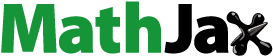
ABSTRACT
We develop a theory for the cascade mixing terms in a moment closure approach to binary active scalar mixing in variable-density turbulence. To address the variable-density complications we apply, as a principle and constraint, the conservation of the probability density function (PDF) through a Fokker–Planck equation with bounded sample space whose attractor is the beta PDF with skewness. Mixing is related to a single-point PDF as a realisability principle to provide mathematically rigorous expressions for the small scale statistics in terms of largescale moments. The problem of the unknown small-scale mixing is replaced with the determination of the drift and diffusion terms of a Fokker–Planck equation in a beta-PDF-convergent stochastic process. We find that realisability of a beta-convergent process requires the mixing time-scale ratio, taken as a constant in passive scalar mixing, to be a function of the mean mass fraction, mean fluid density, the Atwood number, the density-volume correlation and moments of the density field. We develop and compare the new model with direct numerical simulations data of non-stationary homogeneous variable-density turbulence.
1. Introduction
In conventional second moment closure approaches the mixing process is modelled as a drag with a time-scale set from which we derive the same second moment equation in which the mixing terms can be identified as a model for the mixing in a Navier–Stokes-based moment closure approach.
We specifically address the modelling in a moment-based approach to a material mixing cascade in variable-density turbulence (VDT) in which the density field and the hydrodynamics are tightly coupled.
Unlike passive-scalar mixing, mixing between fluids of very different densities exhibits a high degree of skewness even when there are equal amounts of the two pure fluids (in the symmetric initial condition case), see Livescu and Ristorcelli [Citation1,Citation2]. The probability density function (PDF) at the stages of a mixing process by VDT, more general than those given in [Citation2], are given below with data from direct numerical simulations (DNS) from Livescu [Citation3]. For the reader not familiar with this model problem, homogenous Rayleigh–Taylor transition and turbulence, direct numerical simulations are presented with graphics, parameters, and analysis by Livescu and Ristorcelli [Citation1,Citation2] for initially equal amounts of fluids and for lower density ratios, . For DNS of initially unequal amounts of fluids and at higher density ratios, i.e. A = 0.75, the reader is referred to a paper under review [Citation4]. For an excellent description on the flow and its evolution, see Sec. 4 of [Citation1]. Our concern here is with modelling the mixing process.
1.1. Problem statement
The Navier–Stokes-based moment equations for a second moment of the material field is
(1)
(1) where
with v the fluctuating specific volume and ρ the density fluctuation about their respective means. Here the mixing rate is
where d is the dilatation [Citation1,Citation5,Citation6]. The standard phenomenological
model is wrong and there is little we have to guide us in a model of
. One can derive an equation for
[Citation7], but there are too many unclosed terms with new fluid physics.
To model the small scale mixing in terms of large scale quantities we invoke realisability as a modelling principle: we require the mixing process be related to an underlying PDF. We effectively add a conservation law – the conservation of the PDF – to the description of material mixing. We invoke a Fokker–Planck equation (FPE) that allows for a skewed mixing process (Figure ). Beta distributions has been used in assumed PDF methods to describe turbulent mixing in combustion (Girimaji [Citation8] and Fox [Citation9]). Here we invoke, not a beta PDF, but a skewed beta process first derived by Bakosi and Ristorcelli [Citation10].
Figure 1. Time evolution of the density PDF extracted from DNS [Citation2,Citation4] for various Atwood numbers and initial conditions. (Colour online.)
![Figure 1. Time evolution of the density PDF extracted from DNS [Citation2,Citation4] for various Atwood numbers and initial conditions. (Colour online.)](/cms/asset/a405b02e-e76a-476b-9797-b6ccee3623ec/tjot_a_1662030_f0001_oc.jpg)
From the FPE [Citation10] we derive the equations for various second-order moments. In particular, we derive the FPE analog to the Navier–Stokes (NS) version of . The unknown
of the NS problem is thus transferred to specifying the drift and diffusion coefficients of the FPE about which we know considerably more. By this procedure of synthesising second moment equations from the FPE and NSE perspectives, we create a formal mathematical procedure that shows how the small scales are to be slaved to the large scales if the cascade process is realisable. Our goal is a closure model for the cascade using a Fokker Planck equation for the mixing in a second moment equation. Our goal is not a PDF method to predict a PDF to compute in reacting flows; at best this work is a first step in that direction dealing with new mixing phenomena seen in variable density turbulence.
1.2. Outline of the paper
The paper proceeds as follows:
In Section 2 we discuss the peculiar issues of mixing in variable-density turbulence (VDT) using the mathematical structure of the VDT equations. Using the PDFs from the DNS we indicate the unusual skewness features of the VDT mixing process.
In Section 3, we present the model problem – Rayleigh Taylor in a box – and discuss its special features; non-equilibrium issues and skewness of the density and specific volume fields.
Our discussion in Section 4 then turns to some background on the passive scalar-hydrodynamic-cascade time-scale ratio and passive scalar models for mixing. The time-scale ratio will play an important role as a mix-rate metric in variable-density turbulence (VDT). We then further refine the problem goal.
In Section 5 we present the nomenclature peculiar to VDT and present some analytic results that are used in the derivations.
Sections 6 and 7 are the more theoretical sections of the work in which we synthesise information from the perspective of a Fokker–Planck equation with information from Reynolds-averaged moments of Navier–Stokes. After proceeding as far as possible with the mathematics, in Section 7 we add phenomenology, from the DNS, to the modelling problem.
2. Special features of variable-density mixing
The new physics of variable-density mixing can be seen by inspection of, e.g. the instantaneous mass fraction equation of species α, ,
(2)
(2) where
,
, and
are the instantaneous mixture-density, mixture velocity, and diffusive mass fluxes, respectively. The problem described in terms of the mass fraction variables in Equation (Equation2
(2)
(2) ), is highly nonlinear: (1) in the advective terms of the scalar and momentum equations as
, and (2) the diffusive flux,
(unlike if
) depends, very importantly, on
and thus on all components of
.
2.1. Differential acceleration
For binary mixing with densities and
the fluid density is given by
(3)
(3) where A is the Atwood number. The acceleration and advective terms in the mass fraction and momentum equations are
(4)
(4) If
the terms vary considerably for
. This is every different from the passive scalar mixing problem for which
and
. Thus with density varying an order of magnitude in the acceleration terms one can expect some sizable differences from passive scalar mixing.
2.2. Differential diffusion
The diffusive flux in variable-density mixing is represented as
(5)
(5) where the diffusion coefficient
is assumed constant for all species. The diffusive flux of species 1 in binary mixing is
(6)
(6) If
the diffusive flux varies considerably for
. In the binary case one can write the diffusion flux in terms of the mixture-density,
(7)
(7) and the nonlinear nature of the diffusion is clear. This leads to phenomena not seen in the
case as heavy fluid mixing into light is qualitatively different than light mixing into heavy, and the PDF becomes skewed [Citation11] even if it has no initial skewness. This mechanism does not occur in passive scalar mixing and can be seen in the equation for the density skewness given Section 3.4.
In Figure the density PDFs from DNS [Citation3] highlight these new and peculiar features of variable-density mixing. Figure (a) shows a low-Atwood-number mixing (A = 0.05) starting with equal amounts of each pure fluid. The initial conditions and the mixing process are symmetric. Figure (b) shows the Atwood number A = 0.75, reflecting a pure fluid density ratio of 7, with symmetric initial conditions (equal amounts of pure fluids). Immediately we see the generation of skewness in the PDF at high Atwood number. At the first time shown after the initial condition (green line) there is no pure light fluid left while there is plenty of pure heavy fluid, which reflects the earlier observations in [Citation2]: due to inertia the heavy fluid mixes more slowly than the light fluid.
However, this is not simply an Atwood number effect. Comparing the green lines in Figure (c,d) we see that the mean density (or the amount of heavy fluid) plays a role. Observe the different amounts of heavy fluid (blue line, right-hand axis). There is a sizable amount of pure heavy fluid left (green and blue lines) and no light fluid left as is consistent with the high-Atwood inertial argument. However note that in Figure (d) the amount of pure light fluid (green line right-hand side), there is so little heavy fluid that it mixes rapidly. In all cases at late time mixing approaches a passive scalar Gaussian-like process as seen by the single more and more symmetric peak of the distribution.
3. The model mixing problem: Rayleigh–Taylor in a box
The problem we treat is a mixture of two different-density fluids, initially quiescent in a box, driven by the sudden application of a gravitational field. We call this homogeneous variable-density turbulence (HVDT) or homogeneous Rayleigh–Taylor mixing. DNS for this process have been given in [Citation1,Citation2]. The ‘equation of state’ is given by a binary material field as given above in (Equation3(3)
(3) ).
(8)
(8) The Boussinesq, or passive-scalar, mixing problem corresponds to infinitesimal A and r. With increasing A (or r), under the application of a mean pressure gradient, the importance of differential acceleration of the different fluids, seen mathematically above, is demonstrated in the DNS simulations below. The DNS data set [Citation3] of our model problem is at A = 0.05, 0.5, 0.75 corresponding to r = 0.1, 2.0, 6.0 and fluids whose densities are different by a ratio of 1.1, 3, and 7. The subscripts 2, and 1 reflect the heavy and the light fluids, respectively.
The Favre mean mass fraction, , or equivalently, the mean density,
, is a second independent metric defining the problem. For this Rayleigh–Taylor in a box problem the Favre and Reynolds means,
and
, are constant in time.
At t = 0 the PDF of the material field is approximately a double-delta of different heights corresponding to unequal amounts of the two pure fluids. The height of the spikes will be changed in different simulations to study the effects of the mean mass fraction and, importantly, the initial skewness of the PDF. At each density ratio there are three simulations in which the Favre mean mass fraction, , and thus the initial PDF skewnesses, are varied. The DNS database for the symmetric density PDF case is given in [Citation1,Citation2], while Ref. [3] discusses non-symmetric cases.
3.1. Special features of Rayleigh–Taylor in a box
The model problem is a non-equilibrium mixing process that begins as laminar, transitions to a turbulent flow, then decays as the potential energy diving the flow is reduced by the mixing process. The special features of the flow are (1) non-equilibrium and transitional, and (2) highly skewed PDFs at the initial state. The skewness is not monotonically decaying (as in the passive scalar case) and changes sign in some configurations.
3.2. The mixing process is non-equilibrium: a new time-scale
The initial turbulence energy is zero, increases rapidly as transition takes place, peaks in scaled time at , then decays.
In Figure (a,b) we show the kinetic energy and the production-dissipation ratio, , for the database of the 9 DNS runs. When
, near
, the flow transitions to decaying turbulence. We note that at early time the Boussinesq (A = 0.05) flow has the largest production-to-dissipation ratio due to the fact that the flow is not, at the outset, nonlinear enough to create a cascade to supply the dissipation. The higher-A flows go nonlinear and establish a cascade much earlier. During this early time the PDF of the material field is bimodal and becomes more Gaussian-like after
.
Figure 2. Time evolution of (a) the turbulent kinetic energy and (b) the production–dissipation ratio from DNS [Citation3,Citation4] for various Atwood numbers and initial conditions.
![Figure 2. Time evolution of (a) the turbulent kinetic energy and (b) the production–dissipation ratio from DNS [Citation3,Citation4] for various Atwood numbers and initial conditions.](/cms/asset/14ed21bf-9a90-456e-b709-0acff7e4acde/tjot_a_1662030_f0002_oc.jpg)
In equilibrium turbulence modelling one uses as the time-scale rate. This presumes a single important time-scale related to the established equilibrium cascade, where production is approximately equal to dissipation. In non-equilibrium turbulence the dissipation does not track the production or the cascade as inertial range begins development. It is useful to think in terms of an additional time-scale that represents the non-equilibrium nature of the flow and the development of a cascade. We identify the two time scales as
(9)
(9) Thus besides the equilibrium time-scale,
,
represents a second time-scale of the flow during which the small scale dissipation does not track the cascade which track the production. During this period – when the turbulence energy is rapidly increasing and its dissipation is negligible – the turbulence serves primarily to stir but not mix the material field. This is an important difference from fully developed turbulence.
In keeping with the positivity of a time-scale a characteristic time-scale rate is chosen as
(10)
(10) where we have used the equation
where P is the production, (the product of the mass flux and the pressure gradient), see [Citation1,Citation2]. The latter expression is relevant to homogeneous flows. For inhomogeneous flows there are additional transport terms in
that might be included. We shall call the term in the square-root the non-equilibrium factor and it will be used to scale the cascade during the non-equilibrium stages in which stirring dominates mixing. When the flow returns to equilibrium,
, the characteristic time-rate returns to its usual definition.
3.3. The density and specific volume PDF skewness
Mixing in variable-density fluids with large density-differences have some new and interesting features as shown in the density PDF in Figure . In [Citation2] all the simulations begin with symmetric ‘double-delta’ PDFs for the density field. As time evolves the PDF becomes highly asymmetric as the heavy fluid – due to its inertia and resistance to deformation – mixes more slowly, leaving pure heavy fluid long after the pure light fluid has vanished. See also Figure 4 of [Citation2].
3.4. The production of skewness
In homogeneous binary mixing the density skewness, , is governed by [Citation2]
(11)
(11) The equation is not of a simple relaxation type. There is a skewness source; even if the skewness is initially zero, skewness will be generated by
. The simplest examples of skewness generation in density-dependent mixing are the simulations of homogeneous variable-density turbulence by Livescu and Ristorcelli [Citation1,Citation2]. As shown in Figure 4 of [Citation2], the initially symmetric distribution rapidly becomes skewed, reflecting the different and much slower mixing rate of the heavy fluid. This phenomena is not seen in passive scalar mixing where the mixing process if it starts with a symmetric PDF remains symmetric and the process is usefully modelled as a Gaussian mixing process. In passive scalar mixing - if the initial PDF is skewed the subsequent skewness merely decays monotonically. The behaviour is very different in VDT as the Figures and highlight.
Figure 3. Time evolution of (a) the third moment, (b) the skewness of the mixture density, (c) the third specific volume moment, (d) the skewness of the specific volume from DNS [Citation2,Citation4] for various Atwood numbers and initial conditions.
![Figure 3. Time evolution of (a) the third moment, (b) the skewness of the mixture density, (c) the third specific volume moment, (d) the skewness of the specific volume from DNS [Citation2,Citation4] for various Atwood numbers and initial conditions.](/cms/asset/55ffdec1-3564-49d7-a5b2-7449767867b0/tjot_a_1662030_f0003_oc.jpg)
In Figure we show the third moments and skewness measures for the density, ρ, and specific volume, v, fields. Several things are worthy of note: (1) the third moments of ρ versus v do not scale with each other, (2) have opposite signs, also importantly, (3) unlike the second moments, the third moments are not monotonic and can increase or decrease from the initial value, and (4) they change sign during the flow evolution.
4. Background and problem statement
Formal developments in moment closure approaches to VDT mixing are a fairly recent development [Citation5]. It is useful to review how mixing is modelled in the passive scalar problem. The asymptotic behaviour of mixing in VDT is, of course, the passive scalar problem.
4.1. Passive scalar mixing – slaved to the hydrodynamical cascade
The conventional modelling assumption for small scale mixing of a passive scalar for turbulence with a developed inertial range is that the small scales are slaved to the large scales by a rate determining cascade and a model of the form is assumed. In moment closure approaches to passive scalar mixing the mixing cascade
of b reflecting the material field is (in a homogeneous field) modelled as
(12)
(12) where
is taken as a constant,
, as observed in empirical data. This mixing closure is a drag with time-scale set by the hydrodynamical cascade. The above closure can be shown to be derivable from the Fokker–Planck equation for a Langevin process – which models the mixing in Equation (Equation1
(1)
(1) ) as a Gaussian process.
Such a closure implies that the time-scale ratio of the material cascade tracks the hydrodynamical cascade,
(13)
(13) see [Citation12] and references therein for alternate views and exact solutions that invalidate this model. In [Citation12] it was demonstrated that the time-scale ratio
is better understood as the normalised mixing rate or the mixing rate in time normalised by
. The constant time-scale ratio appears a useful engineering approximation for stationary (or asymptotic) passive scalar mixing in a fully developed equilibrium turbulence. However, even in such a simple case, the problem is complex. Reynolds-number-dependent features have been observed [Citation13] that are not consistent with the usual notions of an inviscid cascade, conceptually enforced in LES mixing models, [Citation14]. We show the time-scale ratio in Figure for the 9 DNS cases and see that the time-scale ratio varies substantially during the course of the flow evolution. Clearly,
is not a good approximation at any time. Interestingly, the behaviour of the time-scale ratio is remarkably similar for all the Atwood numbers and different skewnesses and mean densities in the initial conditions. The 9 DNS are for three different Atwood numbers, each with three different initial heights of the initial double-delta mass fraction distribution.
4.2. Problem definition and goal
Our goal is to develop an expression for the material mixing cascade, , that reflects a variable-density mixing process over the course of which the PDF can be bimodal, uni-modal, skewed, and importantly, has bounded sample space.
There are three primary portions of the flow that a model for the cascade in VDT mixing must emulate: (1) the early time behaviour in which the PDF is bimodal and
is large, implying that the mixing is primarily stirring, (2) the peak of the mixing rate as the cascade builds an inertial subrange and
goes through zero and the decay process starts, and (3) the long-time behaviour in which the flow is primarily a passive scalar mixing. These three stages are seen in the time-evolution of the normalised mixing rates in Figure .
To close the small-scale dissipation term one makes arguments that there is a developed cascade and that the small scale mixing is slaved to, and can be written in terms of, quantities related to the large scales of the flow. This modelling closure is done heuristically.
Here we add a conservation law – the conservation of a PDF – as a constraint to the mixing description. We call this realisability which is also a formal requirement if the mixing process is modeled by a stochastic diffusion process. The application of this additional principle – that the statistical description of mixing must be related to a PDF – provides for a mathematically rigorous development that enables developing an expression for the enslavement of the small-scale to the large-scale statistics. There is, in fact, a valid unclosed PDF equation for the mixing process that (unfortunately) involves two-point correlations. Based on the heuristic that the small scales are slaved to the large scales, we use a single-point FPE governing the single-point PDF. To do this we presume that the mixing process is usefully modelled as a second-order diffusion process (in sample space) and derive a set of moment equations from the FPE. As will be seen, this simple requirement produces a cascade model for dependent on large scale variablesas
(14)
(14) The form of Equation (Equation14
(14)
(14) ) comes from the mathematical requirement that the cascade forms consistently with the evolution of a Fokker–Planck diffusion process for the material PDF and satisfies variable-density constraints related to Equation (Equation8
(8)
(8) ).
5. Variable density nomenclature and preliminaries
To develop the mathematics we use, as a model, the isothermal mixing between a heavy fluid of mass fraction , and a light fluid of mass fraction
,
(15)
(15) where
are constant the densities of the pure light and heavy fluids, A is the Atwood number, and
is the specific volume. The isothermal mixing problem, given by Equation (Equation15
(15)
(15) ), is a specific class of variable-density mixing problems that Chassaing et al. [Citation15] in their Section 6.3.1 or 4.1.3 call isothermal and isobaric mixing and implies the neglect of temperature and pressure fluctuations on the fluid density,
. The statement in Equation (Equation15
(15)
(15) ) is mathematically analogous to a moment-based treatment of turbulent combustion in which a single-progress-variable formulation is possible. In the case of premixed reactants the density is similarly written using the ideal gas law with r playing the role of a heat release parameter [Citation16].
5.1. Reynolds and Favre decomposition nomenclature
The following nomenclature for density, mass fraction, and specific volume is used to treat the statistical problem:
(16)
(16)
(17)
(17)
(18)
(18)
(19)
(19) Here the customary definitions are used: for first-order moments the overbar is a Reynolds ensemble average and the tilde a Favre average. The angle brackets used for higher order moments denote ensemble averages.
The following VDT relations and identities from [Citation17] will be useful:
(20)
(20) The following definitions and relations between second-order moments will be used in the developments below
(21)
(21) From the above relations for the mean and the variance it is clear that the mass fraction and the volume fraction are proportional. We follow the Besnard–Harlow–Rauenzahn (BHR) approach to VDT in [Citation5]. In the BHR approach the primary second-order moment for the mixing process is
. BHR first identified b as an important moment due to the central role it plays in the production of the mass flux in pressure-gradient-driven flows.
We will need relations between b and ,
,
, and
. From [Citation17] we have
(22)
(22) The above results can be re-arranged to obtain an identity that is frequently applied
(23)
(23) The term is not singular as
. The quantity b is a measure of the difference between Favre and Reynolds means
(24)
(24) As density and specific volume (or mass fraction) are inversely related to each other, the correlation is always negative. The result
(25)
(25) provides a connection to the Favre variance used in other areas of mixing, see also [Citation17].
6. The Fokker–Plank equation and its moments
As a model for mixing in VDT we invoke a stochastic diffusion process that enforces bounded mass fractions and converges to a beta pdf. We require a process with bounded mass fractions that can develop non-zero skewness in the PDF, and satisfies a conservation law in the instantaneous (i.e. not only in a finite number of statistical moments) sense [Citation18]. A FPE for the PDF of such mixing process for the mass or volume fraction is
(26)
(26) We call the FPE (Equation26
(26)
(26) ) a beta process because its statistically stationary solution, its attractor or invariant, is a beta distribution. The function
in the diffusion term keeps the process bounded in
space, [Citation10,Citation18]. Boundedness of the process is crucially important for non-dilute mixing. If
where replaced with an expression that is not a function of
, one would have an Ornstein-Uhlenbeck process with a Gaussian as its attractor, and a model that is used for symmetric, passive scalar mixing. Note that if the initial PDF f is arbitrary and not a beta PDF, Equation (Equation26
(26)
(26) ) evolves the PDF such that
remains bounded and the solution, f, only asymptotically becomes a beta PDF with a non-zero skewness: a beta-convergent stochastic process. The stationary solution (if β, κ, and S are constants) to the FPE is the beta PDF, [Citation10], with arbitrary non-zero skewness.
We call , and S the drift, diffusion, and relaxation coefficients. They are, at this point, unspecified functions of the statistics of the PDF which are single-point, large scale quantities. Their specification will lead to a model for the small scale
in terms of large scale quantities as is consistent with the usual notion that the small scales are slaved to the large scales. Requiring the mixing process to follow Equation (Equation26
(26)
(26) ) produces a formal mathematical expression for how small scale mixing and its time-evolution are represented in terms of the large scales by a realisable mixing process. The physical mixing process is a small scale process involving two point statistics [Citation19,Citation20].
It is worth contrasting this work to an earlier Fokker–Planck approach to passive scalar mixing by Fox [Citation21] based on models for complex mixing microstructure. Fox models the small two-point structure of the exact PDF equation from Navier-Stokes. Fox uses earlier experimental studies on mixing that use a narrative relating mixing to a lamella-scale microstructure and after some original and complex intuitive reasoning, scaling and normalisation invokes a bivariate Fokker–Planck equation for proxies for the concentration and scaled lamella length. Our approach is more pedestrian: we ignore the two-point structure of the small-scale mixing and assume that the small scales are slaved to the large scales such that a single point FPE is relevant.
At the level of principle Fox's [Citation21] Fokker–Planck equation has a similar structure and solution that of our Equation (Equation26(26)
(26) ) with, in addition to the bimodal treatment, important differences. His mixing process treats zero-skewness, symmetric, passive scalar mixing; in which case our S becomes his
. The more general problem of mixing between unequal amounts of pure fluids (in a box) or in mixing between two different streams of fluids cannot be treated by the symmetric beta model even for passive scalar mixing and in practical problems involving inhomogeneity such as the planetary boundary layer or the bubbles-, and spikes-skewness in the Rayleigh–Taylor mixing layer. In its simplest form this is the case of mixing in a box of homogenous stationary turbulence in which the initial PDF is two unequal-height delta spikes.
In such cases the skewness (Figure ) of the PDF – in variable density turbulence (VDT) – plays a hugely important role which, for our current discussion, results in different drift coefficients. In the beta process Equation (Equation26(26)
(26) )
is responsible for generating the skewness of the PDF. Skewness is important in general passive scalar mixing processes that can be generated by mixing between two fluids with unequal mass fractions.
In summary, the differences between Fox's problem and approach and ours are as follows.
Fox treats symmetric passive scalar mixing with zero skewness. We treat active scalar mixing.
We treat the case in which skewness may arise due to initial conditions or due to variable-density effects. In our FPE skewness will be connected to
.
For mixing in VDT we see that symmetric initial conditions of the PDF (no initial skewness) become skewed. The behaviour of skewness in VDT is not monotonic as it is in the case of passive scalar mixing.
Fox's analysis is useful, interesting, and connects to other methods for passive scalar mixing [Citation22], and is mathematically important as a special limiting case for more general models to attain.
6.1. The mean density equation from Fokker–Planck
Our benchmark mixing problem is the homogeneous, buoyantly-driven VDT problem; turbulence in box for which the mean density and mean Favre mass fraction are constant. The FPE has three unknown coefficients, S, β, and κ. In this section we apply the constraint of constant mean density to find that S is a function of , and the closure problem is reduced to the problem of defining
as S is not an independent object.
Using the definition of and taking the moment of Equation (Equation26
(26)
(26) ) we find that the mean density equation is governed by
(27)
(27) The angle brackets reflect ensemble averages (moments) and
. The above equation is general and reflects the statistical nature of the process and not any physics. We now add the physics of the variable-density relations, Equation (Equation15
(15)
(15) ). Note the following relations, derivable from Equation (Equation15
(15)
(15) )
(28)
(28) For the current model problem
is constant and the mean density equation becomes
(29)
(29) and an exact expression for S in terms of β and κ and various moments is determined. Performing the indicated operations specifies S as
(30)
(30) The higher order moments above can be related to their dimensional equivalents as
(31)
(31)
(32)
(32)
(33)
(33) Our Monte Carlo (MC) simulations verify that the above choice of S in Equation (Equation30
(30)
(30) ) produces a constant mean density. Using the above definitions G is written as
(34)
(34) The FPE coefficient S is now given in terms of the drift and diffusion coefficients and moments of the density field
(35)
(35) With G given by Equation (Equation34
(34)
(34) ) the FPE coefficient S reflects the fluid physics of VDT through Equation (Equation28
(28)
(28) ). The problem of the small scale
has been replaced with the specification of
which will be functions of single point large scale statistics.
A few limits are of interest. The Boussinesq limit is
(36)
(36) and thus
. The asymptotic fully mixed state for which the density moments vanish is
(37)
(37) for arbitrary
. Using the results from Appendix 2 in the no-mix state one has
(38)
(38)
(39)
(39)
(40)
(40)
6.2. The Fokker–Planck 
equation
Having the FPE it is now possible to generate evolution equations for the cascade of any of the moments. The equation for the variance of the Reynolds mass fraction fluctuation, derived from the FPE, is
(41)
(41)
(42)
(42) The
equation is the simplest second moment equation from the FPE that reflects the variable-density mixing process. To insure the long term fully mixed state and that
from above requires that the diffusion coefficient scales as (at the very least)
. The variance equation is then written as
(43)
(43) This is our first interesting result. The first equality shows how
might be modelled if mixing proceeded as a realisable diffusion process (with a beta attractor for the mass fractions). We see that the mix rate depends on a measure of the mix state,
. Realisability for mixing requires a monotone decay of
, which can be ensured if
.
6.3. The Fokker–Planck 
equation
Our primary goal is to develop a model for the mixing cascade in Equation (Equation1
(1)
(1) ), suitable for early time mixing, non-equilibrium conditions, as well as asymptotically. The FPE moment equation for
is found by taking the moments of Equation (Equation26
(26)
(26) ) with ρ and v:
(44)
(44) If we apply Equations (Equation28
(28)
(28) ) and (Equation30
(30)
(30) ) and take the moments we find
(45)
(45) In anticipation of subsequent derivations the equation is rewritten as
(46)
(46) The analysis of the
equation indicated that κ vanishes asymptotically. Without loss of generality we take
and
(47)
(47) Equation (Equation47
(47)
(47) ) is a FPE-based model for
and is our central result: it is a large scale parameterisation of small scale mixing based on realisability as linked to the FPE diffusion process.Footnote1
Parenthetically, for a homogeneous problem, for which is constant, the first moment equation for the Reynolds-averaged mass fraction
(48)
(48) can be used to derive an equation for b by applying Equation (Equation23
(23)
(23) ). The result is
(49)
(49) Equation (Equation47
(47)
(47) ) predicts, a priori, a curious phenomena: within a Markovian framework the asymptotic mixing rate is potentially a function of the initial conditions. The asymptotic b equation is
(50)
(50) suggesting the possibility that the mean initial mass fraction and density ratio determine the asymptotic mixing rate. This is not seen in the DNS data, suggesting that the second term is small compared to the first. The comparison is not conclusive as the DNS data represents a single realisation of the flow for each Atwood number and each
; the theory above is for an ensemble of realisations. Using the above results the next step is to determine
, and S in order to create a realisable skewed non-Gaussian process for the mixing cascade,
which we then we test against DNS data using Monte Carlo simulations.
7. Determining the drift 
and diffusion 
coefficients
The Fokker–Plank b equation (Equation47(47)
(47) ) shows how the mixing depends on the drift and diffusion coefficients. We have proceeded as far as the mathematics will allow for the drift β and diffusion κ coefficients. Our procedure now becomes phenomenological. We have two non-dimensional second moment metrics that describe the mixing process: the time-scale ratio or mix rate metric and a mix state metric. We begin by looking for collapses of the data with variable-density metrics.
7.1. The time-scale ratio is a mix rate metric
In the passive scalar turbulence moment closure problem the conventional model for the mixing is a drag model with time-scale set by the ‘eddy turnover’ rate
(51)
(51) where
and, as discussed above, implies a Gaussian model for the mixing process. At large times we expect passive-scalar Gaussian-like mixing and β to scale as
(52)
(52) It should be made clear that the passive scalar limit with
is an approximation that is known to not be consistent with observed behaviours, [Citation12,Citation13] but qualitatively useful for engineering models.
There are a number of reasons to work with some form of the time-scale ratio [Citation12]
(53)
(53) as a ‘mix rate’ metric reflecting as it does the coupling between the mixing and hydrodynamic cascades. In non-dimensional time,
the time-scale ratio is the mixing rate [Citation12].
The FPE b equation (Equation47(47)
(47) ) suggests that the time-scale ratio of Figure might better collapse when scaled as
(54)
(54) Figure shows this to be the case and we have an indication supporting the FPE strategy. The scatter in the time-scale data is reduced near the peak and also early time and during flow transition. This scaling collapses the time-scale ratio to approximately unity at the initial time.
Figure 5. Time evolution of the time-scale ratio, scaled as in Equation (Equation54(54)
(54) ) from DNS [Citation2,Citation4] for various Atwood numbers and initial conditions.
![Figure 5. Time evolution of the time-scale ratio, scaled as in Equation (Equation54(54) tscrVD=−1+⟨ρ2⟩ρ¯2kεb˙b.(54) ) from DNS [Citation2,Citation4] for various Atwood numbers and initial conditions.](/cms/asset/31b893a7-cbe2-428a-a0dd-ec6db45226f1/tjot_a_1662030_f0005_oc.jpg)
7.2. A second-order mix state metric for variable-density turbulence
There are several second-order mix state metrics [Citation17]. As the primary second-order moment in the BHR strategy in VDT is b [Citation17] and our primary goal is a model for , it is consistent to choose a second moment mix state metric as
(55)
(55) where
is given in Appendix 2. We now query the DNS if there is a variable-density scaling,
, that better collapses the data. We will construct variable-density functions and look to see if we can find more universal behaviour. There are several VDT functions that naturally appear from the Fokker–Planck analysis:
(56)
(56)
(57)
(57)
(58)
(58)
(59)
(59)
(60)
(60) We expect these functions to collapse the mixing process during the stage when the variable-density effects are important. We have arranged them in forms that become, asymptotically, unity and thus reflect the late-stage passive-scalar-mixing limit. We find that the best collapse for Θ is with
thus we adopt a new variable-density mix metric
as
(61)
(61) The quantities Θ and
and their VDT equivalents
and
are shown in Figure . The collapse will be useful.
Figure 6. Time evolution of the second-order mix state metrics, given by Equations (Equation55(55)
(55) ) and (Equation61
(61)
(61) ) from DNS [Citation2,Citation4] for various Atwood numbers and initial conditions.
![Figure 6. Time evolution of the second-order mix state metrics, given by Equations (Equation55(55) Θ=1−bbnm,(55) ) and (Equation61(61) Θ′=121+η1+ηbΘ.(61) ) from DNS [Citation2,Citation4] for various Atwood numbers and initial conditions.](/cms/asset/a905d3db-3dd7-4806-9f3a-d06a46a35638/tjot_a_1662030_f0006_oc.jpg)
7.3. Closing the drift and diffusion coefficients
We take β, and for simplicity κ, to scale as
(62)
(62) It is possible to show that
is second-order (in powers of second moments) relative to
.
The b Equation (Equation47(47)
(47) ), reflecting a realisable non-Gaussian mixing process, becomes
(63)
(63) For simplicity and because our interest is in capturing the second moments, we take
as constant. To capture the behaviour of other higher statistics we expect that a deeper study of κ is required. For now our interest is a proof of concept adequately representing second-order statistics. A more complex dependence of
is now established:
(64)
(64) Equation (Equation64
(64)
(64) ) can be written in terms of the variable-density time-scale ratio as
(65)
(65) and thus
(66)
(66) To leading order, we can think of
as the time-scale ratio (the non-dimensional mix rate).Footnote2
The DNS data shows that the flow can be divided into 3 regimes: early time stirring and transitional mixing, intermediate-time mixing where the kinetic energy is maximum, and a late time decay and asymptotic Gaussian mixing.
Early time,
,
: In this regime the time-scale ratio is consistent with the idea that as the flow begins the fluids are unmixed and primarily undergoing stirring, Θ is small and the time-scale of the material field tracks the time-scale of the hydrodynamic field,
. The PDF bimodal and can be highly skewed.
Maximum kinetic energy mixing,
: Maximum kinetic energy is coincident with maximum mixing at time
at which
. In this regime the PDF develops a single peak, the skewness (depending on the initial conditions) is substantially reduced.
Asymptotic late stage mixing,
:
and for which the variable-density time-scale ratio
. In this regime the PDF is well on its way to becoming more Gaussian-like.
A simple phenomenological approximation consistent with these notions and relating the mix rate metric to the mix state metric is
(67)
(67) In this expression
captures the time-scale ratio behaviour at early time when there is little mixing and
is small. The
term reflects a non-equilibrium peak where
. The
term captures the asymptotic passive scalar limit. The various β's are unknown and we model them according to the following notions consistent with the DNS data.
We expect
and
to be small in the highly non-equilibrium early time stirring phase when little mixing occurs and turbulence production in the large scales dominates dissipation. We expect the mixing rate to be suppressed according to the magnitude of the non-equilibrium metric. During this non-equilibrium time-period the relevant time-scale rate is
as discussed above.
The most non-equilibrium portion of the mixing is near where
is maximum. It is where k is largest and is demarcated as where
after which the turbulence decays.
At late time the flow is quasi-equilibrium and
is small. We expect
and thus
as
.
We have seen that scaling the time by
collapses the different-Atwood-number flows. We need to scale time during the non-equilibrium portion of the flow so we choose a proxy for the Atwood number that reflects the evolution of the mixing – a local-time-scale Atwood-number proxy is
. Thus we incorporate the effect of the current Atwood number with the factor
as suggested from the no-mix result,
. While we have provided physical reasoning as to why this is plausible, our experiments have shown that this causes the MC simulation results to compare move favourably with DNS compared to other scalings.
The above points suggest
(68)
(68) We have, in principle, posed a relation between the time-scale ratio – a mix rate metric and a VDT mix state metric,
based on intuitive arguments and DNS data with a mathematical structure imposed by a realisability requirement.
7.4. Monte Carlo solutions
Every FPE, such as Equation (Equation26(26)
(26) ), is statistically equivalent to a system of stochastic differential equations [Citation23]. We develop our ideas using Monte Carlo (MC) simulations in which we solve, for an ensemble of Lagrangian particles, the stochastic equation
(69)
(69) where
is a Wiener process with independent increments, zero mean and
variance, governing each stochastic particle [Citation10] and β, κ, and S are as given above. The complete model is given in Appendix 1.
Figure 7. Time evolution of two normalised mix rates (left), the normalised mix state, b, (down-right) and its rate (up-right) for A = 0.05. Dotted lines – DNS data [Citation2,Citation4], solid lines – Monte Carlo model predictions.
![Figure 7. Time evolution of two normalised mix rates (left), the normalised mix state, b, (down-right) and its rate (up-right) for A = 0.05. Dotted lines – DNS data [Citation2,Citation4], solid lines – Monte Carlo model predictions.](/cms/asset/0f67183e-36c4-44b8-8c38-b63839b57440/tjot_a_1662030_f0007_oc.jpg)
Figure 8. Time evolution of two normalised mix rates (left), the normalised mix state, b, (down-right) and its rate (up-right) for A = 0.5. Dotted lines – DNS data [Citation2,Citation4], solid lines – Monte Carlo model predictions.
![Figure 8. Time evolution of two normalised mix rates (left), the normalised mix state, b, (down-right) and its rate (up-right) for A = 0.5. Dotted lines – DNS data [Citation2,Citation4], solid lines – Monte Carlo model predictions.](/cms/asset/f9d7799e-3d6f-449c-b035-23af7878f3d9/tjot_a_1662030_f0008_oc.jpg)
Figure 9. Time evolution of two normalised mix rates (left), the normalised mix state, b, (down-right) and its rate (up-right) for A = 0.75. Dotted lines – DNS data [Citation2,Citation4], solid lines – Monte Carlo model predictions.
![Figure 9. Time evolution of two normalised mix rates (left), the normalised mix state, b, (down-right) and its rate (up-right) for A = 0.75. Dotted lines – DNS data [Citation2,Citation4], solid lines – Monte Carlo model predictions.](/cms/asset/df18f993-01ac-4767-acc2-21dae12a48da/tjot_a_1662030_f0009_oc.jpg)
The model predictions using MC are compared to DNS data in Figures – for three different Atwood numbers each for three different initial conditions. The MC drift and diffusion are specified as above with the hydrodynamical quantities, ϵ, k, and P taken from DNS. We have plotted the two time-scale ratios as well as the normalised b and it's time-scale. It should be emphasised that we have predicted the second moments of the mixing from the quiescent (stationary stochastic blobs of fluid) initial condition, through transition, to maximum kinetic energy, and then decay. The agreement is good especially when one considers we are comparing single DNS realisations with a theory for ensemble realisations.
8. General discussion
Having determined the drift and diffusion coefficients of a variable-density mixing process, we have also determined the mixing rate of any second-order moment of the material field. In Figures – we plot the time-evolutions of the time-scale ratios of and their normalised fields for and
as predicted by the model using MC compared to DNS data. The same drift and diffusion coefficients also work for these other second-order fields.
Figure 10. Time evolution of the normalised mix rates of the specific volume (up-left), the mixture density (down-left), and the normalised mix states as measured by the specific volume variance (up-right) and the mixture density (down-right) for A = 0.05. Dotted lines – DNS data [Citation2,Citation4], solid lines – Monte Carlo model predictions.
![Figure 10. Time evolution of the normalised mix rates of the specific volume (up-left), the mixture density (down-left), and the normalised mix states as measured by the specific volume variance (up-right) and the mixture density (down-right) for A = 0.05. Dotted lines – DNS data [Citation2,Citation4], solid lines – Monte Carlo model predictions.](/cms/asset/722f2411-4e55-4636-bc82-5aa5f919122f/tjot_a_1662030_f0010_oc.jpg)
Figure 11. Time evolution of the normalised mix rates of the specific volume (up-left), the mixture density (down-left), and the normalised mix states as measured by the specific volume variance (up-right) and the mixture density (down-right) for A = 0.5. Dotted lines – DNS data [Citation2,Citation4], solid lines – Monte Carlo model predictions.
![Figure 11. Time evolution of the normalised mix rates of the specific volume (up-left), the mixture density (down-left), and the normalised mix states as measured by the specific volume variance (up-right) and the mixture density (down-right) for A = 0.5. Dotted lines – DNS data [Citation2,Citation4], solid lines – Monte Carlo model predictions.](/cms/asset/0f2cb173-b059-41f2-9308-a381ba54bf5d/tjot_a_1662030_f0011_oc.jpg)
Figure 12. Time evolution of the normalised mix rates of the specific volume (up-left), the mixture density (down-left), and the normalised mix states as measured by the specific volume variance (up-right) and the mixture density (down-right) for A = 0.75. Dotted lines – DNS data [Citation2,Citation4], solid lines – Monte Carlo model predictions.
![Figure 12. Time evolution of the normalised mix rates of the specific volume (up-left), the mixture density (down-left), and the normalised mix states as measured by the specific volume variance (up-right) and the mixture density (down-right) for A = 0.75. Dotted lines – DNS data [Citation2,Citation4], solid lines – Monte Carlo model predictions.](/cms/asset/30dc2f9d-1aa8-480b-95e6-cf8f8e84de77/tjot_a_1662030_f0012_oc.jpg)
Additionally one has an expression for the mixing cascade of any quantity one can derive an equation from FPE (not a trivial task for many moments). Equation (Equation64(64)
(64) ) is a moment closure model for the mixing of b and equivalently (in homogeneous flow)
due to Equation (Equation25
(25)
(25) ). From the FPE for the variance
, Equation (Equation43
(43)
(43) ), we have a moment expression for the mixing rate of
as
(70)
(70) with the drift and diffusion coefficients as determined. Second-order moment models for the mixing of
and for
in inhomogeneous flows requires the FPE form equation.
8.1. Ensemble models versus individual realisations
The 9 DNS date we have used represent individual realisations of the flow for 3 Atwood numbers and 3 different mean densities at each Atwood number at a fairly low grid resolution. In comparing MC to DNS it is crucial to keep in mind that the Fokker–Plank development for the cascade model is for an ensemble of realisations. One cannot expect the MC ensemble to reflect the individual DNS realisations and as such we do not expect perfect agreements. We have available data from three additional DNS of this problem with
grid resolution and in a subsequent work (now in progress) following Schwarzkopf et al. [Citation24] we are also studying the model's performance in inhomogeneous flows.
8.2. Comment on application of Monte Carlo simulations as a modelling tool
Statistical approaches to turbulence face the moment closure problem that requires expressions for unclosed moments in terms of known moments. This modelling process is generally done intuitively and is usually only tested by attempting to compute real physics with the model and seeing if it does better for some statistical moments for which one has data. Here our goal of using MC has been to aid model development. We have conducted MC simulations of Equation (Equation26(26)
(26) ) which, by definition, evolves a valid PDF from which we numerically estimatetatistical moments of the density, specific volume, or mass fraction fields and thus can test closure assumptions directly, without a moment closure or DNS calculation of the coupled hydrodynamics + mix problem. We find this particularly useful in VDT where the relation between moments of the specific volume and density fields involves non-Gaussian moments of all orders [Citation17] and thus guessing at closures has many challenges.
9. Concluding comments
Mixing in VDT is a substantially more complicated process than passive scalar mixing, since VDT involves additional effects: (1) active scalar tightly coupled to the momentum equation, (2) non-Gaussian and skewed PDFs, and for our benchmark problem, (3) non-equilibrium turbulence.
We apply the idea that a model for the small scale mixing slaved to the large scales can be based on a suitable underlying single-point PDF. We essentially add a conservation law – the conservation of the PDF – as a constraint to the description of material mixing. This realisability requirement produces a model for the small scale that depends on large scale variables. At the level of principle this requirement is then a mathematically formal expression of the usual notion that in a turbulence where the production is in the large scales the small scale mixing is slaved to the large scales. At the level of practice we have replaced the single small-scale unknown
, about which we know very little, with two unknowns, the FPE drift and diffusion coefficients, about which we know considerably more.
In summary we highlight our findings as, we have
Used moment equations derived from the Fokker–Planck equation together with moment equations from the Navier–Stokes equation to derive models for small-scale dissipative moments in terms of large-scale moments using a realisability principle.
Found useful variable-density scalings for mix state and mix rate metrics.
Developed and applied MC as a theoretical developmental tool for closure validation.
Created an engineering closure for a second-order moment, b, applicable in moment approaches to non-equilibrium situations.
Created a closure for a PDF method treatment of variable-density turbulence mixing.
Acknowledgements
We thank Daniel Livescu for providing extensive data sets from his direct numerical simulations and John Schwarzkopf for advise and input on the manuscript and general creative questions and insights.
Disclosure statement
No potential conflict of interest was reported by the authors.
ORCID
J. Bakosi http://orcid.org/0000-0002-0604-5555
Additional information
Funding
Notes
1 For the problem to model mixing we require . Note that
and
.
2 The denominator does not deviate very substantially from 2.
References
- Livescu D, Ristorcelli JR. Buoyancy-driven variable-density turbulence. J Fluid Mech. 2007;591:43–71. doi: 10.1017/S0022112007008270
- Livescu D, Ristorcelli JR. Variable-density mixing in buoyancy-driven turbulence. J Fluid Mech. 2008;605:145–180. doi: 10.1017/S0022112008001481
- Livescu D. Personal communication; 2016.
- Aslangil D, Livescu D, Arindam B. Variable-density buoyancy-driven turbulence with asymmetric initial density distribution. Under review in Physica D: Nonlinear phenomena; 2019. Available from: https://arxiv.org/abs/1907.12977 . LA-UR-19-27261.
- Besnard D, Harlow FH, Rauenzahn RM. 1992. Turbulence transport equations for variable-density turbulence and their relationship to two-field models. LANL Report No. LA–12303–MS; 1992.
- Livescu D, Ristorcelli JR, Gore RA, et al. High-Reynolds number Rayleigh–Taylor turbulence. J Turbul. 2009;10(13):N13 doi: 10.1080/14685240902870448
- Schwarzkopf JD, Livescu D, Gore RA, et al. Application of a second-moment closure model to mixing processes involving multicomponent miscible fluids. J Turbul. 2011;12:N49. doi: 10.1080/14685248.2011.633084
- Girimaji SS. Assumed β-pdf model for turbulent mixing: validation and extension to multiple scalar mixing. Combust Sci Technol. 1991;78(4):177–196. doi: 10.1080/00102209108951748
- Fox RO. Computational models for turbulent reacting flows. Cambridge: Cambridge University Press; 2003.
- Bakosi J, Ristorcelli JR. Exploring the beta distribution in variable-density turbulent mixing. J Turbul. 2010;11(37):1–31.
- Livescu D, Ristorcelli JR. Mixing asymmetry in variable density turbulence. In: Bruno Eckhardt, editor. Advances in turbulence XII. Vol. 132. Springer Proceedings in Physics. Berlin, Heidelberg: Springer; 2009. p. 545–548.
- Ristorcelli JR. Passive scalar mixing: analytic study of time-scale ratio, variance, and mix rate. Phys Fluids. 2006;18(7):075101. doi: 10.1063/1.2214704
- Wachtor AJ, Grinstein FF, DeVore CR, et al. Implicit large-eddy simulation of passive scalar mixing in statistically stationary isotropic turbulence. Phys Fluids. 2013;25(2):025101. doi: 10.1063/1.4783924
- Pullin DI. A vortex-based model for the subgrid flux of a passive scalar. Phys Fluids. 2000;12(9):2311–2319. doi: 10.1063/1.1287512
- Chassaing P. Variable density fluid turbulence. Fluid mechanics and its applications. Dordrecht: Kluwer Academic Publishers; 2002.
- Libby PA, Williams FA. Turbulent reacting flows. Berlin: Springer Verlag; 1980.
- Ristorcelli JR. Exact statistical results for binary mixing and reaction in variable density turbulence. Phys Fluids. 2017;29(2):020705. doi: 10.1063/1.4974517
- Bakosi J, Ristorcelli JR. Diffusion processes satisfying a conservation law constraint. Int J Stoch Anal. 2014;2014:9, Article ID 603692.
- Pope SB. PDF methods for turbulent reactive flows. Prog Energy Combust. 1985;11:119–192. doi: 10.1016/0360-1285(85)90002-4
- Pope SB. Turbulent flows. Cambridge: Cambridge University Press; 2000.
- Fox RO. The Fokker–Planck closure for turbulent molecular mixing: passive scalars. Phys Fluids. 1992;4(6):1230–1244. doi: 10.1063/1.858241
- Madadi-Kandjani E, Fox RO, Passalacqua A. Application of the Fokker–Planck molecular mixing model to turbulent scalar mixing using moment methods. Phys Fluids. 2017;29(6):065109. doi: 10.1063/1.4989421
- Gardiner CW. Stochastic methods, a handbook for the natural and social sciences. 4th ed. Berlin, Heidelberg: Springer-Verlag; 2009.
- Schwarzkopf JD, Livescu D, Baltzer JR, et al. A two-length scale turbulence model for single-phase multi-fluid mixing. Flow Turbulence Combus. 2016;96(1):1–43. doi: 10.1007/s10494-015-9643-z
- Ristorcelli JR, Bakosi J. Progress on the density variance in the reaction rate. Technical Report LANL Report: LA-UR 16-25224. Los Alamos National Laboratory; 2016.
Appendices
Appendix 1. Final model
The new b equation and model for the time-scale ratio is summarised as follows.
(A1)
(A1)
(A2)
(A2) where
(A3)
(A3)
(A4)
(A4) The skewness is taken from the beta PDF and is given by
(A5)
(A5) The above expression, given in [Citation17], was tested in [Citation25]. Coefficients and definitions are
(A6)
(A6)
(A7)
(A7)
(A8)
(A8)
(A9)
(A9) The exponent a plays a small role at the initial condition at high A; in simulations both
and a = 1 work well. The constant in
sets the asymptotic passive scalar time-scale ratio. We typically take the constant in
as 1 which produces an asymptotic time-scale ratio
for proof of concept; the value 0.8 compares more favorably to DNS data. The objects in Equation (EquationA7
(A7)
(A7) ) can be tweaked according to the users whim.
We have taken the function as our concern is with a second-order moments. If higher order moments are a concern then a deeper inquiry into the functional form of
is required. The coefficient
does not appear to play a strong role for second-order moments.
In working with inhomogeneous flows it is better to express in terms of the mean density
(A10)
(A10)
(A11)
(A11) To close the equation at the second moment level we need to close
in G. First let us see if this is necessary.
(A12)
(A12) Do we need a closure? This question can be answered by answering the following two questions: 1) is
and is
small?
(A13)
(A13)
(A14)
(A14) If not, then we need to test the following two models
(A15)
(A15) Alternatively if one works with Reynolds variables one finds
(A16)
(A16) or go to the beta PDF. It appears that the first ‘no-mix’ model with the variance given by the mixed varince works best and the expression for G becomes
(A17)
(A17)
Appendix 2. No-mix results
The no mix results given in the text are easily obtained using the double-delta distribution
(A18)
(A18) Taking the moments
(A19)
(A19) one finds the value of
. Using the usual
[Citation17] one obtains the following relations between the Favre and Reynolds means
(A20)
(A20) Thus (in the no-molecular-mix limit) one has the following relations between the Favre and Reynolds means
(A21)
(A21) which allows one to express no-mix identities in either Favre or Reynold variables. In general mixing situations
. The mean specific volume is similarly found from the sample space integral to be
(A22)
(A22) The specific volume density variance is computed from
(A23)
(A23) after substituting in for
in terms of
from the above no mix relations in Equation (EquationA20
(A20)
(A20) ). The enumerator is maximum at
and
as the density ratio gets large. This is the same result one gets if one substitutes
in the no mix relation,
.
The no-mix values of other relevant second moments are then
(A24)
(A24)
(A25)
(A25)
(A26)
(A26)
(A27)
(A27)
(A28)
(A28)
(A29)
(A29)
(A30)
(A30)
(A31)
(A31)
(A32)
(A32)
(A33)
(A33) The results satisfy the Cauchy-Schwarz inequality, e.g.
.
The above reflect the maximum values of the second moments and are useful for scaling and ensuring boundedness requirements. In the no-mix limit the density intensity normalised by r is writtenas
(A34)
(A34)
(A35)
(A35) Alternatively, if one works with Reynolds variables one finds
(A36)
(A36) which suggests the closure model under mixing conditions for the cubic moment as
(A37)
(A37) The Atwood number and r are related as
(A38)
(A38) Our DNS data set is at A = 0.05, 0.5, 0.75, corresponding to
, respectively. Following the same methods the fourth-order moment appearing in the constitutive relation for the density variance can be found to be, in the no-mix limit, as
(A39)
(A39)
(A40)
(A40)
Appendix 3. Select 
-PDF facts
Facts regarding the beta PDF. The problem solution requires dealing with both Favre and Reynolds averages. The Favre-, and Reynolds-averaged PDFs are related by
(A41)
(A41) The Favre PDF is a two-parameter PDFs such that
(A42)
(A42) One PDF in that class is the Favre β-PDF:
(A43)
(A43)
(A44)
(A44)
(A45)
(A45)
(A46)
(A46)
(A47)
(A47) The quantity θ is sometimes called (for better or worse) the mixedness. It is more useful to think of θ as a measure of the relative scalar variance: the variance is maximum when
which corresponds to the no-mix limit. For mixed fluids, i.e. low variance,
.
The results above are found by applying the beta PDF identities
(A48)
(A48) We note that
(A49)
(A49)
(A50)
(A50) The centred Favre-skewness is givenby
(A51)
(A51) In the last equation above relations (EquationA44
(A44)
(A44) ), (EquationA45
(A45)
(A45) ) have been used.