ABSTRACT
This paper examines whether the use of blood pressure medication has an influence on social inequalities in blood pressure levels. In Norway, cardiovascular disease has for decades been associated with high mortality and social inequalities. High blood pressure is an important risk factor in this aspect, and prescription drugs have been established as a standard treatment of hypertension. We have seen population blood pressure levels fall, blood pressure inequality levels remaining stabile, and medication use increase. The paper uses panel data from the Nord-Trøndelag Health Study linked with registry data on education and income. Results from fixed effects regression analyses indicate that blood pressure medication overall has a levelling effect. The traditional social gradient is mainly found among non-users of medication. With blood pressure medication being plausibly at a late stage of its diffusion, these findings give some support to the hierarchical diffusion model, while they also imply the need for equal access to sufficient blood pressure treatment.
Introduction
Health problems are disproportionally distributed between the more and less affluent in society. These patterns, often referred to as social inequalities in health, have been widely reported in all Western countries, including egalitarian Norway, during the last decades (Mackenbach, Citation2017). Innovative technologies have been proposed as means to reduce health inequalities, as well as other challenges facing European welfare states; be that increasing costs, decreasing tax revenues, or multi-morbidities associated with ageing populations (Juma & Yee-Cheong, Citation2005; Piot, Citation2012; World Health Organization, Citation1997). However, it is unclear how such technologies affect population health, and whether they contribute to or if they buffer against the health gap between vulnerable and resourceful groups in society. A much-cited example is McKeown’s (Citation1980) thesis of how increases in population health and improved quality of life are not results of advances within medicine, but rather within broader social and economic conditions. A seminal theory in the health inequality field, the fundamental cause theory, focus on social conditions as the basic causes of health, and suggests that technological innovations could be one of the mechanisms that generate health inequalities (Link & Phelan, Citation2002). A related model is that of hierarchical diffusion. Inspired by Rogers’ (Citation2003) work on the diffusion of innovation, the model suggests that innovative technology and new knowledge is diffused unequally across the social strata (Elstad, Citation2013).
Cardiovascular disease (CVD) has been the leading cause of death in Norway in the years 1990-2016, while also having the highest absolute educational inequalities in mortality through all decades since the 1970s (Institute for Health Metrics and Evaluation, Citation2018; Strand et al., Citation2014). Hypertension has been the leading risk factor of CVD and a pivotal component in these inequalities (Institute for Health Metrics and Evaluation, Citation2018; Strand & Tverdal, Citation2004).
While the generally positive effect of drug treatment on hypertension has been demonstrated over the last decades, consequences for inequalities remains unclear (Holmen et al., Citation2016). The greater backdrop for this article is therefore whether the reported social inequalities in cardiovascular disease are affected by medical innovations, specifically how the diffusion of blood pressure (BP) medication affects BP inequalities. I seek to (1) examine social inequalities in BP levels; (2) analyse the impact from medication use on these inequalities; and subsequently (3) discuss the mechanisms connecting medication use with health inequalities. In the following, I will present relevant concepts and research on the role of technology in social health inequalities, before presenting some selected research on social inequalities in BP. In the results section, regression analyses of panel data indicate that medication may have a levelling effect on BP, contributing to a reduction in social inequalities. The late stage of diffusion, reduced marginal gains of drug adoption, and unequal treatment by medical personnel are some of the explanations which are discussed.
Background
In 1995, Link and Phelan introduced the theory of the fundamental causes of social inequalities in health (FCT). Here, they introduce two vital concepts: ‘contextualizing risk factors’ and ‘fundamental causes’ (Link & Phelan, Citation1995, p. 84). The latter are the general forces of social inequality in a society, located upstream in the causal chain leading to health inequalities, while the former is the process of analysing the social mechanisms connecting more proximate risk factors to disease (Link & Phelan, Citation1995). Examples of proximate risk factors could be working and living conditions; diet, exercise and other health behaviours; access to and utilisation of health services; or the diffusion and adoption of technological innovations (Phelan, Link, & Tehranifar, Citation2010; Phelan, Link, Diez-Roux, Kawachi, & Levin, Citation2004). When our ability to control disease increase in a stratified society, health disparities grow; these disparities will also be greater for health problems where potential preventability is high (Phelan et al., Citation2004; Phelan & Link, Citation2005).
The theory has therefore been relevant in describing and explaining the paradoxical persistence of health inequalities in advanced welfare states; the flexible resources associated with high socioeconomic status (SES), such as money, knowledge, power, prestige, and social networks, have produced inequalities also in settings with a high degree of equality in working and living conditions (Mackenbach, Citation2012). Medical technology and new knowledge can here function as inequality-generating mechanisms, by reproducing the unequal distribution of resources into social inequalities in health outcomes. Innovations can thus both ‘modulate the pathways of social influence and also create social influence for conditions traditionally insensitive to social factors’ (Gortmaker & Wise, Citation1997, p. 157). An empirical test of this aspect of the FCT has been to investigate health inequalities in conditions which innovations have increased our ability to prevent and control. Here, social gradients have been demonstrated in preventive practices like Pap smears and mammography (Link, Northridge, Phelan, & Ganz, Citation1998; Zapata-Moya, Willems, & Bracke, Citation2019); the effect of the complex HIV treatment HAART (Goldman & Lakdawalla, Citation2005); the survival rates from diseases associated with high degrees of innovation (Glied & Lleras-Muney, Citation2008); the introduction of statin drugs in glucose treatment (Chang & Lauderdale, Citation2009); and the diffusion of information and colorectal cancer (Wang, Clouston, Rubin, Colen, & Link, Citation2012).
It has been argued that the FCT functions well as a general framework or paradigm, but that there is a need for specifying more concrete, intervening mechanisms, as well as explanations of why some flexible resources return greater health benefits in particular contexts (Mackenbach, Citation2012; Masters, Link, & Phelan, Citation2015; Baker et al., Citation2017). The diffusion of innovative technology or information could function as a specification of the FCT. Here, socioeconomic resources influence health with unequal power and through various pathways over the course of a diffusion process.
Rogers’ (Citation2003) diffusion of innovation theory proposes that health-beneficial practices first start high in the socioeconomic hierarchy, before ‘trickling down’ through social networks, social modelling, or acts of social distinction, which in turn generates socioeconomic lags in use, access, and benefits from health innovations (Elstad, Citation2013; Lindbladh, Lyttkens, Hanson, & Östergren, Citation1997; Rogers, Citation2003). Determinants of hierarchical diffusion can be individual characteristics associated with SES, as well as variables related to the social system and to the innovation itself. In systems characterised by a high degree of inequality and social distance, medical innovations may diffuse at a slower pace across socioeconomic groups, leading to widening inequalities in health outcomes. Further could innovation attributes, such as its relative advantage, compatibility, and complexity, also affect the adoption rate and subsequently outcome inequalities (Goldman & Lakdawalla, Citation2005; Rogers, Citation2003).
Examples of socioeconomic lags can be found for new information on cigarette smoking, nutrition, and HIV (Roberts et al., 2017); innovative coronary surgical procedures (Korda, Clements, & Dixon, Citation2011); and innovations in public health policies like vaccines, vitamin supplementation, and skilled birth delivery in health facilities (Victora et al., Citation2018; Victora, Fenn, Bryce, & Kirkwood, Citation2005; Victora, Vaughan, Barros, Silva, & Tomasi, Citation2000). These findings suggest that the introduction of health-beneficial innovations may be followed by first widening, then possibly contracting, inequalities (Cutler, Deaton, & Lleras-Muney, Citation2006; Rogers, Citation2003). The diffusion mechanism thus offers a specific mechanism and a contextual dimension to the FCT, increasing or decreasing the effect of socioeconomic resources on health at different time points. For example, Zapata-Moya et al. (Citation2019) have demonstrated how SES show strong associations with preventive health measures in an early diffusion stage, and weaker associations with less innovative measures.
Norway is a technologically advanced country with a comprehensive and universal welfare state and health care system, which nevertheless have shown and continue to show a substantial health gradient across numerous measures (Strand & Madsen, Citation2018). A wide array of explanations has been proposed for this so-called paradox – among them are mathematical artefacts, social mobility, and new forms of social stratification and marginalisation (Huijts & Eikemo, Citation2009; Mackenbach, Citation2017). Could the unequal diffusion of innovative technology contribute to these explanations? Previous research has demonstrated that health-beneficial innovations and behaviour tend to follow a social-hierarchical diffusion pattern, first adopted by high-SES groups before reaching the lower social strata later in the diffusion process. This unequal diffusion of innovations may subsequently lead to health inequalities within and between populations; for example, information on health-beneficial behaviour was diffused to the Nordic European countries earlier than the Southern European countries, with larger inequalities in lifestyle-related ‘diseases of affluence’ among the former as a result (Mackenbach, Citation2012).
Study context
Both the international WHO MONICA study and the Norwegian HUNT and Tromsø studies have shown overall declines in BP levels from the mid-1980s to the mid-2000 among both men and women, which is only partly explained by increasing use and effect of medication (Holmen et al., Citation2016; Hopstock et al., Citation2015; Tunstall-Pedoe, Connaghan, Woodward, Tolonen, & Kuulasmaa, Citation2006).
BP medication appears to be a medical innovation far along in a diffusion process. Both in Norway and internationally, drug treatment of hypertension has steadily increased since the first hypertension-combating agents became available in the 1950s (Gavras, Citation2001; Gu, Burt, Dillon, & Yoon, Citation2012; Meland, Citation2009). In Norway, antihypertension drugs are prescribed by one’s general practitioner (GP), and the pharmaceutical sector is strongly regulated. Drug payments are to a large degree reimbursed, co-payments are capped, and disadvantaged groups are exempted from out-of-pocket payment (Ringard, Sagan, Saunes, & Lindahl, Citation2013). Descriptive analyses of HUNT data showed an increase in BP medication use from 12.9% of all respondents in 1984 to 20.6% in 2008, while figures from the Norwegian Institute of Public Health’s (Citation2018) prescription database show an increase from 141 to 168 users per 1,000 inhabitants in the period 2004–2017.
Meta-analyses and review studies have demonstrated this a substantial social gradient in blood pressure across various contexts and measures, also in studies dating back to 1966 (Colhoun, Hemingway, & Poulter, Citation1998; Leng, Jin, Li, Chen, & Jin, Citation2015). Comparisons of self-reports and clinical measures of hypertension have showed an underestimation of socioeconomic inequality when relying on the former (Johnston, Propper, & Shields, Citation2009; Mosca, Bhuachalla, & Kenny, Citation2013). In Norway, Strand and Tverdal (Citation2006) and Ernstsen, Strand, Nilsen, Espnes, and Krokstad (Citation2012) have demonstrated persisting relative inequalities in blood pressure and hypertension from the 1970s to the 2000s.
There may be several mechanisms connecting SES, medication, and BP outcomes. First, low SES has persistently been associated with other lifestyle-related cardiovascular risk factors like BMI, smoking, and cholesterol (Strand & Tverdal, Citation2006). This could contribute to social inequalities in BP prior to medical consulting and prospective drug prescriptions. Second, as medication is dependent on GP prescription, there may be a selection effect: Individuals with high BP may be more likely to have medication prescribed. These two challenges are met by including control variables related to lifestyle and other relevant biomedical factors, and by using fixed effects regression to avoid confounding.
Third, although the Norwegian healthcare system is built on universalistic principles of equal access regardless of social or economic resources and geographic location, research has shown a substantial social gradient in health care utilisation in Norway (Vikum, Bjørngaard, Westin, & Krokstad, Citation2013; Vikum, Krokstad, & Westin, Citation2012). High BP among high-SES groups may thus have a higher probability of being treated.
Lastly, hypertension and BP medication may not be associated with the same degree of innovativeness as the studies referred above. It has been suggested that diffusion processes reach a threshold where the uptake of an innovation is saturated among people of high SES and increasing among people of low SES, leading in turn to reduced health inequalities (Clouston, Rubin, Phelan, & Link, Citation2016). Hypertension may have reached a point in the demographic and epidemiological transition stage where inequalities are starting to decline, possibly due to the increased utilisation of medication among people of lower SES.
Following the FCT, health inequalities is a more or less inevitable consequence of social stratification; if one pathway between social position and health is closed, another emerges. Research on hierarchical diffusion have suggested that inequalities in use, access, and outcome decrease over time as a medical innovation is diffused. In the Norwegian case, BP inequalities have persisted, while BP medication is a highly regulated technology in a universal health care system, presumably late in its diffusion process. Initial bivariate analyses showed few signs of a traditional social gradient in medication use among hypertensive respondents; there were no significant differences between income and education groups in the probability of using BP medication. It is therefore less likely that inequalities in BP are primarily caused by inequalities in access to or use of BP medication, i.e. that technological innovation is a mediating mechanism in this setting.
This article asks whether the contribution from antihypertensives to BP inequalities rather has been as a moderating mechanism, i.e. whether the effect of medication use differs for people of low and high SES. Such ‘differential susceptibility’ is often operationalised as an interaction term, and has traditionally been underexplored (Mackenbach & de Jong, Citation2018). Additionally, if vulnerability to specific health determinants differ by social position, there are implications for policy. Whereas mediation analyses focus on how health determinants (e.g. lifestyle traits, health care, and medical innovations) are unequally distributed, thus suggesting redistribution of determinants to prevent health inequalities, moderation rather points toward more targeted interventions to combat differential vulnerability by SES (Mackenbach & de Jong, Citation2018). If medication use has a mediating effect on health inequalities, a policy response would be to secure equal access. If medication is a moderating mechanism, e.g. that the effect of BP medication is weaker for people of low SES, a policy response would be to strengthen the resilience of these disadvantaged groups, for instance by providing targeted education on how and when BP medication is most efficiently used.
Social inequalities in BP among Norwegian men and women have been thoroughly demonstrated. Figures from the HUNT studies indicate that these inequalities are not likely to be explained by unequal use of medication, while features of the Norwegian health care system suggest that they are not likely explained by inequalities in access. I, therefore, hypothesise whether inequality in effect, that high-SES patients benefit more, could contribute to explaining the social gradient in BP.
Data and methods
The Nord-Trøndelag Health Study (HUNT) is a population-based study in three waves: HUNT1 (1984–1986), HUNT2 (1995–1997), and HUNT3 (2006–2008). The study contains information from approximately 120,000 individuals, and 27,605 individuals participated in all three surveys. Participation rates ranged from 59% to 88% of invited respondents. Nord-Trøndelag County is fairly representative of Norway as a whole in terms of geography, economy, morbidity, and mortality (Holmen et al., Citation2003). Respondents completed two questionnaires at home before and after attending a clinical health exam. In this study, HUNT data is linked with the income and education databases from Statistics Norway (SSB) through a Norwegian personal identification number. The project was approved by the Regional Committee for Medical and Health Research Ethics in Central Norway (REC Central). Statistics software STATA 15 was used to analyse the data material.
Variables
Dependent variables were systolic and diastolic BP, measured manually by professionally trained nurses in HUNT1 and assisted with automatic measures in HUNT2 and HUNT3. As is standard in medical literature, an average of two readings was used to minimise the risk of measurement error. Two variables for SES were included: Education was measured in three levels – primary, secondary, and tertiary; and respondents’ yearly income in 1986, 1997, and 2008 was divided into quartile groups. A large number of respondents was registered with 0 as yearly income. Potential consequences of this distribution are treated in the limitations chapter. By example of Holmen et al. (Citation2016), indicators of a healthy lifestyle and biomedical variables proved to have an association with high BP were added as controls: Age, BMI; former, current, or occasional smoking; weekly physical activity; self-reported diabetes or myocardial infarction; and heart rate. Glucose and cholesterol levels are also known predictors of BP, but these measurements were not comparable across all three HUNT surveys. A survey wave dummy was included in all models. provides descriptive statistics of included variables.
Table 1. Descriptive statistics.
Methods
The HUNT data was analysed using individual fixed effects (FE) regression, where it is possible to control for time-invariant unmeasured variables correlating with one or more of the independent variables (Petersen, Citation2004). This approach analyses within-unit variation, reducing possible selection bias. As proposed by Mummolo and Peterson (Citation2018), the outcome scale has been adjusted to reflect within-unit variation.
SES explanatory variables, the medication use variable, and control variables were added separately to the model. Interaction terms, where the SES variables were multiplicated with the medication variable, were constructed. For readability are only estimates for SES and medication variables displayed in the final models (); results are further displayed as predicted BP levels ( and ). In addition, linear probability models were estimated with high BP (SBP/DBP>140/90) as defined by the European societies of hypertension and cardiology (Mancia et al., Citation2014) as the dependent variable. Results from this are presented in and as marginal predicted probabilities.
Figure 1. Marginal predictions, male population. Note: Based on regression models from . Spikes indicate 95% confidence intervals.
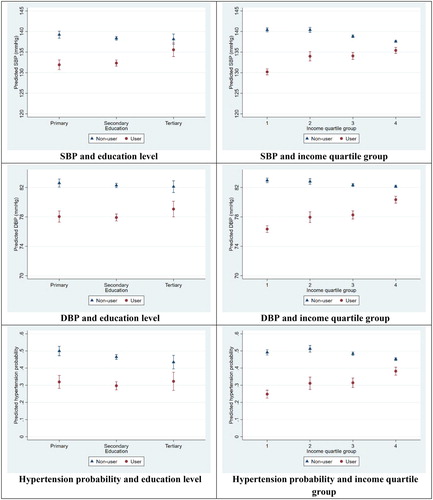
Figure 2. Marginal predictions, female population. Note: Based on regression models from . Spikes indicate 95% confidence intervals.
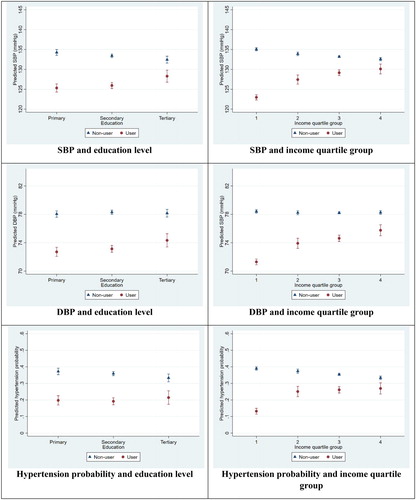
Table 2. Regression models.
Analyses were stratified by gender, as I expected time-varying gender differences for several relevant factors, such as health, social mobility, and health care utilisation (cf. Suominen-Taipale, Martelin, Koskinen, Holmen, & Johnsen, Citation2006). Descriptive statistics showed that the male population had a lower portion of 0-income respondents, overall greater income variance, and a higher mean income over all three survey waves, while there was a higher prevalence of medication users among high-BP women. Gender could not be added to the regression models as a control variable due to a preference for fixed effects models. Stratified analysis was therefore considered the most suited option.
Results
Control variables are only briefly reported; these estimates change little across models and were not connected to any central hypotheses. BMI, exercise, and heart rate were positively associated with both BP measures; self-reported diabetes and myocardial infarction were negatively associated; smoking had no significant associations with neither BP measure. Systolic BP decreased and diastolic BP increased with age, but the squared age variable showed that the age effect diminished as the respondents grow older.
Use of medication was significantly associated with lower BP. Advances in educational attainment was associated with lowered systolic BP; this association was weakened but still significant after adding control variables. Low levels of income were significantly associated with higher levels of systolic BP among both men and women but with modest effect sizes: changes between quartile groups predicted <3 mmHg change in BP after controls were added (). Similar patterns of significant, but modest effects could be observed for the linear probability models using a dichotomous hypertension variable: Low SES was associated with 2–6 percentage points higher risk of hypertension across all models. The SES patterns were largely similar among men and women, but with higher predicted BP levels and hypertension probabilities among men.
The interaction variables were significantly negative for both men and women across both measures of SES and BP, as well as in the linear probability models, before and after including control variables. This means that the negative effects of adopting medication were stronger for people of lower SES. Having low education and starting on medication predicted a 7.3 mmHg decrease in systolic BP among men, while the decrease for the high-educated was 2.6 mmHg (, top left panel). The corresponding estimates for low- and high-educated women were 9.0 and 4.2 mmHg (, top left panel). Low-income men starting on medication were estimated to have a 10.2 mmHg decrease in systolic BP, while for high-income men, the effect was a 2.2 mmHg decrease (, top right panel). Low- and high-income women were predicted to experience respectively a 12.1 and 2.5 mmHg decrease (, top right panel). Predicted systolic and diastolic BP levels are represented on the Y-axes, with SES categories represented on the X-axes. Most panels display an evident social gradient for the non-users, and that the user vs. non-user gaps in BP are larger for the lowest SES groups. Patterns are similar in the linear probability models, with predicted probabilities for hypertension ranging between 13% and 51% across all panels. Two examples: Adopting medication reduced the risk of hypertension by approximately 24 percentage points (from 49 to 25%) for a man with low income, and 7 percentage points for a man with high income (from 45 to 38%). For low- and high-educated women, the corresponding estimates were 18 and 11 percentage points ( and , bottom panels).
The within-R2, the explained variance within each unit, significantly increased for each expansion of the model. The final models displayed in explained between 12% and 19% of male and female systolic BP, and between 19% and 25% of male and female diastolic BP. The corresponding LPM models explained between 4% and 7% of the risk of high blood pressure.
Limitations
In the registry data, a large share of individuals was listed with an income of 0. Reasons for this may be diverse. They may have had no actual income that year, or they may have received certain kinds of private income and/or public benefits exempted from taxation. Value 0 on the income variable could thus include people with a higher actual income, ‘disguising’ a higher SES and further leading to incorrect estimations. Sensitivity regression analysis was performed without the 0-income group. Some of the associations between the lowest income quartile and diastolic BP was altered or lost statistical significance by this; effects of education remained similar. Cross tabulations showed that the 0-income group was diverse, but with an overweight of low educated, and people belonging to the youngest and oldest age groups. I argue that the 0-income group is a valid representation of low SES and should therefore be included in the analyses.
The main limitation with regards to data quality concerns the response rates; they fall from 89% in HUNT1 to 54% in HUNT3. Analyses of non-respondents have shown that between-wave drop-out was associated with male gender, young age, cardiovascular disease, diabetes mellitus, psychiatric disorders, lower socioeconomic status, and higher mortality (Krokstad et al., Citation2012). This could suggest a health selection bias; respondents participating in more than one study wave may be in better health and of higher SES, which potentially could contribute to an underestimation of inequalities. Additionally, we cannot know the causal direction for respondents experiencing both upward social mobility and improved health – e.g. whether attaining additional education led to reduced BP or the other way around. Measures to control for this limitation, such as lagged SES variables or only including BP from HUNT3, is difficult due to the short data structure. Some uncertainty concerning the direction of causality therefore remains.
Discussion
Using educational level and income quartile as socioeconomic indicators, inequalities are observed, particularly in systolic BP. However, and contrary to expectations, when interaction terms between SES and medication use were included, the adoption of BP medication had a stronger, negative effect on blood pressure for people of low SES. The discussion chapter will be structured around two findings: (1) the socioeconomic inequalities in BP levels; (2) the possibly levelling effect of medication on socioeconomic inequalities in BP.
Socioeconomic inequalities in BP levels
Following the FCT, the observed social inequalities in BP may follow from social inequalities in flexible resources such as money, knowledge, power, prestige, and social connections.
SES was negatively associated with medication use among respondents with high BP, and negatively associated with BP among respondents not using medication. This could indicate that people of high SES treat their blood pressure, but with other measures than prescription drugs. One potential inequality-generating mechanism could therefore be unequal use of a medical innovation that is at an earlier point in the diffusion process than BP medication, for instance BP self-measuring technology. Another could be located within the health care institutions; people of high SES in general utilise primary and specialist health services more often and receive longer consultations, thus are they in better chances of having high BP levels discovered and receiving early treatment, possibly by other means than drugs (Brekke, Holmås, Monstad, & Straume, Citation2018; Vikum et al., Citation2012; Vikum et al., Citation2013). Could physicians also be more inclined to advice non-drug treatment to people of high SES? Research have suggested that physicians’ expectations coming into patient-provider interactions may be influenced by the patients’ SES; inequalities in treatment and health outcomes may follow (Hernandez, Citation2013; Shim, Citation2010). This also resonates with previous findings indicating a steeper social gradient for treatments demanding more health agency from the individual patient, and that high SES is associated with greater adherence to medical advice and to change one’s behaviour following a hypertension diagnosis (DiMatteo, Citation2004; Goldman & Lakdawalla, Citation2005; Hernandez, Margolis, & Hummer, Citation2018). These findings suggest that the structural and individual resources available to people of high SES are associated with a willingness and ability to adhere to medical advice, which could be further reflected in physicians’ practices.
Finally, the control variables adjust for inequalities in diet, smoking, exercise, and more. SES was significantly associated with BP also after the controls were included. In the models, BMI is chosen as a measure of dietary traits. However, BMI may not be a precise measurement of more fine-grained dietary differences possibly affecting inequalities in BP levels, such as the intake of fat, alcohol, or salt (Holmen et al., Citation2016).
Levelling effect
Medication use had a significantly stronger effect on BP for low-SES respondents. Innovative medical technology was initially hypothesised as a potential driver of health inequalities, a mechanism connecting inequalities in resources with inequalities in health outcomes. Overall, these findings indicated that the use and utilisation of medication does not fit with this description. However, they suggest support for aspects of the hierarchical diffusion model; BP medication may have reached a late point in its diffusion process, where all groups appear to benefit. But in addition, lower SES groups appear to benefit more, indicating saturation as described by Clouston et al. (Citation2016), where high-SES respondents have less room for BP reduction by adopting medication. Technology may decrease the marginal value of resources and thus benefit low-SES groups more (Chang & Lauderdale, Citation2009). Subsequently, if high SES is associated with treating high BP with both drug and non-drug measures, the marginal effect of medication use may in this case be lower.
Similarly, people of low SES may be in a condition more responsive to treatment, whereas hypertension among high-SES respondents, plausibly practicing healthier lifestyles, may be more refractory. Lastly, health service utilisation could also contribute to explain these results; if people of low SES use health care services less, plausibly waiting longer before entering treatment, it could result in the effect of drug treatment being relatively stronger.
Further, the substantial effect of medication on BP for people of low SES could be a sign of overmedication, leading to hypotension – dangerously low BP levels. Most predicted values were within both upper and lower thresholds of normalcy, but hypotension can nevertheless be a side effect of medication, particularly among elders (Divisón-Garrote et al., Citation2016). Finally, the apparent levelling of BP levels could hide other patterns. The analyses did not distinguish between types of medication – they may differ in e.g. effectiveness, dosage, and potential side effects. Future research may benefit from operationalising these aspects of medication and exploring their associations with SES.
Lastly, since adopting medication had a stronger effect on BP for low-SES respondents, these individuals were also more sensitive to e.g. varying availability of sufficient health care, or differencing practices in medication prescription. In a universal health care system like the Norwegian, unequal access to medication should not be a cause of social inequalities. Nevertheless, the differential vulnerability of BP medication indicates that the strongest predictor of hypertension is the double marginalised position of low SES and no medication. From this arises the question whether there are mechanisms located within the health care system which generate inequalities between low-SES subgroups. Are there inherent barriers to receiving drug treatment that certain resources – not perfectly correlated with education or income – enable you to traverse?
Conclusions
High BP is an example of a condition that in the medical sociology literature can be termed as a disease – diagnosed by an expert or medical professional, rather than an illness – the ill health a person identifies with, or a sickness – the social role of a sick person (Wikman, Marklund, & Alexanderson, Citation2005). Hypertension may thus have little direct effect on daily life and activities, but nevertheless be an important risk factor of more severe cardiovascular diseases. The underlying mechanisms can also be indicative in explaining inequalities in other health outcomes conditions where the diffusion of medical innovations is a relevant context. A main finding in this study is that although there are social inequalities in BP levels, use of BP medication contributes to a levelling of these differences. One explanation of this levelling effect could be that this medication is at a late stage of its diffusion, being equally accessed and used by all social strata. Additionally, a combination of drug and non-drug treatment could make the marginal gain for high-SES groups small.
The FCT has struggled to explain how the same flexible resources and mechanisms can have different associations with health outcomes in different contexts (Masters et al., Citation2015). Conjoining the FCT with aspects of diffusion theory has been proposed as a response to this challenge. The present study suggests that this approach may be beneficial. The context has been an innovation late in its diffusion process, and results indicate that adopting such an innovation may contribute to a contraction of health inequalities. However, if the effect of adopting medication is stronger for those with low SES, this could also indicate that they to a lesser degree deploy their resources towards other BP-beneficial measures, and subsequently are more vulnerable to retrenchments and cut-backs. Some resources appear to be more relevant for explaining how people of low SES gets access to and better utilise a medical innovation in this particular setting – a universal health care system and an innovative technology late in its diffusion process. Future research may benefit from investigating the organisational and institutional obstacles and pathways through which people of low SES gain access to medical innovations and technology in a universal health care system.
Acknowledgements
This research received funding from the strategic research area NTNU Health in 2016 at NTNU, Norwegian University of Science and Technology.
Disclosure statement
No potential conflict of interest was reported by the author(s).
References
- Baker, D. P., Smith, W. C., Muñoz, I. G., Jeon, H., Fu, T., Leon, J., … Horvatek, R. (2017). The population education transition curve: Education gradients across population exposure to new health risks. Demography, 54(5), 1873–1895. doi: 10.1007/s13524-017-0608-9
- Brekke, K. R., Holmås, T. H., Monstad, K., & Straume, O. R. (2018). Socio-economic status and physicians’ treatment decisions. Health Economics, 27(3), 77–89. doi: 10.1002/hec.3621
- Chang, V. W., & Lauderdale, D. S. (2009). Fundamental cause theory, technological innovation, and health disparities: The case of cholesterol in the era of statins. Journal of Health and Social Behavior, 50(3), 245–260. doi: 10.1177/002214650905000301
- Clouston, S. A. P., Rubin, M. S., Phelan, J. C., & Link, B. G. (2016). A social history of disease: Contextualizing the rise and fall of social inequalities in cause-specific mortality. Demography, 53(5), 1631–1656. doi: 10.1007/s13524-016-0495-5
- Colhoun, H. M., Hemingway, H., & Poulter, N. R. (1998). Socio-economic status and blood pressure: An overview analysis. Journal of Human Hypertension, 12(2), 91. doi: 10.1038/sj.jhh.1000558
- Cutler, D. M., Deaton, A., & Lleras-Muney, A. (2006). The determinants of mortality. Journal of Economic Perspectives, 20(3), 97–120. doi: 10.1257/jep.20.3.97
- DiMatteo, M. R. (2004). Variations in patients’ adherence to medical recommendations: A quantitative review of 50 years of research. Medical Care, 5(3), 200–209. doi: 10.1097/01.mlr.0000114908.90348.f9
- Divisón-Garrote, J. A., Banegas, J. R., Juan, J., Escobar-Cervantes, C., De la Sierra, A., Gorostidi, M., … Ruilope, L. M. (2016). Hypotension based on office and ambulatory monitoring blood pressure. Prevalence and clinical profile among a cohort of 70,997 treated hypertensives. Journal of the American Society of Hypertension, 10(9), 714–723. doi: 10.1016/j.jash.2016.06.035
- Elstad, J. I. (2013). The hierarchical diffusion model and the changing patterns in health-related habits in Norway since the 1970s. Research review of social inequalities in health in Norway.
- Ernstsen, L., Strand, B. H., Nilsen, S. M., Espnes, G. A., & Krokstad, S. (2012). Trends in absolute and relative educational inequalities in four modifiable ischaemic heart disease risk factors: Repeated cross-sectional surveys from the Nord-Trøndelag health study (HUNT) 1984–2008. BMC Public Health, 12(1), 266. doi: 10.1186/1471-2458-12-266
- Gavras, H. P. (2001). Issues in hypertension: Drug tolerability and special populations. American Journal of Hypertension, 14(4), 231–236. doi: 10.1016/S0895-7061(01)02132-X
- Glied, S., & Lleras-Muney, A. (2008). Technological innovation and inequality in health. Demography, 45(3), 741–761. doi: 10.1353/dem.0.0017
- Goldman, D. P., & Lakdawalla, D. N. (2005). A theory of health disparities and medical technology. Contributions in Economic Analysis & Policy, 4(1), 1–30. doi: 10.2202/1538-0645.1395
- Gortmaker, S. L., & Wise, P. H. (1997). The first Injustice: Socioeconomic disparities, health services technology, and infant mortality. Annual Review of Sociology, 23(1), 147–170. doi: 10.1146/annurev.soc.23.1.147
- Gu, Q., Burt, V. L., Dillon, C. F., & Yoon, S. (2012). Trends in antihypertensive medication use and blood pressure control among United States adults with hypertension: The national health and nutrition examination survey, 2001 to 2010. Circulation, 126(17), 2105–2114. doi: 10.1161/CIRCULATIONAHA.112.096156
- Hernandez, E. M. (2013). Provider and patient influences on the formation of socioeconomic health behavior disparities among pregnant women. Social Science & Medicine, 82, 35–42. doi: 10.1016/j.socscimed.2013.01.018
- Hernandez, E. M., Margolis, R., & Hummer, R. A. (2018). Educational and gender differences in health behavior changes after a gateway diagnosis. Journal of Aging and Health, 30(3), 342–364. doi: 10.1177/0898264316678756
- Holmen, J., Holmen, T. L., Tverdal, A., Holmen, O. L., Sund, E. R., & Midthjell, K. (2016). Blood pressure changes during 22-year of follow-up in large general population – the HUNT study, Norway. BMC Cardiovascular Disorders, 16(1), 1–9. doi: 10.1186/s12872-016-0257-8
- Holmen, J., Midthjell, K., Krüger, Ø, Langhammer, A., Holmen, T. L., Bratberg, G. H., … Lund-Larsen, P. G. (2003). The Nord-Trøndelag health study 1995–97 (HUNT 2): Objectives, contents, methods and participation. Norsk epidemiologi, 13(1), 19–32.
- Hopstock, L. A., Bønaa, K. H., Eggen, A. E., Grimsgaard, S., Jacobsen, B. K., Løchen, M.-L., … Wilsgaard, T. (2015). Longitudinal and secular trends in blood pressure among women and men in birth cohorts born between 1905 and 1977. Hypertension, 66(3), 496–501. doi: 10.1161/HYPERTENSIONAHA.115.05925
- Huijts, T., & Eikemo, T. A. (2009). Causality, social selectivity or artefacts? Why socioeconomic inequalities in health are not smallest in the Nordic countries. European Journal of Public Health, 19(5), 452–453. doi: 10.1093/eurpub/ckp103
- Institute for Health Metrics and Evaluation. (2018). Country profiles: Norway. Retrieved from https://vizhub.healthdata.org/gbd-compare/
- Johnston, D. W., Propper, C., & Shields, M. A. (2009). Comparing subjective and objective measures of health: Evidence from hypertension for the income/health gradient. Journal of Health Economics, 28(3), 540–552. doi: 10.1016/j.jhealeco.2009.02.010
- Juma, C., & Yee-Cheong, L. (2005). Reinventing global health: The role of science, technology, and innovation. The Lancet, 365(9464), 1105–1107. doi: 10.1016/S0140-6736(05)71147-8
- Korda, R. J., Clements, M. S., & Dixon, J. (2011). Socioeconomic inequalities in the diffusion of health technology: Uptake of coronary procedures as an example. Social Science & Medicine, 72(2), 224–229. doi: 10.1016/j.socscimed.2010.11.002
- Krokstad, S., Langhammer, A., Hveem, K., Holmen, T. L., Midthjell, K., Stene, T. R., & Holmen, J. (2012). Cohort profile: The HUNT study, Norway. International Journal of Epidemiology, 42(4), 968–977. doi: 10.1093/ije/dys095
- Leng, B., Jin, Y., Li, G., Chen, L., & Jin, N. (2015). Socioeconomic status and hypertension: A meta-analysis. Journal of Hypertension, 33(2), 221–229. doi: 10.1097/HJH.0000000000000428
- Lindbladh, E., Lyttkens, C. H., Hanson, B. S., & Östergren, P.-O. (1997). The diffusion model and the social-hierarchical process of change. Health Promotion International, 12(4), 323–330. doi: 10.1093/heapro/12.4.323
- Link, B. G., Northridge, M. E., Phelan, J. C., & Ganz, M. L. (1998). Social epidemiology and the fundamental cause concept: On the structuring of effective cancer screens by socioeconomic status. Milbank Quarterly, 76(3), 375–402. doi: 10.1111/1468-0009.00096
- Link, B. G., & Phelan, J. (1995). Social conditions as fundamental causes of disease. Journal of Health and Social Behavior, 35, 80–94. doi: 10.2307/2626958
- Link, B. G., & Phelan, J. C. (2002). Mckeown and the idea that social conditions are fundamental causes of disease. American Journal of Public Health, 92(5), 730–732. doi: 10.2105/AJPH.92.5.730
- Mackenbach, J. P. (2012). The persistence of health inequalities in modern welfare states: The explanation of a paradox. Social Science & Medicine, 75(4), 761–769. doi: 10.1016/j.socscimed.2012.02.031
- Mackenbach, J. P. (2017). Nordic paradox, southern miracle, eastern disaster: Persistence of inequalities in mortality in Europe. European Journal of Public Health, 27(suppl_4), 14–17. doi: 10.1093/eurpub/ckx160
- Mackenbach, J. P., & de Jong, J. P. (2018). Health inequalities: An interdisciplinary exploration of socioeconomic position, health and causality. FEAM/ALLEA Committee on Health Inequalities.
- Mancia, G., Fagard, R., Narkiewicz, K., Redon, J., Zanchetti, A., Böhm, M., … Zannad, F. (2014). 2013 ESH/ESC practice guidelines for the management of arterial hypertension. Blood Pressure, 23(1), 3–16. doi: 10.3109/08037051.2014.868629
- Masters, R. K., Link, B. G., & Phelan, J. C. (2015). Trends in education gradients of ‘preventable’ mortality: A test of fundamental cause theory. Social Science & Medicine, 127, 19–28. doi: 10.1016/j.socscimed.2014.10.023
- McKeown, T. (1980). The role of medicine: Dream, mirage, or nemesis? Princeton, NJ: Princeton University Press.
- Meland, E. (2009). Tiazider – et førstevalg mot høyt blodtrykk. Tidsskriftet Den Norske Legeforening, 129(18), 1876–1877. doi: 10.4045/tidsskr.09.0172
- Mosca, I., Bhuachalla, B. N., & Kenny, R. A. (2013). Explaining significant differences in subjective and objective measures of cardiovascular health: Evidence for the socioeconomic gradient in a population-based study. BMC Cardiovascular Disorders, 13(1), 64. doi: 10.1186/1471-2261-13-64
- Mummolo, J., & Peterson, E. (2018). Improving the interpretation of fixed effects regression results. Political Science Research and Methods, 6(4), 829–835. doi: 10.1017/psrm.2017.44
- Norwegian Institute of Public Health. (2018). Norwegian prescription database. Retrieved from http://www.reseptregisteret.no/
- Petersen, T. (2004). Analyzing panel data: Fixed-and random-effects models. In M. Hardy & A. Bryman (Eds.), Handbook of data analysis (pp. 331–345). London: Sage.
- Phelan, J. C., & Link, B. G. (2005). Controlling disease and creating disparities: A fundamental cause perspective. The Journals of Gerontology Series B: Psychological Sciences and Social Sciences, 60(Special Issue 2), S27–S33. doi: 10.1093/geronb/60.Special_Issue_2.S27
- Phelan, J. C., Link, B. G., Diez-Roux, A., Kawachi, I., & Levin, B. (2004). ‘Fundamental causes’ of social inequalities in mortality: A test of the theory. Journal of Health and Social Behavior, 45(3), 265–285. doi: 10.1177/002214650404500303
- Phelan, J. C., Link, B. G., & Tehranifar, P. (2010). Social conditions as fundamental causes of health inequalities: Theory, evidence, and policy implications. Journal of Health and Social Behavior, 51(1_suppl), S28–S40. doi: 10.1177/0022146510383498
- Piot, P. (2012). Innovation and technology for global public health. Global Public Health, 7(Suppl. 1), S46–S53. doi: 10.1080/17441692.2012.698294
- Ringard, Å, Sagan, A., Saunes, I. S., & Lindahl, A. (2013). Norway: Health system review. World Health Organization. Regional Office for Europe; WHO IRIS.
- Rogers, E. M. (2003). Diffusion of innovations (5th ed.). New York, NY: Free Press.
- Shim, J. K. (2010). Cultural health capital: A theoretical approach to understanding health care interactions and the dynamics of unequal treatment. Journal of Health and Social Behavior, 51(1), 1–15. doi: 10.1177/0022146509361185
- Strand, B. H., & Madsen, C. (2018). Social inequalities in health. Oslo: Norwegian Institute of Public Health.
- Strand, B. H., Steingrímsdóttir, ÓA, Grøholt, E.-K., Ariansen, I., Graff-Iversen, S., & Næss, Ø. (2014). Trends in educational inequalities in cause specific mortality in Norway from 1960 to 2010: A turning point for educational inequalities in cause specific mortality of Norwegian men after the millennium? BMC Public Health, 14(1), 1208. doi: 10.1186/1471-2458-14-1208
- Strand, B. H., & Tverdal, A. (2004). Can cardiovascular risk factors and lifestyle explain the educational inequalities in mortality from ischaemic heart disease and from other heart diseases? 26 year follow up of 50 000 Norwegian men and women. Journal of Epidemiology & Community Health, 58(8), 705–709. doi: 10.1136/jech.2003.014563
- Strand, B. H., & Tverdal, A. (2006). Trends in educational inequalities in cardiovascular risk factors: A longitudinal study among 48,000 middle-aged Norwegian men and women. European Journal of Epidemiology, 21(10), 731–739. doi: 10.1007/s10654-006-9046-5
- Suominen-Taipale, A. L., Martelin, T., Koskinen, S., Holmen, J., & Johnsen, R. (2006). Gender differences in health care use among the elderly population in areas of Norway and Finland. A cross-sectional analysis based on the HUNT study and the FINRISK Senior survey. BMC Health Services Research, 6(1), 110. doi: 10.1186/1472-6963-6-110
- Tunstall-Pedoe, H., Connaghan, J., Woodward, M., Tolonen, H., & Kuulasmaa, K. (2006). Pattern of declining blood pressure across replicate population surveys of the WHO MONICA project, mid-1980s to mid-1990s, and the role of medication. BMJ, 332(7542), 629–635. doi: 10.1136/bmj.38753.779005.BE
- Victora, C. G., Fenn, B., Bryce, J., & Kirkwood, B. R. (2005). Co-coverage of preventive interventions and implications for child-survival strategies: Evidence from national surveys. The Lancet, 366(9495), 1460–1466. doi: 10.1016/S0140-6736(05)67599-X
- Victora, C. G., Joseph, G., Silva, I. C. M., Maia, F. S., Vaughan, J. P., Barros, F. C., & Barros, A. J. D. (2018). The inverse equity hypothesis: Analyses of institutional deliveries in 286 national surveys. American Journal of Public Health, 108(4), 464–471. doi: 10.2105/AJPH.2017.304277
- Victora, C. G., Vaughan, J. P., Barros, F. C., Silva, A. C., & Tomasi, E. (2000). Explaining trends in inequities: Evidence from Brazilian child health studies. The Lancet, 356(9235), 1093–1098. doi: 10.1016/S0140-6736(00)02741-0
- Vikum, E., Bjørngaard, J. H., Westin, S., & Krokstad, S. (2013). Socio-economic inequalities in Norwegian health care utilization over 3 decades: The HUNT study. European Journal of Public Health, 23(6), 1003–1010. doi: 10.1093/eurpub/ckt053
- Vikum, E., Krokstad, S., & Westin, S. (2012). Socioeconomic inequalities in health care utilisation in Norway: The population-based HUNT3 survey. International Journal for Equity in Health, 11(1), 48. doi: 10.1186/1475-9276-11-48
- Wang, A., Clouston, S. A. P., Rubin, M. S., Colen, C. G., & Link, B. G. (2012). Fundamental causes of colorectal cancer mortality: The implications of Informational diffusion. The Milbank Quarterly, 90(3), 592–618. doi: 10.1111/j.1468-0009.2012.00675.x
- Wikman, A., Marklund, S., & Alexanderson, K. (2005). Illness, disease, and sickness absence: An empirical test of differences between concepts of ill health. Journal of Epidemiology & Community Health, 59(6), 450–454. doi: 10.1136/jech.2004.025346
- World Health Organization. (1997). A research policy agenda for science and technology to support global health development: A synopsis. Geneva: World Health Organization.
- Zapata-Moya, ÁR, Willems, B., & Bracke, P. (2019). The (re) production of health inequalities through the process of disseminating preventive innovations: The dynamic influence of socioeconomic status. Health Sociology Review, 28(2), 177–193. doi: 10.1080/14461242.2019.1601027