Abstract
Automated vehicle acceptance (AVA) is a necessary condition for the realisation of higher-level objectives such as improvements in road safety, reductions in traffic congestion and environmental pollution. On the basis of a systematic literature review of 124 empirical studies, the present study proposes MAVA, a multi-level model to predict AVA. It incorporates a process-oriented view on AVA, considering acceptance as the result of a four-stage decision-making process that ranges from the exposure of the individual to automated vehicles (AVs) in Stage 1, the formation of favourable or unfavourable attitudes towards AVs in Stage 2, making the decision to adopt or reject AVs in Stage 3, to the implementation of AVs into practice in Stage 4. MAVA incorporates 28 acceptance factors that represent seven main acceptance classes. The acceptance factors are located at two levels, i.e., micro and meso. Factors at the micro-level constitute individual difference factors (i.e., socio-demographics, personality and travel behaviour). The meso-level captures the exposure of individuals to AVs, instrumental domain-specific, symbolic-affective and moral-normative factors of AVA. The literature review revealed that 6% of the studies investigated the exposure of individuals to AVs (i.e., knowledge and experience). 22% of the studies investigated domain-specific factors (i.e., performance and effort expectancy, safety, facilitating conditions, and service and vehicle characteristics), 4% symbolic-affective factors (i.e., hedonic motivation and social influence), and 12% moral-normative factors (i.e., perceived benefits and risks). Factors related to a person’s socio-demographic profile, travel behaviour and personality were investigated by 28%, 15% and 14% of the studies, respectively. We recommend that future studies empirically verify MAVA using longitudinal or experimental studies.
Relevance to human factors/Relevance to ergonomics theory
User acceptance is one of the most important human factors constructs to realise the benefits of road vehicle automation. The present study proposes MAVA, a multi-level model on automated vehicle acceptance. Based on 124 empirical studies, MAVA predicts the acceptance of highly (SAE Level 4) and fully automated (SAE Level 5) vehicles. It adopts a procedural view on automated vehicle acceptance and organises the factors of acceptance at the micro and meso level.
1. Introduction
Automated vehicle acceptance (AVA) is a necessary condition for AVs to contribute to improvements in road safety, road capacity, reductions in travel time and greenhouse gas emissions. Research on AVA has gained new momentum, creating a need and opportunity to review theories explaining AVA, in order to synthesise a model predicting AVA and to validate this model using empirical studies.
The idea to integrate theories and models from various influential disciplines is not new, and responds to concerns that erroneous and inconsistent conclusions across studies may be derived if a narrow approach (i.e., not studying the influence of a broad range of the factors affecting the outcome variable and their interactions) to study multilevel processes such as AVA is adopted (Alfonzo 2005; Devine-Wright 2008; Stern 2000). Current studies on AVA have not captured this multi-determination. Instead, they mainly investigate the influence of AVA factors in isolation, and through the lenses of technology acceptance (Hewitt et al. Citation2019; Kaur and Rampersad Citation2018; Leicht Chtourou, and Youssef Citation2018; Madigan et al. Citation2016; Madigan et al. Citation2017; Wu et al. Citation2019; Zhang et al. Citation2019; Zmud and Sener Citation2017). The AVA factors identified by these studies encompass (1) individual characteristics (e.g., socio-demographics and differences in one’s own personality; Alessandrini et al. Citation2014; Haboucha et al. Citation2017; Lavieri et al. Citation2017; TNS Opinion and Social Citation2015), (2) instrumental domain-specific (e.g., performance and effort expectancy, physical vehicle and service characteristics; Nordhoff et al. Citation2018), (3) symbolic-affective (e.g., social influence and hedonic motivation; Bansal and Kockelman Citation2018; Madigan et al. Citation2016), and (4) moral-normative factors of AVA (i.e., risk and benefit perception of AVs; Daziano, Sarrias, and Leard, Citation2017; Kyriakidis, Happee, and De Winter Citation2015; Moták et al. Citation2017; Nordhoff et al. Citation2018; Tennant et al. Citation2016).
No comprehensive model exists to date that integrates these different research streams on the basis of empirical evidence to explain and predict AVA. Our conceptual model of the acceptance of driverless vehicles (Nordhoff, Van Arem, & Happee Citation2016) postulates that AVA is a function of instrumental domain-specific and symbolic-affective factors, ignoring moral-normative factors and the multi-level positioning of AVA factors. The present study fills this gap in research and introduces MAVA – the comprehensive multi-level model of automated vehicle acceptance (AVA) – that accounts for AVA’s multi-determination aspect. Based on a review of 124 empirical studies, the model summarises the current knowledge on AVA and serves as discussion piece for scholars and practitioners who are invited to critically reflect on the factors identified in the model, as well as the relationships between the included factors.
MAVA explains and predicts AVA of driverless Level 4 (L4) and Level 5 (L5) Automated Driving System (ADS)-Dedicated Vehicles (DVs) in line with the definitions provided by the SAE International (Citation2018) taxonomy. ADS-DVs are often designed without standard user interfaces as input devices for braking, accelerating, steering and transmission, and provide high automation levels (L4 or L5). ADS-DVs will generally not have a driver or operator on board, but temporary control might be provided by a conventional or remote driver (SAE International Citation2018). Throughout this article, we refer to L4/L5 ADS-DVs as ADS-DVs. Where L4 ADS-DVs can operate in automated mode on limited road sections, L5 ADS-DVs can operate in automated mode on all publicly accessible roadways (including parking areas and private campuses that permit public access) that are navigable by human drivers (SAE International Citation2018).
An important class of L4 ADS-DVs is represented by driverless automated shuttles as hybrid form of individual-public transport that deliver on-demand transport and serve as (last mile) feeder modes to public transport systems (Fraedrich, Beiker, and Lenz Citation2015). L5 ADS-DVs might be offered as mobility service operation by taxi companies, or companies that operate a fleet of automated carsharing or ridesharing vehicles on the basis of different parameters (e.g., trip, mile, minute or a combination thereof). Alternatively, they might be individually-owned (Lavieri et al. Citation2017). MAVA is constrained to the prediction of AVA of L4/L5 ADS-DVs, and does not cover SAE L2–3 vehicles that are designed to be operated by a human driver during part or all of the trip (SAE International Citation2018). Thus, MAVA does not address challenges specific to SAE L2–3 such as transitions of control, and drivers’ situation awareness and mental workload as the two most important Human Factors constructs determining safe usage of L2–3 automation (De Winter et al. Citation2014).
The article is organised as follows. Section 2 describes our methodology to derive the multi-level model through a systematic literature review. Section 3 first presents and motivates the model structure. It describes the model’s meso-level capturing the main effects of instrumental domain-specific, symbolic-affective and moral-normative factors in addition to the model’s micro-level that unites the individual difference acceptance factors. Finally, concluding remarks and potential implications for future research are presented in Section 4.
2. Methodology
To perform the literature review, we followed the guidelines of the Preferred Reporting Items for Systematic Reviews and Meta-Analyses (PRISMA) (Moher et al. Citation2009). In correspondence to Zhang et al. (Citation2018), we did not generate or register a protocol.
2.1. Information sources and search strategy
To develop a theoretical model that predicts AVA of L4/5 ADS-DVs and explore the determinants of AVA, we thoroughly examined the available Scopus and Web of Science listed peer-reviewed articles up to April 2019. The articles included in the review contained in the title, abstract or keywords any combinations of the following keywords: acceptance, acceptability, perceptions, attitudes, opinions, automated driving, autonomous driving, self-driving vehicle(s), driverless vehicle(s), automated and autonomous vehicle(s). Additional searches were performed using Google and Google Scholar to enlarge the pool of selecting suitable studies into our sample. For consistency, the same keywords were used. The reference lists of all the studies that met the search criteria were reviewed to retrieve other relevant studies. In line with Zhang et al. (Citation2018), we retrieved all types of studies, including journal publications, articles from conference proceedings, theses, reports, posters and presentation slides. This enables the identification of grey literature records and minimises publication bias.
2.2. Study selection and data extraction
In the first stage, we retrieved 1537 potentially-relevant full-text records, which were further reviewed for eligibility. We removed 637 duplicate records, and 776 records which did not fulfil our search criteria. Instead, they investigated different types of technologies (e.g., autonomous underwater, micro air, mining and urban land vehicles, automated highway systems, planes and driverless trains), SAE Levels 1–2 where the interaction with the human driver is prominent, or technical, legal, ethical and policy-related aspects of autonomous vehicles. We also excluded review-based studies, which already discussed the results of some of the studies that met our eligibility criteria. 124 records were thus retained in the qualitative analysis in the final stage.
2.3. Analysis
Following the procedure presented in Nordhoff et al. (Citation2019), we counted how many studies investigated specific AVA factors. Multiple entries of an AVA factor by the same study equaled a frequency of 1. AVA factors that were investigated by fewer than five studies were not included in the model.
3. Theoretical model
Before presenting MAVA, it is important to introduce the underlying theories and assumptions that provide the basis for the model. In correspondence to Kaur and Rampersad (Citation2018), who draw upon the literature on technology adoption and driverless cars to review key factors in the adoption of driverless cars, we synthesised the factors identified by technology acceptance models in conjunction with our findings from the AVA literature.
The first theoretical framework that provides the structural foundation for MAVA is the UTAUT3 proposed by Venkatesh, Thong, and Xu (Citation2016) as a result of a critical review of research studies that applied, extended or integrated the former UTAUT1 and UTAUT2 models (Venkatesh et al. Citation2003; Venkatesh et al. Citation2012). The UTAUT3 distinguishes between individual-level contextual factors (i.e., individual, technology and task attributes and events), higher-level contextual factors (i.e., environmental, organisation and location attributes) and a baseline model that is formed by the main effects of the UTAUT2 model. The strength of the UTAUT3 is its holistic and comprehensive overview of the possible factors impacting technology acceptance, which corresponds with our objective to build a comprehensive model to predict AVA. We follow the proposition of Venkatesh, Thong, and Xu (Citation2016) and locate potentially relevant determinants of AVA at two levels: meso and micro. The meso-level represents the main effects of instrumental domain-specific, symbolic-affective and moral-normative aspects of AVA. In line with our propositions in Nordhoff, Van Arem, and Happee (2016), we also assume interrelations between the factors forming the domain-specific, symbolic-affective, and moral-normative part of the model. The micro-level captures individual difference factors.
The second theoretical foundation of MAVA is the Car Technology Acceptance Model (CTAM) to predict the acceptance of in-car technology introduced by Osswald et al. (Citation2012). The CTAM posits that in-car technology acceptance is associated with the UTAUT1 constructs (i.e., performance and effort expectancy, social influence and facilitating conditions) along with further factors such as the perceived safety.1
MAVA adopts a process-oriented view on AVA, which is supported by the literature on technology acceptance and new mobility concepts (Bagozzi and Lee Citation1999; Endsley Citation1985b; Meijkamp & Theunissen Citation1996; Rogers Citation1983; Schwarz et al. Citation2014). We build upon these ideas and propose a four-stage-decision-making model capturing the information processing steps behind the adoption or rejection of ADS-DVs, illustrated in .
Figure 1. Multi-level model to explain and predict AVA (MAVA).
Note: The individual difference factors at the micro-level influence the factors at the meso-level directly or indirectly through a mediator or moderator effect. We also assume interrelations between the factors forming the domain-specific, symbolic-affective, and moral-normative part of the model.
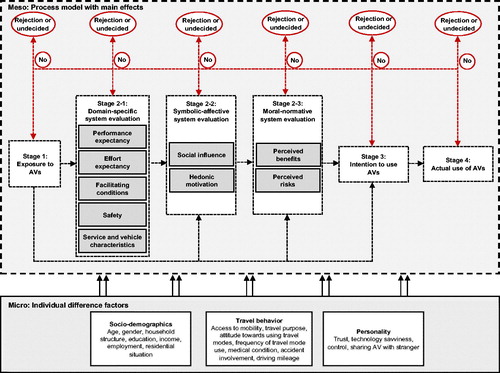
Figure 2. Pie diagram showing the seven main acceptance classes (outer ring) and the corresponding 28 acceptance factors (inner ring). Note: The arc length of the sectors is proportional to the number of studies.
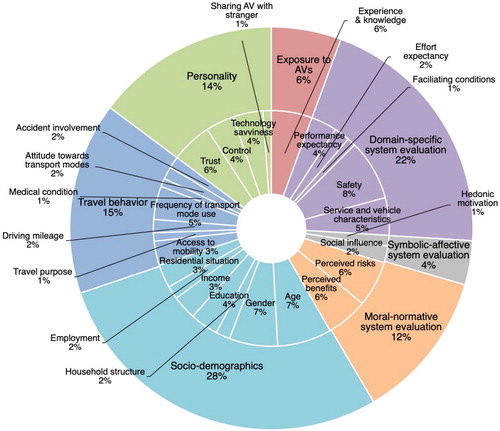
We posit that this process starts with the exposure of the individual to ADS-DVs in Stage 1 by the communication about ADS-DVs by an external stimulus (e.g., word-of-mouth communication from family or friends, advertising, media or test ride), or an internal change such as being aware of a problem or perceiving a need. The outcome of Stage 1 can either be a resistance or rejection of ADS-DVs or an interest to explore ADS-DVs. In the latter case, the individual moves to Stage 2, leading to a favourable or unfavourable attitude towards ADS-DVs on the basis of the evaluation of the instrumental domain-specific, symbolic-affective and moral-normative characteristics of ADS-DVs. In the next stage (Stage 3), the individual makes the decision to either use or reject ADS-DVs, or remains undecided. In the last stage (Stage 4), the individual puts ADS-DVs into use, e.g., by using ADS-DVs as part of a carsharing or ridesharing scheme, or as feeder to public transport systems.
MAVA organises the factors of AVA hierarchically in line with Maslow’s (Citation1954) hierarchy of human needs. As shown in , the evaluation of fundamental and basic instrumental domain-specific aspects of ADS-DVs (Stage 2–1) precedes the symbolic-affective (Stage 2–2) and moral-normative appraisal (Stage 2–3) of ADS-DVs. In other words, individuals will first try to realise the fulfilment of basic and fundamental domain-specific aspects of ADS-DVs before they aim for the realisation of higher-level symbolic-affective and moral-normative factors of AVA. The relevance of fundamental domain-specific aspects of ADS-DVs corresponds with the literature on road vehicle automation. For example, the system dynamics model to simulate the innovation diffusion of AVs proposed by Nieuwenhuijsen et al. (Citation2018) posits that the attractiveness of AVs is determined by safety and comfort, which are domain-specific aspects of ADs-DVs. Note that in line with the limitations of Maslow’s (Citation1954) hierarchy of human needs and Endsley’s (Citation1985b) process model on the implementation of technological change, the evaluation of ADS-DVs does not have to proceed in a linear order where each stage in the model is a necessary condition for entering the subsequent stage. Instead, the sequence can also be dynamic and occur in a non-linear, unsystematic or parallel fashion, where individuals skip stages, jump between stages, or process each stage simultaneously.
3.1. Literature study results at micro- and meso- level
From the 124 studies that were included in the literature study, we selected 28 factors in MAVA. As shown in , these 28 factors form 7 classes:
Table 1. Overview of AVA factors and the number of studies that investigated the AVA factor (n).
Class 1 (Factor 1): Exposure to AVs
Class 2 (Factors 2–6): Domain-specific system evaluation
Class 3 (Factors 7–8): Symbolic-affective system evaluation
Class 4 (Factors 9–10): Moral-normative system evaluation
Class 5 (Factors 11–17): Socio-demographics
Class 6 (Factors 18–24): Travel behavior
Class 7 (Factors 25–28): Personality
Acceptance Classes 1–4 are at the meso-level, while Classes 5–7 are at the micro-level.
As the main objective of MAVA is to investigate AVA at the individual level, we discarded the factors 29–34 at the macro-level:2
Distance home-workplace, home-downtown, home-public-transit
GPD/capita
Employment density, % of families below the poverty line
Population density
Policy measures
Road conditions under which people want to use AVs
Furthermore, as the UTAUT3 on which MAVA is based, does neither include the attitude towards using the technology under investigation nor negative emotions, we further omitted factors 35–36 from MAVA:
Attitude towards using AVs
Negative emotions
Finally, we omitted factors 37–42 that were mentioned by fewer than five studies:
Sensation seeking
Big 5 Inventory: Extraversion, Agreeableness, Conscientiousness, Neuroticism and Openness
Political orientation
Difficulty finding a parking place
Motion sickness susceptibility
Values
presents the extracted factors 1–28 at the micro- and meso-level, their meaning, and the number of studies investigating these factors. Figure 2 provides a pie diagram showing the seven main acceptance classes and their corresponding twenty-eight acceptance factors.
3.2. Meso-level
3.2.1. Exposure to AVs: knowledge and experience
Various authors have investigated individual’s level of knowledge of and experience with AVs (e.g., Berliner et al. Citation2019; Spurlock et al. Citation2019), and supported the notion that AVA is a function of an individual’s knowledge of and experience with AVs (e.g., Bennett et al. Citation2019; König & Neumayr Citation2017; Penmetsa et al. Citation2019; Sener et al. Citation2019). We generally assume that the effect of experience on AVA depends on whether the experience was positive or negative. Tennant et al. (Citation2016) found that the more the respondents reflected on AVs, the more positive they became, and that people using in-car automated technology (e.g., Cruise Control) were more open to accept AVs. In the same vein, Kyriakidis, Happee, and De Winter (Citation2015) found that people currently using Adaptive Cruise Control (ACC) would be willing to pay more for AVs, while they were also more comfortable about driving without a steering wheel. Sanbonmatsu et al. (Citation2018) pointed to the limited knowledge of the public of fully automated vehicles (i.e., only 32.5% was aware that self-driving vehicles would not be equipped with a steering wheel) and found that as knowledge of fully automated vehicles increased, beliefs about driverless cars were more positive. Anania et al. (Citation2018) revealed that, with the exception of Indian females, receiving positive information resulted in a higher willingness to ride in a driverless vehicle compared to receiving negative or no information. Eden et al. (Citation2017) who investigated the opinions and attitudes around safety and comfort of people both before and after riding on an AV shuttle revealed that the safety concerns of people disappeared after the ride. Hartwich et al. (Citation2019) revealed that the initial system experience significantly increased trust and acceptance of highly-automated driving. In contrast, the study of Dekker (Citation2017) did not find a significant influence of experience with AV-DVs on the preferences for AV-DVs. Note that most of the studies on AVA that we retrieved in the process of the literature review are based on respondents’ knowledge (i.e., knowledge respondents gain in the context of the study, through media, other information channels and friends/colleagues) rather than direct physical experience with AVs.
3.2.2. Domain-specific system evaluation: performance and effort expectancy, facilitating conditions, safety, and service and vehicle characteristics
As early as 1985, Endsley (Citation1985a, Citation1985b) discussed the importance of acceptance for the introduction and success of new technologies. Almost 20 years later, Venkatesh et al. (Citation2003) proposed the Unified Theory of Acceptance and Use of Technology (UTAUT), which provides a comprehensive synthesis of research to model technology acceptance and integrates eight influential acceptance models (e.g., Theory of Planned Behavior and Technology Acceptance Model). It postulates that performance expectancy,3 effort expectancy, social influence and facilitating conditions influence the behavioral intention of an individual to use a technology, while behavioral intention and facilitating conditions determine actual system usage (Venkatesh et al. Citation2003). Age, gender and experience moderate the relationship between performance expectancy, effort expectancy, social influence and facilitating conditions and behavioral intention. UTAUT2, which follows from UTAUT1, suggests that an individual’s behavioral intention to use information technology is influenced by three additional constructs in addition to the original UTAUT, i.e., hedonic motivation price value and habit4 (Venkatesh, Thong, and Xu Citation2012, 161). The suitability of the UTAUT1/2 to predict the acceptance of ADS-DVs has been supported by Leicht et al. (Citation2018), Kaur and Rampersad (Citation2018), Moták et al. (Citation2017), Zhang et al. (Citation2019) and Zmud and Sener (Citation2017) who revealed that performance expectancy (or its equivalent ‘perceived usefulness’) was significantly associated with the intention to use AVs. In Nordhoff et al. (Citation2018), performance expectancy and effort expectancy measuring the component ‘shuttle effectiveness’, which pertained to the performance of L4 ADS-DVs in comparison with respondents’ existing travel, significantly correlated with the component ‘intention to use’, which corresponds with the UTAUT construct ‘behavioral intention’. The effect of facilitating conditions (or its equivalent ‘perceived behavioral control’5, ‘helpfulness’, ‘technical support’, ‘(technical) self-efficacy’, ‘conceptual compatibility/fit’, ‘lifestyle fit’ and ‘technology confidence’) on the intentions to use ADS-DVs was supported in the study of Buckley, Kaye, and Pradhan (Citation2018), Brell, Philipsen, and Ziefle (Citation2019a), Hewitt et al. (Citation2019), Jing et al. (Citation2019) and Madigan et al. (Citation2017). Consequently, we conclude that performance and effort expectancy and facilitating conditions are positively correlated with the intention to use ADS-DVs.
Safety is one of the basic human needs and one of the key drivers influencing AVA (Brell et al. Citation2019a; Cho et al. Citation2017; Kaan Citation2017; Liu et al., Citation2019; Overakker Citation2017; Piao et al. Citation2016; Salonen Citation2018; Nazari et al. Citation2018; Xu et al. Citation2018). Topics around safety and security include equipement and system failure, cyber security or the fear of terrorism and hacking, and system performance in poor weather and terrain and unexpected conditions (e.g., Bansal & Kockelman Citation2017; Dong et al. Citation2017; Gurumurthy Citation2017; Penmetsa et al. Citation2019; Tussyadiah et al. Citation2017). At the moment, there seems to be a divide between the opinions of academics and practitioners and the general public regarding the expected safety benefits of AVs. While academics and practitioners do not seem to expect AVs to be error-free (Kalra and Paddock Citation2016), the general public does not seem to be willing to accept fatalities that arise due to automation as exemplified by the public reactions to the accidents with the partly-automated vehicles from Tesla and Uber (Eden et al. Citation2017). Xu et al. (Citation2018) found that perceived safety was significantly correlated with the intention to use and willingness to re-ride in AVs, which aligns with Nazari, Noruzoliaee, and Mohammadian (Citation2018) who revealed that safety concerns reduced interest in shared automated vehicles.
In addition to safety, vehicle and service attributes have been considered important determinants of AVA. Site et al. (Citation2011) revealed that on-board comfort (i.e., whether passengers were sitting or standing) was related to the use of automated vehicles. In our interview study (Nordhoff et al. Citation2019), the majority of respondents’ quotes captured service quality aspects (e.g., availability, flexibility and convenience), hinting that service quality is an important determinant of AVA. Krueger, Rashidi, and Rose (Citation2016) found that service quality aspects including travel time, waiting time and travel costs were significant determinants of AVA. Consequently, we conclude that physical vehicle and service characteristics are correlated with the intention to use ADS-DVs.
3.2.3. Symbolic-affective aspects of AVA: hedonic motivation and social influence
Social influence and hedonic motivation capture the symbolic-affective aspects of AVA, which have been found to play significant roles in consumer adoption behavior and intentions, such as the use of private motorised cars, electric and automated vehicles (Panagiotopoulos & Dimitrakopoulos Citation2018; Rezvani et al. Citation2015; Steg et al. Citation2001). Bansal and Kockelman (Citation2018) corroborated the effect of social influence on the willingness to adopt AVs by showing that some individuals were less likely to rely on the adoption rates of their friends (i.e., disabled, bachelor’s degree holders and familiar with car-sharing) than others (i.e., elderly, single and Caucasian ethnicity). Acheampong and Cugurullo (Citation2019) found a positive relationship between subjective norm (equivalent to social influence) and the perceived benefits (equivalent to performance expectancy, see Xu et al., Citation2018; Zhang et al., Citation2019), and ease of use of automated driving technology (equivalent to effort expectancy), and a positive relationship between subjective norm and perceived behavioral control (equivalent to facilitating conditions). Hartwich, Beggiato, and Krems (Citation2018) postulate that affective variables are of growing importance for drivers’ vehicle choices, and found a significant effect of enjoyment on automated driving. The role of emotions in AVA was substantiated by Rödel et al. (Citation2014) who found that fun6 declines with higher levels of vehicle automation, and Kyriakidis, Happee, and De Winter (Citation2015), who revealed that respondents considered full automation to be the least enjoyable and manual driving the most enjoyable mode of driving. Hohenberger, Sporrle, and Welpe (Citation2016) observed that pleasure was positively correlated with the willingness to use AVs, while anxiety was negatively correlated with the willingness to use AVs. In light of these findings, we conclude that social influence and hedonic motivation are correlated with the intention to use ADS-DVs.
3.2.4. Moral-normative aspects of AVA: risk-benefit perception
In line with Kohl et al. (Citation2018) and Raue et al. (Citation2019), we argue that the perception of risks and benefits are important factors predicting AVA. Similar to Milakis, Van Arem, and Van Wee (Citation2017) in their ripple effect of automated driving model, the moral-normative perception of risks and benefits in our model represents higher-order implications of AVs on energy consumption, air pollution, economy, public health and social equity. Risks that the public tends to associate with the introduction of AVs typically include legal liability of drivers or owners of an automated vehicle in case of an accident, data privacy (e.g., location and destination tracking), loss of driving skills and pleasure, interacting with manually controlled cars, pedestrians and cyclists, lack of assistance for disabled individuals, affordability, traffic delays, and ethical or social consequences (e.g., loss of human interaction and control in public transport, unemployment among bus and taxi drivers, social isolation, dependence on technology, and an overreliance on AV technology) (Bansal and Kockelman Citation2018; Bloom et al. Citation2017; Brinkley et al. Citation2017; Fraedrich & Lenz Citation2016; Gilbert & Daily Citation2018; Greaves et al. Citation2018; Jardim et al. 2013; Kyriakidis et al. Citation2015; Lavieri et al. Citation2017; Lilijamo et al. Citation2018; Medina and Jenkins Citation2017; Menon Citation2015; Pakusch & Bossauer Citation2017; Pettigrew et al. Citation2018, Citation2019; Portouli et al. Citation2017; Regan et al. Citation2017; Sanaullah et al. Citation2016; Sauders & Charness Citation2016; Shabanpour et al. Citation2017, Citation2018; Shin & Shunsuke Citation2017; Ulahannan et al. Citation2018; Wang and Ankar Citation2019; Woisetschläger Citation2016; Woldemanuel and Nguyen Citation2018). Benefits encompass improvements in productivity due to the engagement in non-driving related tasks, benefits for the environment (e.g., reduction of fuel consumption, emissions and traffic congestion, lower vehicle ownership), increased mobility independence and freedom for the physically-impaired and elderly, no need to spend resources on learning how to drive, lower insurance rates, lower repair costs (in case of less accidents), and easier and quicker and less expensive parking (Adnan et al. 2018; Bazilinskyy et al. 2015; Bennett et al. 2019; Dai & Howard 2013; Daziano et al. Citation2017; Hulse et al. 2018; Kelkel 2015; Lustgarten & Le Vine 2018; Medina and Jenkins Citation2017; Portouli et al. Citation2017; Sauders & Charness 2016; Shin & Shunsuke 2017; Tennant et al. Citation2016; Yap et al. 2016). Ward et al. (Citation2017) found that the perception of risks and benefits was significantly correlated with the interest in using an AV, with the level of comfort with automation, and with the desire for highly automated driving features in the next vehicle. Piao et al. (Citation2016) found that the expected benefits of AVs were positively correlated and the expected concerns of AVs were negatively correlated with the attitudes of their respondents. Wu et al. (Citation2019) found that people who value the environmental benefits of AVs and who reported to be concerned about the environment were more likely to value the environmental benefits of AVs and showed a higher willingness to use or buy AVs. On the basis of these considerations, we propose that the perception of risks is negatively correlated and the perception of benefits is positively correlated with the intention to use ADS-DVs.
3.3. Micro-level: individual difference factors
The meso-level processes are defined for the entire population. In this section, we discuss individual difference factors explaining variance in behaviour as reported in empirical studies. In line with Ajzen and Fishbein (Citation2005), Bagozzi and Lee (Citation1999) and Venkatesh, Thong, and Xu (Citation2016), we propose that these individual difference factors are generic AVA factors that influence the intention to use ADS-DVs by mediating or moderating the relationship between the factors at the meso-level. This is confirmed by Zhang et al. (Citation2019) who found that trust (individual difference factor at micro-level) mediated the correlation between perceived safety, usefulness and attitudes towards using AVs, which influenced the intention to use AVs (factors at meso-level). We also expect independent main effects of the individual difference factors on the factors at the meso-level, including the intention to use AVs. For example, Xu et al. (Citation2018) found significant positive effects of trust on perceived usefulness and ease of use and the intention to use AVs.
3.3.1. Socio-demographics
Concerning the influence of socio-demographic characteristics on AVA, three patterns are currently observable based on the literature. Men tend to be more accepting of AVs than women: They are more aware of automated driving functions, tend to pay more for automation, are more interested in AV ownership, rate AVs as more useful, seem to be less concerned about safety, are less afraid to drive in AVs and trust AVs more, and are more comfortable to allow a fully automated car to perform all driving tasks (Choi and Ji Citation2015; Kyriakidis, Happee, and De Winter Citation2015; Liu, Yang, and Xu Citation2019; Nazari, Noruzoliaee, and Mohammadian Citation2018; Regan et al. Citation2017; TNS Opinion and Social Citation2015; Zhang et al. Citation2019).
The effect of age is ambiguous. Bansal, Kockelman, and Singh (Citation2016) and Schoettle and Sivak (Citation2014, Citation2015) reported that younger people were more accepting of AVs than older people. In contrast, Rödel et al. (Citation2014) showed that people aged 36 to 65 had a more positive attitude and a stronger intention to use AVs than people aged 18 to 35. In our study (Nordhoff et al. Citation2018), we found that elderly people were more likely to express an intention to use automated vehicles in public transport and were positive towards the characteristics of the automated vehicle but rated the effectiveness of the vehicle more negatively in comparison with their existing form of travel.
The statistical effects of gender and age on individuals’ behavioral intentions to use AVs are usually weak or disappear when considered with other social-psychological variables being held constant. For example, Madigan et al. (Citation2016) who applied the UTAUT model to understand the predictors of the acceptance and use of AVs in La Rochelle (France) and Lausanne (Switzerland) found that age effects disappeared when they were examined as part of a multiple regression where the influences of all variables under study were held constant. The effect of gender also disappeared in the multiple regression of Payre, Cestac, and Delhomme (Citation2014), when contextual acceptability as measured by four ordinal-scale items (e.g., ‘If driving was boring to me, I would rather delegate it to the automated driving system instead of doing it myself’) was added to the model in the second step. Kyriakidis, Happee, and De Winter (Citation2015) found neither clear age nor gender effects because the correlations were mostly smaller than 0.10, which corresponds with Regan et al. (Citation2017) whose effect sizes of age and gender were found to be small, and with Webb, Wilson, and Kularatne (Citation2019) who found marginally significant to no significant age effects. These findings also correspond with research unrelated to AVs. For example, Stern (Citation2000) found that socio-demographic variables were unrelated to consumer behavior and policy support when social-psychological variables could simultaneously influence the outcome variable, and with Fernández-Heredia et al. (Citation2016) who found that income and Spanish nationality dropped out of their model when their four latent explanatory variables (i.e., convenience, pro-bike attitude, physical determinants and external restrictions) were included.
Besides age and gender, Liu, Guo, Ren, Wang, and Xu (Citation2019) and Kyriakidis, Happee, and De Winter (Citation2015) observed a positive relationship between income and willingness to pay for vehicle automation. Hardman, Berliner, and Tal (Citation2019) revealed that ‘Pioneers’ and ‘Pro-automated consumers’ had the highest incomes, while ‘Driverless sceptics’ and ‘Laggards’ had the lowest incomes. Hudson, Orviska, and Hunady (Citation2019) found that peoples’ degree of comfort with driverless cars increased with their level of education and prosperity, and decreased with being a manual worker, unemployed, retired or a farmer. Bansal, Kockelman, and Singh (Citation2016) and Nazari, Noruzoliaee, and Mohammadian (Citation2018) further revealed that the number/presence of children in a household were positively related to the willingness to pay for automation and the propensity to carpool with AV for commute trips. Hudson, Orviska, and Hunady (Citation2019) revealed that support for driverless cars was lower for those living in villages and small towns, and higher for city dwellers. This corresponds with Regan et al. (Citation2017) who found that residents in the more densely-populated South Australia were more positive towards the potential benefits of fully-automated cars and more agreeable to using fully automated cars compared to residents in the less densely-populated Northern Territory who had more negative perceptions and were less agreeable.
3.3.2. Personality
Personality-related factors that are considered pivotal for AVA are technology savviness, trust, locus of control and sharing an AV with a stranger.
As regards the effect of technology savviness, Bansal, Kockelman, and Singh (Citation2016) found that technology-savvy individuals were more positive towards AVs, which is in agreement with Lavieri et al. (Citation2017) who found that tech-savvy individuals are likely to be early adopters of AVs, and with Haboucha, Ishaq, and Shiftan (Citation2017) who found that individuals with a higher interest in technology were more likely to choose AVs. Wien (2019) revealed a positive relation between technology interest and the perceived utility and the use of an automated bus.
Trust has been considered a valid foundation for human-machine interaction (Hengstler et al. Citation2016), and plays a leading role in determining the willingness of humans to rely on automated systems (Hoff and Bashir Citation2015), and accept AVs (Haspiel et al. Citation2018; Molnar et al. Citation2018; Wintersberger, Frison, and Riener Citation2018; Zhang et al. Citation2018). Choi and Ji (Citation2015) observed that individual generic trust levels positively influenced the perceived usefulness of AVs and the intentions to use them, while it reduced any related perceived risks. Zmud and Sener (Citation2017) revealed that lack of trust in the technology was cited by 41% of the respondents as one of the reasons for being unlikely to ride in self-driving vehicles for everyday use. Abraham et al. (Citation2016) found that higher trust in the different entities to build a self-driving car and more comfort with higher levels of automation were associated with the willingness to pay more for a self-driving car. The focus group analysis of Brinkley et al. (Citation2017), however, identified issues related to risk and trust as the themes that were the least discussed by their focus groups members. We hypothesize that the relevance of trust will be especially strong in the early stages of AVs’ deployment, when people still have limited experience with and knowledge of AVs. Hartwich et al. (Citation2019) support this by showing that the acceptance and trust in automation significantly increased after the primary system experience with highly-automated driving vehicles, but remained stable after the initial system exposure. Note that trust has to be calibrated (i.e., individuals’ level of trust matches the capabilities of automation) to prevent misuse with overtrust and disuse with distrust in AVs (Hoff and Bashir Citation2015; Lee and See Citation2004). In an on-road study using a Tesla Model S operating in Autopilot mode, drivers showed behaviors of complacency and overtrust (Banks et al. Citation2018). These safety-critical behaviors can negatively impact the acceptance of AVs as inferred by the decrease in the level of trust of the public in AVs after the occurrence of fatal and serious accidents with AVs (Claybrook and Kildare Citation2018).
Locus of control captures individuals’ assumptions regarding the responsibility for the outcome of events (Rotter and Hochreich Citation1975; Rudin-Brown and Parker Citation2004). Locus of control was one of the main variables in a psychological model of driving automation (Stanton and Young Citation2000) updated in a later study (Heikoop et al. Citation2016). People with an internal locus of control (‘Internals’) are more likely to rely on their own skills and abilities and maintain a direct involvement with the system regardless of how safe or reliable it is. In contrast, people with an external locus of control (‘Externals’) are more likely to relinquish control to an external device and rely on it to perform a task (Payre, Cestac, and Delhomme Citation2014; Rotter 1966; Rudin-Brown and Parker Citation2004). There is still a paucity of knowledge regarding the extent to which AVs are accepted by ‘Internals’ and ‘Externals’. We expect that ‘Externals’ are more likely to surrender control to AVs, while ‘Internals’ are more inclined to prefer manual controls to intervene in the vehicle’s operations rather than surrendering control entirely. So far, studies generally reveal a preference of individuals to maintain some degree of control over the AV rather than relinquishing complete control to the AV. For example, Hassan et al. (Citation2019) found that the large majority of their respondents (81%) preferred to be in control of their vehicle because autonomous technologies cannot be foolproof. In our questionnaire study (Nordhoff et al. Citation2018), we found that the majority of our respondents agreed on being able to take over control from an AV by using a button inside the vehicle, which corresponds with Schoettle and Sivak (Citation2015), who found that about 96% of the respondents preferred the availability of vehicle controls.
Research has explored the role of the private car in maintaining privacy and personal space and enabling movement amongst people without jeopardizing personal space boundaries (Ibrahim, Citation2003; Petkewich, Citation2005). The automobility frame ‘Cocooning and Fortressing’ proposed by Sovacool and Axsen (Citation2018), which has dominated the literature, considers the car as cocoon, fortress or isolated enclave similar to a mobile living room where drivers are isolated from the world and relax, listen to music and engage in other (private) leisure activities. Sharing an AV with a stranger is an important factor for AVA, especially for AVs that are shared rather than personally-owned such as driverless automated shuttles as feeder modes to public transport systems, or AVs that are used as taxi, carsharing or ridesharing vehicles. Cunningham, Ledger, and Regan (Citation2018) found that ‘travelling in public transport in which the vehicle is driverless’ and ‘sharing a driverless vehicle’ received the lowest agreement among respondents. In the study of Bansal, Kockelman, and Singh (Citation2016), 50% of respondents reported to be comfortable in sharing a ride with a stranger for short durations during the day or with a friend of one of their Facebook friends. Sanguinetti, Kurani, and Ferguson (Citation2019) posit that there is still a paucity of knowledge regarding who will be willing to share rides, with whom and under what circumstances. Therefore, we strongly encourage future research to investigate the capability and willingness of individuals to share rides with strangers in AVs and its role for AVA.
While the above considerations provide evidence for the relationships between personality-related factors at the micro-level and factors at the meso-level, we also expect relationships between the factors within the micro-level. Abraham et al. (Citation2016), for instance, revealed that younger people have higher levels of trust in traditional automakers and the Silicon Valley technology companies than older people. These findings point to possible correlations between socio-demographics (e.g., age) and personality-related factors (e.g., trust), which deserve further investigation.
3.3.3. Travel behavior
The relevance of individuals’ travel behavior for AVA has been supported by the literature. For example, with respect to individuals’ access to mobility, Bansal, Kockelman, and Singh (Citation2016) found that licensed drivers were less likely to frequently use shared AVs. With respect to individuals’ driving experience, Kyriakidis, Happee, and De Winter (Citation2015) revealed that the more people drive, the more they are willing to pay for AVs, while Bansal, Kockelman, and Singh (Citation2016) found that individuals who experienced more accidents are more likely to embrace AVs. With regard to the use of daily modes of transport, Zmud and Sener (Citation2017) found a significant effect of the commuting mode on the intention to use self-driving vehicles with private passenger car drivers seeming to be more reluctant to use AVs than users of other transport modes (i.e., public transport vehicle passengers and walkers or telecommuters). Winter et al. (Citation2016) corroborated the influence of travel mode choices on the preferences for AV technology: Early adopters (i.e., users of the ride-sourcing company Uber, or members of households with at least one subscription to a carsharing company) found modes requiring parking and driving (car, free-floating carsharing) the least attractive and prefer demand-responsive modes that allow for the productive use of in-vehicle travel time by task engagement during the ride (taxi, shared AVs). Nielsen and Haustein (Citation2018) revealed that people who are sceptic towards AVs (‘Sceptics’) are more likely to drive their car just for the fun of it. In contrast, people being indifferent towards AVs (‘Indifferents’) tend to use the car for travel purposes other than commuting, while people being enthusiastic about AVs (‘Enthusiasts’) use the car to get to their holiday destination. Additionally, 45% of Sceptics use the car daily compared to 41% of ‘Enthusiasts’, and 29% of ‘Indifferents’.
4. Discussion
This study presents a comprehensive multi-level model on AVA, referred to as MAVA. MAVA is based on 124 empirical studies that were retrieved in a systematic literature review. The literature review revealed that 6% of the studies investigated the exposure of individuals to AVs (i.e., knowledge and experience). 22% of the studies investigated domain-specific factors (i.e., performance and effort expectancy, facilitating conditions, safety, and service and vehicle characteristics), 4% symbolic-affective factors (i.e., hedonic motivation and social influence) and 12% moral-normative factors (i.e., perceived benefits and risks). Factors related to a person’s socio-demographic profile, travel behaviour and personality were investigated by 28%, 15% and 14% of the studies, respectively. MAVA incorporates a process-oriented view on AVA as a function of factors located at the meso and micro level. The meso-level merges domain-specific, symbolic-affective and moral-normative factors. It is influenced by factors at the micro-level, representing individual difference factors. The model explains acceptance as a sequential, four-stage decision-making process that ranges from the exposure of the public to AVs in Stage 1, the formation of favorable or unfavorable attitudes towards AVs in Stage 2, making the decision to adopt or reject AVs in Stage 3, to the use of AVs in Stage 4.
The model can be operationalised in two ways. First, when the research goal is to understand AVA, the full model could be applied and adapted to the research context. Second, when the research goal is to explain or predict, a more parsimonious and directive (mathematical) model would be more suitable. The meso-level or factors at the meso-level could then be used together with factors at the micro-level to suit the context at hand. Previous studies mostly applied linear regression analyses to investigate the correlations between AVA factors and the acceptance construct itself. MAVA can be implemented as a non-linear multilevel model, capturing the four stages of acceptance in time. It represents a conditional model where actual acceptance will depend on all four stages as well as a probabilistic model with probability functions capturing the relationship between the factors at the micro level and the factors at the meso-level. Multivariate analysis methods such as regression or structural equation modelling can be applied to test and quantify mathematic relations between the factors in the model. Qualitative techniques (e.g., focus groups and interviews) could also be employed to explore the relevance of each factor and adjust the model by new factors that have not been identified yet. Finally, the model indicates the extent to which the factors in our model are causally related. Future studies should, therefore, examine the causal nature of these relationships using longitudinal and experimental studies.
While the strength of the model is its profound grounds in empirical research, the empirical evidence on which MAVA is based should be interpreted with regards to its caveats. That is, while the rapid growth in AVA research has undoubtedly been gratifying, researchers expressed some concerns regarding the current state of the literature. First, Fraedrich and Lenz (Citation2014) pointed out that most of the respondents who participated in the numerous studies lack broad knowledge of or actual experience with AVs, which may pose a threat to the validity of results. Second, Lavieri et al. (Citation2017) stated that the datasets used to investigate people’s attitudes towards AVs are mainly based on surveys with a variety of different assumptions and specifications to describe automated vehicle technologies and scenarios. Third, Langdon et al. (Citation2017) argued that despite comparable findings it is not clear yet how the public understands driverless technology and how this technology can form a part of their lives in the short- and middle-run. A second weakness of MAVA is its relatively low parsimony as it contains a large number of factors, which could make it cumbersome at times for researchers to use the model. Potential critics could refer to previous research, which has shown that only a small number of factors are needed to explain most of the variance in the outcome variable and where the explanatory power of these models could only be marginally increased by virtue of additional factors. For example, a 13-factor-model increased the variance in behavioral intention by only 2% over a 5-factor model (Taylor and Todd Citation1995). Yet, the 13-factor-model in this case provided a better understanding of the research phenomenon by showing which factors are less influential in predicting the intention to use technology. It can also be argued that in previous AVA studies (Böhm et al. Citation2017; Madigan et al. Citation2016; Madigan et al. Citation2017), instrumental domain-specific and symbolic-affective factors explained between 19.6% and 58.6% of the variance in individuals’ behavioral intentions to use AVs. This implies that a substantial amount of variance has not been captured so far, which again justifies the development of MAVA that contains additional predictors of AVA. A third limitation of MAVA is that for the sake of brevity and a paucity of knowledge, it only concentrates on the micro- and meso-level and refrains from detailing the macro-level as an assembly of higher-level external contextual factors (i.e., environment, organisation and location). The macro-level is a potentially relevant determinant of AVA (Sanbonmatsu et al. Citation2018). Future research should also not neglect the role of the factors that were discarded from the analysis of the present study. For example, regarding values, Moták et al. (Citation2017) found that personal values significantly impacted the intentions to use ADS-DVs. The authors concluded: ‘Values represent such a major research trend that it would be difficult to neglect them when seeking to apply as broad an approach as possible to automated shuttles’ acceptability’ (Moták et al. Citation2017, 271). Future research should therefore investigate the value basis of beliefs or behavior (Steg and Vlek Citation2009; Steg et al. Citation2014), and explore how self-enhancement and self-transcendence values7 influence AVA.
The fourth limitation is that MAVA does not specify the weight or strength of the AVA factors. However, in line with the propositions of Ajzen and Fishbein (Citation2005), we would assume that the relative importance of these factors varies as a function of the specific behavior and the population under consideration.
5. Final conclusions
In conclusion, the present article proposes MAVA, a multi-level model to predict automated vehicle acceptance. Building on UTAUT3 and CTAM, MAVA incorporates a process-oriented view and contemplates acceptance as a four-stage decision-making process. The process starts with the exposure of the individual to ADS-DVs in Stage 1, and moves to the formation of either a favorable or unfavorable attitude towards ADS-DVs in Stage 2, to deciding whether to use or reject ADS-DVs, or remain undecided in Stage 3, and to putting ADS-DVs into use in Stage 4. Twenty eight acceptance factors that were identified in the literature review are located at two levels, i.e., micro and meso. The factors at the meso-level represent the procedural view on acceptance with the exposure of individuals to AVs in terms of their experience with and knowledge about AVs preceding the domain-specific factors performance and effort expectancy, facilitating conditions, safety and service and vehicle characteristics, the symbolic-affective factors hedonic motivation and social influence and the moral-normative factors perceived risks and perceived benefits. The individual difference factors socio-demographics, travel behavior and personality at the micro-level influence the factors at the meso-level. We expect our model to provide useful insights to policy makers and other actors involved in the deployment of ADS-DVs. We recommend future research to revisit the model for empirical verification and adaptation and further explore the nature of these factors as well as the relationships between the factors using longitudinal or experimental studies.
Supplemental Material
Download MS Word (527 KB)Disclosure statement
The authors declare that there is no conflict of interest regarding the publication of this article.
Additional information
Notes on contributors
Sina Nordhoff
Sina Nordhoff is doing her PhD at the Delft University of Technology in joint cooperation with the InnoZ on user acceptance of automated public transport systems. In her first article, which was presented at the Transportation Research Board 95th Annual Meeting in Washington DC, she developed a conceptual model – the 4P Acceptance Model – to explain, predict and improve user acceptance of automated vehicles used in public transport. Under the supervision of Prof. Dr. Natasha Merat from the ITS Leeds, she worked on video data from the CityMobil2 project to investigate the interactions between automated vehicles and other road users with academics at ITS Leeds. At the InnoZ in Berlin, she was leading the user acceptance research, involving an automated vehicle from the US based start-up Local Motors, and the French company Easymile. Since January 01, 2019, she is working on the L3Pilot project, analyzing data from an international questionnaire study to investigate acceptance of conditionally-automated driving systems in 8 countries worldwide.
Miltos Kyriakidis
Miltos Kyriakidis joined the Future Resilient Systems programme in April 2016 to conduct research in the fields of human performance, human reliability, safety and resilience of critical infrastructure. Miltos has been actively working on the public acceptance of AVs. Prior to his appointment at FRS, Miltos was working as Marie Curie Fellow at Delft University of Technology, involved in the Human Factors of Automated Driving ITN project. He was investigating the legal and market perspectives of automated driving, as well as the impact of automated driving on road safety, human performance and behaviour, aiming at supporting responsible utilisation of future automation technology.
Bart van Arem
Bart van Arem was appointed full professor Transport Modelling at Delft University of Technology in 2009. He was head of the Department Transport and Planning from 2010–2017 and has been director of the TU Delft Transport Institute since 2011. Bart van Arem’s research and education have evolved around analysing and modelling the implications of intelligent transport systems. Such implications vary from human factors to traffic flow on roads and networks. His research has a strong modelling and simulation component based on empirics wherever possible using our instrumented vehicles and driving simulator. He teaches courses on Intelligent Transport Systems at BSc and MSc level and supervised almost 100 MSc students and 15 PhD researchers. His recent research interests are moving towards understanding of the spatial and societal implications of intelligent transport systems. He is the Principal Investigator of the STAD (Spatial and Transport impacts of Automated Driving) project with 30 academic, industrial and public partners and founder of the Researchlab Automated Driving Delft. In 2017 he received the Institutional Lead Award of the IEEE Intelligent Transportation System Society for his leadership of the TU Delft Automated Driving Research Programme.
Riender Happee
Riender Happee received the MSc degree in mechanical engineering and the PhD degree from Delft University of Technology (TU Delft), The Netherlands, in 1986 and 1992, respectively. He investigated road safety and introduced biomechanical human models for impact and comfort at TNO Automotive (1992–2007). Currently, he investigates the human interaction with automated vehicles focussing on safety, comfort and acceptance at the Delft University of Technology, The Netherlands, where he is associate professor at the Faculties of Mechanical, Maritime and Materials Engineering and Civil Engineering and Geosciences. Key projects include HFAuto (Human factors of automated driving), WEpods (driverless shuttles), SafeVRU (safe interaction with Vulnerable Road Users) and MOTORIST (safety of bicycles and powered two-wheelers).
Notes
1. Belief that using a system will affect his or her well-being.
2. The macro-level represents higher-level contextual factors in a person’s external environment such as environmental, organizational and location factors (Venkateshet et al. Citation2016). Environmental factors may encompass the legal (e.g., availability of public policies to support the implementation of AVs), economic (e.g., GDP per capita), meteorological (e.g., time of day, week, year), ecologic (e.g., high levels of nitrogen dioxide in cities), social (e.g., urbanization), and political environment (e.g., political support for AVs). Organizational factors may encompass specific organizational contexts in which AVs are embedded (e.g., rules and policies of public transport organizations as operators of shared automated vehicles). Location attributes may capture the physical infrastructure (e.g., availability of parking places or charging stations for automated vehicles) (Grotenhuis, Wiegmans, & Rietveld, Citation2007; Stern, Citation2000).
3. Performance expectancy is the degree to which using a technology will provide benefits to users in performing certain activities; Effort expectancy is the degree of ease associated with the use of technology; Social influence describes the extent to which users perceive that important others believe they should use a particular technology; Facilitating conditions refer to users’ perceptions of the objective resources and support available in the environment to perform a behaviour.
4. Hedonic motivation (fun or pleasure derived from using a technology), price value (i.e., monetary cost of technology use) and habit (i.e., extent to which an individual believes the behavior to be automatic).
5. Perceived behavioral control reflects perceptions about internal and external constraints to perform a behavior and encompasses self-efficacy; Helpfulness reflects perceptions about the provision of adequate and responsive aid; (Technical) self-efficacy reflects perceptions about having the ability and competence to use the technology (Venkatesh et al., Citation2003). Conceptual compatibility/fit captures beliefs that AVs will work in ways that make sense to the individual user. Lifestyle fit reflect user beliefs that using AVs will fit into the lifestyle of the individual user. Technology confidence is the degree of confidence in the ability to learn and use new technologies (Lee et al., Citation2017).
6. Fun is the extent to which the activity of using a specific system is perceived to be enjoyable (Rödel et al., Citation2014).
7. Self-enhancement values reflect a key concern with one’s own individual interests, while self-transcendence values reflect a key concern with the collective interest (Steg et al., Citation2014).
References
- *Abraham, H., C. Lee, S. Brady, C. Fitzgerald, B. Mehler, B. Reimer, and J. F. Coughlin. 2017. “Autonomous Vehicles and Alternatives to Driving: Trust, Preferences, and Effects of Age.” Proceedings of the Transportation Research Board 96th Annual Meeting (TRB ‘17), Washington DC.
- *Abraham, H., C. Lee, S. Brady, C. Fitzgerald, B. Mehler, B. Reimer, and J. F. Coughlin. 2016. Autonomous Vehicles, Trust, and Driving Alternatives: A Survey of Consumer Preferences. MIT AgeLab White Paper No. 2016–6. http://agelab.mit.edu/system/files/2018-12/2017_TRB_Abraham.pdf.
- *Abraham, H., B. Reimer, B. Seppelt, C. Fitzgerald, B. Mehler, and J. F. Coughlin. 2017. “Consumer Interest in Automation: Preliminary Observations Exploring a Year’s Change.” http://agelab.mit.edu/sites/default/files/MIT%20-%20NEMPA%20White%20Paper%20FINAL.pdf.
- *Acheampong, R. A., and F. Cugurullo. 2019. “Capturing the Behavioural Determinants behind the Adoption of Autonomous Vehicles: Conceptual Frameworks and Measurement Models to Predict Public Transport, Sharing and Ownership Trends of Self-Driving Cars.” Transportation Research Part F 62: 349–375.
- *Adnan, N., S. M. Nordin, M. A. Bin Bahruddin, and M. Ali. 2018. “How Trust Can Drive Forward the User Acceptance to the Technology? In-Vehicle Technology for Autonomous Vehicle.” Transportation Research Part A: Policy and Practice 118: 819–836.
- Ajzen, I., and M. Fishbein. 2005. “The Influence of Attitudes on Behavior.” In The Handbook of Attitudes, edited by D. Albarracín, B. T. Johnson and M. P. Zanna, 173–222, Mahwah, NJ: Lawrence Erlbaum Associates.
- *Alessandrini, A., R. Alfonsi, P. Delle Site, and D. Stam. 2014. “Users’ Preferences towards Automated Road Public Transport: Results from European Surveys.” Transportation Research Procedia 3:139–144.
- *Alessandrini, A., P. Delle Site, D. Stam, V. Gatta, E. Marucci, and Q. Zhang. 2016. “Using Repeated-Measurement Preference Data to Investigate Users’ Attitudes Towards Automated Buses Within Major Facilities.” In Advances in Systems Science. ICSS 2016. Advances in Intelligent Systems and Computing, J. Świątek and J. Tomczak, vol 539. Cham: Springer.
- Alfonzo, M. A. A. 2005. “To Walk or Not to Walk? the Hierarchy of Walking Needs.” Environment and Behavior 37 (6): 808–836.
- *Anania, E. C., S. Rice, N. W. Walters, M. Pierce, S. R. Winter, and M. N. Milner. 2018. “The Effects of Positive and Negative Information on Consumers’ Willingness to Ride in a Driverless Vehicle.” Transport Policy 72: 218–224.
- Bagozzi, R. P. and K. H. Lee. 1999. “Consumer Resistance to, and Acceptance, of Innovations.” Advances in Consumer Research 26: 218–225.
- Banks, V. A., A. Eriksson, J. O’Donoghue, and N. A. Stanton. 2018. “Is Partially Automated Driving a Bad Idea? Observations from an On-Road Study.” Applied Ergonomics 68: 138–145.
- *Bansal, P., K. M. Kockelman, and A. Singh. 2016. “Assessing Public Opinions of and Interest in New Vehicle Technologies: An Austin Perspective.” Transportation Research Part C 67: 1–14.
- *Bansal, P., and K. M. Kockelman. 2017. “Forecasting Americans Long-Term Adoption of Connected and Autonomous Vehicle Technologies.” Transportation Research Part A: Policy and Practice 95: 49–63.
- *Bansal, P., and K. M. Kockelman. 2018. “Are we Ready to Embrace Connected and Self-Driving Vehicles? A Case Study of Texans.” Transportation 45 (2): 641–675.
- *Bazilinskyy, P., M. Kyriakidis, and J. De Winter. 2015. “An International Crowdsourcing Study into People’s Statements on Fully Automated Driving.” Procedia Manufacturing 3: 2534–2542.
- *Bennett, R., R. Vijaygopal, and R. Kottasz. 2019. “Willingness of People with Mental Health Disabilities to Travel in Driverless Vehicles.” Journal of Transport & Health 12: 1–12.
- *Berliner, R. M., S. Hardman, and G. Tal. 2019. “Uncovering Early Adopters’ Perceptions and Purchase Intentions of Automated Vehicles: Insights from Early Adopters of Electric Vehicles in California.” Transportation Research Part F 60: 712–722.
- *Böhm, P., M. Kocur, M. Firat, and D. Isemann. 2017. “Which Factors Influence Attitudes Towards Using Autonomous Vehicles?” In Proceedings of the 9th International Conference on Automotive User Interfaces and Interactive Vehicular Applications Adjunct (AutomotiveUI ‘17), New York.
- *Bloom, C., J. Tan, J. Ramjohn, and L. Bauer. 2017. “Self-Driving Cars and Data Collection: Privacy Perceptions of Networked Autonomous Vehicles.” In Proceedings of the Thirteenth Symposium on Usable Privacy and Security (SOUPS), Santa Clara, CA, 12–14 July, 357–375. Berkeley, CA: USENIX Association.
- *Brell, T., R. Philipsen, and M. Ziefle. 2019. “Suspicious Minds?: User Perceptions of Autonomous and Connected Driving.” Theoretical Issues in Ergonomics Science 20 (3): 301. doi: 10.1080/1463922X.2018.1485985.
- *Brell, T., R. Philipsen, and M. Ziefle. 2019. “sCARy! Risk Perceptions in Autonomous Driving: The Influence of Experience on Perceived Benefits and Barriers.” Risk Analysis: An Official Publication of the Society for Risk Analysis 39 (2): 342–357.
- *Brinkley, J., B. Posadas, J. Woodward, and J. E. Gilbert. 2017. “Opinions and Preferences of Blind and Low Vision Consumers Regarding Self-Driving Vehicles. Results of Focus Group Discussions.” Paper Presented at ASSETS’17, Oct 29–Nov. 1, Baltimore, MD.
- *Buckley, L., S. A. Kaye, and A. K. Pradhan. 2018. “Psychological Factors Associated with Intended Use of Automated Vehicles: A Simulated Driving Study.” Accident Analysis & Prevention 115: 202–208.
- *Chaudhry, A., P. Liu, A. Hussain, and I. Sanaullah. 2018. “Safety Perceptions of Self-Driving cars: A Survey Study in China and Pakistan.” In Advances in Human Aspects of Transportation. AHFE 2018. Advances in Intelligent Systems and Computing, edited by N. Stanton, Vol 786. Cham: Springer.
- *Cho, Y., J. Park, and E. S. Jung. 2017. “Technology Acceptance Modeling Based on User Experience for Autonomous Vehicles.” Journal of Ergonomics Society Korea 36: 87–108.
- *Choi, J. K. and Y. G. Ji. 2015. “Investigating the Importance of Trust on Adopting an Autonomous Vehicle.” International Journal of Human–Computer Interaction 31 (10): 692–702.
- Claybrook, J. and S. Kildare. 2018. “Autonomous Vehicles: No Driver…No Regulation?” Science (New York, N.Y.) 361 (6397): 36–37.
- *Cunningham, M. L., S. A. Ledger, and M. Regan. 2018. “A Survey of Public Opinion on Automated Vehicles in Australia and New Zealand.” Paper presented at 28th ARRB International Conference – Next Generation Connectivity, Brisbane, Queensland.
- *Dai, D. and D. Howard. 2013. “Public Perceptions of Self-Driving Cars: The Case of Berkeley, California.” Paper Presented at the 93rd Annual Meeting TRB, Washington, DC. http://www.danielledai.com/academic/howard-dai-selfdrivingcars.pdf.
- *Daziano, R., M. Sarrias, and B. Leard. 2017. “Are Consumers Willing to Let Their Cars Drive for Them? Analyzing Responses to Autonomous Vehicles.” Transportation Research Part C: Emerging Technologies 78: 150–164.
- *Dekker, M. J. 2017. “Riding a Self-Driving Bus to Work: Investigating How Travelers Perceive ADS-DVs on the Last Mile.” https://repository.tudelft.nl/islandora/object/uuid%253Ac9773355-d4a3-4063-9799-11343c625c01?collection=education.
- Devine-Wright, P. 2008. “Reconsidering Public Acceptance of Renewable Energy Technologies: A Critical Review.” In Taking Climate Change Seriously: A Low Carbon Future for the Electricity Sector, edited by T. Jamasb, M. Grubb, and M. Pollitt, 443–461. Cambridge: Cambridge University Press.
- De Winter, J. C. F., R. Happee, M. H. Martens, and N. A. Stanton. 2014. “Effects of Adaptive Cruise Control and Highly Automated Driving on Workload and Situation Awareness: A Review of Empirical Evidence.” Transportation Research Part F: Traffic Psychology and Behavior 27: 196–217.
- *Diels, C. and J. E. Bos. 2016. “Self-Driving Carsickness.” Applied Ergonomics 53: 374–382.
- *Diels, C., J. E. Bos, K. Hottelart, and P. Reilhac. 2016. “Motion Sickness in Automated Vehicles: The Elephant in the Room.” In Road Vehicle Automation 3, Lecture Notes in Mobility, 121–129. Cham: Springer International Publishing Switzerland.
- *Diepold, K., A. Riener, K. Götzl, and A. K. Frison. 2017. “Automated Driving: Acceptance and Chances for Elderly People.” Paper presented at Adjunct Proceedings of the 9th International ACM Conference on Automotive User Interfaces and Interactive Vehicular Applications (AutomotiveUI ‘17), September 24–27, Oldenburg, Germany.
- *Distler, V., C. Lallemand, and T. Bellet. 2018. “Acceptability and Acceptance of Autonomous Mobility on Demand: The Impact of an Immersive Experience.” In Proceedings of the 2018 CHI Conference on Human Factors in Computing Systems, 612. New York: ACM.
- *Dong, X., M. DiScenna, and E. Guerra. 2017. “Transit User Perceptions of Driverless Busses.” Transportation 46 (1): 35–50.
- *Eden, G., B. Nanchen, R. Ramseyer, and F. Evequoz. 2017. “Expectation and Experience: Passenger Acceptance of Autonomous Public Transportation Vehicles.” In Human-Computer Interaction – INTERACT 2017. Lecture Notes in Computer Science, edited by R. Bernhaupt, G. Dalvi, A. Joshi, K. D. Balkrishan, J. O’Neill, and M. Winckler, vol 10516, 360–363. Cham: Springer.
- Endsley, M. R. 1985a. “Technological Change and Individual Adjustment.” Paper presented at the Human Factors Society 29th Annual Meeting, Santa Monica, CA.
- Endsley, M. R. 1985b. “The Toll of Technology on Job Commitment and an Implementation to Prevent it.” Proceedings of the Human Factors and Ergonomics Society Annual Meeting 29 (4): 870–874.
- Fernández-Heredia, Á., S. Jara-Díaz, and A. Monzón. 2016. “Modelling Bicycle Use Intention: The Role of Perceptions.” Transportation 43: 1–23.
- *Földes, D., C. Csiszar, and A. Zarkeshev. 2019. “User Expectations Towards Mobility Services Based on Autonomous Vehicle.” Paper Presented at Conference: 8th International Scientific Conference (CMDTUR 2018), October 4–5, 7–14.
- Fraedrich, E., S. Beiker, and B. Lenz. 2015. “Transition Pathways to Fully Automated Driving and Its Implications for the Sociotechnical System of Automobility.” European Journal of Futures Research 3 (1): 1–11.
- *Fraedrich, E. and B. Lenz. 2014. “Automated Driving: Individual and Societal Aspects Entering the Debate.” Transportation Research Record: Journal of the Transportation Research Board 2416 (1): 64–72.
- *Fraedrich, E. and B. Lenz. 2016. “Taking a Drive, Hitching a Ride: Autonomous Driving and Car Usage.” In Autonomous Driving, edited by M. Maurer, J. Gerdes, B. Lenz, and H. Winner. Berlin: Springer.
- *Gilbert, J. E. and S. B. Daily. 2018. “A Survey of Visually Impaired Consumers about Self-Driving Vehicles.” Paper presented at 33rd Annual International Technology and Persons with Disabilities Conference Scientific/Research Proceedings, San Diego.
- *Greaves, S., B. Smith, T. Arnold, D. Olaru, and A. T. Collins. 2018. “Autonomous Vehicles Down Under: An Empirical Investigation of Consumer Sentiment.” Paper presented at Australasian Transport Research Forum 2018 Proceedings 30 October–1 November, Darwin, Australia.
- Grotenhuis, J. W., B. W. Wiegmans, and P. Rietveld. 2007. “The Desired Quality of Integrated Multimodal Travel Information in Public Transport: Customer Needs for Time and Effort Savings.” Transport Policy 14 (1): 27–38.
- *Gurumurthy, K. M. 2017. “Perceptions and Preferences of Autonomous and Shared Autonomous Vehicles: A Focus on Dynamic Ride-Sharing.” https://repositories.lib.utexas.edu/handle/2152/63553.
- *Haboucha, C. J., R. I. Ishaq, and Y. Shiftan. 2017. “User Preferences regarding Autonomous Vehicles.” Transportation Research Part C 78: 37–49.
- *Hardman, S., R. Berliner, and G. Tal. (2019). “Who Will Be the Early Adopters of Automated Vehicles? Insights from a Survey of Electric Vehicle Owners in the United States.” Transportation Research Part D: Transport & Environment 71: 248–264.
- *Hartwich, F., M. Beggiato, and J. F. Krems. 2018. “Driving Comfort, Enjoyment and Acceptance of Automated Driving: Effects of Drivers’ Age and Driving Style Familiarity.” Ergonomics 61 (8): 1017–1032.
- *Hartwich, F., C. Witzlack, M. Beggiato, and J. Krems. (2019). “The First Impression Counts – a Combined Driving Simulator and Test Track Study on the Development of Trust and Acceptance of Highly-Automated Driving.” Transportation Research Part F: Traffic Psychology and Behavior (In Press). https://www.sciencedirect.com/science/article/abs/pii/S1369847816306489
- *Hassan, H. M., M. R. Ferguson, S. Razavi, and B. Vrkljan. 2019. “Factors That Influence Older Canadians’ Preferences for Using Autonomous Vehicle Technology: A Structural Equation Analysis.” Transportation Research Record 2673 (1):469–480.
- Haspiel, J., N. Du, X. J. Yang, D. Tilbury, A. Pradhan, and L. P. Robert. 2018. “Explanations and Expectations: Trust Building in Automated Vehicles.” In Proceedings of the Companion of the 2018 ACM/IEEE International Conference on Human- Robot Interaction (HRI 2018), Chicago, IL.
- Heikoop, D. D., J. C. F. De Winter, B. Van Arem, and N. A. Stanton. 2016. “Psychological Constructs in Driving Automation: A Consensus Model and Critical Component on Construct Proliferation.” Theoretical Issues in Ergonomics Science 17 (3): 284–303.
- Hengstler, M., E. Enkel, and S. Duelli. 2016. “Applied Artificial Intelligence and Trust: The Case of Autonomous Vehicles and Medical Assistance Devices.” Technological Forecasting & Societal Change 105: 105–120.
- *Hewitt, C., I. Politis, T. Amanatidis, and A. Sarkar. 2019. “Assessing Public Perception of Self-Driving Cars: The Autonomous Vehicle Acceptance Model.” Paper presented at 24th International Conference on Intelligent User Interfaces (IUI ‘19), March 17–20, Marina del Ray, CA.
- Hoff, K. A., and M. Bashir. 2015. “Trust in Automation: Integrating Empirical Evidence on Factors That Influence Trust.” Human Factors: The Journal of the Human Factors and Ergonomics Society 57 (3): 407–434.
- *Hohenberger, C., M. Sporrle, and I. M. Welpe. 2016. “How and Why Do Men and Women Differ in Their Willingness to Use Automated Cars? The Influence of Emotions across Different Age Groups.” Transportation Research Part A 94: 374–385.
- *Hudson, J., M. Orviska, and J. Hunady. 2019. “People’s Attitudes to Autonomous Vehicles.” Transportation Research Part A: Policy & Practice 121: 164–176.
- *Hulse, L. M., H. Xie, and E. R. Galea. 2018. “Perceptions of Autonomous Vehicles: Relationships with Road Users, Gender and Age.” Safety Science 102: 1–13.
- Ibrahim, M. F. 2003. “Car Ownership and Attitudes towards Transport Modes of Shopping Purposes in Singapore.” Transportation 30 (4): 435–457.
- *Jardim, A. S., A. M. Quartulli, and S. V. Casley. 2013. “A Study of Public Acceptance of Autonomous Cars.” https://digitalcommons.wpi.edu/cgi/viewcontent.cgi?article=3595&context=iqp-all.
- *Jing, P., H. Huang, B. Ran, F. Zhan, and Y. Shi. 2019. “Exploring the Factors Affecting Mode Choice Intention of Autonomous Vehicle Based on an Extended Theory of Planned Behavior: A Case Study in China.” Sustainability 11 (4): 1120–1155.
- *Kaan, J. 2017. “User Acceptance of Autonomous Vehicles: Factors & Implications.” https://repository.tudelft.nl/islandora/object/uuid%3Addc5f88d-5a15-4312-ac63-edbf5f977cc1.
- Kalra, N. and S. M. Paddock. 2016. “Driving to Safety: How Many Miles of Driving Would It Take to Demonstrate Autonomous Vehicle Reliability?” Transportation Research Part A 94: 182–193.
- *Kaur, K., and G. Rampersad. 2018. “Trust in Driverless Cars: Investigating Key Factors Influencing the Adoption of Driverless Cars.” Journal of Engineering and Technology Management 48: 87–96.
- *Kelkel, R. 2015. “Predicting Consumers Intention to Purchase Fully Autonomous Driving Systems: Which Factors Drive Acceptance?” https://repositorio.ucp.pt/bitstream/10400.14/17252/1/Reiner_Kelkel_152112329.pdf.
- *Kohl, C., M. Knigge, G. Baader, M. Böhm, and H. Krcmar. 2018. “Anticipating Acceptance of Emerging Technologies Using Twitter: The Case of Self-Driving Cars.” Journal or Business Economics 88 (5): 317–642.
- *König, M., and L. Neumayr. 2017. “Users’ Resistance towards Radical Innovations: The Case of the Self-Driving Car.” Transportation Research Part F 44: 42–52.
- *Krueger, R., T. H. Rashidi, and J. M. Rose. 2016. “Preferences for Shared Autonomous Vehicles.” Transportation Research Part C 69: 343–355.
- *Kyriakidis, M., R. Happee, and J. C. F. De Winter. 2015. “Public Opinion on Automated Driving: Results of an International Questionnaire among 5,000 Respondents.” Transportation Research Part F: Traffic Psychology and Behavior 32: 127–140.
- *Langdon, P., I. Politis, M. Bradley, L. Skrypchuk, A. Mouzakitis, and J. Clarkson. 2017. “Obtaining Design Requirements from the Public Understanding of Driverless Technology.” In Advances in Intelligent Systems and Computing, edited by N. Stanton, 749–759. Cham: Springer.
- *Lavieri, P. S., V. M. Garikapati, C. R. Bhat, R. M. Pendyala, S. Astroza, and F. F. Dias. 2017. “Modeling Individual Preferences for Ownership and Sharing of Autonomous Vehicle Technologies.” Transportation Research Record: Journal of the Transportation Research Board 2665 (1): 1–10.
- *Leicht, T., A. Chtourou, and K. B. Youssef. 2018. “Consumer Innovativeness and Intentioned Autonomous Car Adoption.” Journal of High Technology Management Research 29 (1): 1–11.
- Lee, J. D., and K. A. See. 2004. “Trust in Automation: Designing for Appropriate Reliance.” Human Factors 46 (1): 50–80.
- *Lee, C., C. Ward, M. Raue, L. D’Ambrosio, and J. F. Coughlin. 2017. “Age Differences in Acceptance of Self-Driving Cars: A Survey of Perceptions and Attitudes.” In Human Aspects for IT for the Aged Population. Aging, Design and User Experience. ITAP 2017, edited by J. Zhou and G. Salvendy, vol. 10297, 3–13. Cham: Springer.
- *Lee, Y. C., and J. H. Mirman. 2018. “Parents Perspectives on Using Autonomous Vehicles to Enhance Children’s Mobility.” Transportation Research Part C: Emerging Technologies 96: 415–431.
- *Lilijamo, T., H. Limatainen, and M. Pöllänen. 2018. “Attitudes and Concerns on Automated Vehicles.” Transportation Research Part F 59: 24–44.
- *Liu, P., R. Yang, and Z. Xu. 2019. “Public Acceptance of Fully-Automated Driving: Effects of Social Trust and Risk/Benefit Perceptions.” Risk Analysis 39 (2): 326–341.
- *Liu, P., Q. Guo, F. Ren, L. Wang, and Z. Xu. 2019. “Willingness to Pay for Self-Driving Vehicles: Influence of Demographics and Psychological Factors.” Transportation Research Part C: Emerging Technologies 100: 306–317.
- *Lustgarten, P., and S. Le Vine. 2018. “Public Priorities and Consumer Preferences for Selected Attributes of Automated Vehicles.” Journal of Modern Transportation 26 (1): 72–79.
- *Madigan, R., T. Louw, M. Dziennus, T. Graindorge, E. Ortega, M. Graindorge, and N. Merat. 2016. “Acceptance of Automated Road Transport Systems (ARTS): An Adaptation of the UTAUT Model.” Transport Research Procedia 14: 2217–2226.
- *Madigan, R., T. Louw, M. Wilbrink, A. Schieben, and N. Merat. 2017. “What Influences the Decision to Use Automated Public Transport? Using UTAUT to Understand Public Acceptance of Automated Road Transport Systems.” Transportation Research Part F: Traffic Psychology and Behavior 50: 55–64.
- Maslow, A. H. 1954. Motivation and Personality. New York: Harper and Row.
- *Medina, K. F. and R. Jenkins. 2017. “GATEway: Public Perceptions of a Last-Mile Driverless Shuttle.” https://gateway-project.org.uk/wp-content/uploads/2018/06/D3.7_TRL-Workshop-Findings-Report.pdf.
- Meijkamp, R., and R. Theunissen. 1996. “Carsharing: Consumer Acceptance and Changes in Mobility Behavior.” In Proceedings of the European Transport Conference 1996, Delft, The Netherlands.
- *Menon, N. 2015. “Consumer Perception and Anticipated Adoption of Autonomous Vehicle Technology: Results from Multi-Population Surveys.” https://scholarcommons.usf.edu/cgi/viewcontent.cgi?referer= https://www.google.com/&httpsredir=1&article=7188&context=etd.
- Milakis, D., B. Van Arem, and B. Van Wee. 2017. “Policy and Society Related Implications of Automated Driving: A Review of Literature and Directions for Future Research.” Journal of Intelligent Transportation Systems 21 (4): 324–348.
- Moher, D., A. Liberati, J. Tetzlaff, D. G. Altman, and The PRISMA Group. 2009. “Preferred Reporting Items for Systematic Reviews and Meta-Analyses: The PRISMA Statement.” PLoS Medicine 6 (7): e1000097. doi: 10.1371/journal.pmed.1000097.
- *Molnar, L. J., L. H. Ryan, A. K. Pradhan, D. W. Eby, R. M. St. Louis, and J. S. Zakrajsek. 2018. “Understanding Trust and Acceptance of Automated Vehicles: An Exploratory Simulator Study of Transfer of Control between Automated and Manual Driving.” Behavior 58: 319–328.
- *Moták, L., E. Neuville, P. Chambres, F. Marmoiton, F. Monéger, F. Coutarel, and M. Izaute. 2017. “Antecedent Variables of Intentions to Use an Autonomous Shuttle: Moving beyond TAM and TPB?” Revue Européenne de Psychologie Appliquée/European Review of Applied Psychology 67 (5): 269–278.
- *Nazari, F., M. Noruzoliaee, and A. K. Mohammadian. 2018. “Shared versus Private Mobility: Modeling Public Interest in Autonomous Vehicles Accounting for Latent Attitudes.” Transportation Research Part C: Emerging Technologies 97: 456–477.
- *Nees, M. A. 2016. “Acceptance of Self-Driving Cars: An Examination of Idealized Versus Realistic Portrayals with a Self-Driving Car Acceptance Scale 潐. Proceedings of the Human Factors and Ergonomics Society 60 (1): 1449–1453.
- Nieuwenhuijsen, J., G. H. De Almeida Correia, D. Milakis, B. Van Arem, and E. Van Daalen. 2018. “Towards a Quantitative Method to Analyze Long-Term Innovation Diffusion of Automated Vehicles Technology Using System Dynamics.” Transportation Research Part C 86: 300–327.
- *Nielsen, S. T. A., and S. Haustein. 2018. “On Sceptics and Enthusiasts: What Are the Expectations towards Self-Driving Cars?” Transport Policy 66: 49–55.
- Nordhoff, S., B. Van Arem, and R. Happee. 2016. “A Conceptual Model to Explain, Predict, and Improve User Acceptance of Driverless 4P Vehicles.” Transportation Research Record: Journal of the Transportation Research Board 2602 (1): 60–67.
- *Nordhoff, S., J. De Winter, R. Madigan, N. Merat, B. Van Arem, and R. Happee. 2018. “User Acceptance of Automated Shuttles in Berlin-Schöneberg: A Questionnaire Study.” Transportation Research Part F: Traffic Psychology and Behavior 58: 843–854.
- *Nordhoff, S., J. De Winter, M. Kyriakidis, B. Van Arem, and R. Happee. 2018. “Acceptance of Driverless Vehicles: Results from a Large Cross-National Questionnaire Study.” Journal of Advanced Transportation 2018: 1–22. Article ID 5382192.
- *Nordhoff, S., J. De Winter, W. Payre, B. Van Arem, and R. Happee. 2019. “What Impressions Do Users Have after a Ride in an Automated Shuttle? An Interview Study.” Transportation Research Part F: Traffic Psychology and Behavior 63: 252–269.
- *Nordhoff, S., R. Madigan, R. Happee, V. B. Arem, and N. Merat. 2019. “Structural equation modelling discloses interrelations between predictors of automated vehicle acceptance.” Under review.
- Osswald, S., D. Wurhofer, S. Trosterer, E. Beck, and M. Tscheligi. 2012. Predicting Information Technology Usage in the Car: Towards a Car Technology Acceptance Model. In Proceedings of the 4th International Conference on Automotive User Interfaces and Interactive Vehicular Applications, 51–58. New York, NY: ACM.
- *Overakker, B. 2017. “The Social Acceptance of Automated Driving Systems: Safety Aspects.” https://repository.tudelft.nl/islandora/object/uuid%3A31159f82-e33d-4124-a5a2-2b605974870e.
- *Pakusch, C. and P. Bossauer. 2017. “User Acceptance of Fully Autonomous Public Transport.” In Proceedings of the 14th International Joint Conference on e-Business and Telecommunications (ICETE 2017), vol. 2: ICE-B, 52–60.
- *Panagiotopoulos, I., and G. Dimitrakopoulos. 2018. “An Empirical Investigation on Consumers’ Intentions towards Autonomous Driving.” Transportation Research Part C: Emerging Technologies 95: 773–784.
- *Payre, W., J. Cestac, and P. Delhomme. 2014. “Intention to Use a Fully Automated Car: Attitudes and a Priori Acceptability.” Transportation Research Part F: Traffic Psychology and Behavior 27: 252–263.
- *Peng, L., Z. Yawen, and H. Zhen. 2019. “The Effect of Population Age on the Acceptable Safety of Self-Driving Vehicles.” Reliability Engineering & System Safety 185: 341–347.
- *Penmetsa, P., E. K. Adanu, D. Wood, T. Wang, and S. L. Jones. 2019. “Perceptions and Expectations of Autonomous Vehicles: A Snapshot of Vulnerable Road User Opinion.” Technological Forecasting & Social Change 143: 9–13.
- Petkewich, R. 2005. “Taking the Mass out of Transit.” Environmental Science and Technology 39: 61–62.
- *Pettigrew, S., L. Fritschi, and R. Norman. 2018. “The Potential Implications of Autonomous Vehicles in and around the Workplace.” International Journal of Environmental Research and Public Health 15: 1–10.
- *Pettigrew, S., Z. Talati, and R. Norman. 2018. “The Health Benefits of Autonomous Vehicles: Public Awareness and Receptivity in Australia.” Australian and New Zealand Journal of Public Health 42 (5): 480–483.
- *Pettigrew, S., L. M. Dana, and R. Norman. 2019. “Clusters of Potential Autonomous Vehicles Users according to Their Propensity to Use Individual versus Shared Vehicles.” Transport Policy 76: 13–20.
- *Piao, J., M. McDonald, N. Hounsell, M. Graindorge, T. Graindorge, and N. Malhene. 2016. “Public Views towards Implementation of Automated Vehicles in Urban Areas.” Transportation Research Procedia 14: 2168–2177.
- *Portouli, E., G. Karaseitanidis, P. Lytrivis, A. Amditis, O. Raptis, and C. Karaberi. 2017. “Public Attitudes Towards Autonomous Mini Buses Operating in Real Conditions in a Hellenic City.” Proceedings of the 2017 IEEE Intelligent Vehicles Symposium, 71–576. Los Angeles, CA.
- *Raue, M., L. A. D’Ambrosio, C. Ward, C. Lee, C. Jacquillat, and J. F. Coughlin. 2019. “The Influence of Feelings While Driving Regular Cars on the Perception and Acceptance of Self-Driving Cars.” Risk Analysis: An Official Publication of the Society for Risk Analysis 39 (2): 358–374.
- *Regan, M., M. Cunningham, V. Dixit, T. Horberry, A. Bender, K. Weeratunga, S. Cratchley, L. Dalwood, D. Muzorewa, and A. Hassan. 2017. “Preliminary Findings from the First Australian National Survey of Public Opinion about Automated and Driverless Vehicles.” Transportation https://advi.org.au/media-centre/preliminary-findings-from-the-first-australiannational-survey-of-public-opinion-about-automated-and-driverless-vehicles/.
- Rezvani, Z., J. Jansson, and J. Bodin. 2015. “Advances in Consumer Electric Vehicle Adoption Research: A Review and Research Agenda.” Environment 34: 122–136.
- *Rödel, C., S. Stadler, A. Meschtscherjakov, and M. Tscheligi. 2014. “Towards Autonomous Cars: The Effect of Autonomy Levels on Acceptance and User Experience.” Paper presented at 6th International Conference on Automotive User Interfaces and Interactive Vehicular Applications (AutomotiveUI ‘14), New York, 1–8.
- Rogers, E. M. 1983. Diffusion of Innovations. New York: The Free Press.
- Rotter, J. B., and D. J. Hochreich. 1975. Personality. Glenview, IL: Scott, Foresman.
- Rudin-Brown, C. M., and H. A. Parker. 2004. “Behavioral Adaptation to Adaptive Cruise Control (ACC): Implications for Preventive Strategies.” Transportation Research Part F 7 (2): 59–76.
- SAE International. 2018. “Surface Vehicle Recommended Practice. (R) Taxonomy and Definitions for Terms Related to Driving Automation Systems for On-Road Motor Vehicles.” https://saemobilus.sae.org/content/j3016_201806.
- *Salonen, A. O. 2018. “Passengers’ Subjective Traffic Safety, in-Vehicle Security and Emergency Management in the Driverless Shuttle Bus in Finland.” Transport Policy 61: 106–110.
- *Sanaullah, I., A. Hussain, A. Chaudhry, K. Case, and M. Enoch. 2016. “Autonomous Vehicles in Developing Countries: A Case Study on User’s View Point in Pakistan.” Advances in Human Aspects of Transportation 484: 561–569.
- *Sanbonmatsu, D. A., D. L. Strayer, Y. Zhenghui, F. Biondi, and J. M. Cooper. 2018. “Cognitive Underpinnings of Beliefs and Confidence in Beliefs about Fully Automated Vehicles.” Transportation Research Part F 55: 114–122.
- Sanguinetti, A., K. Kurani, and B. Ferguson. 2019. “Is it OK Getting in a Car with a Stranger? Risks and Benefits of Ride-Pooling in Shared Automated Vehicles.” https://cloudfront.escholarship.org/dist/prd/content/qt1cb6n6r9/qt1cb6n6r9.pdf?t=pppvf3
- *Sauders, D. and N. Charness. 2016. “Challenges of Older Drivers’ Adoption of Advanced Assistance Systems and Autonomous Vehicles.” In Human Aspects of IT for the Aged Population. Healthy and Active Aging. ITAP 2016. Lecture Notes in Computer Science, edited by J. Zhou and G. Salvendy, vol 9755, 428–440. Cham: Springer.
- *Schoettle, B. and M. Sivak. 2014. “A Survey of Public Opinion about Autonomous and Self-Driving Vehicles in the U.S., the U.K., and Australia.” Transportation Research Record: Journal of the Transportation Research Board.
- *Schoettle, B. and M. Sivak. 2015. “Motorists’ Preferences for Different Levels of Vehicle Automation.” https://www.gomobilemichigan.org/cm/dpl/downloads/content/99/UM_Study_-_Motorists_Pref_for_Levels_of_Vehicle_Automotion.pdf.
- Schwarz, A., W. W. Chin, R. Hirschheim, and C. Schwarz. 2014. “Toward a Process-Based View of Information Technology Acceptance.” Journal of Information Technology 29 (1): 73–96.
- *Sener, I. N., J. Zmud, and T. Williams. 2019. “Measures of Baseline Intent to Use Automated Vehicles: A Case Study of Texan Cities.” Transportation Research Part F: Traffic Psychology & Behaviour 62: 66–77.
- *Seuwou, P., E. Banissi, G. Ubakanma, M. S. Sharif, and A. Healey. 2017. “Actor-Network theory as a Framework to Analyse Technology Acceptance Model’s External Variables: The Case of Autonomous Vehicles.” In Global Security, Safety and Sustainability - The Security Challenges of the Connected World. ICGS3 2017. Communications in Computer and Information Science, edited by H. Jahankhani, Vol 630, 305–320. Cham: Springer.
- *Shabanpour, R., S. N. D. Mousavi, N. Golshani, J. Auld, and A. Mohammadian. 2017. “Consumer Preferences of Electric and Automated Vehicles.” Paper presented at 5th IEEE International Conference on Models and Technologies for Intelligent Transportation Systems (MT-ITS), Naples, Italy.
- *Shabanpour, R., N. Golshani, A. Shamshiripour, and A. K. Mohammadian. 2018. “Eliciting Preferences for Adoption of Fully Autonomous Vehicles Using Best-Worst Analysis.” Transportation Research Part C 93: 463–478.
- *Shin, K. J., and M. Shunsuke. 2017. “Consumer Demand for Fully Automated Driving Technology: Evidence from Japan.” https://www.rieti.go.jp/jp/publications/dp/17e032.pdf.
- *Site, P. D., F. Filippi, and G. Giustiniani. 2011. “Users’ Preferences towards Innovative and Conventional Public Transport.” Procedia Social and Behavioral Sciences 20: 906–915.
- Sovacool, B. K., and J. Axsen. 2018. “Functional, Symbolic and Societal Frames for Automobility: Implications for Sustainability Transitions.” Transportation Research Part A: Policy & Practice 118: 730–746.
- *Spurlock, C. A., J. Sears, G. Wong-Parodi, V. Walker, M. Taylor, A. Duvall, A. Gopal, and A. Todd. (2019). “Describing the Users: Understanding Adoption of and Interest in Shared, Electrified, and Automated Transportation in the San Francisco Bay Area.” Transportation Research Part D: Transport & Environment 71: 283–301.
- Stanton, N. A., and M. S. Young. 2000. “A Proposed Psychological Model of Driving Automation.” Theoretical Issues in Ergonomics Science 1 (4): 315–331.
- Stern, P. C. 2000. “New Environmental Theories: Toward a Coherent Theory of Environmentally Significant Behavior.” Journal of Social Issues 56 (3): 407–424.
- Steg, L., C. Vlek, and G. Slotegraaf. 2001. “Instrumental-Reasoned and Symbolic-Affective Motives for Using a Motor Car.” Transportation Research Part F 4 (3): 151–169.
- Steg, L., and C. Vlek. 2009. “Encouraging Pro-Environmental Behavior: An Integrative Review and Research Agenda.” Journal of Environmental Psychology 29 (3): 309–317.
- Steg, L., J. W. Bolderdijk, K. Keizer, and G. Perlaviciute. 2014. “An Integrated Framework for Encouraging Pro-Environmental Behavior: The Role of Values, Situational Factors and Goals.” Journal of Environmental Psychology 38: 104–115.
- Schwartz, S. H. 1992. “Universals in the Content and Structure of Values: Theoretical Advances and Empirical Tests in 20 Countries.” Advances in Experimental Social Psychology 25:1–65.
- Taylor, S., and P. A. Todd. 1995. “Understanding Information Technology Usage: A Test of Competing Models.” Information Systems Research 6 (2): 144–176.
- *Tennant, C., S. Howard, B. Franks, M. W. Bauer, S. Stares, P. Pansegrau, M. Stysko-Kunkowska, and A. Cuevas-Badallo. 2016. “Autonomous Vehicles–Negotiating a Place on the Road: A Study on How Drivers Feel about Interacting with Autonomous Vehicles on the Road.” Executive Summary. www.lse.ac.uk/newsAndMedia/PDF/AVs-negotiating-a-place-on-the-road-1110.pdf.
- *TNS Opinion & Social. 2015. “Special Eurobarometer 427 Autonomous systems.” http://ec.europa.eu/public_opinion/archives/ebs/ebs_427_en.pdf.
- *Tussyadiah, I. P., F. J. Zach, and J. Wang. 2017. “Attitudes Towards Autonomous on Demand Mobility System: The Case of Self-Driving Taxi.” In Information and Communication Technologies in Tourism 2017, edited by R. Schegg and B. Stangl. Cham: Springer.
- *Ulahannan, A., R. Cain, G. Dhadyalla, P. Jennings, S. Birrell, M. Waters, and A. Mouzakitis. 2018. “Using the Ideas Café to Explore Trust in Autonomous Vehicles.” In Advances in Human Factors in Communication of Design. AHFE 2018. Advances in Intelligent Systems and Computing, edited by A. Ho, vol 796, 3–14. Cham: Springer.
- Venkatesh, V., M. G. Morris, G. B. Davis, and F. D. Davis. 2003. “User Acceptance of Information Technology: Toward a Unified View.” MIS Quarterly 27: 425–478.
- Venkatesh, V., J. Y. L. Thong, and X. Xu. 2012. “Consumer Acceptance and Use of Information Technology: Extending the Unified Theory of Acceptance and Use of Technology.” MIS Quarterly 36: 157–178.
- Venkatesh, V., J. Y. L. Thong, and X. Xu. 2016. “Unified Theory of Acceptance and Use of Technology: A Synthesis and the Road Ahead.” Journal of the Association for Information Systems 17 (5): 328–376.
- *Wang, K., and G. Ankar. 2019. “Factors Affecting the Adoption of Autonomous Vehicles for Commute Trips: An Analysis with the 2015 and 2017 Puget Sound Travel Surveys.” Transportation Research Record: Journal of the Transportation Research Board 2673 (2): 13–25.
- *Ward, C., M. Raue, C. Lee, L. D’Ambrosio, and J. F. Coughlin. 2017. “Acceptance of Automated Driving Across Generations: The Role of Risk and Benefit Perception, Knowledge, and Trust.” InHuman-Computer Interaction. User Interface Design, Development and Multimodality. HCI 2017. Lecture Notes in Computer Science, edited by M. Kurosu, vol. 10271, 254–266. Cham: Springer.
- *Webb, J., C. Wilson, and T. Kularatne. 2019. “Will People Accept Shared Autonomous Electric Vehicles? a Survey before and after Receipt of the Costs and Benefits.” Economic Analysis and Policy 61: 118–135.
- *Wien, F. J. J. 2019. “An Assessment of the Willingness to Choose a Self-Driving Bus for an Urban Trip: A Public Transport User’s Perspective.” https://repository.tudelft.nl/islandora/object/uuid%3A8064cc17-dc0e-4c0c-9a9c-6efca8564d94.
- *Winter, K., O. Cats, G. H. D A. Correia, and B. van Arem. 2016. “A Stated Choice Experiment on Mode Choice in an Era of Free-Floating Carsharing and Autonomous Vehicles.” Transportation Research Record: Journal of the Transportation Research Board 2542 (1): 75–83.
- *Wintersberger, P., A. K. Frison, and A. Riener. 2018. “Man vs. Machine: Comparing a Fully Automated Bus Shuttle with a Manually Driven Group Taxi in a Field Study.” Paper presented at Adjunct Proceedings of the 10th International ACM Conference on Automotive User Interfaces and Interactive Vehicular Applications (AutomotiveUI ‘18), September 23–25, Toronto, Canada.
- *Woisetschläger. D. M. 2016. “Consumer Perceptions of Automated Driving Technologies: An Examination of Use Cases and Branding Strategies.” In Autonomous Driving, 687–706. Berlin: Springer.
- *Woldemanuel, M. and D. Nguyen. 2018. “Perceived Benefits and Concerns of Autonomous Vehicles: An Exploratory Study of Millennials Sentiments of an Emerging Market.” Research in Transportation Economics 71: 44–53.
- *Wu, J., H. Liao, J. W. Wang, and T. Chen. 2019. “The Role of Environmental Concern in the Public Acceptance of Autonomous Electric Vehicles: A Survey from China.” Transportation Research Part F: Traffic Psychology & Behaviour 60: 37–46.
- *Xu, Z., K. Zhang, H. Min, Z. Wang, X. Zhao, and P. Liu. 2018. “What Drives People to Accept Automated Vehicles? Findings from a Field Experiment.” Transportation Research Part C: Emerging Technologies 95: 320–334.
- *Yap, M. D., G. Correia, and B. Van Arem. 2016. “Preferences of Travelers for Using Automated Vehicles as Last Mile Public Transport of Multimodal Train Trips.” Transportation Research Part A: Policy and Practice 94: 1–16.
- Zhang, B., J. De Winter, S. Varotto, R. Happee, and M. Martens. 2018. “Determinants of Take-Over Time from Automated Driving: A Meta-Analysis of 129 Studies.” Transportation Research Part F: Traffic Psychology and Behaviour 64: 285–307.
- *Zhang, Q., D. Na, L. P. Robert, and X. J. Yang. 2018. “Trust in AVs: The Impact of Expectations and Individual Differences.” Paper Presented at the Conference on Autonomous Vehicles in Society: Building a Research Agenda, May 18–19, East Lansing, MI.
- *Zhang, T., D. Tao, X. Qu, X. Zhang, R. Lin, and W. Zhang. 2019. “The Roles of Initial Trust and Perceived Risk in Public’s Acceptance of Automated Vehicles.” Transportation Research Part C: Emerging Technologies 98: 207–220.
- *Zmud, J. and I. Sener. 2017. “Towards an Understanding of the Travel Behavior Impact of Autonomous Vehicles.” Transportation Research Procedia 25: 2500–2519.