2018; Fernández-theoduloz et al., Citation2019; Gilmour & Patten, Citation2007; Lim et al., Citation2018; Singh & Misra, Citation2009; Organization, Citation2017, December; Yap et al., Citation2004). Importantly for the present study, depression also influences cognitive abilities such as autobiographic, episodic, and working memory (Dietsche et al., Citation2014; Evans et al., Citation2019; Joormann & Gotlib, Citation2008; Salazar-Villanea et al., Citation2015; Söderlund et al., Citation2014; Wilson & Gregory, Citation2018).
However, much less is known about the association between depressive symptoms and prospective memory (PM), that is, the ability to plan and remember delayed intentions (McDaniel & Einstein, Citation2000). PM can be evaluated through two types of tasks: 1) event-based tasks, which consist of executing the intention when a specific external cue is encountered (i.e., remembering to buy bread when passing by the bakery), and 2) time-based tasks, which consist of executing the intention after a certain amount of time has elapsed (i.e., remembering to take your medication 30 min after dinner). In terms of cognitive processes, PM is divided in two components: 1) a retrospective component, which refers to remembering the content of the intention to execute (i.e., taking a specific type of medication and related to episodic memory), and 2) a prospective component, which refers to remembering appropriate moment to execute the intention (i.e., 30 min after dinner, related to monitoring and executive functions). PM plays a crucial role in everyday autonomy as it predicts maintaining independence and higher quality of life: remembering to meet with friends, cooking one’s own meal, paying the bills on time but also taking medications are all examples of typical PM tasks that stress its importance for health, social functioning, and autonomy (Hering et al., Citation2018; Kliegel et al., Citation2016; Laera et al., Citation2021; Woods et al., Citation2012).
Thus, the scarce literature on the relationship between depressive symptoms and PM in older adults can be considered as a particularly important knowledge gap. In younger adults, a meta-analysis demonstrated that individuals with higher levels of depressive symptoms were impaired on PM tasks (Zhou et al., Citation2017). In older adults, however, only two studiesFootnote1 have so far investigated the relationship between PM and depressive symptoms, leading to inconclusive results (Albiński et al., Citation2012; Livner et al., Citation2008). Assessing 332 older adults, Livner et al. (Citation2008) demonstrated that depressive symptoms were associated with impaired performance on the retrospective component of an event-based PM task, but there was no association between depressive symptoms and the prospective component of this task. Interestingly, the second study revealed no significant link between depression and an event-based PM task but a positive association between depression and a time-based PM task in a sample of 60 older adults (Albiński et al., Citation2012). Thus, the available literature is highly inconsistent, and researchers have pointed out the need to further examine the relationship between depression and PM in older adults. As for possible factors underlying these inconsistencies across previous studies, considering different levels of depressive symptoms, education, metamemory representations, and age might be of interest. Indeed, previous research on these factors in relation to depressive symptoms demonstrated interesting results in other cognitive domains, including retrospective memory.
Depressive symptoms
Previous studies have suggested that different levels of depressive symptoms may differentially relate to cognitive outcomes. Specifically, an inverted U-shape relation was revealed in individuals with depressive symptoms for certain tasks such as contingency problems (Alloy & Abramson, Citation1979; Andrews & Thomson, Citation2009; ; Harkness et al., Citation2005; L. L. Lee et al., Citation2005; Marsh & Weary, Citation1994). Indeed, in these studies, individuals with relatively high but still subclinical levels of depressive symptoms performed better on cognitive tasks than individuals with lower depressive symptoms. Yet, interestingly, they also performed better than those with very high, clinical levels of depressive symptoms. This suggested that the performance of individuals with subclinical levels of depressive symptoms might benefit from the analytical rumination associated with depressive affect. The analytical rumination hypothesis consists of two components activated in individuals with depressed affect: (a) the causal analysis, which aims to understand the origins of the depression-related problems, and (b) the problem-solving analysis, which focuses on the manner to solve these problems (Andrews & Thomson, Citation2009; Bartoskova et al., Citation2018; Sevcikova et al., Citation2020). Other previous studies investigating rumination indicated that rumination is composed of two subcomponents: the brooding and reflection components (Krys, Citation2020; Schoofs et al., Citation2010; Treynor et al., Citation2003). Brooding is to compare one’s own present situation to unachieved goals and standards and is considered maladaptive, while reflection is defined as engaging into cognitive problem-solving by allocating attention and effort to a task and is, therefore, an adaptive component (Krys, Citation2020; Schoofs et al., Citation2010; Treynor et al., Citation2003). Thus, individuals suffering from subclinical depressive symptoms may rely on analytical rumination and reflection, enabling them to outperform individuals with lower depressive symptoms and clinically depressed individuals. This could explain why in Albiński et al.’s study (Albiński et al., Citation2012), participants with higher levels of depressive symptoms performed better on a time-based PM than participants with lower levels of depressive symptoms. Discriminating between individuals with and without depressive symptoms seems very relevant to understand how they can be associated with episodic memory and PM in particular. However, in addition to rumination, educational attainment could be a factor helping depressive individuals to perform well on cognitive tasks as it is protective against cognitive impairment in this population (James et al., Citation2021).
Education
In their meta-analytic review, James et al. (Citation2021) demonstrated that individuals with depressive symptoms perform as well as controls on retrospective memory tasks when they have higher education compared to those with lower education (e.g., McLaren et al., Citation2015; Murphy & O’Leary, Citation2009). Regarding PM performance, Huppert and Beardsall (Citation1993) and Simard et al. (Citation2019) have shown that lower education impaired PM performance in older adults compared to those with higher education. Surprisingly, both available studies on the relationship between depressive symptoms and PM functioning in older adults did not investigate the association between education and PM performance (Albiński et al., Citation2012; Livner et al., Citation2008). However, when inspecting the study samples, they systematically differed in participants’ mean education (i.e., 12 years versus 9.38 years; Albiński et al., Citation2012; Livner et al., Citation2008). To explain the relationship between educational attainment and performance of episodic memory in older adults, certain authors postulated an influence of cognitive reserve (i.e., cognitive stimulation accumulated across the lifespan that preserves cognitive functioning in older adults) and metamemory (Guerrero-Sastoque et al., Citation2021b; Stern, Citation2009, Citation2012; Szajer & Murphy, Citation2013).
Metamemory representations
Metamemory refers to the knowledge that individuals possess about their own memory abilities (Flavell, Citation1979; Nelson & Narens, Citation1990). Having higher education may improve metamemory accuracy, meaning that individuals’ representations of their memory abilities are more accurate than participants with less education. Accuracy of one’s representations is crucial for memory performance as it is related to the implementation of effective strategies (Nelson & Narens, Citation1990; Szajer & Murphy, Citation2013). A discrepancy between subjective performance and actual performance, such as overconfidence, may prevent individuals from implementing strategies to improve their memory performance (e.g., putting heart medication on the dinner table to remember to take it while eating). This is of particular importance 1) for PM, as PM failures represent half of the memory failures that occur in daily-life, suggesting that strategies might be needed to improve PM (Crovitz & Daniel, Citation1984; Haas et al., Citation2020); 2) for older adults, as their memory is declining with age, possibly creating a gap between their representations and actual performance, and impairing their autonomy. Previous studies focusing on older age, metamemory, and PM demonstrated that older adults are overconfident in their PM abilities, which may lead to less implementation of strategies and a higher rate of PM failures (Cauvin et al., Citation2019). Metamemory representations may also be influenced by depressive symptoms as they are associated with dysfunctional representations about the world, the future, and oneself (Beck, Citation1976; Wells, Citation2011, Citation2008). Indeed, depressive symptoms have been associated with more memory complaints, with lower representations of performance and lower accuracy on retrospective memory tasks (Balash et al., Citation2013; Cipolli et al., Citation1996; Metternich et al., Citation2009; Zandi, Citation2004). Moreover, in older adults, the influence of depression on retrospective memory might be mediated by the relationship between metamemory and retrospective memory. As for PM specific effects, to our knowledge, only one study investigated the association between depressive symptoms and metamemory representations with PM performance in younger adults (Huber et al., Citation2022). The authors indicated that the depressed and healthy groups did not differ in their PM performance nor in the correlation between PM metamemory representations and actual performance. However, they reported that depressed younger adults had more PM complaints than the healthy group. This relationship between depressive symptoms, metamemory, and PM has never been investigated in older adults. Yet, this is of great importance to understand whether and how metamemory representations are associated with depressive symptoms and PM performance in older adults too. Considering that with increasing age older adults are overconfident in their PM abilities, but that depressive symptoms elicit negative representations of one’s own abilities, depressive symptoms, and age may have opposite effects on metamemory representations. Then, categorically discriminating between higher and lower depressive symptoms, higher and lower metamemory representations, but also young-old and old-old adults may be interesting to explore and disentangle these effects.
Age
Age might play a role in metamemory representations as mentioned previously, but also differentially relate to depressive symptoms and PM. Previous studies have demonstrated that several factors may influence and characterize depressive symptoms differently depending on age. For example, it has been shown that depressive symptoms are particularly influenced by family relationships and apathy in old-old adults (S.-W. Mehta et al., Citation2008; S.-W. Lee et al., Citation2020). As PM performance is not spared by age-related decline, several authors distinguished between young-old and old-old adults, demonstrating that young-old adults often perform better than old-old participants (for a meta-analysis on age-related decline see, Henry et al., Citation2004; see also, Kvavilashvili et al., Citation2009; Zimmermann & Meier, Citation2006; Zuber & Kliegel, Citation2020). Moreover, as previously mentioned, a recent meta-analysis of James et al. (Citation2021) indicate that depression is related to deficits in episodic memory, which are larger in older age. Interestingly, the available two studies investigating depressive symptoms and PM have sampled from two different decades in the lifespan (68.3 years old in Albiński et al., Citation2012 versus 84.65 years old in Livner et al., Citation2008). Despite the fact that most studies consider older age as a homogenous group, previous studies draw our attention toward possible differences between young-old and old-old adults, which deserves further investigation.
Gender
Finally, gender might play an important role in the relationship between depressive symptoms and PM in old age as women experience more depression in old age (Girgus et al., Citation2017; Newman & Brach, Citation2001). In addition, until 1970s, there was an important gender gap in education, meaning that older women born in the 1940s may not have benefitted from the same education as their male counterparts (Sundstrom, Citation2004). Considering these associations, it seems relevant to consider gender in the relationship between depressive symptoms, education, age, and metamemory representations in PM performance.
The present study
Taken together, considering all of those potential factors of influence, we aimed to examine the role of 1) lower and higher depressive symptoms, 2) lower and higher education, 3) lower and higher metamemory representations, 4) age in a young-old versus old-old groups, and finally 5) how these effects may interact together on PM performance in a large-scale population-based cohort of older adults aged 70 and older, while controlling for gender. Based on the analytical rumination hypothesis and results of previous studies presented in Andrews and Thomson’s (Citation2009) paper, we expected higher PM performance for participants presenting higher depressive symptoms. Considering previous findings on the link between education and cognition, we expect that participants with higher educational levels will perform better on the PM task than participants with lower educational levels. We expect that participants with higher metamemory representations will perform better on the PM task than participants with lower metamemory representations. Finally, as supported by previous findings, we expect that young and old participants will perform better than old-old participants on the PM task, as they may have more cognitive resources available. Considering that this is the first study to investigate depressive symptoms, education, metamemory, and age, we did not have specific hypothesis regarding the effects of all these variables together, especially because opposite effects may emerge.
Method
Data stem from the second wave of the longitudinal study “Vivre – Leben – Vivere” (VLV), which investigated healthy aging across the lifespan in Switzerland (for more details on the procedure, see, Ihle et al., Citation2018; Mella et al., Citation2018; Vallet et al., Citation2020). Participants were assessed in 2017 in a face-to-face computer-assisted personal interview (Ihle et al., Citation2018). Participants were included in the present analyses if their global cognitive functioning assessed by the Mini Mental State Examination (MMSE; Folstein et al., Citation1975) was equal and above 24, and if they had no missing data on any variables of interest (depressive symptoms, MSE, education, age, PM, and MMSE). Then, to compare PM performance depending on depressive symptoms levels, we selected participants with the highest and lowest levels based on the first versus fourth quartiles of depressive symptoms. The final sample consisted of 394 older adults (47.6% females), with a mean age of 80.10 years (SD = 6.09; range = 70–98 years). Their MMSE mean score was 28.50 (SD = 1.62; range = 24–30), and their educational mean level was 3.93 (SD = 1.38; range = 1–6), which indicates that on average participants’ educational level was just below “high-school graduation.” All participants gave their written informed consent for inclusion before they participated in the study. The study was conducted in accordance with the Declaration of Helsinki (World Health Organization, Citation2017, December), and the ethics commission of the Faculty of Psychology and Social Sciences of the University of Geneva had approved the protocol (project identification code: CE_FPSE_05.04.2017).
Measures
Depressive symptoms
Participants’ depressive symptoms were assessed with the Brief Self-Assessing Depression Scale (SADS; Wang et al., Citation1975). The SADS was administered to participants in their native tongue, which was either French or German. They indicated on a 4 points-Likert scale ranging from 0 (never) to 3 (always) how frequently in their present life situation they experienced sadness, good appetite (inverse-coded), crying, sleep issues, self-confidence (inverse-coded), interests in doing things (inverse-coded), irritability, being hopeful for the future, being tired-coded, and being tired. The SADS does not provide cutoff values to identify mild, moderate, and severe depressive symptoms. The higher the score, the more participants experienced depressive symptoms. Participants’ scores could range from 0 to 30. Reliability of the SADS in our sample was good, as indicated by a Cronbach’s alpha of 0.84 (Gliem & Gliem, Citation2003; Pallant, Citation2020).
Metamemory representations
Metamemory representations were assessed with the validated French Version of the Memory Self-Efficacy Questionnaire (MSE; Beaudoin et al., Citation2008; Desrichard & Köpetz, Citation2005) in which participants evaluated their ability to execute various memory tasks with different levels of difficulty. The questionnaire describes six memory tasks: three laboratory-type memory tasks like recalling a list of numbers and three everyday-type memory tasks like remembering a list of grocery shopping items. Participants indicated between five levels of difficulty the one they think they will successfully complete (e.g., “if someone showed you the pictures of 16 familiar objects (e.g., lamp, umbrella, etc.), would you be able to look at them once and recall the names … of all 16 objects, of 12 of the 16 objects, of eight of the 16 objects, of four of the 16 objects, of two of the 16 objects?”). Answers were recoded so that 0 indicated that participants thought they would recall the minimum number of items, while 4 indicated that participants thought they would recall the maximum. Participants’ score could range from 0 to 30 points. The higher the score, the better participants thought they would perform on different memory tasks, and therefore had higher representations of their memory abilities.
Education
Participants were asked to indicate the highest educational level they reached between “primary school” (coded “1”), “inferior secondary school” (coded “2”), “apprenticeship graduation” (coded “3”), “superior secondary school” (coded “4”), “superior vocational college” (coded “5”), and “university degree” (coded “6”).
Prospective memory
PM performance was assessed using four event-based single-trial PM tasks embedded into the interview session (for other studies using the same PM task setting and reliability analyses of this approach see, Cuttler & Graf, Citation2007; Dobbs & Rule, Citation1987; Ihle et al., Citation2019; Zeintl et al., Citation2007). Specifically, participants were instructed 1) to remember to say “red pencil” when the interviewer talked about a red pencil later during the interview (i.e., red-pencil task), 2) to remember to knock twice on the table when the interviewer talked about physical activities (i.e., knock-on-table task), 3) to remember to tell the interviewer their year of birth when talking about activities in the course of the participant’s life (i.e., year-of-birth task), and 4) to remember to remind the interviewer to switch on the mobile phone at the end of the interview (i.e., mobile phone task). Each task was scored 1 point when successfully remembered and 0 when the participants did not remember to execute the task. Participants’ total scores ranged from 0 to 4.
Analytical approach
Based on the analytical rumination hypothesis, we treated depressive symptoms categorically in our study. To compare PM performance depending on depressive symptoms levels, we selected participants with the highest and lowest levels based on the first versus fourth quartiles of depressive symptoms. We created age groups based on the recommendations of the WHO and the American Geriatric society who consider that individuals aged 80 years and older represent the “old-old” (Escourrou et al., Citation2020). Thus, participants younger than 80 years old were included in the “young-old” age group (M = 75.48, SD = 2.54), while participants aged 80 years and older were included in the “old-old” age group (M = 85.93, SD = 3.83). We also created education groups based on categories of lower education (consisting of “primary school,” “inferior secondary school,” “apprenticeship graduation”) and higher education (consisting of “superior secondary school,” “superior vocational college” and “university degree”; Gabriel et al., Citation2015; for another VLV study using these cutoffs see, Ihle et al., Citation2018). We performed a median split based on our participants’ answers to examine metamemory representations (“higher MSE” vs. “lower MSE”; for a similar procedure see, Iacullo et al., Citation2016).
Considering the ambiguous results provided by the two previous studies on our research topic, we conducted Bayesian analyses as they allow to clearly quantify which of the null or alternative hypothesis is more plausible. Age, education, metamemory representations, and depressive symptoms were entered as factors in the model, while PM was entered as a continuous dependent variable. Then, for the Bayesian ANCOVA, independent variables were depressive symptoms (“higher depressive symptoms” vs. “lower depressive symptoms), metamemory (“higher MSE” vs. “lower MSE”), education (“higher education” vs. “lower education”), age (“old-old” vs. “young-old”), and the dependent variable was PM. We controlled for gender. We investigated the main effects and interactions between dichotomic variables on PM. To further explore significant interactions, we performed Bayesian independent sample t-tests. As this is the first study considering depressive symptoms, education, metamemory, and age, we decided to use default priors and parameters set by JASP (Version 0.16.0.0; JASP Team, 2021). Default priors are recommended when knowledge provided by previous studies is not clear (Van Doorn et al., Citation2019). In our case, only two previous studies investigated the relationship between depressive symptoms and PM. These studies demonstrated results going in opposite directions, encouraging the use of a default prior. Moreover, they did not investigate the combined effects of depressive symptoms, age, education, metamemory representations, and gender. Thus, defining an informative prior based on these previous results might bias our results because the direction is unclear, but also because our model does not match previous studies. Default priors are used in our study, meaning that the r scale fixed effects’ coefficient is 0.5, the r scale random effects’ coefficient is 1, and the r scale covariates’ coefficient is 0.354. We examine which model is the best among the possible models by comparing the Bayes Factors. According to Lee and Wagenmakers (Citation2013), Bayes Factors superior to 100 indicate extreme evidence for H1, 30 to 100 indicate very strong evidence, 10 to 30 indicate strong evidence, from 3 to 10 indicate moderate evidence, from 1 to 3 indicate anecdotal evidence for H1, while 1/3 to 1 indicate anecdotal evidence for H0, 1/3 to 1/10 indicate moderate evidence for H0, 1/10 to 1/30 indicate strong evidence for H0, 1/30 to 1/100 indicate very strong evidence for H0 and Bayes Factors inferior to 1/100 indicate extreme evidence for H0. We assessed significance of posterior means (further called estimates δ) for independent variables using Bayes factors and bounds (95% credible intervals; e.g., Van de Schoot et al., Citation2014). Bayes factors indicate whether the data are in favor of the alternative (H1) or the null (H0) hypotheses, while the credible intervals indicate significance (Lavine & Schervish, Citation1999; Van de Schoot et al., Citation2014). When the credible interval did not contain the value 0 (meaning that both ends of the interval are either larger or smaller than 0), we rejected H0 and concluded that the effect is significant. In order to disentangle interaction effects between our variables of interest, we conducted multiple comparisons using Bayesian t-tests and applied adequate corrections based on the Westfall approach (for a similar approach, see, e.g., De Jong, Citation2019).
Results
Descriptive statistics
shows descriptive statistics describing mean and standard deviation for depressive symptoms, MSE, education, and MMSE according to age groups. This table indicates that depressive symptoms and metamemory representations differed between young-old and old-old adults. We performed Bayesian t-tests on these data to assess possible differences in our sample. Bayesian t-test between young-old (Mage = 75.48) and old-old adults (Mage = 85.93) indicate that they indeed differ in age. The higher depressive symptoms group has significantly higher levels of depressive symptoms, in the young-old age group (BF−0 = 4.56x1092) and in the old-old age group (BF−0 = 2.48x1068). In the young-old participants with lower depressive symptoms, participants in the lower MSE group (M = 16.07) have significantly lower MSE than the higher MSE group (M = 20.99; BF−0 = 2.30x1022). This effect was replicated in the young-old participants with higher depressive symptoms, the lower MSE group (M = 14.52) has significantly lower MSE than the higher MSE group (20.44; BF−0 = 4.13x1014). In the old-old participants with lower depressive symptoms, the lower MSE group (M = 15.05) has significantly lower MSE than the higher MSE group (M = 22.07; BF−0 = 8.37x1014). This effect was also replicated in old-old adults with higher depressive symptoms; indeed, the lower MSE group (M = 14.95) has significantly lower MSE than the higher MSE group ((M = 20.74; BF−0 = 5.41x1013). All Bayes factor provided indicate extreme evidence for significant differences between these groups. describes the percentage of PM success in each group of age and depressive symptoms.
Figure 1. Percentage of Participants per PM Score depending on Groups of Age and Depressive Symptoms.
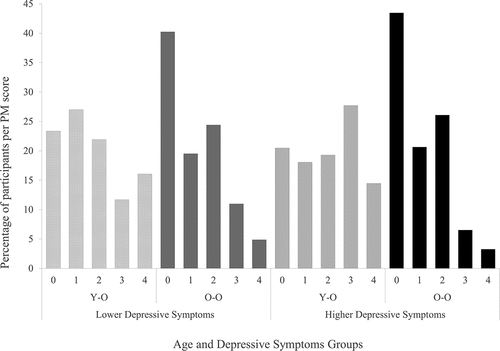
Table 1. Descriptive Statistics and Bayesian T-Tests of Variables of Interest by Age, Depressive Symptoms, and Metamemory Representations Groups
Bayesian ANCOVA
A 2 Age (young-old vs. old-old) x 2 Education (lower education vs. higher education) x 2 Depressive Symptoms (lower depressive symptoms vs. higher depressive symptoms) x 2 MSE (lower MSE vs. higher MSE) x Gender Bayesian ANCOVA was conducted. The Bayesian model comparison revealed extreme evidence that the model with age, education, depressive symptoms, and MSE and a 3-way interaction between MSE, age, and depressive symptoms was the best model compared to the null model (BF10 = 203.89). It means that the data were 203.89 times more likely to occur under this model than under the null model (Supplementary Material Table details estimates, standard deviations, credible intervals for all independent variables, and interactions of the model). This model explained in mean 8% of the variance of the data (R2 = 0.08; 95%CI [0.04; 0.12]). Results indicated the main effects of age and education, meaning that young-old participants (M = 1.80, SD = 1.38) had a better PM performance than old-old participants (M = 1.13, SD = 1.17) and that participants with higher education (M = 1.65, SD = 1.37) performed better than participants with lower education (M = 1.32, SD = 1.27). The 3-way interaction between depressive symptoms, age, and MSE was significant (see, ). Further Bayesian t-tests were performed to explain the direction of this 3-way interaction. First, results indicated that for participants with lower depressive symptoms and lower MSE, the young-old (M = 1.88, SD = 1.33) outperform the old-old individuals on the PM task (M = 0.84, SD = 1.09; BF10 = 15.71, δ = 0.78, 95% CI [0.32, 1.24]). Second, for participants with lower depressive symptoms, young-old adults with higher MSE (M = 1.62; SD = 1.39) perform better on PM than old-old adults with lower MSE individuals (M = 0.84; SD = 1.09; BF10 = 2.34 δ = 0.54, 95% CI [0.17, 0.93]). Third, for participants with higher depressive symptoms and lower MSE, the young-old (M = 1.79; SD = 1.37) performed better than the old-old individuals on PM (M = 1.06; SD = 1.12; BF10 = 1.94, δ = 0.54, 95% CI [0.16, 0.94]). Fourth, for participants with higher depressive symptoms and higher MSE, PM performance was higher in young-old (M = 2.17; SD = 1.36) than in old-old adults (M = 1.04; SD = 1.16; BF10 = 7.98 δ = 0.80, 95% CI [0.30, 1.31]). Fifth, for participants with higher depressive symptoms, young-old adults with higher MSE (M = 2.17; SD = 1.36) performed better than old-old adults with lower MSE (M = 1.06; SD = 1.12; BF10 = 254.62 δ = 0.86, 95% CI [0.45, 1.23]). This effect was not present for the lower depressive symptoms group.
Figure 2. Representation of the 3-way Interaction between Depressive Symptoms, Age, and MSE on PM Performance.
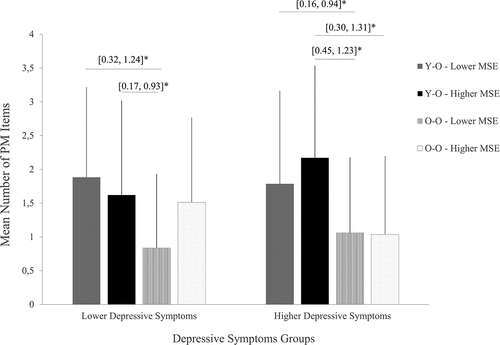
Discussion
The aim of the present study was to examine the role of levels of 1) depressive symptoms, 2) education, 3) metamemory representations, 4) age, and finally 5) how these effects may interact together with PM performance. The present findings reveal that age and metamemory representations are indeed important variables to consider when aiming to understand if and how depressive symptoms may be related to interindividual differences in older adults’ PM performance, while education does not seem to be a key factor in this area. Specifically, results indicate that the association between depressive symptoms and PM performance is (a) limited to individuals with higher metamemory representations and that (b) the direction of this impact depends on age. In the following, we will discuss the respective main effects and interactions in more detail.
Age effects on PM within old age
The fact that young-old adults perform better on PM than old-old adults is consistent with previous literature on aging and cognition in general and on PM and aging in particular (Ballhausen et al., Citation2019; Kvavilashvili et al., Citation2009; Zimmermann & Meier, Citation2006; Zuber & Kliegel, Citation2020). Thus, these results emphasize the fact that discriminating between young-old and old-old adults is an important factor when investigating PM in older adults.
Education effects on PM
Results indicate that participants with higher levels of education perform better than participants with lower levels of education. This confirms the general literature indicating that education is associated with better cognitive and PM performance, which can be explained by the concept of cognitive reserve (Cherry & Lecompte, Citation1999; Huppert & Beardsall, Citation1993; Lövdén et al., Citation2020; Simard et al., Citation2019; Stern, Citation2009, Citation2012). Across the lifespan, individuals accumulate cognitive reserve through professional and leisure activities, but also education, subsequently helping them to perform cognitive tasks, including PM tasks. Yet, importantly for present purposes, education did not interact with the impact of depressive symptoms on older adults’ PM performance. Thus, one has to conclude that education exerts a global effect cognitive performance in old age as suggested by Lövdén et al. (Citation2020).
Depressive symptoms
Contrary to our hypothesis that individuals with higher depressive symptoms would outperform individuals with lower depressive symptoms because of the analytical rumination hypothesis, we did not demonstrate any main effect of depressive symptoms on PM performance in our sample. As explained previously, Andrews and Thomson (Citation2009) postulate the existence of an inverted U-shape curve of cognitive performance in individuals without and with depressive symptoms: individuals with subclinical symptoms would perform better than both individuals with clinical and without symptoms. As the SADS does not provide a clear cutoff for discriminating between mild, moderate, and severe depressive symptoms, we selected participants in the lowest and highest quartiles of the depressive symptoms scores. However, this could have resulted in merging participants suffering from subclinical and clinical depressive symptoms into the same group (i.e., the higher depressive symptoms group). This merging could have led to 1) an averaging of the differences in PM performance within the higher depressive symptoms group, 2) and, consequently, to a reduction of the difference in PM performance between both depressive symptoms’ groups. However, the effect of depressive symptoms emerges in a 3-way interaction with metamemory representations and age, suggesting that the relationship between depressive symptoms and PM performance may be more complex than the main effect.
Interaction effects between depressive symptoms, metamemory representations, and age
As a key result, a 3-way interaction between depressive symptoms, metamemory representations, and age emerged in our sample of participants. Having higher metamemory representations seems to have differential consequences on young-old versus old-old adults depending on their depressive symptoms level. In the lower depressive symptoms group, old-old adults with higher metamemory representations perform as well as young-old adults independently of their metamemory representations. On the contrary, in individuals with higher depressive symptoms and higher metamemory representations, old-old adults perform more poorly than young-old adults. Why might this be?
Previous models considering affect and motivation described that people with low ability need to invest more effort to perform as well as people with high ability. When the task difficulty is subjectively perceived as too challenging, individuals will disengage from the task, meaning they do not mobilize effort to succeed (Brinkmann, Citation2008; Gendolla, Citation2004). Moreover, this threshold of disengagement happens “earlier” for people with low ability than for people with high ability (see the Attributional Theory of Performance and Risk-Taking Model, respectively from Kukla, Citation1972, and Atkinson, Citation1957 cited in Gendolla, Citation2004). These theories and research on affect and motivation also included participants with depressive symptoms. They indicate that the threshold of disengagement supposedly happens “earlier” for individuals experiencing depressive symptoms than for individuals without depressive symptoms (Brinkmann, Citation2008; Brinkmann & Gendolla, Citation2008). In their study, Brinkmann and Gendolla (Citation2008) demonstrated that for a memory task, participants with depressive symptoms were judging the task as more difficult than participants in the same condition but without depressive symptoms. Moreover, the authors concluded that individuals with depressive symptoms can be very engaged (i.e., mobilize effort) in tasks that they consider easy, while they disengage and withhold effort for tasks that they perceive as difficult.
In the light of these theories and previous studies, we can interpret our 3-way interaction results. First, we will focus on the mechanisms that contribute to performance for participants in the lower depressive symptoms group. Young-old participants outperform old-old participants on PM performance when metamemory representations are lower, but not when metamemory representations are higher. Hence, old-old adults might not be as confident as young-old adults in their memory abilities because they perceive the task as too difficult considering their remaining cognitive resources, leading them to disengage from the task. Indeed, with greater age comes greater cost to engage in cognitive tasks which elicit fatigue (Hess, Citation2014). Thus, older adults would wisely select in which tasks they are willing to engage effort and disengage earlier for those requiring too many resources to succeed (Hess, Citation2014). However, for participants with higher metamemory, old-old adults may see themselves as high performers who can achieve the PM task, subsequently mobilizing effort and performing similarly to young-old adults. Thus, having higher metamemory representations seems protective against age-related cognitive decline.
Second, we now focus on the mechanisms that contribute to performance for participants in the higher depressive symptoms group. Old-old adults perform similarly independently of their metamemory representations. In turn, the two old-old groups performed significantly worse than the young-old adults with higher metamemory representations. Hence, when depressive symptoms are higher, the protective effect of higher metamemory representations on PM performance seems to disappear as depressive symptoms might take over and disrupt task engagement, regardless of old-old adults’ metamemory representations. As Brinkmann and Gendolla (Citation2008) included only younger adults in their study, we extend previous findings and thereby provide new insights on older age to the field of affect, metamemory, and cognitive performance.
Interestingly, even though certain old-old adults with higher depressive symptoms have higher metamemory representations, these representations might be an overestimation of their actual abilities, as it was demonstrated in a previous study (Cauvin et al., Citation2019), creating a gap between their representations and abilities. Yet, if old-old adults do not update these representations, they will not be able to implement new – and more effective- strategies to compensate for the negative influence of their depressive symptoms on PM abilities. Importantly, depressive symptoms often elicit worry, which has been related to impairment in cognitive functioning in older adults (De Vito et al., Citation2019). Indeed, it has been demonstrated that worry interferes with processing speed, working-memory, and attention (Eysenck et al., Citation2007; Schoen & Holtzer, Citation2017). It is then also possible that old-old individuals are overwhelmed by worry about their future performance on PM, which distracts their attention from planning PM intentions.
For example, research shows that when older adults are confronted with the memory demands of a PM task, they perform worse compared to when the same task is presented as a non-memory task (e.g., Zuber et al., Citation2019). It would therefore be interesting to further investigate the relationship between depressive symptoms, worry, and metamemory in older adults to offer possible interventions targeting PM. Indeed, a recent meta-analysis indicates that metacognitive interventions work as well as psychological treatment to alleviate depressive symptoms (Philipp et al., Citation2019). Moreover, a study by Pearman et al. (Citation2020) indicates that metacognitive intervention helps reduce PM failures in everyday life in older adults. However, fewer studies have focused on both affective and cognitive outcomes using metacognitive interventions. This is a gap that future studies should consider investigating.
Limitations and perspectives
Noteworthy is that, in this secondary-analysis study, depressive symptoms were assessed by the SADS, which is not a diagnostic scale (yet, it has been used in several studies see, Lalive d’Epinay & Bickel, Citation2003; Perrig-Chiello et al., Citation2016; Vedder et al., Citation2022, and its items are very similar to the Beck Depression Inventory, Beck et al., Citation1996). Even though participants in the lower and higher depressive groups were significantly different, and that the reliability of the SADS reached a Cronbach’s alpha of 0.84 for our sample, we recommend that future studies use more clinical and validated assessment tools, like the Geriatric Depression Scale (GDS; Yesavage et al., Citation1988), to ensure that participants present meaningful levels of depressive symptoms.
Considering that mechanisms of depressive symptoms on PM performance are currently still not well understood, future studies should consider investigating the rumination process and other possible factors. For instance, it would be interesting to use a rumination questionnaire like the Ruminative Responses Scale (RRS; Treynor et al., Citation2003) or the Analytical Rumination Questionnaire (ARQ; Barbic et al., Citation2014) to disentangle the possible effects of depression levels and ruminations on cognitive tasks.
Metamemory representations were measured with the French Version of the Memory Self-Efficacy Questionnaire (Beaudoin et al., Citation2008; Desrichard & Köpetz, Citation2005). This self-report questionnaire relies on participants representations but do not inform on the accuracy of these representations. Also, this questionnaire has not been specifically designed to assess PM representations, but it focuses on retrospective memory. As PM does not only rely on retrospective memory, it would be interesting to conduct future studies using questionnaires about both retrospective memory and PM like the PRMQ (Crawford et al., Citation2003), which provides normative data. Using a questionnaire focusing on both retrospective and prospective memory would also enable the comparison of representations with actual performance. Indeed, understanding whether participants have accurate representations of their memory abilities is very informative as it could explain cognitive impairment and help to implement interventions on representations and the use of strategies.
Future studies should consider examining depressive symptoms, metamemory, and PM in longitudinal designs. Moreover, as a 3-way interaction and the t-tests applied to disentangle its effect are not equal to a moderation test, we suggest that they investigate this relationship using formal moderation tests. This would enable us to better understand how the relationship between age, depressive symptoms and PM depends on metamemory representations, and how they develop together across the lifespan.
Conclusion
Taken together, the present study critically adds to the scarce literature on depressive symptoms and PM in older adults by introducing new important factors to consider, such as age and metamemory representations. These findings are of great importance as they provide new insights into the effects of depressive symptoms on PM in older adults, which are still not well documented nor understood. More specifically, the present findings indicate that the association between depressive symptoms and PM performance depends on age and metamemory representations. Indeed, metamemory representations buffer the negative effect of age on PM performance only in old-old adults with low depressive symptoms. On the contrary, old-old adults with higher metamemory representations suffering from depressive symptoms might need interventions, not only to reduce their depressive symptoms and increase their well-being, but also to help them to adapt their representations and implement new strategies. The aim is to enable these individuals to perform as well as young-old adults on PM, and thus help them to maintain their autonomy as long as possible.
Supplemental Material
Download MS Word (34.5 KB)Supplemental Material
Download MS Word (34.5 KB)Acknowledgments
This work was supported by the Swiss National Centre of Competence in Research LIVES – Overcoming Vulnerability: Life Course Perspectives, which is financed by the Swiss National Science Foundation (grant number: 51NF40-160590). The authors acknowledge further funding from the Swiss National Science Foundation (SNSF; P500PS_210853).
Disclosure statement
No potential conflict of interest was reported by the author(s).
Supplementary material
Supplemental data for this article can be accessed online at https://doi.org/10.1080/13803395.2023.2195618
Additional information
Funding
Notes
1. In a previous study using the same data set as in the present study, the effects of socioeconomic condition in childhood, adulthood and later-life on PM were investigated (controlling for depressive symptoms; for further information, see Künzi et al., 2021).
References
- Albiński, R., Kliegel, M., Sȩdek, G., & Kleszczewska-Albińska, A. (2012). Positive effects of subclinical depression in prospective memory and ongoing tasks in young and old adults. Aging, Neuropsychology, and Cognition, 19(1–2), 35–57.
- Alloy, L. B., & Abramson, L. Y. (1979). judgment of contingency in depressed and nondepressed students: Sadder but Wiser?. Journal of Experimental Psychology. General, 108(4), 441–485. doi:10.1037/0096-3445.108.4.441
- Andrews, P. W., & Thomson, J. A. (2009). The bright side of being blue: Depression as an adaptation for analyzing complex problems. Psychological Review, 116(3), 620–654.
- Atkinson, J. W. (1957). Motivational determinants of risk-taking behavior. Psychological Review, 64(6p1), 359.
- Balash, Y., Mordechovich, M., Shabtai, H., Giladi, N., Gurevich, T., & Korczyn, A. D. (2013). Subjective memory complaints in elders: Depression, anxiety, or cognitive decline? Acta Neurologica Scandinavica, 127(5), 344–350. doi:https://doi.org/10.1111/ane.12038
- Ballhausen, N., Hering, A., Rendell, P. G., & Kliegel, M. (2019). Prospective Memory. Prospective Memory across the Lifespan, 135–156. https://doi.org/10.4324/9781351000154-9
- Barbic, S. P., Durisko, Z., & Andrews, P. W. (2014). Measuring the bright side of being blue: A new tool for assessing analytical rumination in depression. PLoS ONE, 9(11), 11. doi:https://doi.org/10.1371/journal.pone.0112077
- Bartoskova, M., Sevcikova, M., Durisko, Z., Maslej, M. M., Barbic, S. P., Preiss, M., & Andrews, P. W. (2018). The form and function of depressive rumination. Evolution and Human Behavior, 39(3), 277–289. https://doi.org/10.1016/j.evolhumbehav.2018.01.005
- Beaudoin, M., Agrigoroaei, S., Desrichard, O., Fournet, N., & Roulin, J. L. (2008). Validation of the French version of the memory self-efficacy questionnaire. Revue Europeenne de Psychologie Appliquee, 58(3), 165–176. https://doi.org/10.1016/j.erap.2007.09.001
- Beck, A. T. 1976 Cognitive therapy and the emotional disorders Int.Univ.Press, New York https://doi.org/10.1176/appi.psychotherapy.1977.31.4.633
- Beck, A. T., Steer, R. A., & Brown, G. K. (1996). BDI-II: Beck Depression Inventory Manual (2nd ed.). San Antonio, TX: Psychological Corporation.
- Brinkmann, K. (2008). Depression and motivation: the influence of dysphoria and task characteristics on cardiovascular measures of motivational intensity, (Doctoral dissertation University of Geneva).
- Brinkmann, K., & Gendolla, G. H. E. (2008). Does depression interfere with effort mobilization? Effects of dysphoria and task difficulty on cardiovascular response. Journal of Personality and Social Psychology, 94(1), 146–157.
- Cauvin, S., Moulin, C. J. A., Souchay, C., Kliegel, M., & Schnitzspahn, K. M. (2019). Prospective memory predictions in aging: Increased overconfidence in older adults. Experimental Aging Research, 45(5), 436–459. doi:https://doi.org/10.1080/0361073X.2019.1664471
- Cherry, K. E., & Lecompte, D. C. (1999). Age and individual differences influence prospective memory. Psychology and Aging, 14(1), 60–76. doi:10.1037/0882-7974.14.1.60
- Cipolli, C., Neri, M., De Vreese, L. P., Pinelli, M., Rubichi, S., & Lalla, M. (1996). The influence of depression on memory and metamemory in the elderly. Archives of Gerontology and Geriatrics, 23(2), 111–127. doi:https://doi.org/10.1016/0167-4943(96)
- Crawford, J., Smith, G., Maylor, E., Della Sala, S., & Logie, R. (2003). The prospective and retrospective memory questionnaire (PRMQ): Normative data and latent structure in a large non-clinical sample. Memory, 11(3), 261–275. doi:https://doi.org/10.1080/09658210244000027
- Crovitz, H. F., & Daniel, W. F. (1984). Measurements of everyday memory: Toward the prevention of forgetting. Bulletin of the Psychonomic Society, 22(5), 413–414. doi:https://doi.org/10.3758/BF03333861
- Cuttler, C., & Graf, P. (2007). Personality predicts prospective memory task performance: An adult lifespan study. Scandinavian Journal of Psychology, 48(3), 215–231. doi:https://doi.org/10.1111/j.1467-9450.2007.00570.x
- de Jong, T. (2019). A bayesian approach to the correction for multiplicity [Preprint]. PsyArXiv. https://doi.org/10.31234/osf.io/s56mk
- Desrichard, O., & Köpetz, C. (2005). A threat in the elder: The impact of task-instructions, self-efficacy and performance expectations on memory performance in the elderly. European Journal of Social Psychology, 35(4), 537–552. doi:https://doi.org/10.1002/ejsp.249
- de Vito, A., Calamia, M., Greening, S., & Roye, S. (2019). The association of anxiety, depression, and worry symptoms on cognitive performance in older adults. Aging, Neuropsychology, and Cognition, 26(2), 161–173. doi:https://doi.org/10.1080/13825585.2017.1416057
- Dietsche, B., Backes, H., Stratmann, M., Konrad, C., Kircher, T., & Krug, A. (2014). Altered neural function during episodic memory encoding and retrieval in major depression. Human Brain Mapping, 35(9), 4293–4302. doi:https://doi.org/10.1002/hbm.22475
- Dobbs, A. R., & Rule, B. G. (1987). prospective memory and self-reports of memory abilities in older adults *. Canadian Journal of Psychology, 41(2), 209–222. doi:10.1037/h0084152
- Enns, M. W., Bernstein, C. N., Kroeker, K., Graff, L., Walker, J. R., Lix, L. M., Hitchon, C. A., El-Gabalawy, R., Fisk, J. D., & Marrie, R. A. (2018). The association of fatigue, pain, depression and anxiety with work and activity impairment in immune mediated inflammatory diseases. PLoS ONE, 13(6), 1–18. https://doi.org/10.1371/journal.pone.0198975
- Escourrou, E., Durrieu, F., Chicoulaa, B., Dupouy, J., Oustric, S., Andrieu, S., & Gardette, V. (2020). Cognitive, functional, physical, and nutritional status of the oldest old encountered in primary care: A systematic review. BMC Family Practice, 21(1), 58. doi:https://doi.org/10.1186/s12875-020-01128-7
- Evans, J., Charness, N., Dijkstra, K., Fitzgibbons, J. M., & Yoon, J. S. (2019). Is episodic memory performance more vulnerable to depressive affect in older adulthood? Aging, Neuropsychology, and Cognition, 26(2), 244–263. https://doi.org/10.1080/13825585.2018.1424314
- Eysenck, M. W., Derakshan, N., Santos, R., & Calvo, M. G. (2007). Anxiety and cognitive performance: Attentional control theory. Emotion, 7(2), 336–353.
- Fernández-theoduloz, G., Paz, V., Nicolaisen-sobesky, E., Pérez, A., & Buunk, A. P. (2019). Supplemental material for social avoidance in depression: A study using a social decision-making task. Journal of Abnormal Psychology, 128(3), 234–244.
- Flavell, J. H. (1979). Metacognition and cognitive monitoring: A new area of cognitive-developmental inquiry. American Psychologist, 34(10), 906–911.
- Folstein, M. F., Folstein, S. E., & McHugh, P. R. (1975). “Mini-mental state.” A practical method for grading the cognitive state of patients for the clinician. Journal of Psychiatric Research, 12(3), 189–198. doi:https://doi.org/10.1016/0022-3956(75)
- Gabriel, R., Oris, M., Studer, M., & Baeriswyl, M. (2015). The persistence of social stratification? A life course perspective on poverty in old-age in Switzerland. Schweizerische Zeitschrift für Soziologie, 41(3), 465–487.
- Gendolla, G. H. (2004). The Intensity of Motivation When the Self is Involved: An Application of Brehm's Theory of Motivation to Effort-Related Cardiovascular Response. Lawrence Erlbaum Associates Publishers.
- Gilmour, H., & Patten, S. B. (2007). Depression and work impairment. Health Reports/Statistics Canada, Canadian Centre for Health Information = Rapports Sur La Santé/Statistique Canada, Centre Canadien d’information Sur La Santé, 18(1), 9–22.
- Girgus, J., Yang, K., & Ferri, C. (2017). the gender difference in depression: Are elderly women at greater risk for depression than elderly men? Geriatrics, 2(4), 35. doi:https://doi.org/10.3390/geriatrics2040035
- Gliem, J. A., & Gliem, R. R. (2003). Calculating, Interpreting, and Reporting Cronbach’s Alpha Reliability Coefficient for Likert-Type Scales. Midwest Research-to-Practice Conference in Adult, Continuing, and Community Education.
- Guerrero-Sastoque, L., Bouazzaoui, B., Burger, L., & Taconnat, L. (2021b). Educational level effect on episodic memory performance in older adults: Mediating role of metamemory. Psychologie. doi:https://doi.org/10.1080/09658211.2019.1707227
- Haas, M., Zuber, S., Kliegel, M., & Ballhausen, N. (2020). Prospective memory errors in everyday life: Does instruction matter?. Memory, 28(2), 196–203. https://doi.org/10.1080/09658211.2019.1707227
- Harkness, K., Sabbagh, M., Jacobson, J., Chowdrey, N., & Chen, T. (2005). Enhanced accuracy of mental state decoding in dysphoric college students. Cognition & Emotion, 19(7), 999–1025. doi:https://doi.org/10.1080/02699930541000110
- Henry, J. D., MacLeod, M. S., Phillips, L. H., & Crawford, J. R. (2004). A meta-analytic review of prospective memory and aging. Psychology and Aging, 19(1), 27–39. https://doi.org/10.1037/0882-7974.19.1.27
- Hering, A., Kliegel, M., Rendell, P. G., Craik, F. I. M., & Rose, N. S. (2018). Prospective memory is a key predictor of functional Independence in older adults. Journal of the International Neuropsychological Society, 24(6), 640–645. doi:https://doi.org/10.1017/S1355617718000152
- Hess, T. M. (2014). Selective engagement of cognitive resources: motivational influences on older adults’ cognitive functioning. Perspectives on Psychological Science, 9(4), 388–407. doi:https://doi.org/10.1177/1745691614527465
- Huber, B. N., Fulton, E. K., & Gray, D. (2022). Meta-prospective memory accuracy in young adults with and without depressive symptoms. Applied Neuropsychology. Adult, 1–12. https://doi.org/10.1080/23279095.2022.2068372
- Huppert, F. A., & Beardsall, L. (1993). Prospective memory impairment as an early indicator of dementia. Journal of Clinical and Experimental Neuropsychology, 15(5), 805–821. doi:https://doi.org/10.1080/01688639308402597
- Iacullo, V. M., Marucci, F. S., & Mazzoni, G. (2016). Inducing false memories by manipulating memory self-efficacy. Learning and Individual Differences, 49, 237–244. doi:https://doi.org/10.1016/j.lindif.2016.06.016
- Ihle, A., Ghisletta, P., Ballhausen, N., Fagot, D., Vallet, F., Baeriswyl, M., Sauter, J., Oris, M., Maurer, J., & Kliegel, M. (2018). The role of cognitive reserve accumulated in midlife for the relation between chronic diseases and cognitive decline in old age: A longitudinal follow-up across six years. Neuropsychologia, 121, 37–46. doi:https://doi.org/10.1016/j.neuropsychologia.2018.10.013
- Ihle, A., Kliegel, M., Ballhausen, N., Da Silva Coelho, C., Haas, M., Hering, A., Künzi, M., Laera, G., Mikneviciute, G., Tinello, D., & Zuber, S. (2019). Prospective memory relates to attentional control: Differential patterns in old age. Dementia and Geriatric Cognitive Disorders, 48(1–2), 79–82. doi:https://doi.org/10.1159/000502639
- James, T. A., Weiss-Cowie, S., Hopton, Z., Verhaeghen, P., Dotson, V. M., & Duarte, A. (2021). Depression and episodic memory across the adult lifespan: A meta-analytic review. Psychological Bulletin, 147(11), 1184–1214. https://doi.org/10.1037/bul0000344
- Joormann, J., & Gotlib, I. H. (2008). Updating the contents of working memory in depression: interference from irrelevant negative material. Journal of Abnormal Psychology, 117(1), 182–192.
- Kliegel, M., Ballhausen, N., Hering, A., Ihle, A., Schnitzspahn, K. M., & Zuber, S. (2016). prospective memory in older adults: Where we are now and what is next. Gerontology, 62(4), 459–466. doi:https://doi.org/10.1159/000443698
- Krys, S. (2020). Goal-directed rumination and its antagonistic effects on problem solving: A two-week diary study. Anxiety, Stress, and Coping, 33(5), 530–544. doi:https://doi.org/10.1080/10615806.2020.1763139
- Kukla, A. (1972). Foundations of an attributional theory of performance. Psychological Review, 79(6), 454.
- Künzi, M., Joly-Burra, E., Zuber, S., Haas, M., Tinello, D., Da Silva Coelho, C., Hering, A., Ihle, A., Laera, G., Mikneviciute, G., Stringhini, S., Draganski, B., Kliegel, M., & Ballhausen, N. (2021). The Relationship between Life Course Socioeconomic Conditions and Objective and Subjective Memory in Older Age. Brain Sciences, 11(1), 61. https://doi.org/10.3390/brainsci11010061
- Kvavilashvili, L., Kornbrot, D. E., Mash, V., Cockburn, J., & Milne, A. (2009). Differential effects of age on prospective and retrospective memory tasks in young, young-old, and old-old adults. Memory, 17(2), 180–196. doi:https://doi.org/10.1080/09658210802194366
- Laera, G., Joly-Burra, E., Zuber, S., Ballhausen, N., Künzi, M., Ihle, A., Da Silva Coelho, C., Haas, M., Mikneviciute, G., Tinello, D., Kliegel, M., & Hering, A. (2021). Do executive functions explain older adults’ health-related quality of life beyond event-based prospective memory? Aging, Neuropsychology, and Cognition, 1–15. https://doi.org/10.1080/13825585.2021.1989368
- Lalive d’Epinay, C. J., & Bickel, J.-F. (2003). Do “young-old” exercisers feel better than sedentary persons? A cohort study in Switzerland. Canadian Journal on Aging/La Revue Canadienne Du Vieillissement, 22(2), 155–165. https://doi.org/10.1017/S0714980800004475
- Lavine, M., & Schervish, M. J. (1999). Bayes factors: What they are and what they are not. American Statistician, 53(2), 119–122. https://doi.org/10.1080/00031305.1999.10474443
- Lee, S.-W., Choi, J.-S., & Lee, M. (2020). Life satisfaction and depression in the oldest old: A longitudinal study. The International Journal of Aging and Human Development, 91(1), 37–59. doi:https://doi.org/10.1177/0091415019843448
- Lee, L., Harkness, K. L., Sabbagh, M. A., & Jacobson, J. A. (2005). Mental state decoding abilities in clinical depression. Journal of Affective Disorders, 86(2–3), 247–258. doi:https://doi.org/10.1016/j.jad.2005.02.007
- Lee, M. D., & Wagenmakers, E.-J. (2013). Bayesian cognitive modeling: A practical course (pp. 276). Cambridge University Press.
- Lim, G. Y., Tam, W. W., Lu, Y., Ho, C. S., Zhang, M. W., & Ho, R. C. (2018). Prevalence of depression in the community from 30 countries between 1994 and 2014 /692/699/476/1414 /692/499 article. Scientific Reports, 8(1), 1–10. doi:https://doi.org/10.1038/s41598-018-21243-x
- Livner, Å., Berger, A. K., Karlsson, S., & Bäckman, L. (2008). Differential effects of depressive symptoms on prospective and retrospective memory in old age. Journal of Clinical and Experimental Neuropsychology, 30(3), 272–279. https://doi.org/10.1080/13803390701380591
- Lövdén, M., Fratiglioni, L., Glymour, M. M., Lindenberger, U., & Tucker-Drob, E. M. (2020). Education and cognitive functioning across the life span. Psychological Science in the Public Interest, 21(1), 6–41. doi:https://doi.org/10.1177/1529100620920576
- Marsh, K. L., & Weary, G. (1994). Severity of depression and responsiveness to attributional information. Journal of Social and Clinical Psychology, 13(1), 15–32. doi:https://doi.org/10.1521/jscp.1994.13.1.15
- McDaniel, M. A., & Einstein, G. O. (2000). Strategic and automatic processes in prospective memory retrieval: A multiprocess framework. Applied Cognitive Psychology, 14( SPEC.ISS), 127–144. https://doi.org/10.1002/acp.775
- McLaren, M. E., Szymkowicz, S. M., Kirton, J. W., & Dotson, V. M. (2015). Impact of education on memory deficits in subclinical depression. Archives of Clinical Neuropsychology, 30(5), 387–393. doi:https://doi.org/10.1093/arclin/acv038
- Mehta, M., Whyte, E., Lenze, E., Hardy, S., Roumani, Y., Subashan, P., Huang, W., & Studenski, S. (2008). Depressive symptoms in late life: Associations with apathy, resilience and disability vary between young-old and old-old. International Journal of Geriatric Psychiatry, 23(3), 238–243. doi:https://doi.org/10.1002/gps.1868
- Mella, N., Vallet, F., Beaudoin, M., Fagot, D., Baeriswyl, M., Ballhausen, N., Métral, G., Sauter, J., Ihle, A., Gabriel, R., Oris, M., Kliegel, M., & Desrichard, O. (2018). Distinct effects of cognitive versus somatic anxiety on cognitive performance in old age: The role of working memory capacity. Aging & mental health, 1–7. https://doi.org/10.1080/13607863.2018.1548566
- Metternich, B., Schmidtke, K., & Hüll, M. (2009). How are memory complaints in functional memory disorder related to measures of affect, metamemory and cognition? Journal of Psychosomatic Research, 66(5), 435–444. doi:https://doi.org/10.1016/j.jpsychores.2008.07.005
- Murphy, M., & O’Leary, E. (2009). Depression, cognitive reserve and memory performance in older adults. International Journal of Geriatric Psychiatry, 25(7), 665–671. doi:https://doi.org/10.1002/gps.2404
- Nelson, T. O., & Narens, L. (1990). Metamemory: A Theoretical Framework and New Findings. Psychology of Learning and Motivation - Advances in Research and Theory, 26(C), 125–173. https://doi.org/10.1016/S0079-7421(08)
- Newman, A. B., & Brach, J. S. (2001). Gender gap in longevity and disability in older persons. Epidemiologic Reviews, 23(2), 343–355. doi:https://doi.org/10.1093/oxfordjournals.epirev.a000810
- Pallant, J. (2020). SPSS survival manual: A step by step guide to data analysis using IBM SPSS. McGraw-hill education (UK).
- Pearman, A., Lustig, E., Hughes, M. L., & Hertzog, C. (2020). Initial evidence for the efficacy of an everyday memory and metacognitive intervention. Innovation in Aging, 4(6), igaa054. https://doi.org/10.1093/geroni/igaa054
- Perrig-Chiello, P., Spahni, S., Höpflinger, F., & Carr, D. (2016). Cohort and gender differences in psychosocial adjustment to later-life widowhood. The Journals of Gerontology. Series B, Psychological Sciences and Social Sciences, 71(4), 765–774. doi:https://doi.org/10.1093/geronb/gbv004
- Philipp, R., Kriston, L., Lanio, J., Kühne, F., Härter, M., Moritz, S., & Meister, R. (2019). Effectiveness of metacognitive interventions for mental disorders in adults—A systematic review and meta-analysis (METACOG). Clinical Psychology & Psychotherapy, 26(2), 227–240. doi:https://doi.org/10.1002/cpp.2345
- Salazar-Villanea, M., Liebmann, E., Garnier-Villareal, M., Montenegro-Montenegro, E., & Johnson, D. K. (2015). Depressive symptoms affect working memory in healthy older adult hispanics. J Depress Anxiety, 4, 4. https://doi.org/10.4172/2167-1044.1000204.Depressive
- Schoen, C. B., & Holtzer, R. (2017). Differential relationships of somatic and cognitive anxiety with measures of processing speed in older adults. Aging, Neuropsychology, and Cognition, 24(5), 481–495. doi:https://doi.org/10.1080/13825585.2016.1226247
- Schoofs, H., Hermans, D., & Raes, F. (2010). Brooding and reflection as subtypes of rumination: evidence from confirmatory factor analysis in nonclinical samples using the dutch ruminative response scale. Journal of Psychopathology and Behavioral Assessment, 32(4), 609–617. doi:https://doi.org/10.1007/s10862-010-9182-9
- Sevcikova, M., Maslej, M. M., Stipl, J., Andrews, P. W., Pastrnak, M., Vechetova, G., Bartoskova, M., & Preiss, M. (2020). Testing the Analytical Rumination Hypothesis: Exploring the Longitudinal Effects of Problem Solving Analysis on Depression. Frontiers in Psychology, 11. https://doi.org/10.3389/fpsyg.2020.01344
- Simard, M., Rouleau, I., Kadlec, H., Taler, V., Tuokko, H., Voll, S., O’Connell, M. E., Griffith, L. E., Wolfson, C., Kirkland, S., & Raina, P. (2019). Miami prospective memory test in the canadian longitudinal study on aging. Clinical Neuropsychologist, 33(1), 137–165. https://doi.org/10.1080/13854046.2018.1435824
- Singh, A., & Misra, N. (2009). Loneliness, depression and sociability in old age. Industrial Psychiatry Journal, 18(1), 51. doi:https://doi.org/10.4103/0972-6748.57861
- Söderlund, H., Moscovitch, M., Kumar, N., Daskalakis, Z. J., Flint, A., Herrmann, N., & Levine, B. (2014). Autobiographical episodic memory in major depressive disorder. Journal of Abnormal Psychology, 123(1), 51–60. https://doi.org/10.1037/a0035610
- Stern, Y. (2009). Cognitive reserve. Neuropsychologia, 47(10), 2015–2028. doi:https://doi.org/10.1016/j.neuropsychologia.2009.03.004
- Stern, Y. (2012). Cognitive reserve in ageing and Alzheimer’s disease. The Lancet Neurology, 11(11), 1006–1012. https://doi.org/10.1016/S1474-4422(12)70191-6
- Sundstrom, W. A. (2004). The college gender gap in the United States, 1940-2000: Trends and international comparisons. SSRN Electronic Journal. doi:https://doi.org/10.2139/ssrn.1104053
- Szajer, J., & Murphy, C. (2013). Education level predicts retrospective metamemory accuracy in healthy aging and Alzheimers disease. Journal of Clinical and Experimental Neuropsychology, 35(9), 971–982. https://doi.org/10.1080/13803395.2013.844771
- Treynor, W., Gonzalez, R., & Nolen-Hoeksema, S. (2003). Rumination reconsidered: A psychometric analysis. In Cognitive Therapy and Research (pp. 13). Plenum Publishing Corporation.
- Vallet, F., Mella, N., Ihle, A., Beaudoin, M., Fagot, D., Ballhausen, N., Baeriswyl, M., Schlemmer, M., Oris, M., Kliegel, M., & Desrichard, O. (2020). Motivation as a mediator of the relation between cognitive reserve and cognitive performance. The Journals of Gerontology: Series B, 75(6), 1199–1205.
- van de Schoot, R., Kaplan, D., Denissen, J., Asendorpf, J. B., Neyer, F. J., & Aken, M. A. G. V. (2014). A Gentle Introduction to Bayesian Analysis: Applications to Developmental Research. Child Development, 85(3), 842–860. doi:https://doi.org/10.1111/cdev.12169
- van Doorn, J., van den Bergh, D., Bohm, U., Dablander, F., Derks, K., Draws, T., Evans, N. J., Gronau, Q. F., Hinne, M., Kucharský, Š., Ly, A., Marsman, M., Matzke, D., Raj, A., Sarafoglou, A., Stefan, A., Voelkel, J. G., & Wagenmakers, E.-J. (2019). The JASP guidelines for conducting and reporting a bayesian analysis. PsyArxiv Preprint, February, 0–31. https://doi.org/10.31234/osf.io/yqxfr
- Vedder, A., Boerner, K., Stokes, J. E., Schut, H. A. W., Boelen, P. A., & Stroebe, M. S. (2022). A systematic review of loneliness in bereavement: Current research and future directions. Current Opinion in Psychology, 43, 48–64. doi:https://doi.org/10.1016/j.copsyc.2021.06.003
- Wang, R. I. H., Treul, S., & Alverno, L. (1975). A brief self‐assessing depression scale. The Journal of Clinical Pharmacology, 15(2–3), 163–167. https://doi.org/10.1002/j.1552-4604.1975.tb02351.x
- Wells, A. (2008a). Emotional disorders and metacognition: Innovative cognitive therapy. Emotional Disorders and Metacognition: Innovative Cognitive Therapy. https://doi.org/10.1002/9780470713662
- Wells, A. (2011). Metacognitive therapy for anxiety and depression. Guilford Press.
- Wilson, F. C. L., & Gregory, J. D. (2018). Overgeneral autobiographical memory and depression in older adults: A systematic review. Aging & mental health, 22(5), 575–586. https://doi.org/10.1080/13607863.2017.1326461
- Woods, S. P., Weinborn, M., Velnoweth, A., Rooney, A., & Bucks, R. S. (2012). Memory for intentions is uniquely associated with instrumental activities of daily living in healthy older adults. Journal of the International Neuropsychological Society, 18(1), 134–138. https://doi.org/10.1017/S1355617711001263
- World Health Organization. (2017, December). Mental health of older adults. https://www.who.int/news-room/fact-sheets/detail/mental-health-of-older-adults
- Yap, A. U. J., Chua, E. K., Tan, K. B. C., & Chan, Y. H. (2004). Relationships between depression/somatization and self-reports of pain and disability. Journal of Orofacial Pain, 18(3), 220–225.
- Yesavage, J. A. (1988). Geriatric depression scale. Psychopharmacology Bulletin, 24(4), 709–711.
- Zandi, T. (2004). Relationship between subjective memory complaints, objective memory performance, and depression among older adults. American Journal of Alzheimer’s Disease and Other Dementias, 19(6), 353–360. https://doi.org/10.1177/153331750401900610
- Zeintl, M., Kliegel, M., & Hofer, S. M. (2007). The role of processing resources in age-related prospective and retrospective memory within old age. Psychology and Aging, 22(4), 826–834. doi:https://doi.org/10.1037/0882-7974.22.4.826
- Zhou, F. C., Wang, Y. Y., Zheng, W., Zhang, Q., Ungvari, G. S., Ng, C. H., Zhang, J., & Xiang, Y. T. (2017). Prospective memory deficits in patients with depression: A meta-analysis. Journal of Affective Disorders, 220(January), 79–85. doi:https://doi.org/10.1016/j.jad.2017.05.042
- Zimmermann, T. D., & Meier, B. (2006). The rise and decline of prospective memory performance across the lifespan. Quarterly Journal of Experimental Psychology, 59(12), 2040–2046. doi:https://doi.org/10.1080/17470210600917835
- Zuber, S., Ihle, A., Loaiza, V. M., Schnitzspahn, K. M., Stahl, C., Phillips, L. H., Kaller, C. P., & Kliegel, M. (2019). Explaining age differences in working memory: The role of updating, inhibition, and shifting. Psychology & neuroscience, 12(2), 191–208. https://doi.org/10.1037/pne0000151
- Zuber, S., & Kliegel, M. (2020). Prospective memory development across the lifespan: An integrative framework. European Psychologist, 25(3), 162–173. doi:https://doi.org/10.1027/1016-9040/a000380