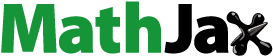
ABSTRACT
The underlying mechanisms for how maternal perinatal obesity and intrauterine environment influence foetal development are not well understood and thus require further understanding. In this paper, energy balance concepts are used to develop a comprehensive dynamical systems model for foetal growth that illustrates how maternal factors (energy intake and physical activity) influence foetal weight and related components (fat mass, fat-free mass, and placental volume) over time. The model is estimated from intensive measurements of foetal weight and placental volume obtained as part of Healthy Mom Zone (HMZ), a novel intervention for managing gestational weight gain in obese/overweight women. The overall result of the modelling procedure is a parsimonious system of equations that reliably predicts foetal weight gain and birth weight based on a sensible number of assessments. This model can inform clinical care recommendations as well as how adaptive interventions, such as HMZ, can influence foetal growth and birth outcomes.
1. Introduction
High infant birth weight is associated with subsequent childhood and adult-onset obesity, type 2 diabetes, cardiovascular disease, and some forms of cancer [Citation1–Citation8]. High maternal body mass index (BMI) and excessive gestational weight gain (GWG) are independent predictors of higher infant fat mass and, in turn, large for gestational age birth weight [Citation9]. High BMI and GWG are also associated with complications such as intrauterine growth restriction, caesarean delivery, and preterm birth [Citation1,Citation10,Citation11]. Nearly 70% of pregnant women in the United States failed to adhere to the Institute of Medicine guidelines for appropriate GWG, with 50% or more women exceeding guidelines [Citation12]. Given the increased risk for high infant birth weight among women who are obese or have excessive GWG, research is warranted to better understand the underlying mechanisms of foetal growth, and efficient interventions are needed to regulate maternal and foetal weight [Citation13].
Our prior work has described a conceptual framework for managing GWG in overweight/obese women [Citation14] and for regulating infant birth weight [Citation15]; this framework relies on methods from control systems engineering to develop decision policies that optimize the adaptation for participant response. The implementation of such a framework calls for developing advanced control systems which rely on dynamical models that are able to predict individualized responses to different intervention components and subsequently predict GWG, the intrauterine growth profile, and infant birth weight [Citation15–Citation17]. In particular, one important end use of a dynamical systems model of intrauterine foetal growth is as the internal model in a model-based controller that accomplishes an optimized, adaptive intervention [Citation18–Citation20].
Energy balance for modelling weight and body composition change has been examined extensively, including among pregnant women [Citation16,Citation21]. Modelling intrauterine growth has received some prior examination [Citation22]; however, further modelling efforts are needed to better understand how prenatal status ‘programs’ foetal growth [Citation23,Citation24]. To address this gap, we use intensive longitudinal data from Healthy Mom Zone (HMZ) [Citation14], an ongoing trial, which is an individually-tailored, adaptive intervention to manage weight gain in overweight and obese pregnant women. While the model developed in this paper extends from prior work [Citation22,Citation25], it grounds a more complete theoretical understanding for how external maternal factors (e.g. daily energy intake and physical activity) influence foetal growth profiles. The following are number of particular advancements made in this work:
A single-output energy balance model. Building from the model in [Citation22], the proposed energy balance model in this work features a single, easy-to-measure output (total foetal weight). In addition to grounding a better theoretical understanding of external factors and pre-existing conditions directly influence intrauterine foetal weight growth, this reformulation highlights less expensive and invasive requirements for estimating individualized model parameters; measurements of total foetal weight can be far more reliable than measurements of body composition (more so in the first trimester [Citation26]).
Application of the Second Law of Thermodynamics. The foetal energy balance model in this work provides a succinct, well-established accounting for the impact of entropy on foetal growth. Despite that the idea of entropy of new tissue formation has originated in Christiansen et al. [Citation25], the work in [Citation25] features an obesity model and the formulation cannot be directly re-purposed for quantifying foetal growth dynamics. Part of the contribution in this work is to merge efforts from Christiansen et al. and Thomas et al. to produce a more rigorous and complete reformulation of foetal growth.
Use of HMZ study data. Utilizing data from the HMZ study [Citation14], the developed foetal model presents a method for quantifying the impact of daily changes of physical activity on foetal growth. Moreover, using intensive, longitudinal participant data from HMZ, it is possible to estimate and validate the general first-principles foetal model structure developed in this work, as well as estimate a logistic profile of foetal fat mass accretion whose structure is supported by the literature.
An improved placental volume model. As is discussed in Section 2.3, in this work, the curvature of the proposed placental volume model is more independently parameterized, which gives a more intuitive and easier model to estimate. This model also implicitly enforces the initial condition at conception; hence, for model estimation and simulation, the proposed model does not require a placental volume measurement for establishing an initial condition.
In this paper, we present parameter estimation and model validation results drawn from four representative HMZ participants. The final foetal energy balance model parameters are estimated by solving a nonlinear least squares optimization problem; the set of estimated model parameters is then used to generate simulations for model validation.
This paper is organized as follows: Section 2 presents the underlying modelling assumptions and describes the derivation of the proposed foetal energy balance model. Section 3 features the optimization problem that accomplishes model parameter estimation from ultrasound measurements, followed by a presentation of the metrics and criteria used for model validation. Section 4 summarizes conclusions and future work.
2. Foetal energy balance model
We begin by outlining important assumptions and simplifications leading to the final proposed foetal energy balance model. Next, building on insights from prior researchers [Citation22,Citation25], we establish a first-principles energy balance model of foetal growth. Following the first law of thermodynamics, this foetal energy balance model applies the conservation of energy principle. Further, the presented derivation explicitly accounts for the energy loss due to new foetal tissue formation, as dictated by the second law of thermodynamics from which it follows that the conversion of energy requires energy. Finally, explicitly defined logistic growth functions are established to estimate the rate of foetal fat mass deposition and the placental volume.
2.1. Model development
2.1.1. Initial assumptions
The following initial assumptions and simplifications lead to the proposed foetal model (equation (21)):
Foetal body mass is divided into two main components: fat and fat-free tissues.
Foetal energy expenditure due to diet-induced thermogenesis is negligible.
Foetal physical activity in the womb is negligible.
The rate of foetal fat-mass deposition is only regulated by the total foetal body mass [Citation25].
The contribution of daily maternal diet to foetal nutrition substantially exceeds additional nutrient supply originating from maternal body components.
Foetal energy imbalances are always positive and follow from the diet of a healthy, well-nourished mother.
The proportion of foetal body fat that contributes to expenditure is equal to that of foetal fat-free tissues.
Following the derivation of the foetal model in Sections 2.1.2 and 2.1.3, a discussion of the rationale for assumptions 6 and 7 is provided.
2.1.2. Energy balance equation
The basis for determining foetal growth is a daily energy balance based on the First Law of Thermodynamics that takes into account the metabolizable energy intake (provided by the mother) and foetal energy expenditure
to define a rate of accumulation of the total foetal energy
. Considering the foetus as the system of interest, we have
with
accounting for foetal energy expenditure towards maintaining and sustaining life () and the energy required for the conversion of excess energy into new tissue (
).
Considering a two-compartment energy balance model (i.e. total body mass divided into fat and fat-free mass components), the positive rate of change of the total combustible foetal energy content, , can also be calculated by accounting for changes of foetal body components [Citation22], giving
which, in turn, when combined with (1), yields
with and
denoting the total foetal fat and fat-free masses, respectively;
and
are the energy densities of the foetal fat and fat-free components, respectively (i.e. energy content per unit fat/fat-free mass). As depicted in equation (4), both
and
are assumed time-invariant.
Equation (4) presents the basic foetal energy balance result following directly from the first law of thermodynamics, as similarly highlighted in the Thomas et al. (2008) model [Citation22]. However, equation (4) involves terms that need to be further defined, are difficult to measure experimentally, or expensive to track in an intervention setting. More specifically, in this work, profiles describing the evolution of the foetal body composition (FM and FFM), portion of maternal energy intake contributing to foetal nutrition, and the influence of maternal physical activity are all terms that are explored and expanded further from (4). Moreover, the expenditure term, , requires estimates for the efficiency of energy conversion into new foetal fat and fat-free tissues (energy loss due to entropy); these efficiencies are difficult and expensive to measure experimentally. Furthermore, given current imaging technologies that build from well-studied sonographic methods to estimate total foetal weight, it is advantageous to reformulate the basic foetal energy balance equation shown as (4) in terms of the total foetal body mass (also referred to as total foetal weight,
). In the following section, the primary aim is to establish a parsimonious foetal energy balance model that proves to overcome these challenges.
2.1.3. Efficiency of energy conversion & energy balance reformulation
The goal of this section is to formulate equation (4) in terms of total foetal weight. To achieve this outcome, we built from concepts used to develop human obesity models by Christiansen et al. (2005) [Citation25]. The time-varying rate of foetal fat mass deposition (with respect to total foetal weight) is defined as follows:
which leads to the following expressions for the rate of change of and
in terms of total foetal weight
,
With an explicitly defined , the components
and
become explicit functions of the total foetal weight,
. Using equations (3) and (6), we now have
Second, we also define the efficiencies of new foetal tissue formation arising from the second law of thermodynamics as follows [Citation27]:
where captures the total energy required to increase the total foetal body energy content by
. The efficiencies in (8) provide a useful parametric representation for the energy loss due to new foetal fat and fat-free tissue formation, respectively. Thus, from equations (6) and (8) we have
As first realized by [Citation25], the dynamic rate of change of can be calculated by establishing the available energy for new foetal tissue deposition; i.e. the difference between the foetal energy intake and the energy expenditure required for sustaining and maintaining life of existing foetal tissues, thus
Combining (9) and (10) gives
which, when substituted into (7), gives
where now the ratio between and
represents the overall time-varying thermodynamic efficiency of energy conversion into new foetal tissue,
; this was first similarly established by [Citation25], however, with a constant
assumed. Inserting equation (2) into equation (1) and contrasting with (12) provides an accounting method for the energy loss due to new tissue formation
with per equation (12).
To establish the term in (12), it is known that the foetal energy intake through the placenta (whose volume is denoted by
) originates mainly from two nutritional sources: maternal diet,
, and maternal body components (e.g. muscles, fats, bones, etc.) [Citation28], hence giving
where is daily total maternal weight;
is a function that captures the daily fraction of maternal body mass directly contributing to foetal nutrition ([=] kcal/kg/d).
denotes the daily glycaemic impact of intake (ranges from 0 to 1);
is a conversion coefficient that is associated with maternal physical activity, as postulated in equation (22) (and discussed later in the paper). However, for the case of a healthy, non-fasting and well-nourished mother, it may be accepted to assume that the basic nutritional needs for foetal growth can be met by daily maternal diet alone [Citation29,Citation30]. Hence, it is assumed that
, giving (identical to [Citation22])
The foetal energy expenditure term in (12) () can be considered, for simplicity, as a direct proportion of total foetal body mass [Citation22]:
where is the daily energy expenditure per unit foetal body mass. Hence, from (7) and (12) we have
Applying equations (15) and (16) gives
Furthermore, dividing equation (18) by and defining
yields a final foetal energy balance equation in terms of the total foetal weight:
Equation (21) features an intrauterine foetal weight growth model that conforms with the description of a first-order quasi Linear Parameter-Varying (quasi-LPV) system whose scheduling variable is the output, i.e. the total foetal weight, . In equation (21), the growth parameter
is the time constant [Citation31] which characterizes the speed of response. The parameters
and
appearing in equation (19) are discussed in the explanation of equations (22) and (30), respectively.
In addition to achieving the goal of reformulating equation (4) in terms of a single, measurable output variable (i.e. the total foetal weight), equation (21) features an intuitive, well-understood first-order dynamical systems model structure that is more amenable to system identification and control. A further outcome following from the development of (21) is that estimates for and
can be determined directly from ultrasound measurements.
Following the development of the model in equation (21) we make the following remarks:
Given that the exact mechanism governing the influence of maternal physical activity on foetal weight is yet to become sufficiently understood, we follow Thomas et al., 2008 [Citation22] in assuming that maternal physical activity influences the placenta function, and thereby influences the foetus’ nutrition. This is further established in Clapp [Citation32] from which it is known that the effect of maternal exercise on foetal growth depends on numerous factors such as type, frequency, intensity, and the time point in pregnancy when the exercise is performed. Hence, for simplicity, we assume that, over a baseline, maternal physical activity is proportional to placental function, which is captured via the
parameter in (21); that is
(22)
(22)
where
denotes the daily maternal physical activity,
is the proportionality constant, and
is the established baseline. Following the literature review presented by [Citation22], we further assume that
and
.
It follows from assumption 6 that
during gestation; thus, from equation (21) we have
(23)
(23)
providing one important criterion for model validation. Additionally, the inequality in (23) can serve as an approximate (yet useful) diagnostic tool indicating rate of foetal growth (as will be discussed later in ).
Kennaugh and Hay (1987) [Citation33] reported estimates where
and
need not be averaged into a single proportion of energy expenditure (energy requirement); in which case, contrary to assumption 7, if
, it can be shown that equation (21) becomes
(24)
(24)
with
where
and
are the proportions of energy expenditure corresponding to maintaining and sustaining life of foetal fat and fat-free tissues, respectively. Nonetheless, given the desire for a parsimonious model, we continue to assume that
, where equation (21) applies.
The model parameters
,
, and
are assumed to vary on an individual level. According to the formulation of (21), these parameters may capture between- and/or within-group differences (e.g. genetic variations [Citation34], exercising vs. non-exercising [Citation22]).
2.2. Rate of foetal fat mass deposition
From Section 2.1, the importance of understanding the rate of foetal fat deposition, , as a key variable to attaining a predictive foetal energy balance model is now clear. From data presented and analyzed in a foetal body composition study by Demerath et al. (2016) [Citation35], good a priori knowledge is now available to establish the dependence of
on
. Literature also strongly suggests that the accretion of foetal fat starts accumulating after 26–30 weeks gestation [Citation22,Citation36]; to this effect, the following piecewise modified logistic equation can be considered
with identifiable parameters ,
,
, and initial time,
, estimated as described in Section 3;
is a constant. When
at
, we get
where is the initial weight at the initial time
. From equations (5) and (25),
is now a well-defined function; namely,
For simplicity, in this work we will assume that (or
). Finally, given a well-defined
(equal to (26) or otherwise), estimations of the foetal body components readily follow from equation (6); namely,
2.3. Placental volume growth model
Following Thomas et al. (2008) [Citation22,Citation37], we consider the placental volume as the most suitable variable to characterize placental growth. There is a substantial literature where placental development and growth profiles are presented and characterized throughout gestation for humans [Citation38–Citation40] and animals [Citation41]. The placenta grows in three phases: first, a ‘lag’ phase in which cells begin to form; second, an exponential growth phase where cells continue to form and rapidly divide; and finally, due to space restrictions, a deceleration in the growth rate is expected in the final weeks towards birth. These three growth phases are adequately captured with a logistic function [Citation22]. features a standard logistic growth profile where these three phases are depicted.
Similar to equation (25), the ‘modified’ logistic function is considered
with and
, where
,
, and
(
is the ‘ultimate’ carrying capacity) are identifiable model parameters from the estimation procedure described in Section 3.2; it follows from that
. The algebraic model in equation (29) differs from the placental volume in [Citation22] in that its curvature features are more independently parameterized: the parameter
assigns the rate of growth,
assigns the inflection point, and
assigns the ultimate carrying capacity or the scale of the profile (note
in for illustration). In [Citation22], the proposed model does not apply when the initial condition is
, and hence, requires an additional estimated ultrasound measurement of EPV. Moreover, the parameters (including the initial condition) of the model in [Citation22] play simultaneous role in determining its final curvature features, which makes it less intuitive.
It has been reported that the size and growth rates of the placenta are associated with physical activity [Citation22,Citation32] and additional genetic factors [Citation42]. In the presence of more intensive ultrasound measurements, the carrying capacity parameter can be further investigated such that moderations of placenta size over time by physical activity or genetic differences are more understood; this also applies to the growth rate
and mid-point
parameters. In our parameter estimation, we assume constant parameters
, and
such that averaged, fixed-effects are captured.
3. Parameter estimation and model validation
3.1. HMZ estimation data
The Healthy Mom Zone (HMZ) study [Citation14] is an ongoing individual-tailored behavioral, adaptive intervention for managing weight in pregnant women with overweight and obesity. The target sample is 30 pregnant women who are randomized to either the intervention or control group from approximately 8 to 36 weeks gestation. Study measures including weight, physical activity, and energy intake. are obtained at baseline, throughout the course of the intervention (e.g. daily, weekly, or monthly), and at follow-up. The detailed intervention protocols that includes eligibility, recruitment, intervention description, dosages, and measurement schedule have been published elsewhere [Citation14]. In addition, an ancillary project provides six ultrasound measures used to estimate foetal weight, placental volume, and foetal body composition. In this section, further discussion of each estimated measurement is presented; four representative completed participants ( = 4; three overweight, one obese; mean age = 30.3 years, two intervention, two control) are considered.
3.1.1. Estimated foetal weight
An estimated foetal weight (EFW) can be drawn from ultrasounds when specific biomarkers are measured as displayed in the example . Using these biomarkers, one of the best known and well-established correlations that can be applied is the Hadlock et al. (1984–91) [Citation43] estimation. For our model estimation, we use a set of six EFW measurements in addition to birth weight, as is described in more detail in Section 3.2. The first EFW is used to establish the upper and lower bounds for the initial condition used for solving equation (21). In this study, on average, the first ultrasound measurement was taken at 14 weeks gestation, followed by five additional measurements each every four weeks through 34 weeks gestation. Infant’s birth weight was measured immediately after delivery.
3.1.2. Estimated placental volume
As the case with EFW, up to six ultrasound measurements are used to obtain the estimated placental volume (EPV) measurements using the Azupura et al. (2010) approximation method, for which a number of simplifying assumptions have been made [Citation37]. As detailed in Section 3.2.2, EPV measurements are incorporated in the estimation cost function with lower emphasis than EFW measurements. This is justified given the following:
Absence of EPV measurements at or near birth. The bias that can result from equally emphasizing EPV measurements with EFW in (32) given the missing value at birth is crucial since fitting to earlier measurements only will tend to produce an exponential growth profile that can be, misleadingly, well-captured with the modified logistic equation in (29).
The Azpurua et al. EPV estimation method using 2D ultrasound measurements (similar to ) provides a rather simplified approximation (assumes spherical topology) that is mainly targeted for establishing EPV in the first and second trimesters of pregnancy for patients with normal BMI index (in this paper, participants are either overweight or obese); this approximation can become exceedingly inaccurate at advanced gestational ages due to remaining technical difficulties associated with existing ultrasound technology [Citation37].
The EPV approximation method in Azpurua et al. does not estimate standard errors; only 10th, 50 th, and 90 th percentile trajectories are given.
3.1.3. Foetal body composition
Studying ultrasound reports similar to (namely, anterior abdominal wall thickness and abdominal circumference) also produced at least two acceptable estimates per participant for the foetal % body fat using the correlation presented by Bernstein and Catalano (1991) [Citation26]. While the number of estimates can be as many as available ultrasounds, it is known that this estimate becomes more reliable at advanced gestational ages, and therefore we only consider a subset of the ultrasound measurements for the estimation of foetal % body fat.
3.1.4. Glycaemic index
Glycaemic index (GI) was estimated using food items and portion sizes reported in a smart phone application. For each food, carbohydrate content (g) of the reported portion size was determined using the USDA Food and Nutrient Database for Dietary Studies (FNDDS) 2013–2014 data set. Next, a GI value for each food was determined by matching foods to the database generated by Flood et al. [Citation44]. For foods without an exact match, the GI value of the closest matching item was used. Estimated GI of each day was then calculated as the average GI of all foods consumed in a day, weighted by their contribution to total carbohydrate intake for that day, i.e.
In equation (21), , is understood as a key variable for estimating foetal energy intake. One can generally presume a time-varying profile of
on a daily scale; however, given that the collected
time series shows to be stationary with a low variance, one can assume a constant
value drawn from the average of all available average daily estimates,
. lists the fractional average and standard deviation of estimated daily GI values for the four participants presented in this paper.
Table 1. Mean and standard deviation values of daily glycaemic index estimations for four representative HMZ participants.
3.1.5. Maternal physical activity
As noted in section 2.1.3, equation (22) characterizes the assumed simple, linear dependence of the foetal energy balance model in (21) on maternal physical activity. It is assumed that physical activity moderates the energy intake to the foetus by regulating the placental function (e.g. through blood flow [Citation45]). In the HMZ study, intensive objective assessment of physical activity is carried out using wrist-worn activity tracker. Missing and implausible physical activity measurements are imputed with mean replacement. These data are also used to establish the estimated daily maternal energy intake in equation (31).
3.1.6. Maternal energy intake
The daily maternal energy intake variable, , can be reliably estimated with the availability of daily maternal weights and estimated energy expenditure data; the latter are estimated by correlating with daily physical activity and estimated/measured resting metabolic rates. As presented in Guo et al. (2016) [Citation17], back-calculated maternal energy intake from measured daily maternal weights and physical activity measures is considered; namely
where and
are gains (coefficients) that map changes of daily energy intake and physical activity, respectively, into maternal weight gain/loss;
is the sampling time;
and
are the maternal daily physical activity and resting metabolic rate, respectively. To reduce the significant variability in equation (31), it is necessary to smooth the weight measurement
. A 9 day moving average filter is considered for all participants, except for participant D where a 13 day moving average filter is considered.
3.2. Model estimation problem formulation
In this section, we establish a problem formulation for the least squares objective from which, with the presence of sufficient estimation and validation data, model parameters can be estimated and validated using nonlinear regression. Next, we describe in more detail how emphasis is split between different measured variables, and how the nonlinear optimization solver is initialized. Finally, in the results section, we present simulations of the estimated individual models and list the mean value and standard deviation associated with all model parameters.
3.2.1. Problem formulation
The parameter estimation problem statement is formulated as a constrained optimization problem. The prediction error is minimized over estimation data using a non-linear least squares objective. For model estimation, using a total of EFW measurements inferred from ultrasound reports (similar to the example sonographic images shown in , including birth weight),
EPV measurements, and
estimated body composition data points, the approach considered is to solve
where
,
, and
with
,
, and
denoting the estimated ultrasound measurements of the foetal weight, placental volume, and foetal fat mass at day
, respectively;
is a positive semi-definite weighting matrix used to establish the desired emphasis for model estimation.
is obtained from the numerical solution of the following foetal model
with
with (initial time of simulation) and
per Equation (31).Lower and upper parameter bounds,
and
, are known a priori. The physical activity parameter
is constrained as shown in Equation (33c). In this paper, for purposes of simplicity, the value of the
parameter is fixed at 0.000234 ml
, which is equal to the estimated nominal value of
in [Citation22]. Thermodynamic efficiencies
and
, by definition, range from 0 to 1. Also, given the strict growth of both
and
profiles, the parameters
and
are bounded below at
, and are unbounded above.
The optimization is initialized using nominal parameter values/ranges drawn from literature. For example, Christiansen et al. [Citation25,Citation34] reports values for thermodynamic efficiencies drawn from animal studies; Thomas et al. [Citation22] gives an estimate for the conversion parameter, ; Demerath et al. [Citation35] provides fat and fat-free mass profiles from preterm infants that are used for initializing
,
and
using standard regression; finally, also by similar means, EPV measurements calculated from our ultrasound data are used for initializing
,
, and
. In the following section, we report in additional detail on the final set of parameter values used for solver initialization.
3.2.2. Relative weights and initialization
In this section, the specific relative weights ( in the diagonal
matrix in equation (32)) are presented for each participant. In addition, the specific initialization points (initial guesses) are also established in this section. It must be noted that given the limited amount of estimation data and the non-convexity of the optimization problem, the non-linear least squares solver becomes increasingly sensitive to relative weights and proper initialization as multiple local minima are expected. To avoid undesired solutions, solver features such as multistart can be used [Citation46].
Judicious selection of values is important for establishing an effective estimation cost function for each of the HMZ participants evaluated with this method. In the selection of
values, output emphasis, scaling, number of measurements, and measurement standard errors are all taken into consideration. While each data point can have its specific assigned
weight, we group measurements per model state (i.e. EFW, EPV, foetal FM) with one relative weight as
. For participant A, the established ratios are
, whereas for participants B, C, and D the ratios are
.
lists established initialization points for the studied HMZ participants. In the selection of these initializations, approximations from the literature, actual measurement values, and multiple iterations are all influencing factors. More specifically, initial guesses for and
were drawn from [Citation25] followed by multiple iterations (multiple solutions);
was drawn from [Citation22];
,
,
,
, and
were initialized from examining the actual measurements followed by multiple iterations; finally, the initialization of
was established after multiple iterations.
Table 2. Initialization points for four representative HMZ participants.
3.2.3. Estimation results
In this section, for each of the examined HMZ participants, qualitative and quantitative model fit to data are presented from simulations when actual measured inputs are applied to the model. In addition to the intrauterine foetal weight (primary model state), other model states (i.e. placental volume, body composition) and the evolution of intermediate constructs over time (e.g. ,
, and
) are also shown. Finally, estimated model parameters tabulated in are discussed.
Table 3. Estimated model parameter values for four representative HMZ participants (Mean and Standard Deviation (SD) are included).
– feature simulations of the estimated models for one intervention participant (participant A) and one control participant (participant B). Overall, the goodness of fit does not appear to differ across intervention and control participants. In –, the simulation start time is selected to match the day of the first ultrasound measurement; the simulation is carried out through the reported actual day of birth. In these simulations, measurements of the two model inputs, i.e. maternal energy intake (back-calculated EI) and maternal PA (direct measurements), are displayed. In addition, the model states, i.e. foetal weight, placental volume, and body composition, are plotted and contrasted against estimated ultrasound measurements to qualitatively demonstrate the goodness of fit. Moreover, in , , and from Appendix A featuring the time-varying profiles of ,
, and
, it can be seen that, across all individuals, both
and
appear to exponentially increase over time as the foetus continues to grow. It is noted that [Citation22] provides a significantly higher estimate for the overall efficiency (
) than the estimated ranges from our data (approximately, in the 0.1–0.4 range). Finally, summarizes the estimated model parameters with mean and standard deviation values for the examined participants.
Figure 3. Time-domain response (foetal weight, placental volume, and foetal % body fat) with energy intake and physical activity for a representative HMZ intervention participant (participant A) (simulation starts at the day of first ultrasound measurement and ends at birth).
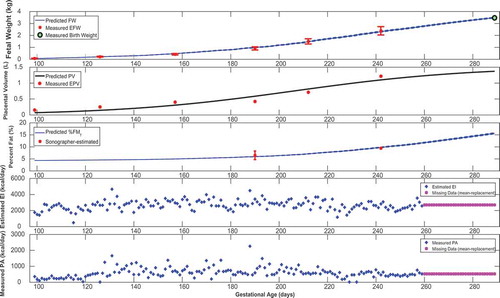
Figure 4. Time-domain response (foetal weight, placental volume, and foetal % body fat) with energy intake and physical activity for a representative HMZ control participant (participant B) (simulation starts at the day of first ultrasound measurement and ends at birth).
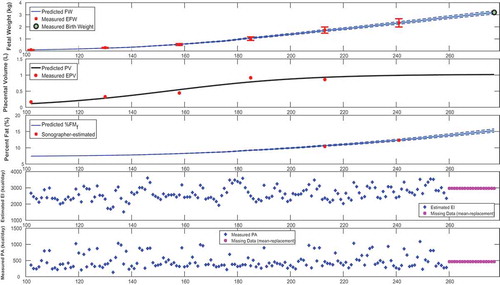
3.3. Model validation
Model validation is determined by goodness-of-fit metrics as well as contrasting diverse estimated model features such as structure, parameter ranges, and output profiles against a priori knowledge from the literature.
First, all simulated foetal weight and placental volume growth profiles in , , , and are plausible and consistent with expected growth profiles from literature (Hadlock et al. [Citation43,Citation47,Citation48]; Arleo et al. [Citation49]). Comparing model predictions against the experimentally observed data (ultrasounds), a summary of individualized model outputs fit against available data is presented in .
Table 4. Summary of the goodness-of-fit from various metrics for four representative HMZ participants.
The Normalized Root-Mean-Square Error (NRMSE) is defined as
is considered as the primary metric for establishing the model goodness of fit against the HMZ data. is the simulated output,
is the measured output,
is the mean of all measured
values, and
denotes the
-norm.
,
, and
denote the coefficients of determination for fitting to estimated foetal weights, placental volumes, and foetal fat mass in utero, respectively. For a qualitative evaluation of model fit, the reader may refer to –.
Further giving validity to our model is that the estimated values are consistently larger than
, in agreement with reported patterns only available from animal studies [Citation25]. Moreover, the mean estimated value of the placental volume growth rate parameter
(with a narrow standard deviation of
) matches the reported and validated value in [Citation22,Citation50]:
. Furthermore, from , , , and , predicted % body fat at birth approximately ranges from 10 to 18%, which fall into the typical ranges reported in literature [Citation26,Citation36,Citation51,Citation52]. In agreement with Demerath et al. (2016) [Citation35], and show that predicted
profiles can be described as linear, while the
are curvilinear (linear-exponential).
Finally, confirms that, except for only two brief instances in Participant A’s simulations, all estimated models satisfy the constraint in equation (23) and hence validates the positive energy balance assumption throughout gestation. From , one can observe the estimated rate of foetal energy intake (note the negative
values in the two instances where Participant A’s positivity constraint is violated); comparing this to the maternal energy intake
(‘Estimated EI’ in , , , and ) provides support for the assumption of a well-nourished mother.
4. Conclusions and future work
In conclusion, a dynamical systems model of intrauterine growth has been developed from first-principles, relying on the first and second laws of thermodynamics. This proposed model provides a rigorous yet more simple formulation than the foetal energy balance model of current literature (Thomas et al., 2008). In the parameter estimation of this model, a non-linear least squares, constrained multi-objective optimization problem was formulated and guided by a priori knowledge of ranges of model parameters. For the first time (to the authors’ knowledge), estimates (and an estimation method) for the thermodynamic efficiencies governing the formation of new tissues of human foetuses are established. This developed model has been estimated and validated against ultrasound measurements provided from the Healthy Mom Zone study; despite the explained challenges with the estimation measurements, predictions follow from this model show good agreement with the data.
The availability of more intensive estimation and validation datasets (i.e. datasets with more frequent measurements) in a future study should create opportunities for parameter refinement and increased model understanding. More intensive measurements will allow for further investigation of the contribution of maternal body components in foetal nutrition (see Equation (14)). Moreover, additional ultrasound measurements (particularly closer to delivery) may allow estimation of a less biased foetal model described in Equation (24). A better theoretical understanding of mechanisms behind the evolution of placental volume and the rate of foetal fat mass deposition is needed. This work considered a linear dependence of placental function on maternal physical activity; in future work, a more developed characterization of the influence of maternal physical activity may generate more resilient models: models with good predictions when input levels are far from those used in model estimation. Furthermore, a broader future goal is to use a combination of more experimental data and increased physiological understanding to reduce the modelling assumptions (outlined in Section 2.1) as much as possible.
Finally, the aims of this paper (achieved using a limited number of HMZ intervention and control participants) was to develop a more comprehensive energy balance model for foetal weight gain derived from first-principles modelling that can be validated through data. These aims were facilitated by the availability of intensive, longitudinal participant data from the HMZ intervention, which is ongoing. Model estimation and validation efforts for the remaining participants () could enable making conclusions regarding participant differences and intervention versus control outcomes, which was not the scope of this paper. However, studying group differences (intervention vs. control) is a subject of current and future research.
Acknowledgments
The authors acknowledge Dr. Jaimey Pauli (Penn State College of Medicine), Dr. Harvey J. Kliman (Yale School of Medicine), and Krista Leonard, Abigail M. Pauley, Katherine McNitt, and Lindsey Hess from Penn State University for their assistance with this project. Advice from Dr. Diana M. Thomas (US Military Academy, West Point) and Dr. Paulo Lopes dos Santos (University of Porto) is acknowledged and greatly appreciated.
Support for this work has been provided by the National Institutes of Health (NIH) through grants R56-HL126799 and R01-HL119245. The opinions expressed in this article are the authors’ own and do not necessarily reflect the views of NIH.
Disclosure statement
No potential conflict of interest was reported by the authors.
Additional information
Funding
References
- T.A. Hillier, K.L. Pedula, M.M. Schmidt, J.A. Mullen, M.-A. Charles, and D.J. Pettitt, Childhood obesity and metabolic imprinting, Diabetes Care 30 (9) (2007), pp. 2287–2292. doi:10.2337/dc06-2361.
- Y. Qiao, J. Ma, Y. Wang, W. Li, P.T. Katzmarzyk, J.-P. Chaput, M. Fogelholm, W.D. Johnson, R. Kuriyan, A. Kurpad, et al., Birth weight and childhood obesity: A 12-country study, Int J Obes Supplements 5 (2015), pp. S74–S79. doi:10.1038/ijosup.2015.23.
- P.H. Whincup, S.J. Kaye, C.G. Owen, R. Huxley, D.G. Cook, S. Anazawa, E. Barrett-Connor, S.K. Bhargava, B.E. Birgisdottir, S. Carlsson, et al., Birth weight and risk of type 2 diabetes: A systematic review, J Am Med Assoc (JAMA) 300 (24) (2008), pp. 2886–2897. doi:10.1001/jama.2008.886.
- J.W. Rich-Edwards, M.J. Stampfer, J.E. Manson, B. Rosner, S.E. Hankinson, G.A. Colditz, C.H. Hennekens, and W.C. Willet, Birth weight and risk of cardiovascular disease in a cohort of women followed up since 1976, BMJ 315 (7105) (1997), pp. 396–400. doi:10.1136/bmj.315.7105.396.
- D. Barker, The developmental origins of adult disease, J Am Coll Nutr 23 (sup6) (2004), pp. 588S–595S. doi:10.1080/07315724.2004.10719428.
- K.A. O’Neill, M.F. Murphy, K.J. Bunch, S.E. Puumala, S.E. Carozza, E.J. Chow, B.A. Mueller, C.C. McLaughlin, P. Reynolds, T.J. Vincent, et al., Infant birthweight and risk of childhood cancer: International population-based case control studies of 40,000 cases, Int J Epidemiol 44 (1) (2015), pp. 153–168. doi:10.1093/ije/dyv069.
- M. Ahlgren, J. Wohlfahrt, L.W. Olsen, T.I. Sørensen, and M. Melbye, Birth weight and risk of cancer, Cancer 110 (2) (2007), pp. 412–419. doi:10.1002/cncr.22812.
- C.N. Spracklen, R.B. Wallace, S. Sealy-Jefferson, J.G. Robinson, J.L. Freudenheim, M.F. Wellons, A.F. Saftlas, L.G. Snetselaar, J.E. Manson, L. Hou, et al., Birth weight and subsequent risk of cancer, Cancer Epidemiol 38 (5) (2014), pp. 538–543. doi:10.1016/j.canep.2014.07.004.
- Z. Ferraro, N. Barrowman, D. Prud’Homme, M. Walker, S. Wen, M. Rodger, and K. Adamo, Excessive gestational weight gain predicts large for gestational age neonates independent of maternal body mass index, J Maternal-Fetal Med 25 (5) (2012), pp. 538–542. doi:10.3109/14767058.2011.638953.
- E. Oken, E.M. Taveras, K.P. Kleinman, J.W. Rich-Edwards, and M.W. Gillman, Gestational weight gain and child adiposity at age 3 years, Am J Obstetrics Gynecol 196 (4) (2007), pp. 322–e1. doi:10.1016/j.ajog.2006.11.027.
- M. Haugen, A.L. Brantsæter, A. Winkvist, L. Lissner, J. Alexander, B. Oftedal, P. Magnus, and H.M. Meltzer, Associations of pre-pregnancy body mass index and gestational weight gain with pregnancy outcome and postpartum weight retention: A prospective observational cohort study, BMC Pregnancy Childbirth 14 (1) (2014), pp. 201. doi:10.1186/1471-2393-14-201.
- J.W. Dudenhausen, A. Grünebaum, and W. Kirschner, Prepregnancy body weight and gestational weight gain—Recommendations and reality in the USA and in Germany, Am J Obstetrics Gynecol 213 (4) (2015), pp. 591–592. doi:10.1016/j.ajog.2015.06.016.
- National Research Council, Weight Gain during Pregnancy: Reexamining the Guidelines, National Academies Press, Washington, DC, 2010.
- D. Symons Downs, J.S. Savage, D.E. Rivera, J.M. Smyth, B.J. Rolls, E.E. Hohman, K.M. McNitt, A.R. Kunselman, C. Stetter, A.M. Pauley, K.S. Leonard, and P. Guo, Individually tailored, adaptive intervention to manage gestational weight gain: Protocol for a randomized controlled trial in women with overweight and obesity, JMIR Res Protoc. 7 (2018), pp. e150. doi:10.2196/resprot.9220
- J.S. Savage, D. Symons Downs, Y. Dong, and D.E. Rivera, Control systems engineering for optimizing a prenatal weight gain intervention to regulate infant birth weight, Am J Public Health 104 (7) (2014), pp. 1247–1254. doi:10.2105/AJPH.2014.302167.
- D.M. Thomas, J.E. Navarro-Barrientos, D.E. Rivera, S.B. Heymsfield, C. Bredlau, L.M. Redman, C.K. Martin, S.A. Lederman, L.M. Collins, and N.F. Butte, Dynamic energy-balance model predicting gestational weight gain, Am J Clin Nutr 95 (1) (2012), pp. 115–122. doi:10.3945/ajcn.111.024307.
- P. Guo, D.E. Rivera, D. Symons Downs, and J.S. Savage, “Semi-physical identification and state estimation of energy intake for interventions to manage gestational weight gain,” in 2016 American Control Conference (ACC), pp. 1271–1276, July 2016.
- D.E. Rivera, M.D. Pew, and L.M. Collins, Using engineering control principles to inform the design of adaptive interventions: A conceptual introduction, Drug Alcohol Depend 88 (2) (2007), pp. S31–S40. doi:10.1016/j.drugalcdep.2006.10.020.
- D.E. Rivera, C.A. Martín, K.P. Timms, S. Deshpande, N.N. Nandola, and E.B. Hekler, Control systems engineering for optimizing behavioral mHealth interventions, in Mobile Health. Sensors, Analytic Methods, and Applications, J.M. Rehg, S.A. Murphy, and S. Kumar, eds., Springer International Publishing, New York, NY, 2017, pp. 455–493.
- D.E. Rivera, E.B. Hekler, J.S. Savage, and D. Symons Downs, Intensively adaptive interventions using control systems engineering: Two illustrative examples, in Optimization of Behavioral, Biobehavioral, and Biomedical Interventions: Advanced Topics, L.M. Collins and K.C. Kugler, eds., Springer, New York, NY, 2018, pp. 121–173.
- K.D. Hall, Estimating human energy intake using mathematical models, Am J Clin Nutr 100 (3) (2014), pp. 744–745. doi:10.3945/ajcn.114.094441.
- D.M. Thomas, J.F. Clapp, and S. Shernce, A foetal energy balance equation based on maternal exercise and diet, J Royal Soc Interface 5 (21) (2008), pp. 449–455. doi:10.1098/rsif.2007.1161.
- P.C. Chandler-Laney and N.C. Bush, Maternal obesity, metabolic health, and prenatal programming of offspring obesity, Open Obes J 3 (2011), pp. 42–50. doi:10.2174/1876823701103010042.
- P. Catalano and H. Ehrenberg, Review article: The short-and long-term implications of maternal obesity on the mother and her offspring, BJOG: Int J Obstetrics Gynaecol 113 (10) (2006), pp. 1126–1133. doi:10.1111/j.1471-0528.2006.00989.x.
- E. Christiansen, L. Garby, and T.I. Sørensen, Quantitative analysis of the energy requirements for development of obesity, J Theor Biol 234 (1) (2005), pp. 99–106. doi:10.1016/j.jtbi.2004.11.012.
- I.M. Bernstein and P.M. Catalano, Ultrasonographic estimation of fetal body composition for children of diabetic mothers, Invest Radiol 26 (8) (1991), pp. 722–726. doi:10.1097/00004424-199108000-00005.
- Y.A. Çengel and M.A. Boles, Thermodynamics: An Engineering Approach (Mcgraw-Hill Series in Mechanical Engineering), McGraw-Hill Science/Engineering/Math, New York, NY, 2005.
- D. Barker, Nutrition in the Womb: How Better Nutrition during Development Will Prevent Heart Disease, Diabetes and Stroke: An Account of the Developmental Origins of Health and Disease, and a Call for Action, David Barker, Northwich, Cheshire, UK, 2008.
- J. Langhoff-Roos, G. Lindmark, and M. Gebre-Medhin, Maternal fat stores and fat accretion during pregnancy in relation to infant birthweight, Br J Obstet Gynaecol 94 (1987), pp. 1170–1177. doi:10.1111/j.1471-0528.1987.tb02318.x.
- I. Cetin, G. Alvino, and M. Cardellicchio, Long chain fatty acids and dietary fats in fetal nutrition, J Physiol (Lond) 587 (14) (2009), pp. 3441–3451. doi:10.1113/jphysiol.2009.173062.
- B.A. Ogunnaike and W.H. Ray, Process Dynamics, Modeling, and Control, Oxford University Press, New York, 1994.
- J.F. Clapp, The effects of maternal exercise on fetal oxygenation and feto-placental growth, Eur J Obstetrics Gynecol Reprod Biol 110 (2003), pp. S80–S85. doi:10.1016/S0301-2115(03)00176-3.
- J.M. Kennaugh and W.W. Hay Jr, Nutrition of the fetus and newborn, West J Med 147 (1987), pp. 435.
- J. Noblet, C. Karege, S. Dubois, and J. Van Milgen, Metabolic utilization of energy and maintenance requirements in growing pigs: Effects of sex and genotype., J Anim Sci 77 (5) (1999), pp. 1208–1216. doi:10.2527/1999.7751208x.
- E.W. Demerath, W. Johnson, B.A. Davern, C.G. Anderson, J.S. Shenberger, S. Misra, and S.E. Ramel, New body composition reference charts for preterm infants, Am J Clin Nutr. 105 (2016), pp. 70–77. doi:10.3945/ajcn.116.138248
- J. Schwartz and H. Galan, Ultrasound in assessment of fetal growth disorders: Is there a role for subcutaneous measurements? Ultrasound in Obstetrics & Gynecology 22 (4) (2003), pp. 329–335. doi:10.1002/uog.887.
- H. Azpurua, E.F. Funai, L.M. Coraluzzi, L.F. Doherty, I.E. Sasson, M. Kliman, and H.J. Kliman, Determination of placental weight using two-dimensional sonography and volumetric mathematic modeling, Am J Perinatol 27 (2) (2010), pp. 151–155. doi:10.1055/s-0029-1234034.
- R.M. Pitkin, Nutritional support in obstetrics and gynecology, Clin Bbstetrics Gynecol 19 (3) (1976), pp. 489–513. doi:10.1097/00003081-197609000-00002.
- J. Thompson, L. Irgens, R. Skjaerven, and S. Rasmussen, Placenta weight percentile curves for singleton deliveries, BJOG: Int J Obstetrics Gynaecol 114 (6) (2007), pp. 715–720. doi:10.1111/j.1471-0528.2007.01327.x.
- J. Wallace, S. Bhattacharya, and G. Horgan, Gestational age, gender and parity specific centile charts for placental weight for singleton deliveries in Aberdeen, UK, Placenta 34 (3) (2013), pp. 269–274. doi:10.1016/j.placenta.2012.12.007.
- J. Mu, J.C. Slevin, D. Qu, S. McCormick, and S.L. Adamson, In vivo quantification of embryonic and placental growth during gestation in mice using micro-ultrasound, Reprod Biol Endocrinol 6 (1) (2008), pp. 34. doi:10.1186/1477-7827-6-34.
- T.R. Regnault, S.W. Limesand, and W.W. Hay Jr, Factors influencing fetal growth, NeoReviews 2 (6) (2001), pp. e119–e128. doi:10.1542/neo.2-6-e119.
- F.P. Hadlock, R.B. Harrist, and J. Martinez-Poyer, In utero analysis of fetal growth: A sonographic weight standard., Radiology 181 (1) (1991), pp. 129–133. doi:10.1148/radiology.181.1.1887021.
- A. Flood, A.F. Subar, S.G. Hull, T.P. Zimmerman, D.J. Jenkins, and A. Schatzkin, Methodology for adding glycemic load values to the National Cancer Institute diet history questionnaire database, J Am Diet Assoc 106 (3) (2006), pp. 393–402. doi:10.1016/j.jada.2006.07.012.
- Z.M. Ferraro, L. Gaudet, and K.B. Adamo, The potential impact of physical activity during pregnancy on maternal and neonatal outcomes, Obstet Gynecol Surv 67 (2) (2012), pp. 99–110. doi:10.1097/OGX.0b013e318242030e.
- Z. Ugray, L. Lasdon, J. Plummer, F. Glover, J. Kelly, and R. Mart, Scatter search and local nlp solvers: A multistart framework for global optimization, INFORMS J Comput 19 (3) (2007), pp. 328–340. doi:10.1287/ijoc.1060.0175.
- F. Hadlock, R. Harrist, R. Carpenter, R. Deter, and S. Park, Sonographic estimation of fetal weight. the value of femur length in addition to head and abdomen measurements., Radiology 150 (1984), pp. 535–540.
- F.P. Hadlock, R. Harrist, R.S. Sharman, R.L. Deter, and S.K. Park, Estimation of fetal weight with the use of head, body, and femur measurements—A prospective study, Am J Obstetrics Gynecol 151 (1985), pp. 333–337.
- E.K. Arleo, R.N. Troiano, R. Da Silva, D. Greenbaum, and H.J. Kliman, Utilizing two-dimensional ultrasound to develop normative curves for estimated placental volume, Am J Perinatol 31 (2014), pp. 683–688.
- K. Orzechowski, D. Thomas, C.J. McNamara, and R.C. Miller, Volumetric assessment of longitudinal placental growth., Obstetrics Gynecol 123 (2014), pp. 164S.
- E.M. Widdowson and C.M. Spray, Chemical development in utero, Arch Dis Child 26 (1951), pp. 205.
- E.W. Demerath and D.A. Fields, Body composition assessment in the infant, Am J Hum Biol 26 (2014), pp. 291–304.
Nomenclature
Appendix A
Simulations in support of Section 3.3 featuring additional HMZ participants (participants C and D), ancillary time-domain responses, and positive energy balance.
Figure A1. Time-domain response (foetal weight, placental volume, and foetal % body fat) with energy intake and physical activity for a representative HMZ intervention participant (participant C) (simulation starts at the day of first ultrasound measurement and ends at birth).
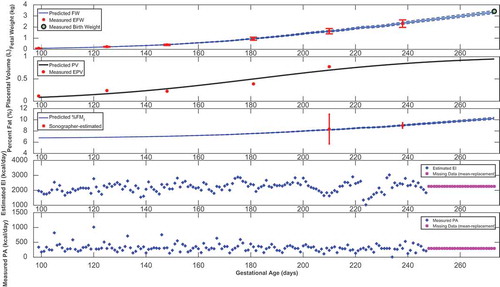
Figure A2. Time-domain response (foetal weight, placental volume, and foetal % body fat) with energy intake and physical activity for a representative HMZ control participant (participant D) (simulation starts at the day of first ultrasound measurement and ends at birth).
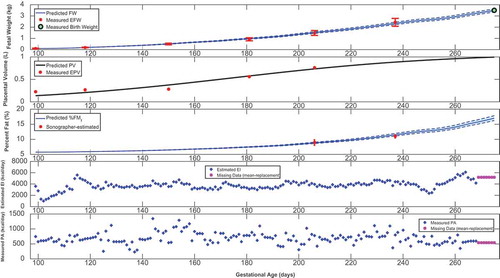
Figure A3. Foetal fat mass and fat-free mass growth profiles over time for representative HMZ participants (participants C and D).
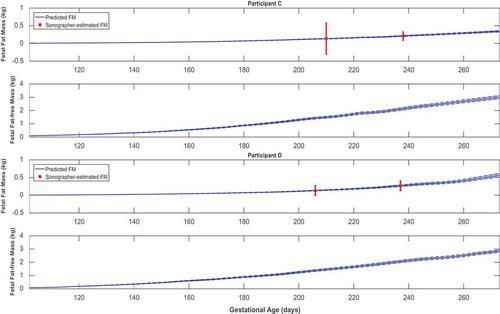
Figure A5. Time-varying gain and the establishment of positive foetal energy balance for representative HMZ participants (see equation (23)).
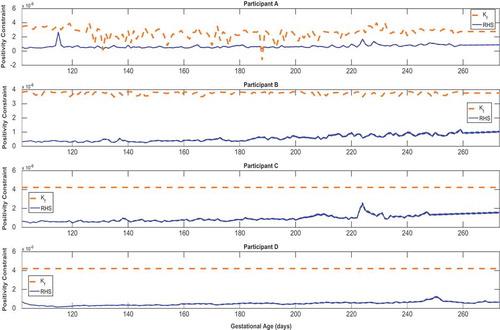
Figure A6. Predicted time-domain profile of foetal energy intake for representative HMZ participants (see equation (15)).
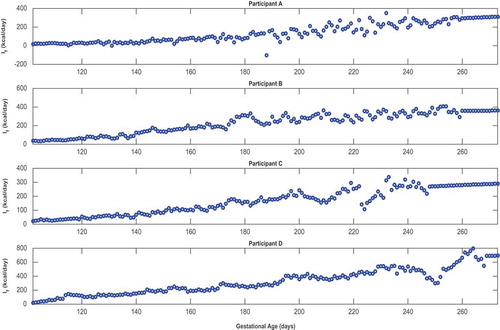