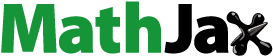
Abstract
The Commodity Futures Trading Commission (CFTC) has recently licensed a commercial prediction market to operate in the US. With regulatory restrictions lifted, these markets can now play the important role that has been often envisaged for them. For example, investors can use them to hedge various event-related risks directly rather than indirectly via portfolios expected to move a certain way if events occur. Commercial prediction markets charge fees, an element that has not been incorporated into previous theoretical work on these markets. We examine the impact of fees on prediction market prices and returns by introducing them to a model in which the market price equals the true probability when there are no fees. We find that existing fee models mean contract prices for low probability outcomes are below the true probability but the impact of fees means prediction markets feature a form of favorite-longshot bias: Post-fee loss rates depend negatively on the probability of the event being backed. We show this result holds even if prediction market operators set a fee structure that is more generous to contracts with a low probability of success.
1. Introduction
Prediction markets facilitate people to place wagers that events will happen by matching them with other people who wager that the event will not happen. These markets have long been considered potentially useful for their ability to summarize the public's information. Governments can use them to obtain information about the likelihood of events to assist with policy. Businesses can design their strategy using information about the likelihood of certain events. Investors can use them to hedge various event-related risks directly rather than indirectly via portfolios expected to move a certain way if events occur. Popular books by Surowiecki (Citation2004) and Sunstein (Citation2006) have promoted the idea that these markets should be much more widely used.
The development of prediction markets has been limited, however, by regulatory restrictions in the United States. A 2008 article in Science by 22 high profile social scientists including four economics Nobel prize winners, recommended reform of these restrictions and argued the case for ‘The Promise of Prediction Markets’ (Arrow et al. Citation2008). This promise may be about to be delivered on because the Commodity Futures Trading Commission (CFTC) decided in November 2020 to license a market of this type offered by startup firm Kalshi.Footnote1 Kalshi has begun trading as a licensed prediction market—officially its products are considered swaps—and it seems likely that competitors will soon join the market.
An important difference between this new era of commercial prediction markets and the Iowa Electronic Markets (IEM) site that has been widely studied in the previous literature is that firms like Kalshi are commercial operations and they charge trading fees, while trading with the IEM was commission-free. Longer running commercial prediction market operators outside the US also earn fees by charging commissions on winnings. Previous theoretical work has assumed the absence of fees, so in this paper, we examine how fees will affect prices and participants in prediction markets.Footnote2
We first look at whether fees impact the ability of prediction market prices to accurately summarize the public's beliefs. In theory, the conditions for prices in prediction markets without fees to equal the public's average belief are restrictive. Manski (Citation2006) showed that when observing a price p in a prediction market, the average belief could be as low as or as high as
. He and Treich (Citation2017) show that if participants have CRRA utility functions, prediction market prices equal the average belief of participants if and only they have log utility, even if the distribution of beliefs is symmetric. Ottaviani and Sørensen (Citation2015) also show that if agents combine prior beliefs with a common public signal, prediction market prices will under-react to the signal.
Despite these theoretical results, the evidence suggests that prediction markets without fees work extremely well. In particular, the extensively studied IEM has consistently produced accurate forecasts that have beaten other sources of predictions such as opinion polls.Footnote3 One caveat to this is the evidence presented by Page and Clemen (Citation2013) that prices from the InTrade prediction market tended to be too high for longshots and too low for favorites but this bias was relatively small and largely applied to very long-dated contracts. Since prediction markets without fees appear to work well in practice, we are going to assume they work in theory. In our model, agents have log utility and symmetric beliefs that are correct on average about probabilities so the prediction market price equals the true probability when the market has no fees. We then illustrate the implications of adding fees to the model.
We find that prediction market prices are affected by fees and will generally not equal the corresponding average value of the public's beliefs, though the precise pattern depends on the form of the fee structure. For example, a flat trading fee across all contract types and a flat commission rate on winnings both lead to prices being lower than probabilities when the true probability of success is below a half with the opposite applying for more likely outcomes. However, we also provide examples of fee structures that lead to prices for outcomes that have a probability below a half being higher than the true probability. Overall, however, we find that the deviations of prices from the public's average beliefs are generally modest for realistic commission rates.
We also examine how fees impact the returns on money invested by participants. Without fees, prediction markets just exchange money between two parties so on average there are no profits or losses but once the market charges fees, participants lose money on average. We show that these losses follow a pronounced pattern: Contracts backing low probability outcomes lose a greater percentage of their investment than contracts backing higher probability outcomes. This result echoes the well-known ‘favorite-longshot bias’ from the literature on sports betting. However, while researchers have come up with many possible behavioral explanations for this bias, the explanation in this context is somewhat different.Footnote4
Consider a fixed fee F on both sides of a contract. We show that in this case the price, p, of a contract tends to be pretty close to the true probability, π. This means that pre-fee average returns will be approximately zero so the average loss on all contracts is approximately F. Average loss rates on investments backing events with are greater than for taking the other side because F is larger relative to the financial investment of
than it is relative to the other side of the contract which has an investment of
. This result generalizes to more complex fee structures. While the model does not contain asymmetries so that people systematically overweight small probabilities—beliefs about probabilities are symmetric around the true value—those who buy contracts with a low probability of winning turn out to be proportionately more out of line in their assessment of their contract's rate of return than those who buy contracts likely to succeed.
The rest of the paper is organized as follows. Section 2 introduces the model and its predictions in the absence of fees. Section 3 describes the impact of a flat trading fee for all contracts. Section 4 examines the fee formula currently used by Kalshi in which contract fees depend on the price and shows this is less distortionary than flat fees. Section 5 analyzes the more common approach of charging a commission rate only on winnings and Section 6 provides two examples of fee structures that are more generous to longshot contracts but shows that these contracts still have larger loss rates in these cases because the prices of longshot contracts are raised above their true probabilities.
2. The model without fees
Here we introduce the model without fees and the subsequent sections then examine the impact of different fee structures. We model a prediction market as follows, following similar presentations in Gjerstad (Citation2004) and Wolfers and Zitzewitz (Citation2006). There is an event in which an outcome has a probability π of occurring. There is a continuum of potential market participants of size 1 who each have a subjective belief about the true probability π that is independently distributed across the population. As in Ali (Citation1977), these beliefs are characterized by a continuous symmetric cumulative distribution function
with mean π, meaning the potential participants are, on average, correct in their belief about π.
The prediction market operator arranges trading in an Arrow-Debreu security that has price p and pays out 1 if the event occurs and zero otherwise. Trading takes place via peer-to-peer matching arranged by the prediction market operator. This means that for every ‘back contract’ on the event happening, there is a corresponding ‘lay contract’ on it not happening. The person buying the back contract commits p in funds and the person buying the lay contract commits 1−p and then one of them wins the combined funds of 1. Lay contracts are thus Arrow-Debreu securities with price 1−p that pay out 1 when the event does not happen and zero when it does.
Participants have log utility and wealth of that is uncorrelated with their beliefs about π, so we assume the same distribution of wealth
for all values of
. In making their decisions on purchasing contracts, participants take any trading fees or commissions on winnings into account. The trading process is a double auction with agents submitting the quantities of each type of contract they would buy at each price. The equilibrium price is the one that matches demand for back contracts with demand for lay contracts.
With no fees, participant i solves the problem
(1)
(1)
where a positive
denotes purchases of back contracts and a negative
represents purchases of lay contracts. The optimal quantity of contracts is
(2)
(2)
Those who believe the contract is under-priced (those with
) will want to buy positive amounts while others will want to buy negative amounts.
The equilibrium price equates the volume of back and lay contracts so the average value of is zero.
(3)
(3)
The independence of wealth and beliefs means this can be simplified to
(4)
(4)
so the equilibrium price equals the true probability
(5)
(5)
The expected losses on these two contracts are
(6)
(6)
(7)
(7)
so both contracts break even on average.
3. A flat trading fee on all contracts
We first model the impact of a trading fee F charged on each contract. Optimal contract demand now solves
(8)
(8)
The first-order condition is
(9)
(9)
where
(10)
(10)
The first-order condition can be re-expressed as a formula determining the ratio of the marginal utilities of wealth for the two outcomes
(11)
(11)
The left side equals one when x = 0 and depends negatively on x. To have
, this ratio of marginal utilities has to be less than one, which requires
(12)
(12)
Similarly, x<0 requires
(13)
(13)
This means that people with beliefs such that
will not buy any contracts. Ironically, given the hope that prediction markets will accurately summarize the public's information, it turns out to be those with the most accurate beliefs who chose not to participate in the market.
For those who purchase contracts, the condition determining the quantity demanded is the same as before except now the effective prices for contracts are p + F and 1−p + F rather than p and 1−p. Contract demands are
(14)
(14)
(15)
(15)
and the equilibrium price satisfies
(16)
(16)
If these two denominators were the same, we could use the symmetry of the probability distribution of beliefs and the symmetry of the numerators of the demand functions to show that the equilibrium price continues to be
despite the introduction of the trading fee. The different denominators means this is not generally true. However, it is true for
and for most of the range of feasible values of π, the ratio of these two denominators is close to one. So for most values of π we will have
.
The exception is when p is either very high or low. The denominator of the demand function is a logistic function of the effective price of the contract. For contracts with a low price, the addition of even a small fee raises this function a lot more than it does for contracts with a high price. So, for example, consider the case where p = 0.1 and F = 0.01 (we describe below why this is a realistic baseline value for F). For these values, the term is 16 percent higher than
. For the same gap between beliefs and prices, demand for the back contract at p = 0.1 will be 16 percent lower than the corresponding demand for the matching lay contract that costs 1−p = 0.9. This lower demand for longshot contracts means the equilibrating market prices will be lower than π when
and higher than it when
.
In the absence of an analytical solution, we need to specify the probability density function of beliefs and solve the model numerically to illustrate its properties. We set F = 0.01 and use the assumption that beliefs are uniform on
where
. With this specification, when
beliefs go from
to
and this range becomes gradually smaller as π moves towards either zero or one. This calibration of disagreement is chosen because intuitively there is less room for sensible people to disagree if an event is almost certain to happen or not happen than if it is a toss-up and it avoids assigning people probability beliefs that are either negative or greater than one.Footnote5 However, the same patterns shown here emerge for all reasonable belief specifications examined, including using Normal distributions.
The upper panel of Figure shows that for most values of π, prices are close to the true probability but for contracts that are less likely to succeed, prices drop in a nonlinear fashion below the true probability. In this calibration, prices for the lowest probability contracts head towards about 15% below the true probability. This figure suggests there may be an asymmetry because back contracts with are a larger percentage below their true probabilities than contract with
. However, the pricing of back and lay contracts is still symmetric because the risk and reward features of a back contract with probability
are identical to those of a lay contract at probability
. The ratio of the price a back contract to π when
is equal to the ratio of the price of a lay contract to
when
.
Figure 1. Ratios of equilibrium prices to true probabilities and pre- and post-fee loss rates with flat fees of F = 0.01. Agents have uniform beliefs on . (a) Market Price (p)/True Probability. (b) Loss rates without and (c) Loss rates with.
![Figure 1. Ratios of equilibrium prices to true probabilities and pre- and post-fee loss rates with flat fees of F = 0.01. Agents have uniform beliefs on [π−π(1−π),π+π(1−π)]. (a) Market Price (p)/True Probability. (b) Loss rates without and (c) Loss rates with.](/cms/asset/91301361-30ec-4a8a-ac1b-8f86ad968ac8/rquf_a_2257756_f0001_oc.jpg)
The average outcome for participants depends on both the price they paid and the fees. The average profit for a back contract without considering fees will be , so before fees are taken into account, low probability back contracts will make profits, as illustrated by the negative loss rates in the middle panel. However, once fees are factored in, this effect is offset and low probability contracts actually experience much larger loss rates relative to the cost of the investment (either p + F or 1−p + F). The bottom panel shows these loss rates tending towards about 25 percent in this calibration.
The intuition for this result is as follows. Suppose . In this case average losses on contracts would be approximately the same as in Equations (Equation6
(6)
(6) ) and (Equation7
(7)
(7) ) but with the additional fee deducted. This means both contracts lose approximately F on average. Relative to the fee-inclusive cost of the investments, the loss rates on the two contracts are
(17)
(17)
This approximation shows loss rates tend towards close to zero as the probability of the contract increases towards one and towards 100% as π falls towards zero. In our example, the equilibrium price does fall notably below π for low probability contracts but not enough to offset the basic pattern that fees have a higher impact on cheap contracts than on expensive ones.
This pattern of higher loss rates on longshot contracts is superficially similar to the finding that bets on longshots in sports tend to have larger loss rates than bets on favorites. Various behavioral explanations have been suggested to explain this phenomenon but they do not apply to this example. For example, one explanation is that bettors like the high variance that comes with betting on longshots but our agents are risk averse. Another argument is Ali's (Citation1977) demonstration that a symmetric distribution of beliefs among risk-neutral bettors implied favorite-longshot bias in pari-mutuel betting markets, which pool the funds placed by gamblers and pay them out (minus a fraction for the operator) proportionately to those who picked the winner. Ali explained how odds on favorites need to be better than odds on longshots to be consistent with pari-mutuel betting's property that more money is placed on the favorite than the longshot. However, prediction markets by definition place most of the money at risk on favorites and, without fees, deliver equal returns to both sides of contracts in our model.
A closer analogy is the idea that people may overweight small probabilities when assessing the potential return on bets, as in Kahneman and Tversky's (Citation1979) prospect theory. In our case, there is no asymmetry of the kind where people systematically overweight small probabilities. However, those who do overweight these probabilities have the worst outcomes in terms of the return on investment. Purchasers of both contracts have beliefs that over-estimate the return on their chosen contract: Back contract buyers think its probability of success is at least times higher than it is while those who purchase lay contracts think its probability of success is at least
times higher than it is. When
, the former mis-estimation is larger than the latter and leads to bigger percentage losses.
Worth noting is that Page and Clemen's (Citation2013) evidence that prices were too low for longshot contracts and too high for favorites came from data collected from the prediction market InTrade, which ceased operating in 2013. In its early years, Intrade charged a fixed fee on contracts but switched in 2010 to a monthly service fee unrelated to contract purchases. Page and Clemen's data seems to have been mainly collected from the period when fixed fees were charged on contracts. Our calculations show that adding contract fees to the usual ideal assumptions of log utility and symmetric beliefs centered on the true probability does not generate the empirical pattern they documented.
4. Kalshi's fee formula
The new prediction market Kalshi charges the same fee on both sides of each contract but it varies the fees with the price. Specifically, Kalshi charge a trading fee of where at present
.Footnote6 This pricing schedule has an inverse-U shaped cost per contract peaking at
and falling towards zero as the price of the contract heads towards either zero or one.
Kalshi's pricing approach implies smaller distortions in prices than the flat fee on all contracts. The source of this distortion was the relative increase in the variance of low probability contracts but Kalshi's fees being lower for cheap contracts reduces this element. Solving numerically under the same assumptions about beliefs as before but with F replaced with where
, the upper panel of Figure shows the same pattern of prices being lower than probabilities when
and above them otherwise but the distortion in prices is much smaller, with this calibration showing even the lowest prices only 3 percent below the true probability.
Figure 2. Ratios of equilibrium prices to true probabilities and pre- and post-fee loss rates with Kalshi fees of and
. Agents have uniform beliefs on
. (a) Market Price (p)/True Probability (π). (b) Loss rates without fees and (c) Loss rates with.
![Figure 2. Ratios of equilibrium prices to true probabilities and pre- and post-fee loss rates with Kalshi fees of θp(1−p) and θ=0.07. Agents have uniform beliefs on [π−π(1−π),π+π(1−π)]. (a) Market Price (p)/True Probability (π). (b) Loss rates without fees and (c) Loss rates with.](/cms/asset/b2b1d979-f908-469e-a78f-056ed479d64d/rquf_a_2257756_f0002_oc.jpg)
In terms of the incidence of losses, the more accurate prices under Kalshi's formula mean lower pre-fee profits for longshot contracts (the middle panel) so that loss rates incorporating fees are still greater for contracts with . However, this pricing model does not show post-fee loss rates falling monotonically as π rises as it did with a flat fee rate on all contracts (the bottom panel). Instead, loss rates rise slightly as π moves above zero, from about 3.5% in this example for the lowest probabilities to 3.8% for
before falling again as π rises, though with nothing like as nonlinear a pattern as with the flat 1 cent fee. Compared with Figure , the lower loss rates for extreme longshot contracts come at the expense of higher loss rates for contracts in the middle of the probability distribution. As an alternative to comparing charts across different figures, Figure also shows the key model outputs for the five fee structures considered in the paper together on the same charts.
To justify the comparison between the numbers in Figures and , note that if the events being speculated on were equally likely to have probabilities of success anywhere between zero and one, then total fees across all contracts from Kalshi's approach could be calculated as
(18)
(18)
Hence, a flat fee with a charge of
would raise the same fee income across all contracts as Kalshi's formula. At their current pricing of
, this shows Kalshi's approach would raise approximately the same revenue as a flat 1 cent fee on contracts but does so without causing the same pattern of significant price distortion or extremely high loss rates for longshot contracts that Figure shows this 1 cent fee would induce.
5. A flat commission rate on winnings
We now consider how prediction markets work when they only charge a fee on winnings, as operated by commercial prediction markets outside the US, such as UK-based firms Smarkets and Betfair. There are some behavioral arguments that suggest a charge on winnings as being a more likely model to emerge in future commercial prediction markets. A charge on all contracts, whether they are going to win or lose, is highly salient to participants. Losing money on a contract is bad enough, having to pay a trading fee as well may be further discouraging, particularly if agents are motivated by loss aversion. Conversely, a charge on your winnings may not feel so bad: You still came out ahead and the market operator can hardly be expected to do their job for free. The fact that Betfair—owned by the large international betting firm Flutter which also owns Paddy Power and FanDuel—implement this method indicates that experienced and sophisticated participants in this market believe it is the best approach.
In this version of the model, the market operator charges a commission rate on winnings. People with beliefs
perceive expected post-commission winnings from purchasing the back contract to be
and expected losses to be
. They will be willing to purchase this contract if
(19)
(19)
Similarly, participants will perceive expected post-commission winnings from purchasing the lay contract to be
and expected losses as
. They will be willing to purchase this contract if
(20)
(20)
In deciding the quantity of back contracts
that participant i with belief
and wealth
wants to buy, she solves the problem
(21)
(21)
Again, demand for contracts is determined by the ratio of the perceived expected profit to the perceived variance
(22)
(22)
while the demand for lay contracts for participant i with belief
is
(23)
(23)
The equilibrium price satisfies
(24)
(24)
Again, to illustrate the model fully, we need a numerical solution. However, we can describe the general properties of the equilibrium price that will hold for all symmetric belief distributions. The equilibrium price is characterized by
(25)
(25)
The left side depends negatively on p and the right side depends positively on it, so there is a unique equilibrium price. And again the pricing of back and lay contracts will be symmetric.
Two other features from the previous results also hold here. First, we can again show that when , the equilibrium price is p = 0.5. In this case, the belief thresholds for purchasing contracts are
and
. These thresholds sum to one, which means that for this value of π (and only this value) the thresholds are equidistant from the true probability. Also, for
, the price p = 0.5 makes the volumes
and
at equal distances above and below the thresholds equal to each other. These two demands being equal means
(26)
(26)
Inserting p = 0.5,
and
we can see the identity holds. The symmetry of belief thresholds and demands together mean a price of
equates total demand for the two types of contracts.
Finally, we again can show that for , the equilibrium price will be lower than the true probability and vice versa for
. The intuition for this result is that contracts with a lower probability of success win more when they are successful. For this reason, a commission rate on winnings does more to discourage purchases of these contracts and thus their relative price declines.
Technically, this result is obtained via two channels. The introduction of fees increases the threshold for taking the back contract and reduces the threshold for taking the lay contract, increasing the range of beliefs in which people purchase neither contract. However, we show in an appendix that calculating the partial derivatives and
implies the size of these effects is only equal for
and for
, the fee crowds out more back contract buyers than lay contract buyers with the opposite applying when
.
In addition, the appendix also calculates and
and shows that apart from at p = 0.5, once there are fees on winnings, contract demands no longer change in a symmetric manner as beliefs move above and below the belief thresholds. As beliefs move further above or further below the threshold levels, participants will be willing to buy more contracts. However, when p<0.5, the fraction of wealth allocated to back contracts is less sensitive to changes in
than the fraction allocated to the corresponding lay contracts. Because the equilibrium price needs to equate volumes on both contracts, it needs to be lower than the no-fee case when
to encourage more longshot backers and discourage some favorite backers.
Solving the model with the same formulation of beliefs as before and with , Figure shows a somewhat smaller distortion of prices to the previous two cases. The implications for returns on contracts are relatively similar to the case of Kalshi's pricing, though the loss rates for longshot contracts are a bit higher and they fall monotonically as π rises. Since the approximation
works well, we can provide a simple comparison of how Kalshi's pricing compares with commission fees charged on winnings. Total commission fees on winnings are
(27)
(27)
In other words, a commission on winnings at rate
earns approximate the same amount of fees as Kalshi's trading fees with
. So, Kalshi's complex-looking formula with
can be understood as raising the same amount of fees as a 7 percent fee on winnings, which is why we use 7 percent here. Compared with the 2 percent fee on winnings charged by BetFair and Smarkets, Kalshi's current pricing looks expensive.
Figure 3. Ratios of equilibrium prices to true probabilities and pre- and post-fee loss rates with a commission rate on winnings of . Agents have uniform beliefs on
. (a) Market Price (p)/True Probability (π). (b) Loss rates without fees and (c) Loss rates with fees.
![Figure 3. Ratios of equilibrium prices to true probabilities and pre- and post-fee loss rates with a commission rate on winnings of 1−μ=0.07. Agents have uniform beliefs on [π−π(1−π),π+π(1−π)]. (a) Market Price (p)/True Probability (π). (b) Loss rates without fees and (c) Loss rates with fees.](/cms/asset/1c36a16d-dc2e-4c68-80e6-ae22d48e919e/rquf_a_2257756_f0003_oc.jpg)
Unlike Page and Clemen (Citation2013), the agents in this model do not discount their expected payments from winning contracts. It is interesting to note that a fee on winnings has the same impact as discounting because it multiplies downward the value of a winning payment. However, in this model, adding this feature does not produce the outcome in Clemen and Page's model in which relative prices of contracts with a lower probability of success rise when the discount rate increase. In fact, we have the opposite result: As the fee rises, relative prices of longshot contracts fall. This difference in results likely stems from other features in their model, in which agents are risk-neutral and invest all of their wealth in their chosen contract but cannot borrow. That this ‘bounded wealth’ model induces favorite-longshot bias in prediction market prices has been shown by Ottaviani and Sørensen (Citation2015).
6. Two other fee models
Finally, we briefly consider two other potential fee models that could emerge as prediction markets evolve, both of which are more generous in their treatment of purchases of longshot contracts.
6.1. An ad valorem charge
The first alternative model is an ad valorem charge proportional to the price paid for the contract, so that if the contract price is p, then the purchaser has to pay with
being retained by the prediction market operator. Repeating the previous steps, we can show that those who purchase the back contract will have beliefs such that
while those who purchase the lay contract will have beliefs such that
. For those who purchase contracts, the quantities purchased will be
(28)
(28)
(29)
(29)
and equilibrium prices are calculated using the same method as before.
Figure illustrates how the model behaves with ad valorem fee of . This would raise the same amount of fee income as the 1 cent charge on all contracts considered above. One difference between an ad valorem charge and the previous fee regimes is that it makes contracts with a very high probability of winning unattractive and possible untenable. No contracts with pre-fee prices above
could be sold because these would have a fee-inclusive cost above $1 and so could not make a profit. We have thus restricted the illustration of this case in Figure to be limited to show win probabilities between 0.05 and 0.95.
Figure 4. Ratios of equilibrium prices to true probabilities and pre- and post-fee loss rates with an ad valorem charge of 2% on contract purchases. Agents have uniform beliefs on . (a) Market Price (p)/True Probability (π). (b) Loss rates without fees and (c) Loss rates with fees.
![Figure 4. Ratios of equilibrium prices to true probabilities and pre- and post-fee loss rates with an ad valorem charge of 2% on contract purchases. Agents have uniform beliefs on [π−π(1−π),π+π(1−π)]. (a) Market Price (p)/True Probability (π). (b) Loss rates without fees and (c) Loss rates with fees.](/cms/asset/f82c07d3-461b-40fd-8de5-5bae664c848f/rquf_a_2257756_f0004_oc.jpg)
The upper panel shows that the preferential treatment for longshot contracts leads to prices for contracts with now being higher than the underlying true probability and the opposite applying once
. These distortions are larger than for the previous examples despite this approach raising the same amount of fees. Paradoxically, loss rates turn out to be higher for longshot contracts despite the apparently more longshot-friendly pricing. The pricing being unfriendly to favorites means people can only be found to back favorites if the longshot investors provide more money than their contract is actually worth.
Interestingly, although their impact on contract prices are quite different, the bottom chart in Figure , which compares loss rates on back contracts across different fee structures, shows loss rates are the same under ad valorem fees as under flat fees per contract. These calculations show that attempts to provide better value pricing for longshot contracts end up being undone by the endogenous dynamics of the market and thus that this is an innate feature of these markets.
6.2. A Kalshi-style commission on winnings
As a final example, we consider a hybrid commission fee approach, which is to charge Kalshi's fee but only on those who win. In other words, the prediction market operator does not charge a trading fee but when the back contract wins 1−p, its owner pays a fee of and when the lay contract wins p, they also pay
. Total commission fees would be the same as for Kalshi's trading fee model provided the value of θ is doubled.
This pricing structure would also appear attractive to those interested in buying longshot contracts because the fees as a fraction of profits—which would be the appropriate way to quote them in this context—depend positively on p With a fee of this type on winnings, the model's new belief thresholds and demands are derived by replacing µ in the formulas in the Section 5 with the new contract-specific post-commission payout rates on winnings, for back contracts and
for lay contracts.
Figure shows the equilibrium prices in this case, with the same assumptions as before and , which would replicate the total fee amounts from the commission rate on winnings with
as shown in Figure . Because the commission rate depends negatively on p for back contracts and positively on it for lay contracts, the results in the previous section on the impact of commission rates flip sign and the equilibrium price is now higher than the true probability when
and lower than it once
(the top panel). As with the ad valorem fee structure, equilibrium prices now over-state the lowest probabilities but the distortions are smaller in this case. Figure in the appendix shows that post-fee loss rates for contracts are very similar to when the Kalshi formula is applied to all contracts with
, with loss rates being approximately flat until as the probability of success reaches 0.5 before dropping nonlinearly above that. These results illustrate that the finding of rising loss rates as the probability of contract success declines does not depend on specifying a particular fee structure.
7. Conclusions
Prediction markets have the potential to play an important role in assisting governments, businesses and investors make decisions but their usefulness will depend on the accuracy of their prices and the willingness of people with a sufficiently wide range of opinions to participate in them. Our calculations suggest both good and bad news for the future of commercial prediction markets. The good news is that even in the presence of relatively high fees, prediction markets can still generate prices that are pretty close to the true probability of an event once the usual conditions hold for these markets to have this property in the absence of fees. Even better news is that the trading fee pricing formula adopted by Kalshi, the first officially licensed US prediction market, distorts prices much less than a flat fee such as 1 cent per contract.
Less good is the fact that the introduction of fees means those who have the most accurate view of the underlying probability will chose not to participate. The assumption of symmetry of beliefs around the true probability means the market in our model still delivers an accurate price because the two sets of more extreme opinions offset each other. In reality, however, cutting out the ‘sensible middleground’ of opinion and hoping that those with more extreme opinions on either side will average out to the correct opinion may or may not work well depending on the event being predicted.
The other bad aspect is the systematic pattern of loss rates. Unlike stock markets where the average investor can be a winner, prediction markets are innately zero sum in nature and, once an operator has fees, the average outcome for participants is a negative sum. We show that this results in larger loss rates for money invested in contracts backing events that are unlikely to happen and that this finding still holds even if a commission rate formula is adjusted to be more favorable to these contracts. If people participating in prediction markets begin to realize they are experiencing significant losses on the cheaper contracts, then these markets may fail to attract people with the wide range of opinions that they need for their prices to summarize the public's beliefs.
Ultimately, these markets will work best as a way of summarizing the public's information when there is competitive pressure to lower fees. Kalshi's fees, which are equivalent to a 7% charge on winnings, are high relative to UK firms operating similar business models. Admittedly, Kalshi are particularly ambitious in the wide range of markets they are running but it seems likely that competitive pressures coming from future CFTC approvals will bring down fee rates and contribute to the markets working more efficiently.
Disclosure statement
No potential conflict of interest was reported by the author(s).
Notes
1 The CFTC decision on Kalshi can be found at https://www.cftc.gov/PressRoom/PressReleases/8302-20
2 There are, of course, some papers that examine the related topic of how the revenue raising methods of bookmakers impact odds set in betting markets. See, for example, Hurley and McDonough (Citation1995) and Ottaviani and Sørensen (Citation2010) for pari-mutuel betting markets and Whelan (Citation2022) for fixed-odds betting markets.
3 See for example, studies such as Berg et al. (Citation2008) and Forsythe et al. (Citation1992).
4 For surveys of the literature on favorite-longshot bias, see Ottaviani and Sørensen (Citation2008) and Snowberg and Wolfers (Citation2008).
5 An alternative would be specify truncated belief distributions that have masses at zero or one but this approach implies highly skewed distributions when π is near zero one, producing counterfactually large pricing distortions.
6 Technically, Kalshi charge a fee that is a round up to the next cent of where x is the number of contracts purchased. See https://kalshi.com/docs/kalshi-fee-schedule.pdf for details. This pricing structure means fees for very small purchases will tend to higher than the formula but even for investments as small as $50, fees will correspond either exactly or almost exactly to the formula.
References
- Ali, M., Probability and utility estimates for racetrack bettors. J. Polit. Econ., 1977, 85, 803–815.
- Arrow, K.J., Forsythe, R., Gorham, M., Hahn, R., HansonFollow, R., Ledyard, J.O., Levmore, S., Litan, R., Milgrom, P., Nelson, F.D., Neumann, G.R., Ottaviani, M., Schelling, T.C., Shiller, R.J., Smith, V.L., Snowberg, E., Sunstein, C.R., Tetlock, P.C., Tetlock, P.E., Varian, H.R., Wolfers, J. and Zitzewitz, E., The promise of prediction markets. Science, 2008, 320(5878), 877–878.
- Berg, J., Nelson, F. and Rietz, T., Prediction market accuracy in the long run. Int. J. Forecast., 2008, 24, 285–300.
- Forsythe, R., Nelson, F., Neumann, G. and Wright, J., Anatomy of an experimental political stock market. Am. Econ. Rev., 1992, 82, 1143–1161.
- Gjerstad, S., Risk aversion, beliefs and prediction market equilibrium. Working Paper, University of Arizona, 04–17, 2004.
- He, X.-Z. and Treich, N., Prediction market prices under risk aversion and heterogeneous beliefs. J. Math. Econ., 2017, 70, 105–114.
- Hurley, W. and McDonough, L., A note on the Hayek hypothesis and the favorite-longshot bias in parimutuel betting. Am. Econ. Rev., 1995, 85, 949–955.
- Kahneman, D. and Tversky, A., Prospect theory: An analysis of decision under risk. Econometrica, 1979, 47, 263–292.
- Manski, C., Interpreting the predictions of prediction markets. Econ. Lett., 2006, 91, 425–429.
- Ottaviani, M. and Sørensen, P.N., The favorite-longshot bias: An overview of the main explanations. In Handbook of Sports and Lottery Markets, edited by D. B. Hausch and W. T. Ziemba, 2008 (Elsevier: Amsterdam).
- Ottaviani, M. and Sørensen, P.N., Noise, information and the favorite-longshot bias in parimutuel predictions. Am. Econ. J. Microecon., 2010, 2, 58–85.
- Ottaviani, M. and Sørensen, P.N., Price reaction to information with heterogeneous beliefs and wealth effects: Underreaction, momentum, and reversal. Am. Econ. Rev., 2015, 105, 1–34.
- Page, L. and Clemen, R., Do prediction markets produce well-Calibrated probability forecasts? Econ. J., 2013, 123, 491–513.
- Snowberg, E. and Wolfers, J., Examining explanations of a market anomaly: Preferences or perceptions. In Handbook of Sports and Lottery Markets, edited by D. B. Hausch and W. T. Ziemba, 2008 (Elsevier: Amsterdam).
- Sunstein, C., Infotopia: How Many Minds Produce Knowledge, 2006 (Oxford University Press: New York).
- Surowiecki, J., The Wisdom of Crowds, 2004 (Random House: New York).
- Wolfers, J. and Zitzewitz, E., Interpreting prediction market prices as probabilities. Working Paper, NBER 12200, 2006.
- Whelan, K., Risk aversion and favorite-longshot bias in a competitive fixed-odds betting market. CEPR Discussion Paper No. 17518, 2022. Forthcoming in Economica.
Appendices
Appendix 1.
Comparisons across models
Figure compares the key model outputs across the five different fee structures examined in the paper. The upper panel shows the ratio of the equilibrium price of contracts to the true probability and the lower panel shows loss rates for back contracts after fees are considered.
Figure A1. Comparisons across different fee structures. Agents have uniform beliefs on . (a) Market Price (p)/True Probability (π) and (b) Loss rates for back contracts after fees
![Figure A1. Comparisons across different fee structures. Agents have uniform beliefs on [π−π(1−π),π+π(1−π)]. (a) Market Price (p)/True Probability (π) and (b) Loss rates for back contracts after fees](/cms/asset/f6422675-e4d4-45d1-b6dd-48dd92618d10/rquf_a_2257756_f0006_oc.jpg)
Appendix 2.
Omitted derivations
Here we provide derivations for two results mentioned in Section 5.
A.1. Derivation that 
if p<0.5
The thresholds are
(A1)
(A1)
(A2)
(A2)
The partial derivatives are
(A3)
(A3)
(A4)
(A4)
So a higher µ (a reduced fee rate) reduces the threshold for taking the back contract and increases it for taking the lay contract, bringing in more purchases on both sides. The condition in question holds if
(A5)
(A5)
which holds if
(A6)
(A6)
which re-arranges to
(A7)
(A7)
which holds if p<0.5.
A.2. Derivation that 
if p<0.5
Starting from
(A8)
(A8)
(A9)
(A9)
These partial derivatives are
(A10)
(A10)
(A11)
(A11)
The condition for
becomes
(A12)
(A12)
which gives the same inequality as above so again the condition holds if p<0.5.