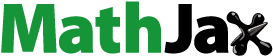
Abstract
We consider rough stochastic volatility models where the driving noise of volatility has fractional scaling, in the ‘rough’ regime of Hurst parameter . This regime recently attracted a lot of attention both from the statistical and option pricing point of view. With focus on the latter, we sharpen the large deviation results of Forde-Zhang [Asymptotics for rough stochastic volatility models. SIAM J. Financ. Math., 2017, 8(1), 114–145] in a way that allows us to zoom-in around the money while maintaining full analytical tractability. More precisely, this amounts to proving higher order moderate deviation estimates, only recently introduced in the option pricing context. This in turn allows us to push the applicability range of known at-the-money skew approximation formulae from CLT type log-moneyness deviations of order
(works of Alòs, León & Vives and Fukasawa) to the wider moderate deviations regime.
1. Introduction
Since the groundbreaking work of Gatheral et al. (Citation2014), the past two years have brought about a gradual shift in volatility modeling, leading away from classical diffusive stochastic volatility models towards so-called rough volatility models. The term was coined in Gatheral et al. (Citation2014) and Bayer et al. (Citation2016), and it essentially describes a family of (continuous-path) stochastic volatility models where the driving noise of the volatility process has Hölder regularity lower than Brownian motion, typically achieved by modeling the fundamental noise innovations of the volatility process as a fractional Brownian motion with Hurst exponent (and hence Hölder regularity) . Here, we would also like to mention pioneering work on asymptotics for rough volatility models in Alòs et al. (Citation2007) and Fukasawa (Citation2011). A major appeal of such rough volatility models lies in the fact that they effectively capture several stylized facts of financial markets both from a statistical (Gatheral et al. Citation2014; Bennedsen et al. Citation2016) and an option-pricing point of view (Bayer et al. Citation2016). In particular, with regards to the latter point of view, a widely observed empirical phenomenon in equity markets is the ‘steepness of the smile on the short end’ describing the fact that as time to maturity becomes small the empirical implied volatility skew follows a power law with negative exponent, and thus becomes arbitrarily large near zero. While standard stochastic volatility models with continuous paths struggle to capture this phenomenon, predicting instead a constant at-the-money implied volatility behavior on the short end (Gatheral Citation2011), models in the fractional stochastic volatility family (and more specifically so-called rough volatility models) constitute a class, well-tailored to fit empirical implied volatilities for short dated options.
Typically, the popularity of asset pricing models hinges on the availability of efficient numerical pricing methods. In the case of diffusions, these include Monte Carlo estimators, PDE discretization schemes, asymptotic expansions and transform methods. With fractional Brownian motion being the prime example of a process beyond the semimartingale framework, most currently prevalent option pricing methods – particularly the ones assuming semimartingality or Markovianity – may not easily carry over to the rough setting. In fact, the memory property (aka non-Markovianity) of fractional Brownian motion rules out PDE methods, heat kernel methods and all related methods involving a Feynman-Kac-type Ansatz. Previous work has thus focused on finding efficient Monte Carlo simulation schemes (Bayer et al. Citation2016; Bennedsen et al. Citation2017; Bayer et al. Citation2017) or – in the special case of the Rough Heston model – on an explicit formula for the characteristic function of the log-price (see El Euch and Rosenbaum Citation2016), thus in this particular model making pricing amenable to Fourier based methods. In our work, we rely on small-maturity approximations of option prices. This is a well-studied topic for which we mention (with no claim to completeness) a number of works, either based on large deviations or central limit type scaling regime, that inspired this work: Alòs et al. (Citation2007), Fukasawa (Citation2011), Deuschel et al. (Citation2014a), Deuschel et al. (Citation2014b) and Fukasawa (Citation2017), also Medvedev and Scaillet (Citation2003, Citation2007), Osajima (Citation2007, Citation2015), Guennoun et al. (Citation2014), Mijatović and Tankov (Citation2016) and especially Forde and Zhang (Citation2017). Rather recently, Friz et al. (Citation2018) introduced another regime called moderately-out-of-the-money (MOTM), which, in a sense, effectively navigates between the two regimes mentioned above, by rescaling the strike with respect to the time to maturity. This approach has various advantages. On the one hand, it reflects the market reality that as time to maturity approaches zero, strikes with acceptable bid-ask spreads tend to move closer to the money (see Friz et al. Citation2018 for more details). On the other hand, it allows us to zoom in on the term structure of implied volatility around the money at a high resolution scale. To be more specific, our paper adds to the existing literature in two ways. First, we obtain a generalization of the Osajima energy expansion (Osajima Citation2015) to a non-Markovian case, and using the new expansion, we extend the analysis of Friz et al. (Citation2018) to the case, where the volatility is driven by a rough fractional Brownian motion. Indeed, Laplace approximation methods on Wiener space in the spirit of Azencott (Citation1982, Citation1985), Ben Arous (Citation1988) and Bismut (Citation1984) can be adapted to the present context, so that our analysis builds upon this framework in a fractional setting. Unlike many other works in this field, we do not rely on density expansions. Finally, using a version of the ‘rough Bergomi model’ (Bayer et al. Citation2016), we demonstrate numerically that our implied volatility asymptotics capture very well the geometry of the term structure of implied volatility over a wide array of maturities, extending up to a year.
The paper is organized as follows: In Section 2 we set the scene, describing the class of models included in our framework ((Equation1(1)
(1) ) and (Equation2
(2)
(2) )) and recalling some known results ((Equation4
(4)
(4) ) and (Equation7
(7)
(7) )), which are the starting point of our analysis. Most importantly, we argue that for small-time considerations it would suffice to restrict our attention to a class of stochastic volatility models of the form (Equation3
(3)
(3) ) with a volatility process driven by a Gaussian Volterra process such as in (Equation2
(2)
(2) ). We formulate general assumptions on the Volterra kernel (Assumptions 2.1 and 2.5) and on the function σ in (Equation3
(3)
(3) ) (Assumption 2.4) under which our results are valid. In Section 3 we gather our main results, concerning a higher order expansion of the energy (Theorem 3.1), and a general expansion formula for the corresponding call prices. We derive the classical Black-Scholes expansion for the call price, using the latter result mentioned above. In addition, in Section 3 we formulate moderate deviation expansions, which allow us to derive the corresponding asymptotic formulae for implied volatilities and implied volatility skews. Finally, Section 4 displays our simulation results. Sections 5–7 are devoted to proofs of the energy expansion, the price expansion and the moderate deviations expansion, respectively. In the appendix, we have collected some auxiliary lemmas, which are used in different sections.
2. Exposition and assumptions
We consider a rough stochastic volatility model, normalized to r=0 and , of the form suggested by Forde and Zhang (Citation2017)
(1)
(1) Here
are two independent standard Brownian motions,
a correlation parameter, and
. Then
is another standard Brownian motion which has constant correlation ρ with the factor B, which drives the stochastic volatility
Here
is some real-valued function, typically smooth but not bounded, and we will denote by
the spot volatility, with
a Gaussian (Volterra) process of the form
(2)
(2) for some kernel K, which shall be further specified in Assumptions 2.1 and 2.5 below. The log-price
satisfies
(3)
(3) Recall that by Brownian scaling, for fixed t>0,
As a direct consequence, classical short-time SDE problems can be analyzed as small-noise problems on a unit time horizon. For our analysis, it will also be crucial to impose such a scaling property on the Gaussian process
(more precisely, on the kernel K in (Equation2
(2)
(2) )) driving the volatility process in our model:
Assumption 2.1
Small time self-similarity
There exists a number with
and a function
such that
In fact, we will always have
which covers the examples of interest, in particular standard fractional Brownian motion
or Riemann-Liouville fBM with explicit kernel
. (This is very natural, even from a general perspective of self-similar processes, see Lamperti Citation1962.)
We insist that no (global) self-similarity of is required, as only
for arbitrarily small t matters.
Remark 2.2
It should be possible to replace the fractional Brownian motion by a certain fractional Ornstein-Uhlenbeck process in the results obtained in this paper. Intuitively, this replacement creates a negligible perturbation (for ) of the fBm environment. A similar situation was in fact encountered in Cass and Friz (Citation2010), where fractional scaling at times near zero was important. To quantify the perturbation, the authors of Cass and Friz (Citation2010) introduced an easy to verify coupling condition (see Corollary 2 in Cass and Friz Citation2010). It should be possible to employ a version of this condition in the present paper to justify the replacement mentioned above. We will however not pursue this point further here.
Remark 2.3
Throughout this article, one can consider a classical (Markovian, diffusion) stochastic volatility setting by taking , or equivalently
, by simply ignoring all hats (
) in the sequel. In particular then,
in all subsequent formulae.
General facts on large deviations of Gaussian measures on Banach spaces (Deuschel and Stroock Citation1989) such as the path space imply that a large deviation principle holds for the triple
, with speed
and rate function
(4)
(4) where
for
, the space of absolutely continuous paths with
derivative
(5)
(5) This enables us to derive a large deviations principle for X in (Equation3
(3)
(3) ): the (local) small-time self-similarity property of
(Assumption 2.1) implies that
where
For what follows, it will be convenient to consider a rescaled version of (Equation3
(3)
(3) )
Under a linear growth condition on the function σ, Forde and Zhang (Citation2017) use the extended contraction principle to establish a large deviations principle for (
) with speed
. More precisely, with
(6)
(6) the rate function is given by
(7)
(7) where
denotes the inner product on
. Several other proofs (under varying assumptions on σ) have appeared since (Jacquier et al. Citation2017; Bayer et al. Citation2017; Gulisashvili Citation2017).
As a matter of fact, this paper relies on moderate – rather than large – deviations, as emphasized in (iiic) below. To this end, let us make
Assumption 2.4
Positive spot vol
Assume
is smooth with
.
Roughness
The Hurst parameter H satisfies
.
Martingality
The price process
is a martingale.
Short-time moments
.
While condition (iiia) hardly needs justification, we emphasize that conditions (iiia-b) are only used to the extent that they imply condition (iiic) given below (which thus may replace (iiia-b) as an alternative, if more technical, assumption). The reason we point this out explicitly is that all the conditions (iiia-c) are implicit (growth) conditions on the function . For instance, (iiia-b) was seen to hold under a linear growth assumption (Forde and Zhang Citation2017; Gulisashvili Citation2017), whereas the log-normal volatility case (think of
) is complicated. Martingality, for instance, requires
and there is a critical moment
, even when
. See Sin (Citation1998), Jourdain (Citation2004) and Lions and Musiela (Citation2007) for the case
and the forthcoming work (Friz and Gassiat Citation2018) for the general rough case
. We view (iiic) simply as a more flexible condition that can hold in situations where (iiib) fails.
(Call price upper moderate deviation bound) For every
, and every fixed x>0, and
,
This condition is reminiscent of the ‘upper part’ of the large deviation estimate obtained in Forde and Zhang (Citation2017)
(8)
(8) If fact, if one formally applies this with x replaced by
, followed by Taylor expanding the rate function,
one readily arrives at the estimate (iiic). Unfortunately,
in (Equation8
(8)
(8) ), which is a serious obstacle in making this argument rigorous. Instead, we will give a direct argument (Lemma 7.1) to see how (iiia-b) implies (iiic).
In the sequel, we will use another mild assumption on the kernel.
Assumption 2.5
The kernel K has the following properties
has a continuous
in
version on
.
.
Note that the Riemann-Liouville kernel ,
satisfies Assumption 2.5.
Remark 2.6
Assumption 2.5 implies that the Cameron-Martin space of
is given by the image of
under K, i.e.
See Lemma 5.3 and Remark 5.4 for more details. A reference and also a sufficient condition for Assumption 2.5 (i) can be found e.g. in Decreusefond (Citation2005, Section 3).
3. Main results
The following result can be seen as a non-Markovian extension of work by Osajima (Citation2015). The statement here is a combination of Theorem 5.10 and Proposition (5.14) below. Recall that represents spot-volatility. We also set
.
Theorem 3.1
Energy expansion
The rate function or energy
I in (Equation7
(7)
(7) ) is smooth in a neighborhood of x=0
at-the-money
and it is of the form
The next result is an exact representation of call prices, valid in a non-Markovian generality, and amenable to moderate- and large-deviation analysis (Theorem 3.4 below).
Theorem 3.2
Pricing formula
For a fixed log-strike and time to maturity
set
where
and
as before. Then we have
(9)
(9) where
and
is a random variable of the form
(10)
(10) with
a centred Gaussian random variable, explicitly given in equation (Equation38
(38)
(38) ) below, and
is a
random
remainder term, in the sense of a stochastic Taylor expansion in
see Lemma 6.2 for more details.
Example 3.3
Black-Scholes model
We fix volatility , and
so that
and all
can be omitted. Energy is given by
and
with
independent of ϵ. Moreover,
(11)
(11) with
, and, in terms of the standard Gaussian cdf Φ,
Using the expansion
, as
one deduces, for fixed x>0, the asymptotic relation, as
,
(12)
(12) We will be interested (cf. Theorem 3.4) in replacing x by
for
. This gives
and the above analysis, now based on
, remains validFootnote1 for β in the ‘moderate’ regime
and we obtain
(13)
(13) Let us point out, for the sake of completeness, that a similar expansion is not valid for
. To see this, first note that (Equation9
(9)
(9) ) implies that
is precisely the ATM call price with time
from expiration. Well-known ATM asymptotics then imply that
as
. These asymptotics are unchanged in case of
out-of-moneyness (‘almost-at-the-money’ in the terminology of Friz et al. Citation2018), which readily implies
At last, we have the borderline case
, or
. From e.g. Muhle-Karbe and Nutz (Citation2011, Theorem 3.1), we see that
with positive constant
. A look at (Equation9
(9)
(9) ) then reveals
For the call price expansion in the large / moderate deviations regime,
, the polynomial in ϵ-behavior of (Equation13
(13)
(13) ) implies that the J-term in the pricing formula will be negligible on the moderate / large deviation scale, in the sense for any
, we have
as
. Consequently, with
, for
, k>0,
, we get the ‘moderate’ Black-Scholes call price expansion,
While the above can be confirmed by elementary analysis of the Black–Scholes formula, the following theorem exhibits it as an instance of a general principle. See Friz et al. (Citation2018) for a general diffusion statement.
Theorem 3.4
Moderate Deviations
In the rough volatility regime consider log-strikes of the form
(i) For
and every
we have
(ii) For
and every
we have
Moreover,
where
is the inner product in
.
Remark 3.5
In principle, further terms (of order ,
) can be added to this expansion of log call prices, given that the energy has sufficient regularity, see Theorem 3.6. We also note that, for small enough β, the error term
can be omitted. In any case, one can replace the additive error bounds by (cruder) ones, where the right-most term in the expansion is multiplied with
, as was done in Friz et al. (Citation2018).
Proof of Theorem 3.4
We apply Theorem 3.2 with , i.e. with
. In particular, we so get, with
and
,
The technical Proposition 7.3 asserts that, for fixed k>0, the factor J is negligible in the sense that, for every
,
The theorem now follows immediately from the Taylor expansion of
around x=0 (see Theorem 3.1), plugging in
. Indeed, replacing
by the Taylor-jet seen in (i),(ii), leads exactly to an error term
, resp.
.
Fix real numbers k>0, ,
, and an integer
. For every
, set
and denote
Here,
can be arbitrarily small. It is clear that for all small t and θ small enough,
while
The following statement provides an asymptotic formula for the implied variance.
Theorem 3.6
Suppose and
small enough. Then as
and for
(14)
(14) The
-estimate in (Equation14
(14)
(14) ) depends on n, H, β, θ, and k. It is uniform on compact subsets of
with respect to the variable k.
Remark 3.7
Using the multinomial formula, we can represent the expression on the left-hand side of (Equation14(14)
(14) ) in terms of certain powers of t. However, the coefficients become rather complicated.
Remark 3.8
Let an integer be fixed, and suppose we would like to use only the derivatives
for
in formula (Equation14
(14)
(14) ) to approximate
. Then, the optimal range for β is the following:
. On the other hand, if β is outside of the interval
, more derivatives of the energy function at zero may be needed to get a good approximation of the implied variance in formula (Equation14
(14)
(14) ).
We will next derive from Theorem 3.6 several asymptotic formulas for the implied volatility. In the next corollary, we take n=2.
Corollary 3.9
As
(15)
(15)
Corollary 3.9 follows from Theorem 3.6 with n=2, the equality
(16)
(16) given in Theorem 3.4, and the Taylor expansion
as
.
In the next corollary, we consider the case where n=3.
Corollary 3.10
Suppose . Then, as
(17)
(17)
Corollary 3.10 follows from Theorem 3.6 with n=3, formula (Equation16(16)
(16) ), the equality
(18)
(18) (see Theorem 3.4), and the expansion
as
.
Using Corollary 3.10, we establish the following implied volatility skew formula in the moderate deviation regime.
Corollary 3.11
Let
and fix y,z>0 with
. Then as
(19)
(19)
Remark 3.12
Corollary 3.11 complements earlier works of Alòs et al. (Citation2007) and Fukasawa (Citation2011, Citation2017). For instance, the following formula can be found in Fukasawa (Citation2017, p. 6), see also Fukasawa (Citation2011, p. 14):
(20)
(20) In formula (Equation20
(20)
(20) ), we employ the notation used in the present paper. Our analysis shows that the applicability range of skew approximation formulas is by no means restricted to the Central Limit Theorem type log-moneyness deviations of order
. It also includes the moderate deviations regime of order
. The previous rate is clearly
as
.
Remark 3.13
Symmetry
Write for the ‘Itô-type map’
It equals, in law,
, and indeed all our formulae are invariant under this transformation. In particular, the skew remains unchanged when the pair
is replaced by
.
4. Simulation results
We verify our theoretical results numerically with a variant of the rough Bergomi model (Bayer et al. Citation2016) which fits nicely into the general rough volatility framework considered in this paper. As before, the model has been normalized such that and r=0. We let
be two independent Brownian motions and
with
such that
is another Brownian motion having constant correlation ρ with B. For some spot volatility
and volatility of volatility parameter η, we then assume the following dynamics for some asset S:
(21)
(21)
(22)
(22) where
is a Riemann-Liouville fBM given by
The approach taken for the Monte Carlo simulations of the quantities we are interested in is the one initially explored in the original rough Bergomi pricing paper (Bayer et al. Citation2016). That is, exploiting their joint Gaussianity, where we use the well-known Cholesky method to simulate the joint paths of
on some discretization grid
. With (Equation22
(22)
(22) ) being an explicit function in terms of the rough driver, an Euler discretisation of the Ito SDE (Equation21
(21)
(21) ) on
then yields estimates for the price paths.
The Cholesky algorithm critically hinges on the availability and explicit computability of the joint covariance matrix of whose terms we readily compute below.Footnote2
Lemma 4.1
For convenience, define constants and
and define an auxiliary function
by
(23)
(23) where
denotes the Gaussian hypergeometric function (Olver et al. Citation2010). Then the joint process
has zero mean and covariance structure governed by
Numerical simulationsFootnote3 confirm the theoretical results obtained in the last section. In particular – as can be seen in figure – the asymptotic formula for the implied volatility (Equation17(17)
(17) ) captures very well the geometry of the term structure of implied volatility, with particularly good results for higher H and worsening results as
. Quite surprisingly, despite being an asymptotic formula, it seems to be fairly accurate over a wide array of maturities extending up to a single year.
Figure 1. Illustration of the term structure of implied volatility of the Modified Rough Bergomi model in the Moderate deviations regime with time-varying log-strike . Depicted are the asymptotic formula (equation (Equation17
(17)
(17) ), dashed line) and an estimate based on
samples of a MC Cholesky Option Pricer (solid line) with 500 time steps. Model parameters are given by spot vol
, vvol
and correlation parameter
.
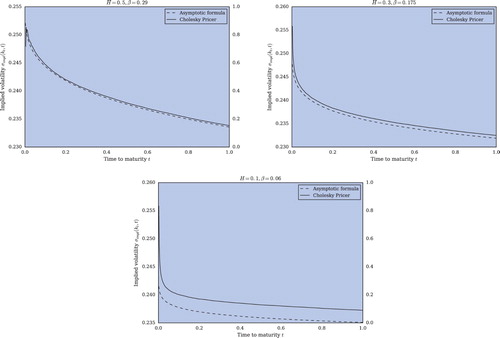
5. Proof of the energy expansion
Consider
where
for a fixed Volterra kernel (recall (Equation3
(3)
(3) ) in the previous section). We study the small noise problem
where
is replaced by
. The following proposition roughly says that
Proposition 5.1
Forde and Zhang Citation2017
Under suitable assumptions cf. Section 2), the rescaled process
satisfies an LDP
with speed
and rate function
(24)
(24) where
The rest of this section is devoted to analysis of the function I as defined in (Equation24(24)
(24) ). First, we derive the first order optimality condition for the above minimization problem.
Proposition 5.2
First order optimality condition
For any we have at any local minimizer
of the functional
in (Equation24
(24)
(24) ) that
(25)
(25) for all
.
Proof.
We denote whenever
for a small parameter δ. We expand
If
is a minimizer then
has a minimum at
for all g. We expand
As a consequence, we must have, for
and every
Recall
, any x. We now test with
for a fixed
and obtain
5.1. Smoothness of the energy
Having formally identified the first order condition for minimality in (Equation24(24)
(24) ), we will now show that the energy
is a smooth function. More precisely, we will use the implicit function theorem to show that the minimizing configuration
is a smooth function in x (locally at x=0). As
is a smooth function, too, this will imply smoothness of
, at least in a neighborhood of 0.
As the Cameron-Martin space of the process
continuously embeds into
, K maps
continuously into
, i.e. there is a constant C>0 such that for any
we have
(26)
(26) This result will follow from
Lemma 5.3
Let be a continuous, centred Gaussian process and
its Cameron-Martin space. Then we have the continuous embedding
. That is, for some constant C,
Proof.
By a fundamental result of Fernique, applied to the law of V as Gaussian measure on the Banach space , the random variable
has Gaussian integrability. In particular,
On the other hand, a generic element
can be written as
where Z is a centred Gaussian random variable with variance
, see, e.g. Friz and Hairer (Citation2014, page 150). By Cauchy–Schwarz,
and conclude by taking the
over on the l.h.s. over
.
Remark 5.4
Assume V is of Volterra form, i.e. . Then it can be shown (see Decreusefond Citation2005, Section 3) that
is the image of
under the map
and
. In particular then, applying the above with
, gives
5.1.1. The uncorrelated case
We start with the case as the formulas are much simpler in this case.
By Proposition 5.2, any local optimizer of the functional
in the uncorrelated case
satisfies for any
We define a map
by
(27)
(27) Hence, for given
, any local optimizer f must solve
. As one particular solution is given by the pair
, we are in the realm of the implicit function theorem. We need to prove that
is locally smooth (in the sense of Fréchet);
is invertible in
.
Note that invertibility should hold for x small enough, as for some R, which is invertible as long as R has a bounded norm for sufficiently small x.
Remark 5.5
The method of proof in this section is purely local in . Hence, we only really need smoothness of σ locally around 0. Note, however, that stochastic Taylor expansions used in Section 6 will actually require global smoothness of σ.
Lemma 5.6
The functions and
defined by
are smooth in the sense of Fréchet.
Proof.
For we note that the Gateaux derivative of F satisfies
By Lemma 5.3, we can bound
for
.Footnote4
Thus,
is a multi-linear form on
with operator norm
independent of f. As
is continuous, we conclude that
as given above is, in fact, a Fréchet derivative.
Let us next consider the functional . Note that
for
. Hence, Assumption 2.5 implies that
We see that the multi-linear map
has operator norm bounded by
independent of f. From continuity of
, it follows that
is the N'th Fréchet derivative.
Theorem 5.7
Zero correlation
Assuming the energy
as defined in (Equation24
(24)
(24) )) is smooth in a neighborhood of x=0.
Proof.
By construction, we have
for
defined by
Here,
As verified above, H is smooth in the sense of Fréchet. Trivially,
is invertible and
. Therefore, the implicit function theorem implies that there are open neighborhoods U and V of
and
, respectively, and a smooth map
from V to U such that
and
is unique in U with this property.
For the energy, we prove that in a neighborhood of x=0. First of all, we show that a minimizer exists. If not, there is a function
with
. For small enough x such a g must be inside a ball with radius ε around
, as
and
. Then note that for any
where
denotes the second derivative of
. By continuity,
stays positive definite for
in a neighborhood of
. As noted, for x small enough, both g and
(and the line connecting them) lie in this neighborhood. For
, this implies
since
and
. This contradicts the assumption that
, and we conclude that
is, indeed, a minimizer of
, implying that
locally.
Finally, as is smooth and
is smooth, we see that
is smooth in a neighborhood of 0. (Note that this arguments relies on
, implying that
for f in a neighborhood to 0.)
Remark 5.8
Classical counter-examples in the context of the direct method of calculus of variations show that the step of verifying the existence of a minimizer should not be taken too lightly. For instance, the functional
does not have a minimizer in
, but J can be made arbitrarily close to 0 by choosing piecewise-linear functions u with slope
oscillating around 0. We refer to any text book on calculus of variations. In the situation above, local ‘convexity’ in the sense of a positive definite second derivative prevents this phenomenon. An alternative method of proof for the existence of a minimizer is to show that J is (lower semi-) continuous in the weak sense.
5.1.2. The general case
In the general case (cf. Proposition 5.2), we define the function by
(28)
(28) where
are defined by
(29)
(29)
(30)
(30)
.
One easily checks that G, ,
are smooth in the Fréchet sense.
Lemma 5.9
The functions
and
are smooth in Fréchet sense.
Proof.
The proof of smoothness is clear. We report the actual derivatives. For G we get
For
and, respectively,
, we obtain
and
Theorem 5.10
Let σ be smooth with . Then the energy
as defined in (Equation24
(24)
(24) ) is smooth in a neighborhood of x=0.
Proof.
The proof is similar to the proof of Theorem 5.7. In fact, the only difference is in establishing invertibility of and the existence of a minimizer.
Note that (Equation28(28)
(28) ) contains three terms. The derivative of the first term (
) is always equal to
. For the second term, we note that
Hence, the only non-vanishing contribution to the derivative of the second term evaluated in direction
at x=0, f=0 and
is
For the same reason, the derivative of the third term at
vanishes entirely. Hence,
It is easy to see that
is invertible. Indeed, let us construct the pre-image
of some
. At t=1 we have
implying
. For
, we then get
or
.
For existence of the minimizer, note that
which is again positive definite.
Remark 5.11
Though only formulated in terms of ‘smoothness’, it is easy to show that implies that
(locally at 0).
5.2. Energy expansion
Having established smoothness of the energy I as well as of the minimizing configuration
locally around x=0, we can proceed with computing the Taylor expansion of
around x=0. We will once more rely on the first order optimality condition given in Proposition 5.2. Plugging the Taylor expansion of
into
will then give us the local Taylor expansion of
.
5.2.1. Expansion of the minimizing configuration
Theorem 5.12
We have
Remark 5.13
Non-Markovian transversality
In the RL-fBM case, with
one computes
Interestingly, the transversality condition known from the Markovian setting (
, which readily translates to
there) remains valid here (for
), at least to order
, in the sense that
Proof of Theorem 5.12
First order expansion:
Up to the order needed in order to get the first order term, we have
Therefore,
This yields for the first order term in (Equation25
(25)
(25) )
Setting t=1, we get
which is solved by
. Inserting this term back into the equation for
, we get
(31)
(31)
Second order expansion:
Using (Equation31(31)
(31) ) and the ansatz
, we re-compute the relevant terms appearing in the (Equation25
(25)
(25) ). We have
and analogously for σ replaced by
,
. This implies
Using the notation introduced earlier, we have
This directly implies
We next compute some auxiliary terms appearing in (Equation25
(25)
(25) ).
The corresponding denominator is
. Using the formula
we obtain
(32)
(32) For the second term in (Equation25
(25)
(25) ), let
The corresponding denominator is
. Hence,
(33)
(33) Combining (Equation32
(32)
(32) ) and (Equation33
(33)
(33) ), we get
We shall next compute
. Taking the second order terms on both sides and letting t=1, we obtain
Moving
to the other side with
and collecting terms on the right hand side, we arrive at
We conclude that
Hence, we obtain
5.2.2. Energy expansion in the general case
Now we compute the Taylor expansion of as defined in Proposition 5.1. We start with the second term. Plugging in the optimal path
(and using
as
) we obtain
Inserting
into the above formula for
, we get
Recall the denominator
Using the expansion of a fraction
we obtain from
We note that
Adding both terms, we arrive at the
Proposition 5.14
The energy expansion to third order gives
5.2.3. Energy expansion for the Riemann-Liouville kernel
Let us specialize the energy expansion given in Proposition 5.14 for the Riemann-Liouville fBm. Choose and recall that the kernel K takes the form
. We get
The key term
appearing in the energy expansion now gives
Plugging the above formula into the energy expansion, we obtain the energy expansion for the Riemann-Liouville fractional Browian motion
For completeness, let us also fully describe the time-dependence of the second order term
in the expansion of the optimal trajectory
. Unlike the first order time, here we do not have a linear movement any more. Indeed
(34)
(34)
(35)
(35)
6. Proof of the pricing formula
Fix and
where
and
. We have
where we recall
Consider a Cameron-Martin perturbation of
. That is, for a Cameron-Martin path
consider a measure change corresponding to a transformation
(transforming the Brownian motions to Brownian motions with drift), we obtain the Girsanov density
(36)
(36) Under the new measure,
becomes
, where
Definition 6.1
For fixed , write
if
. Call such
admissible for arrival at log-strike x. Call
the cheapest admissible control, which attains
where we recall that
and
For any Cameron-Martin path , the perturbed random variable
admits a stochastic Taylor expansion with respect to
.
Lemma 6.2
Fix and define
accordingly. Then
(37)
(37) where
is a Gaussian random variable, given explicitly by
(38)
(38) and
(39)
(39)
Proof.
By a stochastic Taylor expansion for the controlled process with control
as in Definition 6.1 and thanks to
, we have at t=1
Collecting terms in powers of
and with the random variable
as in (Equation38
(38)
(38) ) (recalling that
), we have
furthermore, since
, by the definition of
, it holds that
This proves the statement (Equation37
(37)
(37) ) and the statement that
is Gaussian is immediate from the form (Equation38
(38)
(38) ).
Finally, we determine an explicit form of the Girsanov density for the choice where
in (Equation36
(36)
(36) ) are chosen the cheapest admissible control (cf. Definition 6.1. Similarly to classical works of Azencott, Ben Arous and others, see, for instance, Ben Arous (Citation1988), we show that the stochastic integrals in the exponent of
are proportional to the first order term
(with factor
) when evaluated at the minimizing configuration
.
Lemma 6.3
We have
Proof.
See Lemma A.2.
With these preparations in place, we are now ready to prove the pricing formula from Section 3.
Proof of Theorem 3.2
With a Girsanov factor (all integrals on )
and (evaluated at the minimizer)
we have, setting
7. Proof of the moderate deviation expansions
In Section 2, we pointed out that (iiic) is exactly what one gets from (call price) large deviations (Equation8(8)
(8) ), if heuristically applied to
. We now give a proper derivation based on moderate deviations.
Lemma 7.1
Assume (iiia-b) from Assumption 2.4. Then an upper moderate deviation estimate holds both for calls and digital calls. That is, we have
For every
and every fixed
and
and also
(40)
(40)
Proof.
Recall smooth but unbounded and recall
. In case of
and
a large deviation principle (LDP) for
is readily reduced, via exponential equivalence, to a LDP for the family of stochastic Itô integrals given by
for some Brownian Z, ρ-correlated with B. There are then many ways to establish a LDP for this family. A particularly convenient one, that requires no growth restriction on σ, uses continuity of stochastic integration with respect to the rough path
in suitable metrics, for which a LDP is known (Friz and Hairer Citation2014, Ch 9.3). It was pointed out in Bayer et al. (Citation2017) that a similar reasoning is possible when
, the rough path is then replaced by a ‘richer enhancement’ of
, the precise size of which depends on H, for which again one has a LDP. A moderate deviation priniple (MDP) for
is a LDP for
for
. This can be reduced to a LDP, with
, for
with speed
. Since
converges (with all derivatives) locally uniformly to the constant function
, and one checks that the above is exponentially equivalent to the (Gaussian) family given by
, with law
which gives (Equation40
(40)
(40) ), even with equality. (By localization, exponential equivalence can again be done for σ without growth restrictions.)
We have not yet used either assumption (iiia-b). These become important in order to extend estimate (Equation40(40)
(40) ) to the case of genuine call payoffs. We can follow here a well-known argument (e.g. Forde and Jacquier Citation2009; Pham Citation2010; Forde and Zhang Citation2017) with the ‘moderate’ caveat to carry along a factor
. In fact, this is follows precisely the argument of Forde and Zhang (Citation2017) where the authors carry along a factor
. (This provides a unified view on rough and moderate deviations.) The remaining details then follow essentially ‘Appendix C. Proof of Corollary 4.13., part (ii) upper bound’ of Forde and Zhang (Citation2017), noting perhaps that the authors use their assumptions to show validity of what we simply assumed as condition (iiib), and also that one works with the quadratic rate function
throughout.
Remark 7.2
By an easy argument similar to ‘Appendix C. Proof of Corollary 4.13., part (i) lower bound’ of Forde and Zhang (Citation2017) one sees that validity of the call price upper bound (iiic) implies the corresponding digital call price upper bound (Equation40(40)
(40) .) For this reason, we only emphasized (iiic) but not (Equation40
(40)
(40) ) in Section 2.
In a classical work Azencott (Citation1982) (see also Azencott Citation1985; Ben Arous Citation1988, Théorème 2) obtained asymptotic expansions of functionals of Laplace type on Wiener space, of the type ‘’, for small noise diffusions
. This refines the large deviation (equivalently: Laplace) principle of Freidlin–Wentzell for small noise diffusions. In a nutshell, for fixed
, Azencott gets expansions of the form
. His ideas (used by virtually all subsequent works in this direction) are a Girsanov transform, to make the minimizing path ‘typical’, followed by localization around the minimizer (justified by a good large deviation principle), and finally a local (stochastic Taylor) type analysis near the minimizer. None of these ingredients rely on the Markovian structure (or, relatedly, PDE arguments). As a consequence (and motivation for this work) such expansions were also obtained in the (non-Markovian) context of rough differential equations driven by fractional Brownian motion (Inahama Citation2013; Baudoin and Ouyang Citation2015) with
.
And yet, our situation is different in the sense that call price Wiener functionals do not fit the form studied by Azencott and others, nor can we in fact expect a similar expansion: Example 3.3 gives a Black-Scholes call price expansion of the form constant times . Azencott's ideas are nonetheless very relevant to us: we already used the Girsanov formula in Theorem 3.2 in order to have a tractable expression for J. It thus ‘only’ remains to carry out the localization and do some local analysis.
Proposition 7.3
Let x>0 and . Then the factor J is negligible in the sense that, for every
Proof.
Step 1. Localization Write . By definition,
Fix
and write
. We claim that (the positive quantity)
(41)
(41) is exponentially small, in the sense that, for some c>0 and
,
There is a battle here between the exploding factor
, with exponent
and on the other hand
where the given estimate is an easy consequence of Lemma 7.1. Since
we see that the last factor ‘exponentially over-compensates’ the rest, so that the difference is indeed exponentially negligible.
Step 2. Upper bound. For any x>0, recall that decomposes into a Gaussian random variable
and remainder
. In order to control this remainder without imposing boundedness assumption on
, we will crucially used a ‘localized remainder tail estimate’ as given in Proposition 7.4 below. We have, for any
,
(42)
(42) To proceed, recall
so that, for any
,
Since
for small enough x>0, it follows that
on the event
, which leads us to
where, by Proposition 7.4, the constant
is uniform in small ϵ and x. The square-root terms are computed resp. (Fernique) estimated by
for some c>0 which depends on the law of B (hence H), but is uniform in ϵ and x. Hence, for x small enough, the resulting exponent
is negative, which is more than enough to conclude the upper bound.
Step 3. Lower bound. Write and estimate
where we used Cauchy–Schwarz and discarded the event
. The localized remainder estimate provides an upper bound on
, uniformly over small (enough) ϵ and x.
It then suffices to get a suitable lower bound of the left-hand side above. Indeed, for , with η small enough, not dependent on ϵ,
(43)
(43) for a constant
which can also be taken uniformly in small
. Then estimate
As a quick sanity check, pretend zero remainder so that
: dropping further the (exponentially close to probability one) event
, a Gaussian computation then shows that we are left with (
times
times)
In general, set
, so thatFootnote5
At this stage, it is difficult to treat
as perturbation of g since, on the given event
, all terms are of order
. We can solve this issue by realizing that we can replace, throughout, x by
. Since
, with see from (Equation43
(43)
(43) ), that in the above estimate the event
(resp.
) can be replaced by
(resp.
), possibly with an insignificantly modified constant η. It is now straight-forward to show that the behavior of
is of the same order as
, the correct behavior (i.e. positive power of
is obtained by spelling out the (Gaussian) integral.
Proposition 7.4
Localized remainder tail estimate
For every there exists
such that, for all r and uniformly in small
we have
Proof.
We decompose in terms of the (local) martingale
and the (bounded variation) process
Let
be the stopping time when
first leaves the uniform ball of radius κ. Then
still yields a (local) martingale. The point is that
. On this event,
and we can thus replace
, in the definition of the remainder, by
. Let
be the κ-fattening of
, recall
, then, for
,
Clearly, we can replace K by
which contains all
for small x. To summarize, we have, on the event
,
with
and, as seen by a similar (but easier) reasoning,
, always for fixed
, but uniformly in small ϵ (equivalently,
) and small x>0. This clearly shows that
has exponential tails. The same is true for the martingale part, whose bracket is
. This is exactly the situation for the ‘model’ martingal increment
which clearly has exponential tails. To make this rigorous, recall that Gaussian resp. exponential tails are characertized by
resp.
-growth of the
-norms. The statement is then an easy consequence of the sharp (upper) BDG constant (Carlen and Kree Citation1991), known to be
.
8. Proof of the implied volatility expansion
With Theorem 3.2 in place, we now turn to the proof of the implied volatility expansion, formulated in Theorem 3.6.
Proof of Theorem 3.6
We will use an asymptotic formula for the dimensionless implied variance
obtained in Gao and Lee (Citation2014). It follows from the first formula in Remark 7.3 in Gao and Lee (Citation2014) that
(44)
(44) where
, t>0.
We will need the following formula that was established in the proof of Theorem 3.4:
(45)
(45) as
, for all
and
and any
. Let us first assume
. Using the energy expansion, we obtain from (Equation45
(45)
(45) ) that
(46)
(46) as
. The second term in the brackets on the right-hand side of (Equation46
(46)
(46) ) disappears if n=2.
Remark 8.1
Suppose and
. Then formula (Equation46
(46)
(46) ) is optimal. Next, suppose
and
. In this case, there exists
such that
, and hence (Equation46
(46)
(46) ) holds with m instead of n. However, we can replace m by n, by making the error term worse. It is not hard to see that the following formula holds for all
and
:
(47)
(47) as
provided we choose θ small enough.
Let us continue the proof of Theorem 3.6. Since and
as
, (Equation44
(44)
(44) ) implies that
(48)
(48) Next, using the Taylor formula for the function
, and setting
we obtain from (Equation46
(46)
(46) ) that
as
. It follows from
that
, and hence
as
. Now, (Equation48
(48)
(48) ) gives
as
. Finally, by canceling a factor of t in the previous formula, we obtain formula (Equation14
(14)
(14) ) for
. The proof in the case where
is similar. Here we take into account Remark 8.1. This completes the proof of Theorem 3.6.
Acknowledgments
Two referees are thanked for their useful comments. We further thank Martin Forde for valuable feedback.
Disclosure statement
No potential conflict of interest was reported by the authors.
Additional information
Funding
Notes
† More terms in the expansion of Φ are needed.
† Note that expressions for the exact same scenario have have been computed before in the original pricing paper (Bayer et al. Citation2016), yet in that version the expression for the autocorrelation of the fBM was incorrect. We compute and state here all the relevant terms for the sake of completeness.
† The Python 3 code used to run the simulations can be found at github.com/RoughStochVol.
† More precisely, since neither σ nor its derivatives need to be bounded, we need to actually work with a local version of the above estimate, for instance by replacing the max with a sup over a compact set containing .
† Write for the expected valued restricted to the event
References
- Alòs, E., León, J.A. and Vives, J., On the short-time behavior of the implied volatility for jump-diffusion models with stochastic volatility. Finance Stoch., 2007, 11(4), 571–589. doi: 10.1007/s00780-007-0049-1
- Azencott, R., Formule de Taylor stochastique et développement asymptotique d'intégrales de Feynman. In Seminar on Probability, XVI, Supplement, volume 921 of Lecture Notes in Math., pp. 237–285, 1982 (Springer: Berlin-New York).
- Azencott, R., Petites perturbations aléatoires des systemes dynamiques: développements asymptotiques. Bull. Sci. Math., 1985, 109(3), 253–308.
- Baudoin, F. and Ouyang, C., On small time asymptotics for rough differential equations driven by fractional Brownian motions. In Large Deviations and Asymptotic Methods in Finance, edited by P.K. Friz, J. Gatheral, A. Gulisashvili, A. Jacquier, and J. Teichmann, pp. 413–438, 2015 (Springer International Publishing: Cham).
- Bayer, C., Friz, P.K., Gassiat, P., Martin, J. and Stemper, B., A regularity structure for rough volatility. Preprint, 2017. arXiv:1710.07481.
- Bayer, C., Friz, P.K. and Gatheral, J., Pricing under rough volatility. Quant. Finance, 2016, 16(6), 887–904. doi: 10.1080/14697688.2015.1099717
- Ben Arous, G., Methods de Laplace et de la phase stationnaire sur l'espace de Wiener. Stochastics, 1988, 25(3), 125–153. doi: 10.1080/17442508808833536
- Bennedsen, M., Lunde, A. and Pakkanen, M.S., Decoupling the short- and long-term behavior of stochastic volatility. Preprint, 2016. arXiv:1610.00332.
- Bennedsen, M., Lunde, A. and Pakkanen, M.S., Hybrid scheme for Brownian semistationary processes. Finance Stoch., 2017, 21(4), 931–965. doi: 10.1007/s00780-017-0335-5
- Bismut, J.-M., Large Deviations and the Malliavin Calculus. Progress in Mathematics, Vol. 45. 1984 (Birkhäuser Boston, Inc.: Boston, MA).
- Carlen, E. and Kree, P., Estimates on iterated stochastic integrals. Ann. Probab., 1991, 19(1), 354–368. doi: 10.1214/aop/1176990549
- Cass, T. and Friz, P., Densities for rough differential equations under Hörmander's condition. Ann. Math., 2010, 0, 2115–2141. doi: 10.4007/annals.2010.171.2115
- Decreusefond, L., Stochastic integration with respect to Volterra processes. Ann. l. H. Poincare Probab. Statist., 2005, 41(2), 123–149. doi: 10.1016/j.anihpb.2004.03.004
- Deuschel, J.-D., Friz, P.K., Jacquier, A. and Violante, S., Marginal density expansions for diffusions and stochastic volatility I: Theoretical foundations. Comm. Pure Appl. Math., 2014a, 67(1), 40–82. doi: 10.1002/cpa.21478
- Deuschel, J.-D., Friz, P.K., Jacquier, A. and Violante, S., Marginal density expansions for diffusions and stochastic volatility II: Applications. Comm. Pure Appl. Math., 2014b, 67(2), 321–350. doi: 10.1002/cpa.21483
- Deuschel, J.-D. and Stroock, D.W., Large Deviations, Vol. 137, 1989 (Academic Press: Boston, MA).
- El Euch, O. and Rosenbaum, M., The characteristic function of rough Heston models. Preprint, 2016. To appear in Math. Finance.
- Forde, M. and Jacquier, A., Small-time asymptotics for implied volatility under the heston model. Int. J. Theoret. Appl. Finance, 2009, 12(06), 861–876. doi: 10.1142/S021902490900549X
- Forde, M. and Zhang, H., Asymptotics for rough stochastic volatility models. SIAM J. Financ. Math., 2017, 8(1), 114–145. doi: 10.1137/15M1009330
- Friz, P.K. and Gassiat, P., Martingality and moments for lognormal rough volatility. In preparation, 2018.
- Friz, P.K., Gerhold, S. and Pinter, A., Option Pricing in the Moderate Deviations Regime. Math. Finance, 2018, 28(3), 962–988. doi: 10.1111/mafi.12156
- Friz, P. and Hairer, M., A Course on Rough Paths, 2014 (Springer: Cham).
- Fukasawa, M., Asymptotic analysis for stochastic volatility: martingale expansion. Finance Stoch., 2011, 15(4), 635–654. doi: 10.1007/s00780-010-0136-6
- Fukasawa, M., Short-time at-the-money skew and rough fractional volatility. Quant. Finance, 2017, 17(2), 189–198. doi: 10.1080/14697688.2016.1197410
- Gao, K. and Lee, R., Asymptotics of implied volatility to arbitrary order. Finance Stoch., 2014, 18(2), 349–392. doi: 10.1007/s00780-013-0223-6
- Gatheral, J., The Volatility Surface: A Practitioner's Guide, 2011 (John Wiley & Sons: Hoboken, NJ).
- Gatheral, J., Jaisson, T. and Rosenbaum, M., Volatility is rough. Preprint, 2014. To appear in Quant. Finance.
- Guennoun, H., Jacquier, A. and Roome, P., Asymptotic behaviour of the fractional Heston model. Preprint, 2014. arXiv:1411.7653.
- Gulisashvili, A., Large deviation principle for Volterra type fractional stochastic volatility models. ArXiv e-prints, October 2017. To appear in SIAM J. Financ. Math.
- Inahama, Y., Laplace approximation for rough differential equation driven by fractional brownian motion. Ann. Probab., 2013, 41(1), 170–205. doi: 10.1214/11-AOP733
- Jacquier, A., Pakkanen, M.S. and Stone, H., Pathwise large deviations for the Rough Bergomi model. ArXiv e-prints, June 2017.
- Jourdain, B., Loss of martingality in asset price models with lognormal stochastic volatility. Int. J. Theoret. Appl. Finance, 2004, 13, 767–787.
- Lamperti, J., Semi-stable stochastic processes. Trans. Am. Math. Soc., 1962, 104(1), 62–78. doi: 10.1090/S0002-9947-1962-0138128-7
- Lions, P.-L. and Musiela, M., Correlations and bounds for stochastic volatility models. Ann. Inst. H. Poincaré Anal. Non Linéaire, 2007, 24(1), 1–16.
- Medvedev, A. and Scaillet, O., A simple calibration procedure of stochastic volatility models with jumps by short term asymptotics. Preprint, 2003. Available at SSRN 477441.
- Medvedev, A. and Scaillet, O., Approximation and calibration of short-term implied volatilities under jump-diffusion stochastic volatility. Rev. Financ. Stud., 2007, 20(2), 427–459. doi: 10.1093/rfs/hhl013
- Mijatović, A. and Tankov, P., A new look at short-term implied volatility in asset price models with jumps. Math. Finance, 2016, 26(1), 149–183. doi: 10.1111/mafi.12055
- Muhle-Karbe, J. and Nutz, M., Small-time asymptotics of option prices and first absolute moments. J. Appl. Probab., 2011, 48(4), 1003–1020. doi: 10.1239/jap/1324046015
- Olver, F.W.J., Lozier, D.W., Boisvert, R.F. and Clark, C.W (Eds.), NIST Handbook of Mathematical Functions, 2010 (Cambridge University Press: New York).
- Osajima, Y., The asymptotic expansion formula of implied volatility for dynamic SABR model and FX hybrid model. Preprint, 2007. Available at SSRN 965265.
- Osajima, Y., General asymptotics of Wiener functionals and application to implied volatilities, In Large Deviations and Asymptotic Methods in Finance, edited by P.K. Friz, J. Gatheral, A. Gulisashvili, A. Jacquier, and J. Teichmann, pp. 137–173, 2015 (Springer International Publishing: Cham).
- Pham, H., Large deviations in mathematical finance, 2010. Available online at: https://www.lpsm.paris/pageperso/pham/GD-finance.pdf.
- Sin, C.A., Complications with stochastic volatility models. Adv. Appl. Probab., 1998, 30(1), 256–268. doi: 10.1239/aap/1035228003
Appendix. Auxiliary lemmas
In this section we provide and prove some auxiliary lemmas, which are used in the preparations to the proof of Theorem 3.2. We start with a technical Lemma, that justifies the derivation.
Lemma .1
Assume and
. Then
is a Hilbert manifold near any
.
Proof.
Similar to Bismut (Citation1984, p. 25) we need to show that is surjective where
with
From
the functional derivative
can be computed explicitly. In fact, even the computation
is sufficient to guarantee surjectivity of
.
We now give the proof of Lemma 6.3, which determines the form of the Girsanov measure change (Equation36(36)
(36) ) for the minimizing configuration.
Lemma A.2
(i) Any optimal control is a critical point of
(ii) it holds that
Proof.
(Step 1) Write and
Let
an optimal control. Then
(This requires
to be a Hilbert manifold near
, as was seen in the last lemma.)
(Step 2) For fixed , define
with equality at t=0 (since
and
) and non-negativity for all t because
is an admissible control for reaching
(so that
.)
(Step 3) We note that is a consequence of
near 0,
and
. In other words,
is a critical point for
(Step 4) The functional derivative of this map at
must hence be zero. In particular, for all
,
(Step 5) With and
By continuous extension, replace by
above and note that
since indeed
. Hence