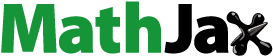
ABSTRACT
Introduction
The increasing availability of data and computing power has made machine learning (ML) a viable approach to faster, more efficient healthcare delivery.
Methods
A systematic literature review (SLR) of published SLRs evaluating ML applications in healthcare settings published between1 January 2010 and 27 March 2023 was conducted.
Results
In total 220 SLRs covering 10,462 ML algorithms were reviewed. The main application of AI in medicine related to the clinical prediction and disease prognosis in oncology and neurology with the use of imaging data. Accuracy, specificity, and sensitivity were provided in 56%, 28%, and 25% SLRs respectively. Internal and external validation was reported in 53% and less than 1% of the cases respectively. The most common modeling approach was neural networks (2,454 ML algorithms), followed by support vector machine and random forest/decision trees (1,578 and 1,522 ML algorithms, respectively).
Expert opinion
The review indicated considerable reporting gaps in terms of the ML’s performance, both internal and external validation. Greater accessibility to healthcare data for developers can ensure the faster adoption of ML algorithms into clinical practice.
1. Introduction
Along with many other sectors, medicine has become a prominent beneficiary of artificial intelligence (AI)-driven innovations, owing to the growing availability of data. The transformation of healthcare began with the widespread adoption of electronic health records (EHRs) in the early 1990s, with up to 93% of primary care doctors using EHR across 24 OECD countries in 2021 [Citation1].
The growing number of new data sources such as sensors, wearables, and mobile applications is transforming healthcare. The digital footprint of a patient’s journey produces new insights that inform decision-making processes and makes them readily available for developing machine learning (ML) algorithms.
Therefore, the abundance of data can help healthcare organizations develop an holistic picture of a patient’s health over time and can also introduce new insights into unmet medical needs with new data-driven technologies.
The potential for digital transformation to improve health outcomes and introduce efficiency gains has already been observed in recent developments. There are numerous examples such as the application of AI to the diagnosis of cardiac diseases [Citation2], neoplastic diseases [Citation3], pathologies of the voice [Citation4] and more recently during the COVID-19 pandemic [Citation5,Citation6] have the potential to enhance diagnostic precision and throughput, and patient outcomes [Citation2].
Several experts claim that medicine is already moving from the past decade, that focused on ML development, to the subsequent decade, driven by the challenges of ensuring ML algorithm deployment in clinical settings [Citation7].
Although the International Medical Device Regulators Forum (IMDRF) introduced the terms ‘software as a medical device’ (SaMD) and ‘software in a medical device’ in 2013, there have been limited efforts so far to develop the value assessment framework for ML algorithms in healthcare system similarly to the pricing & reimbursement of medical devices and pharmaceuticals.
1.1. Aims
In order for the adoption process of artificial intelligence in the healthcare to become effective and implementable there is, however, the need to learn more about the opportunities and challenges with the applicability of AI in medicine. Therefore, our ultimate goal was to summarize the state-of-the-art regarding the availability and performance of AI solutions in healthcare. We conducted a review of systematic literature reviews (SLRs) covering ML algorithms developed for medical purposes. The objective of our research was two-folds: First, to describe the number of ML solutions already available in healthcare; and second, to assess the types of data commonly reported in scientific publications on ML algorithms. Based on our review results, we recommend actions for developers and healthcare payers to facilitate AI integration into medicine.
2. Methodology
This review was carried out according to the guidelines of Preferred Reporting Items for Systematic Review and Meta-Analyses (PRISMA) [Citation8].
2.1. Literature search
This review had the following formal protocol.
2.2. Selection of studies
The systematic literature reviews (SLRs) reporting the use of ML in healthcare in any country, written in English and published in peer-reviewed journals between 1 January 2010 and 27 March 2023 were included.
Searches were conducted in PubMed, IEEE Xplore, Scopus (www.scopus.com), Web of Science, EBSCO, and the Cochrane Library (www.cochranelibrary.com). The following words were searched in the titles and abstracts of published studies: ‘SRL,’ ‘ML’ and ‘Machine Learning,’ using the Boolean operator ‘AND’ and wildcard symbols as appropriate for each database; with additional key terms such as outcome prediction, diagnosis, screening and/or treatment of any disease.
Studies on animal, plant or in vitro investigations were excluded, as were studies assessing ML applications in non-medicine related. Furthermore, explorative articles without details about the performance of MLs were excluded as well.
Four researchers (hereafter referred to as reviewers) performed the initial review in the following steps:
Identification: The titles, keywords, and abstracts of all identified publications were screened by two reviewers that independently evaluated whether the paper had the potential to be relevant. When the initial assessment was different, consensus was reached through discussion.
Full-text screening: The full texts of all publications identified in the previous step were obtained and assessed independently by two reviewers for inclusion in the review and for data extraction against the inclusion/exclusion criteria and study objectives. When the initial assessment of the reviewers was different, a final decision was reached by consensus.
2.3. Data extraction
Data were extracted from each SLR in two phases. First, two recent checklists were analyzed to define the set of review criteria [Citation9,Citation10]. Second, a random sample of 30 SLRs was analyzed to assess the most commonly reported information across the included publications and to develop an extraction grid capable of ensuring a standard, rigors and comprehensive data extraction.
All identified publications were entered into the Covidence systematic review software for the remainder of the review.
Data extraction was initiated after the initial process. Each SLR was reviewed for basic descriptive statistics, including quality assessment and reporting methods, along with an assignment to one of the three categories.
Categorization (classification of data into categories or clusters)
Prediction (making predictions regarding outputs providing historical data)
Discovery (analysis of the structure of data)
2.4. Data analysis
ICD-10 codes were used to analyze the therapeutic area covered by the SLRs, and basic statistics such as the sources of data, accuracy, specificity, and sensitivity were extracted separately from each publication reported in each of the included SLR.
These performance parameters are commonly employed to assess the performance of categorization methods; they are defined as follow:
Where: FN = false negative; FP = false positive; TN = true negative; TP = true positive
Accuracy represents the overall correctness of the model prediction; sensitivity consists of the fraction of correctly identified positive cases while specificity is the fraction of correctly identified negative cases.
Methods of validation and handling missing data were also extracted. Details regarding the external validation with respect to the comparison of AI against humans were also extracted and reviewed, not only from systematic literature reviews but also from primary studies. The details of the types of ML techniques were also extracted, and the number of primary studies reporting the use of different ML algorithm typologies was determined for each SLR included.
3. Results
A total of 2,342 SLRs were identified. Based on the title and abstract reviews, 1,233 hits were removed during the identification phase. A total of 686 duplicates were identified (). The screening phase included 423 publications. After full-text analysis, 220 articles [Citation11–231] covering 10,462 ML algorithms were finally included in the review ().
The number of studies covered by each SLR varied from 4 (166) to 921 (83) articles (). Approximately 88% of these articles were published between 2020–2021 and no study before 2017 ().About 67% (147) of the selected SLRs were published in 2021; SLRs published in 2020 and 2022 represented 9% (19) and 15% (33) of the total, respectively.
Table 1. Review of included systematic reviews.
In total, 74% of studies employed PRISMA or other methods to report their SLR. A quality assessment was not conducted in 117 of the 220 included studies (). A review of the ICD codes revealed that neoplasms (Chapter II) were the most frequently studied clinical areas, followed by diseases of the nervous system (Chapter VI) (). As far as the data sources used are concerned, imaging was used most frequently with clinical notes and lab tests following as the second frequently used ().
Table 2. Review of sources of data used across included studies.
Considerable variations were observed across the included publications in terms of ML accuracy, specificity, and sensitivity. ICD-10 Chapters III and XVIII reported the lowest results, while some ML algorithms for ICD-10 Chapters II and VI reported 100% accuracy for all three parameters (Appendix 1). In total, 231 of 10,963 studies (7 of 220 SLR) provided information about the accuracy, specificity, and sensitivity of all included studies. A total of 3,164 studies (51 SLRs) did not report any results across the three dimensions ().
Table 3. Number of studies included in reviewed SLRs that reported accuracy, sensitivity and specificity.
Four thousand nine hundred ninety-two of the 10,963 studies (103 of 220 SLRs) conducted internal validation procedures. The most common approach was the k-fold cross-validation (1,325 studies), followed by leave-one-out cross-validation (205 cases) (Appendix 2).
Regarding external validation, a comparison of ML with a human comparator was mentioned in 90 of the 10,963 studies () [Citation241–311,Citation313–328]. In total, 50 cases provided evidence of comparable performance, 33 (four) publications confirmed the superiority (inferiority) of ML over clinicians and three did not indicate any results. The median number of clinical experts included in the validation was six (range 1–511).
Table 4. Reviews with comparison of ML vs. clinical experts.
The methodological approach to the missing data was discussed in 144 studies, with the most common being imputation ().
Table 5. Reviews with missing data (MD) discussed.
In total, over 10,000 ML algorithms were used for the included SLRs (). The most common modeling approach was neural networks (2,454 studies), followed by SVM and RF/decision trees (1,578 and 1,522 studies, respectively).
Table 6. Types of machine learning in reviews depending on ICD chapters.
4. Discussion
To the best of our knowledge, this is the first attempt at systematically studying the integration of ML algorithms in healthcare. The number of identified SLRs and AI algorithms along with the coverage of disease areas demonstrates the level of interest and effort dedicated to the application of ML in medical settings.
Key findings revealed:
The relative high frequency of reported ML application in oncology and neurology
The reported high level of ML prediction ability in many diseases area
How disease area impacts the type of ML algorithms and data sources used.
4.1. Disease areas of application and ML ability
The frequent quotations of oncology and neurology () as disease area of the identified SLR likely reflects the prevalence of such diseases and the impact on patients’ lives.
The key highlights of this review were the low reporting quality of publications dedicated to the development and adaptation of ML algorithms in clinical practice. There was a significant share of studies without data on accuracy (44%), sensitivity (72%), and specificity (75%), as well as internal (65%) and external (99%) validations. Additionally, only 44 studies (2% of total) reported a methodological approach for handling of missing data.
The most commonly used type of data was radiological imaging adopted for the development of ML solutions toward the clinical prediction and categorization as well as the disease prognosis in the field of oncology and neurology.
4.2. Type of ML algorithms
However, the most frequently published type of ML was the artificial neural network (ANN). Neural networks try to replicate how neurons work with information provided and processed based on activation functions. These methods can achieve high accuracy but tend to be time-consuming. ANNs can detect complex nonlinear relationships and interactions between the dependent and independent variables (universal approximators). Deep learning (DL) methods are primarily used in oncological or respiratory disease studies. The increasing use of DL has been observed during the COVID-19 pandemic. A systematic literature review of 34 studies indicated that ML could enhance the sensitivity and specificity of radiographic images compared with radiologists’ diagnoses.
Our review indicated that apart from neural networks, SVM is the most frequently used after deep neural networks. SVM use hyperplanes to separate data; they can achieve high accuracy but generally slow to train. The highly similar performance of SVM, particularly in terms of classification accuracy, makes them rank among the most popular ML classifiers. In addition to deep networks and SVM, Decision tree (DT) and Random Forest (RF) were the most often used. Decision tree progressively segment data into smaller and smaller groups. It is a quick method to implement but may not reach high accuracy. Random Forests is an ensemble bagging technique whereby numerous decision trees are combined to obtain final modeling of the results. This process combines both bootstrapping and aggregation. The key advantage of this approach is that it can be used for either classification or regression problems; hence, this is likely one of the reasons it is used in cardiology. Although RF can provide higher diagnostic accuracy and reduce variance without increasing bias, the operating time might be too long and incompatible to clinical situations.
Even though boosted methods are known to improve the performance of the corresponding methods, they have not been extensively encountered in this review.
Other common ML algorithms were also identified but not frequently; among these linear regression methods (including logistic regression), kNN that base their prediction on the proximity among known data to the case under consideration (generally quick to implement but suffer from the curse of dimensionality) and Linear discriminant analysis (LDA) that segments data according to a hyper-plane orthogonal to the vector between the mean input values of the different classes. LDA classifications are easy to implement but may not achieve high accuracy.
4.3. Strength and weakness
This study has several limitations. Firstly, our review was limited to literature reviews; consequently, certain information might have been misunderstood if it had not been presented in the given SLR. There may have been some over-counting of the number of ML algorithms identified. We did not have sufficient details to understand whether any of the publications used the same data source. Secondly, we did not review studies that were missed in any SLR; hence, we could have a biased picture of the utilization of ML in healthcare. Third, we restricted the review to studies published in English, thus potentially introducing a selection bias toward certain countries. Finally, publication bias can not be excluded, as reports of unsatisfactory or unsuccessful ML applications are rarely encountered; thus, the actual performance of ML could be overestimated. Moreover, several techniques may have been reported differently and may be missed or incorrectly categorized. For example, principal component analysis (PCA) was also reported in the included SLRs, despite not being strictly a ML algorithm but a dimensionality reduction technique.
Finally, our ML performance evaluation covered accuracy, sensitivity and specificity. Such performance parameters are relevant in classification problems. It has to be added however, the goodness of fitting of predictive models for quantitative variables such as length of stay (LoS) can be evaluated through different parameters such as residual mean square error (RMSE) or the coefficient of determination (R2).
Despite these limitations, this review provides important insights into the current state of AI integration in the healthcare sector. It indicates that over 10,000 ML algorithms have been already developed for medical use. This is not surprising, considering that AI is becoming a major driver of innovation in healthcare. For example, the number of patents granted solely for digital communication or medical technologies will almost double that for drugs by 2022 in Europe [Citation329]. A rough comparison indicates that fewer than 60 drugs and over 100 ML/AI-enabled medical devices have been approved annually by the FDA since 2019 (up to 523 until the end of January 2023) [Citation330]. Additionally, our study indicated that majority of identified ML algorithms were developed based on the imaging data with the adoption of ANN methods. This implies that artificial intelligence is used for medical purposes mainly to support clinical decision making. It aligns with another study that found 189 out of 222 FDA-approved medical devices (85%) are designed for use by healthcare professionals, while the remaining 33 (15%) are intended for use by patients [Citation331].
5. Conclusions
There is still unrealized potential for AI in healthcare. Despite the growing number of published ML algorithms, there is limited evidence of their impact on clinical practice.
More evidence concerning external and internal validation can drive the change toward a greater, more robust, and safer adoption of AI. Consequently, it may allow payers, clinicians, and patients to increase their trust in ML algorithms. The key is ensuring that AI development is examined through the lens of the health problems in question. Unmet medical needs are heterogeneously shaped by patients and influenced by the care setting, baseline characteristics, and cultural differences. Thus, there is a need to prepare a landing field for ML algorithms for healthcare applications. However, we are not there yet; hence, by moving forward, AI will only face more challenges. Currently, we are in a different era. Let us be ready with the right data at the appropriate time.
6. Expert opinion
Looking into the future, it is provoking to ask how to ensure greater adoption of ML algorithms in the healthcare systems while taking into consideration patients’ benefits, developers’ business needs as well as limitations of public budgets.
Our review was driven by a central question: How can we bridge the divide between the development and implementation of ML algorithms in healthcare? From our findings, we can extract recommendations for both developers and payers.
6.1. Recommendations for ML developers in healthcare
With respect to the performance of artificial intelligence, the results of our review appear promising at first glance. At first glance, it may be perceived as an impressive finding if one acknowledges that within 12 therapeutic areas (out of 22 ICD chapters) we are already having access to some ML algorithms with an accuracy pointing toward 100%. In addition, five other ICD chapters had scores above 88% (Appendix 1). However, to embrace the clinical applicability of AI, a review of such bare numbers may not offer a comprehensive perspective. The adoption of ML algorithms into the clinical settings requires further consideration. As far as internal validation is concerned, the lack of testing of the predictive power on separate datasets may overestimate ML performance in practical situations. It is the cross-validation that leads to more accurate estimates of the performance of the ML model on an unseen dataset. Unfortunately, it was found across only 15% of the included 10,462 studies (Appendix 1). Cross-validation divides the sample into k subsets, with kth subsets used as the test set/validation set and (k-1)th subsets for training. The model is trained on the training data and predictions are made using the model on the testing data. The sensitivity and/or specificity are averaged by testing multiple times on k-folds subsets. As most of the data was used for fitting, the k-fold approach significantly reduced the bias and variance, as most of the data is also used in the validation set. Thus it reduces the risk of undertraining when a large amount of noise is introduced into the training data and, consequently, bias. It helps prevent overfitting, which occurs when the model attempts to learn each detail and noise of the data, leading to poor model performance on test sets [Citation332].
The cross-validation performed better with larger datasets. This is vital, particularly when one considers the importance of ML for diagnosis which initiates a sequence of subsequent actions. Hence, it is up to the correct prediction that can allow the healthcare system to be effective and efficient. This helps optimizing treatment pathways for previously diagnosed patients. The availability of data enables healthcare professionals to use predictive modeling techniques for prevention and prophylaxis actions more than ever.
Generally, the larger the dataset, the greater the statistical power and chances of better prediction. A negative relationship between sample size and classification accuracy has already been reported [Citation332,Citation333], and it is important to note that as many as 83 out of identified 220 systematic literature reviews did not provide information regarding the size of the datasets used for ML algorithm development. Simultaneously, the majority of the included SLRs reported a large variance between the smallest and largest sample sizes, despite having similar clinical objectives (). However, it is not only the size of the training dataset that has significant importance, but also the variability of the available data. Lack of diversity in training datasets, often driven by the use of data obtained from a localized patient’s population, is a main source of inadequate generalizability of the model outputs to different patient populations. As such, it is likely that the most effective approach in reducing biases and expand the applicability of models in multiple settings and/or populations is to train models on multi-institutional datasets. Some studies have indicated that other sources of potential variety driven by medical device manufacturer software are adopted, in which AI models trained on cardiac magnetic resonance imaging (MRI) scans provide different accuracy results from different scanners [Citation334] and more than a two-folds difference were found in the error rate between two different optical coherence tomography (OCT) scans [Citation335]. The limited diversity in the data used for ML is a problem, and a scoping review of publications related to AI that appeared in PubMed in 2019 revealed that over half of the datasets used for clinical AI originated from either the US or China [Citation336]. In addition, the U.S. and China contributed over 40% of the publications [Citation336]. Barriers to accessing data lead to the overutilization of available datasets. For instance, there are only four major databases in ophthalmology: ESSIDOR, DRIVE, EyePACS, and E-ophtha, with unknown publicly available datasets for ophthalmological images in 172 countries that constitute roughly 45% of the global population [Citation337].
Shifting away from the rigorous demands of cross-validation, developers ought to perform more often the studies to test their technology in the mode of external validation as well. To date, the published comparative data between ML algorithms and humans has shown favorable outcomes for the former. For instance, across 12 studies using deep neural networks for ECG analysis to detect structural cardiac pathologies, the predictive accuracy of the neural network DL models was superior to that of expert interpretations by board-certified cardiologists. The same was found in the comparison of computer-aided detection (CAD) systems with 53 general endoscopists for detecting early neoplasia in patients with Barrett’s esophagus (BE). The CAD achieved higher accuracy than any of the clinicians, regardless of the level of endoscopic expertise [Citation338]; in both cases, details regarding the choice of the clinical group were missing.
It should be noted however that less than 1% of the included studies reported external validation. This is a significant gap in the evidence. This has considerable consequences for its implementation in clinical practice. For true external validation, a tuned algorithm must be applied to a new set of data from different sources. The ultimate objective is to ensure the generalizability of the results with the adoption of ML across various care compositions. As Bang and colleagues mentioned in their systematic literature review: ‘CAD algorithms demonstrated high accuracy for the automatic endoscopic diagnosis of oesophageal cancer and neoplasms. The limitation of a lack of performance in external validation and clinical applications should be overcome’ [Citation25].
Considering the broader perspective on the necessity of ML algorithm external validation, it’s crucial to emphasize that embracing a suitable methodology for translation of efficacy (clinical trials data) into effectiveness (real world data) has been introduced as the minimum requirement within the evidence-based healthcare, a concept initially introduced for pharmaceuticals.
Our findings are similar to those of another review of DL studies that focused on the comparison of ML against human comparators covering the period from January 2010 to June 2022. Only ten RCTs (including eight ongoing RCTs) and 81 non-randomized clinical trials compared diagnostic algorithms performance against clinicians [Citation339]. In another systematic literature review of 82 publications, only 14 studies compared the diagnostic performance of DL models based on medical imaging with that of healthcare professionals [Citation340].
6.2. Recommendations for regulators and payers
Will improvements in both internal and external validations make ML algorithms directly eligible for registration and refundable? While the former is likely more about internal validity, as its primary objective addresses the risk – benefit ratio, the latter may be more about external validity, as its primary objective is to address the value for money. Therefore, the next question is how regulators and public payers should balance the requirements with respect to the evidence of the usability of ML algorithms against the need to ensure safety and treatment effectiveness. Given the existence of strict regulations for both pricing and reimbursement for pharmaceuticals and medical devices, it is necessary to enquire whether similar hurdles of evidence generation should also be introduced for ML algorithms. To address this issue, it is important to recall that only approximately 12% of drugs entering clinical trials are ultimately approved by the FDA [Citation341] and the average time to reimbursement for innovative treatments in Europe is 511 days [Citation342]. Hence, some claim that overregulation may harm innovation. However, the development of the majority of AI-driven innovations may be relatively short compared to other time-consuming research and development technologies, and there is potential for greater disruption in the healthcare sector by ML algorithms than what we have witnessed thus far. Therefore, the types of regulations that should be developed to support the adoption of ML algorithms remain unclear. Overall, there is a need to establish a matrix of criteria to assess the ability of AI solutions to be integrated into healthcare systems. There are already several recommendations in this respect, such as a scoping review of 72 guidelines that, among others, identified quality criteria regarding the development, evaluation, and implementation of ML in healthcare [Citation343]. Other experts have suggested grouping ML algorithms into one of the following categories: assistive, augmentative, or autonomous [Citation344].
Still, there is a need for decision-makers (regulators and public payers) to form a common unified approach toward the development of a common set of standards for the assessment of AI-driven health technologies, as ML is rarely jurisdiction-specific. The maximum accuracy varied from 27% (ICD-10 Chapter XVIII) to 100% (ICD-10 Chapter II) across the included studies. Therefore, the question is whether the same rules should be applied, irrespective of the area under consideration. This may require the involvement of clinical experts and a clear understanding of the unmet medical needs in each disease field. Therefore, our recommendations focus on the interoperability in the journey toward unified P&R regulations for ML algorithms. The underlying rationale is to ensure the accessibility of data such as electronic medical records (EMRs) to AI developers. Thus far, there have been limited efforts related to the availability of real-world data (RWD) for validation as eluded earlier. In the era of digital transformation, we should move further and ensure the integration of EMRs with unstructured data. Additionally, healthcare decision-makers must prepare data repositories to facilitate external validation and invest in local data analytics capabilities to facilitate internal validation. Such efforts should be welcomed by developers, as expressed by many experts [Citation345]. The overarching objective is to ensure that ML algorithms have complete access to health-related data irrespective of geographical, demographic, or institutional composition. Without an appropriate understanding of the health problems in question, ML algorithms can only be utilized for the populations and medical conditions for which they were trained, failing to provide any value for populations or concomitant medical conditions that were omitted or underrepresented in the training set owing to racial, ethnic, or simple misrepresentation. Such activities will inevitably bring an additional burden on both payers and developers; however, AI is as good as the data it possesses, as demonstrated in this study.
6.3. Five years view
Machine learning is poised to revolutionize the healthcare system to an extent not seen before. It will reshape decision-making processes, with individuals playing a more significant role, thanks to data delivered directly from the Internet of Things. The role of clinicians will shift from decision-makers to consultants, supporting patients in interpreting the collected data. With the rapid advancements in sensor technologies and the widespread availability of semiconductors, machine learning algorithms tailored to mobile phones will empower patients and, most importantly, provide numerous opportunities for preventive care. Significant savings for public payers can be realized as data-driven trends lead to human-centric healthcare ecosystems, provided they find a solid framework in the legal structure. The digital revolution is set to retire the healthcare system as we have known it so far.
Article highlights
Artificial Intelligence and Machine Learning (ML) have to the potential to improve health outcomes and increase healthcare system’s efficiency.
A systematic literature review (SLR) identified 220 published SLRs evaluating ML applications in healthcare settings covering 10,462 ML.
The most common modeling approach was neural networks (2,454 ML algorithms), followed by support vector machine and random forest/decision trees (1,578 and 1,522 ML algorithms, respectively).
Internal validation was reported in 53% of the ML algorithms and external validation in less than 1% of cases. The lack of assessment of the AI performance should be overcome to facilitate the application of AI/ML in healthcare.
Declaration of interests
This paper was not funded. The authors have no relevant affiliations or financial involvement with any organization or entity with a financial interest in or financial conflict with the subject matter or materials discussed in the manuscript. This includes employment, consultancies, honoraria, stock ownership or options, expert testimony, grants or patents received or pending, or royalties.
Reviewer disclosures
Peer reviewers on this manuscript have no relevant financial or other relationships to disclose.
Authors contribution
KK and SP participated in the design and execution of the SRL and oversaw studies selection and the synthesis of the results obtained; they also finalized the discussion and the conclusions. JEP participated in the design of the SRL and actively contributed to the selection of studies and data extraction. BA, MHV, KJK contributed data extraction as well as to the drafting of the manuscript.
All authors read and approved the final version of the manuscript for publication.
Supplemental Material
Download MS Word (253.8 KB)Supplementary material
Supplemental data for this article can be accessed online at https://doi.org/10.1080/14737167.2023.2279107
Additional information
Funding
References
- OECD. Health at a glance 2021: OECD Indicators: Digital health. 2021.
- Enad H, Mohammed M. A Review on Artificial Intelligence and Quantum Machine Learning for Heart Disease Diagnosis: Current Techniques, Challenges and Issues. Recent Dev Fut Directions. 2023;11(1):08–25. doi: 10.54216/FPA.110101
- Mohammed MA, Lakhan A, Abdulkareem KH, et al. Federated auto-encoder and XGBoost schemes for multi-omics cancer detection in distributed fog computing paradigm. Chemometr Intell Lab Syst. 2023;241:104932. doi: 10.1016/j.chemolab.2023.104932
- Abdulmajeed NQ, Al-Khateeb B, Mohammed MA. Voice pathology identification system using a deep learning approach based on unique feature selection sets. Expert Syst. 2023;3:e13327.
- Arif ZH, Cengiz K. Severity classification for COVID-19 infections based on lasso-logistic regression model. Int J Math Stat Comput Sci. 2023;1:25–32. doi: 10.59543/ijmscs.v1i.7715
- Salman AO, Geman O. Evaluating three Machine learning classification methods for effective COVID-19 diagnosis. Int J Math Stat Comput Sci. 2023;1:1–14. doi: 10.59543/ijmscs.v1i.7693
- Zeng Z, Zhan J, Zhang K, et al. Global, regional, and national burden of urinary tract infections from 1990 to 2019: an analysis of the global burden of disease study 2019. World J Urol. 2022;40(3):755–763. doi: 10.1007/s00345-021-03913-0
- Moher D, Liberati A, Tetzlaff J, et al. Preferred reporting items for systematic reviews and meta-analyses: the PRISMA statement. BMJ. 2009;339:b2535. jul21 1. doi: 10.1136/bmj.b2535.
- Loftus TJ, Tighe PJ, Ozrazgat-Baslanti T, et al. Ideal algorithms in healthcare: explainable, dynamic, precise, autonomous, fair, and reproducible. PLOS Digit Health. 2022;1(1):e0000006. doi: 10.1371/journal.pdig.0000006
- Padula WV, Kreif N, Vanness DJ, et al. Machine learning methods in Health Economics and outcomes research-the PALISADE checklist: a good practices report of an ISPOR task force. Value Health. 2022;25(7):1063–1080. doi: 10.1016/j.jval.2022.03.022
- Abu Bakar AR, Lai KW, Hamzaid NA. The emergence of machine learning in auditory neural impairment: a systematic review. Neurosci Lett. 2021;765:136250. doi: 10.1016/j.neulet.2021.136250
- Adamidi ES, Mitsis K, Nikita KS. Artificial intelligence in clinical care amidst COVID-19 pandemic: A systematic review. Comput Struct Biotechnol J. 2021;19:2833–2850. doi: 10.1016/j.csbj.2021.05.010
- Adeoye J, Tan JY, Choi SW, et al. Prediction models applying machine learning to oral cavity cancer outcomes: a systematic review. Int J Med Inform. 2021;154:154. doi: 10.1016/j.ijmedinf.2021.104557
- Ahsan MM, Siddique Z. Machine learning-based heart disease diagnosis: a systematic literature review. Artif Intell Med. 2022;128:102289. doi: 10.1016/j.artmed.2022.102289
- Akazawa M, Hashimoto K. Artificial intelligence in gynecologic cancers: current status and future challenges – a systematic review. Artif Intell Med. 2021;120:102164. doi: 10.1016/j.artmed.2021.102164
- Al Hinai G, Jammoul S, Vajihi Z, et al. Deep learning analysis of resting electrocardiograms for the detection of myocardial dysfunction, hypertrophy, and ischaemia: a systematic review. Eur Heart J Digit Health. 2021;2(3):416–423. doi: 10.1093/ehjdh/ztab048
- Alabi RO, Youssef O, Pirinen M, et al. Machine learning in oral squamous cell carcinoma: Current status, clinical concerns and prospects for future—A systematic review. Artif Intell Med. 2021;115:102060. doi: 10.1016/j.artmed.2021.102060
- Albahri AS, Hamid RA, Alwan J, et al. Role of biological data mining and Machine learning techniques in detecting and diagnosing the Novel coronavirus (COVID-19): a systematic review. J Med Syst. 2020;44(7). doi: 10.1007/s10916-020-01582-x
- Alballa N, Al-Turaiki I. Machine learning approaches in COVID-19 diagnosis, mortality, and severity risk prediction: a review. IMU. 2021;24:100564. doi: 10.1016/j.imu.2021.100564
- Alharbi ET, Nadeem F, Cherif A. Predictive models for personalized asthma attacks based on patient’s biosignals and environmental factors: a systematic review. BMC Med Inform Decis Mak. 2021;21(1). doi: 10.1186/s12911-021-01704-6
- Alhasan AS. Clinical applications of Artificial intelligence, Machine learning, and Deep learning in the imaging of Gliomas: a systematic review. Cureus. 2021;13(11). doi: 10.7759/cureus.19580
- Alsolai H, Qureshi S, Iqbal SMZ, et al. A systematic review of literature on Automated sleep Scoring. IEEE Access. 2022;10:79419–79443. doi: 10.1109/ACCESS.2022.3194145
- Anteby R, Klang E, Horesh N, et al. Deep learning for noninvasive liver fibrosis classification: a systematic review. Liver Int. 2021;41(10):2269–2278. doi: 10.1111/liv.14966
- Bang CS, Lee JJ, Baik GH. Computer-aided diagnosis of Gastrointestinal ulcer and hemorrhage using wireless capsule endoscopy: systematic review and diagnostic test accuracy meta-analysis. J Med Internet Res. 2021;23(12):e33267. doi: 10.2196/33267
- Bang CS, Lee JJ, Baik GH. Computer-aided diagnosis of esophageal cancer and neoplasms in endoscopic images: a systematic review and meta-analysis of diagnostic test accuracy. Gastrointest Endosc. 2021;93(5):1006–1015.e1013. doi: 10.1016/j.gie.2020.11.025
- Barrett L, Hu J, Howell P. Systematic review of Machine learning approaches for detecting developmental stuttering. IEEE/ACM Trans Audio Speech Lang Process. 2022;30:1160–1172. doi: 10.1109/TASLP.2022.3155295
- Bazoukis G, Stavrakis S, Zhou J, et al. Machine learning versus conventional clinical methods in guiding management of heart failure patients—a systematic review. Heart Fail Rev. 2021;26(1):23–34. doi: 10.1007/s10741-020-10007-3
- Bedrikovetski S, Dudi-Venkata NN, Kroon HM, et al. Artificial intelligence for pre-operative lymph node staging in colorectal cancer: a systematic review and meta-analysis. BMC Cancer. 2021;21(1):1058. doi: 10.1186/s12885-021-08773-w
- Bedrikovetski S, Dudi-Venkata NN, Maicas G, et al. Artificial intelligence for the diagnosis of lymph node metastases in patients with abdominopelvic malignancy: a systematic review and meta-analysis. Artif Intell Med. 2021;113:102022. doi: 10.1016/j.artmed.2021.102022
- Benoit J, Onyeaka H, Keshavan M, et al. Systematic review of digital phenotyping and machine learning in psychosis spectrum illnesses. Harv Rev Psychiatry. 2020;28(5):296–304. doi: 10.1097/HRP.0000000000000268
- Bernert RA, Hilberg AM, Melia R, et al. Artificial intelligence and suicide prevention: a systematic review of Machine learning investigations. Int J Environ Res Public Health. 2020;17(16):5929. doi: 10.3390/ijerph17165929
- Bertl M, Metsallik J, Ross P. A systematic literature review of AI-based digital decision support systems for post-traumatic stress disorder. Front Psychiatry. 2022;13: doi: 10.3389/fpsyt.2022.923613
- Binvignat M, Pedoia V, Butte AJ, et al. Use of machine learning in osteoarthritis research: a systematic literature review. RMD Open. 2022;8(1):e001998. doi: 10.1136/rmdopen-2021-001998
- Boonstra A, Laven M. Influence of artificial intelligence on the work design of emergency department clinicians a systematic literature review. BMC Health Serv Res. 2022;22(1). doi: 10.1186/s12913-022-08070-7
- Boyd C, Brown G, Kleinig T, et al. Machine learning quantitation of cardiovascular and cerebrovascular disease: a systematic review of clinical applications. Diagnostics. 2021;11(3):551. doi: 10.3390/diagnostics11030551
- Bracher-Smith M, Crawford K, Escott-Price V. Machine learning for genetic prediction of psychiatric disorders: a systematic review. Mol Psychiatry. 2021;26(1):70–79. doi: 10.1038/s41380-020-0825-2
- Buchlak QD, Esmaili N, Leveque JC, et al. Machine learning applications to clinical decision support in neurosurgery: an artificial intelligence augmented systematic review. Neurosurg Rev. 2020;43(5):1235–1253. doi: 10.1007/s10143-019-01163-8
- Buisson M, Navel V, Labbe A, et al. Deep learning versus ophthalmologists for screening for glaucoma on fundus examination: a systematic review and meta-analysis. Clin Exp Ophthalmol. 2021;49(9):1027–1038. doi: 10.1111/ceo.14000
- Cabitza F, Locoro A, Banfi G. Machine learning in orthopedics: a literature review. Front Bioeng Biotechnol. 2018;6:75. doi: 10.3389/fbioe.2018.00075
- Castaldo R, Cavaliere C, Soricelli A, et al. Radiomic and genomic machine learning method performance for prostate cancer diagnosis: systematic literature review. J Med Internet Res. 2021;23(4):e22394. doi: 10.2196/22394
- Cavus N, Lawan AA, Ibrahim Z, et al. A systematic literature review on the application of machine-learning models in behavioral assessment of Autism spectrum disorder. J Pers Med. 2021;11(4):299. doi: 10.3390/jpm11040299
- Celtikci E. A systematic review on machine learning in neurosurgery: the future of decision-making in patient care. Turk Neurosurg. 2018;28(2):167–173. doi: 10.5137/1019-5149.JTN.20059-17.1
- Chandra G, Irisha KD, Vica VI, et al. Systematic literature review on application of artificial intelligence in cancer detection using image processing. In: 2022 3rd International Conference on Artificial Intelligence and Data Sciences (AiDAS); Ipoh, Malaysia; 2022. p. 273–277.
- Chee ML, Ong MEH, Siddiqui FJ, et al. Artificial intelligence applications for COVID-19 in intensive care and emergency settings: a systematic review. Int J Environ Res Public Health. 2021;18(9):4749. doi: 10.3390/ijerph18094749
- Chiesa-Estomba CM, Graña M, Medela A, et al. Machine learning algorithms as a computer-assisted decision tool for Oral cancer prognosis and management decisions: a systematic review. ORL J Otorhinolaryngol Relat Spec. 2022;84(4):1–11. doi: 10.1159/000520672
- Cho SJ, Sunwoo L, Baik SH, et al. Brain metastasis detection using machine learning: a systematic review and meta-analysis. Neuro Oncol. 2021;23(2):214–225. doi: 10.1093/neuonc/noaa232
- Choudhury A, Asan O. Role of Artificial intelligence in patient safety outcomes: systematic literature review. JMIR Med Inform. 2020;8(7):e18599. doi: 10.2196/18599
- Choudhury A, Renjilian E, Asan O. Use of machine learning in geriatric clinical care for chronic diseases: a systematic literature review. JAMIA Open. 2020;3(3):459–471. doi: 10.1093/jamiaopen/ooaa034
- da Silva Neto SR, Tabosa Oliveira T, Teixeira IV, et al. Machine learning and deep learning techniques to support clinical diagnosis of arboviral diseases: a systematic review. PLoS Negl Trop Dis. 2022;16(1):e0010061. doi: 10.1371/journal.pntd.0010061
- Dallora AL, Eivazzadeh S, Mendes E, et al. Machine learning and microsimulation techniques on the prognosis of dementia: a systematic literature review. PLoS One. 2017;12(6):e0179804. doi: 10.1371/journal.pone.0179804
- Dallora AL, Anderberg P, Kvist O, et al. Bone age assessment with various machine learning techniques: a systematic literature review and meta-analysis. PLoS One. 2019;14(7):e0220242. doi: 10.1371/journal.pone.0220242
- Daniel, Cenggoro TW, Pardamean B. A systematic literature review of machine learning application in COVID-19 medical image classification. Procedia Comput Sci. 2023;216:749–756. doi: 10.1016/j.procs.2022.12.192
- D’Antoni F, Russo F, Ambrosio L, et al. Artificial intelligence and Computer vision in low back Pain: a systematic review. Int J Environ Res Public Health. 2021;18(20):10909. doi: 10.3390/ijerph182010909
- Das PK, V A D, Meher S, et al. A systematic review on recent advancements in deep and machine learning based detection and classification of acute lymphoblastic leukemia. IEEE Access. 2022;10:81741–81763. doi: 10.1109/ACCESS.2022.3196037
- Das T, Kaur H, Gour P, et al. Intersection of network medicine and machine learning towards investigating the key biomarkers and pathways underlying amyotrophic lateral sclerosis: a systematic review. Brief Bioinform. 2022;23(6). doi: 10.1093/bib/bbac442
- de Bardeci M, Ip CT, Olbrich S. Deep learning applied to electroencephalogram data in mental disorders: a systematic review. Biol Psychol. 2021;162:108117. doi: 10.1016/j.biopsycho.2021.108117
- Decharatanachart P, Chaiteerakij R, Tiyarattanachai T, et al. Application of artificial intelligence in chronic liver diseases: a systematic review and meta-analysis. BMC Gastroenterol. 2021;21(1):10. doi: 10.1186/s12876-020-01585-5
- Decharatanachart P, Chaiteerakij R, Tiyarattanachai T, et al. Application of artificial intelligence in non-alcoholic fatty liver disease and liver fibrosis: a systematic review and meta-analysis. Therap Adv Gastroenterol. 2021;14:17562848211062807. doi: 10.1177/17562848211062807
- DelSole EM, Keck WL, Patel AA. The state of machine learning in spine surgery: a systematic review. Clin Spine Surg. 2021;35(2):80–89. doi: 10.1097/BSD.0000000000001208
- Dogan O, Tiwari S, Jabbar MA, et al. A systematic review on AI/ML approaches against COVID-19 outbreak. Complex Intell Systems. 2021;7(5):1–24. doi: 10.1007/s40747-021-00424-8
- Dudchenko A, Kopanitsa G. Decision support systems in cardiology: a systematic review. Stud Health Technol Inform. 2017;237:209–214.
- Ebrahimi A, Wiil UK, Schmidt T, et al. Predicting the risk of alcohol use disorder using machine learning: a systematic literature review. IEEE Access. 2021;9:151697–151712. doi: 10.1109/ACCESS.2021.3126777
- Ebrahimighahnavieh MA, Luo S, Chiong R. Deep learning to detect Alzheimer’s disease from neuroimaging: a systematic literature review. Comput Methods Programs Biomed. 2020;187:105242. doi: 10.1016/j.cmpb.2019.105242
- El-Daw S, El-Tantawy A, Aly T, et al. Role of machine learning in management of degenerative spondylolisthesis: a systematic review. Curr Orthop Pract. 2021;32(3):302–308. doi: 10.1097/BCO.0000000000000992
- Falconer N, Abdel-Hafez A, Scott IA, et al. Systematic review of machine learning models for personalised dosing of heparin. Br J Clin Pharmacol. 2021;87(11):4124–4139. doi: 10.1111/bcp.14852
- Farook TH, Jamayet NB, Abdullah JY, et al. Machine learning and intelligent Diagnostics in Dental and orofacial pain management: a systematic review. Pain Res Manag. 2021;2021:1–9. doi: 10.1155/2021/6659133
- Fernandes F, Barbalho I, Barros D, et al. Biomedical signals and machine learning in amyotrophic lateral sclerosis: a systematic review. Biomed Eng Online. 2021;20(1). doi: 10.1186/s12938-021-00896-2
- Fregoso-Aparicio L, Noguez J, Montesinos L, et al. Machine learning and deep learning predictive models for type 2 diabetes: a systematic review. Diabetol Metab Syndr. 2021;13(1):148. doi: 10.1186/s13098-021-00767-9
- Frondelius T, Atkova I, Miettunen J, et al. Diagnostic and prognostic prediction models in ventilator-associated pneumonia: systematic review and meta-analysis of prediction modelling studies. J Crit Care. 2022;67:44–56. doi: 10.1016/j.jcrc.2021.10.001
- Fusco R, Grassi R, Granata V, et al. Artificial intelligence and COVID-19 using chest ct scan and chest x-ray images: machine learning and deep learning approaches for diagnosis and treatment. J Pers Med. 2021;11(10):993. doi: 10.3390/jpm11100993
- Garrow CR, Kowalewski KF, Li L, et al. Machine learning for surgical phase recognition: a systematic review. Ann Surg. 2021;273(4):684–693. doi: 10.1097/SLA.0000000000004425
- Ghaderzadeh M, Asadi F. Deep learning in the detection and diagnosis of COVID-19 using radiology modalities: a systematic review. J Healthc Eng. 2021;2021,:6677314. doi: 10.1155/2021/6677314
- Grueso S, Viejo-Sobera R. Machine learning methods for predicting progression from mild cognitive impairment to Alzheimer’s disease dementia: a systematic review. Alzheimers Res Ther. 2021;13(1):162. doi: 10.1186/s13195-021-00900-w
- Gutiérrez-Tobal GC, Álvarez D, Kheirandish-Gozal L, et al. Reliability of machine learning to diagnose pediatric obstructive sleep apnea: systematic review and meta-analysis. Pediatr Pulmonol. 2021;57(8):1931–1943. doi: 10.1002/ppul.25423
- Haggenmüller S, Maron RC, Hekler A, et al. Skin cancer classification via convolutional neural networks: systematic review of studies involving human experts. Eur J Cancer. 2021;156:202–216. doi: 10.1016/j.ejca.2021.06.049
- Hameed BMZ, Shah M, Naik N, et al. The ascent of artificial intelligence in endourology: a systematic review over the last 2 decades. Curr Urol Rep. 2021;22(10):53. doi: 10.1007/s11934-021-01069-3
- Hasan N, Bao YKK. Understanding current states of machine learning approaches in medical informatics: a systematic literature review. Health Technol. 2021;11(3):471–482. doi: 10.1007/s12553-021-00538-6
- Hassan N, Slight R, Weiand D, et al. Preventing sepsis; how can artificial intelligence inform the clinical decision-making process? a systematic review. Int J Med Inform. 2021;150:104457. doi: 10.1016/j.ijmedinf.2021.104457
- Henn J, Buness A, Schmid M, et al. Machine learning to guide clinical decision-making in abdominal surgery—a systematic literature review. Langenbecks Arch Surg. 2021;407(1):51–61. doi: 10.1007/s00423-021-02348-w
- Hickman SE, Woitek R, Le EPV, et al. Machine learning for workflow applications in screening Mammography: systematic review and meta-analysis. Radiology. 2022;302(1):88–104. doi: 10.1148/radiol.2021210391
- Hinterwimmer F, Lazic I, Suren C, et al. Machine learning in knee arthroplasty: specific data are key—a systematic review. Knee Surg Sports Traumatol Arthrosc. 2022;30(2):376–388. doi: 10.1007/s00167-021-06848-6
- Hoekstra O, Hurst W, Tummers J. Healthcare related event prediction from textual data with machine learning: a systematic literature review. Healthc Anal. 2022;2:100107. doi: 10.1016/j.health.2022.100107
- Hoodbhoy Z, Masroor Jeelani S, Aziz A, et al. Machine learning for child and adolescent health: a systematic review. Pediatrics. 2021;147(1):e2020011833. doi: 10.1542/peds.2020-011833
- Hosni M, Abnane I, Idri A, et al. Reviewing ensemble classification methods in breast cancer. Comput Methods Programs Biomed. 2019;177:89–112. doi: 10.1016/j.cmpb.2019.05.019
- Hoyos W, Aguilar J, Toro M. Dengue models based on machine learning techniques: A systematic literature review. Artif Intell Med. 2021;119:102157. doi: 10.1016/j.artmed.2021.102157
- Huang J, Shlobin NA, DeCuypere M, et al. Deep learning for outcome prediction in Neurosurgery: a systematic review of design, reporting, and reproducibility. Neurosurgery. 2022;90(1):16–38. doi: 10.1227/NEU.0000000000001736
- Huang S, Dang J, Sheckter CC, et al. A systematic review of machine learning and automation in burn wound evaluation: a promising but developing frontier. Burns. 2021;47(8):1691–1704. doi: 10.1016/j.burns.2021.07.007
- Huang Z, Aarab G, Ravesloot MJL, et al. Prediction of the obstruction sites in the upper airway in sleep-disordered breathing based on snoring sound parameters: a systematic review. Sleep Med. 2021;88:116–133. doi: 10.1016/j.sleep.2021.10.015
- Ibrahim B, Suppiah S, Ibrahim N, et al. Diagnostic power of resting-state fMRI for detection of network connectivity in Alzheimer’s disease and mild cognitive impairment: a systematic review. Hum Brain Mapp. 2021;42(9):2941–2968. doi: 10.1002/hbm.25369
- Infante T, Cavaliere C, Punzo B, et al. Radiogenomics and artificial intelligence approaches applied to cardiac computed tomography angiography and cardiac magnetic resonance for precision medicine in coronary heart disease: a systematic review. Circ Cardiovasc Imaging. 2021;14(12):1133–1146. doi: 10.1161/CIRCIMAGING.121.013025
- Irgang L, Barth H, Holmén M. Data-driven technologies as enablers for value Creation in the prevention of surgical site infections: a systematic review. J Healthc Informatics Res. 2023;7(1):1–41. doi: 10.1007/s41666-023-00129-2
- Islam MN, Mustafina SN, Mahmud T, et al. Machine learning to predict pregnancy outcomes: a systematic review, synthesizing framework and future research agenda. Bmc Pregnancy Childbirth. 2022;22(1). doi: 10.1186/s12884-022-04594-2
- Jiang K, Jiang X, Pan J, et al. Current evidence and future perspective of accuracy of Artificial intelligence Application for early gastric cancer diagnosis with endoscopy: a systematic and meta-analysis. Front Med. 2021;8:629080. doi: 10.3389/fmed.2021.629080
- Jiang MY, Ma YX, Guo SY, et al. Using Machine learning technologies in pressure injury management: systematic review. JMIR Med Inform. 2021;9(3):e25704. doi: 10.2196/25704
- Jones OT, Calanzani N, Saji S, et al. Artificial intelligence techniques that may be applied to primary care data to facilitate earlier diagnosis of cancer: systematic review. J Med Internet Res. 2021;23(3):N.PAG–N.PAG. doi: 10.2196/23483
- Kalhori SRN, Tanhapour M, Gholamzadeh M. Enhanced childhood diseases treatment using computational models: systematic review of intelligent experiments heading to precision medicine. J Biomed Informat. 2021;115:115. doi: 10.1016/j.jbi.2021.103687
- Kareemi H, Vaillancourt C, Rosenberg H, et al. Machine learning versus usual care for diagnostic and prognostic prediction in the emergency department: a systematic review. Acad Emerg Med. 2021;28(2):184–196. doi: 10.1111/acem.14190
- Karwath A, Bunting KV, Gill SK, et al. Redefining β-blocker response in heart failure patients with sinus rhythm and atrial fibrillation: a machine learning cluster analysis. Lancet. 2021;398(10309):1427–1435. doi: 10.1016/S0140-6736(21)01638-X
- Kassem MA, Hosny KM, Damaševičius R, et al. Machine learning and Deep learning methods for skin lesion classification and diagnosis: a systematic review. Diagnostics. 2021;11(8):1390. doi: 10.3390/diagnostics11081390
- Kausch SL, Moorman JR, Lake DE, et al. Physiological machine learning models for prediction of sepsis in hospitalized adults: an integrative review. Intensive Crit Care Nurs. 2021;65:103035. doi: 10.1016/j.iccn.2021.103035
- Kawamoto A, Takenaka K, Okamoto R, et al. Systematic review of artificial intelligence-based image diagnosis for inflammatory bowel disease. Dig Endosc. 2022;34(7):1311–1319. doi: 10.1111/den.14334
- Kedra J, Radstake T, Pandit A, et al. Current status of use of big data and artificial intelligence in RMDs: a systematic literature review informing EULAR recommendations. RMD Open. 2019;5(2):e001004. doi: 10.1136/rmdopen-2019-001004
- Kennedy EE, Bowles KH, Aryal S. Systematic review of prediction models for postacute care destination decision-making. J Am Med Informatics Assoc. 2022;29(1):176–186. doi: 10.1093/jamia/ocab197
- Khanagar SB, Naik S, Al Kheraif AA, et al. Application and performance of Artificial intelligence technology in Oral cancer diagnosis and prediction of prognosis: a systematic review. Diagnostics. 2021;11(6):1004. doi: 10.3390/diagnostics11061004
- Kim HR, Sung M, Park JA, et al. Analyzing adverse drug reaction using statistical and machine learning methods: a systematic review. Medicine. 2022;101(25):E29387. doi: 10.1097/MD.0000000000029387
- Kim SS. Recent trends of artificial intelligence and machine learning for insomnia research. Chronobiol Med. 2021;3(1):16–19. doi: 10.33069/cim.2021.0008
- Kodama S, Fujihara K, Shiozaki H, et al. Ability of current machine learning algorithms to predict and detect hypoglycemia in patients with diabetes mellitus: meta-analysis. JMIR Diabetes. 2021;6(1):e22458. doi: 10.2196/22458
- Komolafe TE, Cao Y, Nguchu BA, et al. Diagnostic test accuracy of deep learning detection of COVID-19: a systematic review and meta-analysis. Acad Radiol. 2021;28(11):1507–1523. doi: 10.1016/j.acra.2021.08.008
- Kourou K, Exarchos KP, Papaloukas C, et al. Applied machine learning in cancer research: a systematic review for patient diagnosis, classification and prognosis. Comput Struct Biotechnol J. 2021;19:5546–5555. doi: 10.1016/j.csbj.2021.10.006
- Kozikowski M, Suarez-Ibarrola R, Osiecki R, et al. Role of radiomics in the prediction of muscle-invasive bladder cancer: a systematic review and meta-analysis. Eur Urol Focus. 2021;8(3):728–738. doi: 10.1016/j.euf.2021.05.005
- Kumar S, Oh I, Schindler S, et al. Machine learning for modeling the progression of Alzheimer disease dementia using clinical data: a systematic literature review. JAMIA Open. 2021;4(3):ooab052. doi: 10.1093/jamiaopen/ooab052
- Kumar Y, Gupta S, Singla R, et al. A systematic review of artificial intelligence techniques in cancer prediction and diagnosis. Archiv Comput Methods Eng. 2022;29(4):2043–2070.
- Kuntz S, Krieghoff-Henning E, Kather JN, et al. Gastrointestinal cancer classification and prognostication from histology using deep learning: systematic review. Eur J Cancer. 2021;155:200–215. doi: 10.1016/j.ejca.2021.07.012
- La Greca Saint-Esteven A, Vuong D, Tschanz F, et al. Systematic review on the association of radiomics with tumor biological endpoints. Cancers (Basel). 2021;13(12). doi: 10.3390/cancers13123015
- Langarizadeh M, Sayadi M. Machine learning techniques for diagnosis of lower gastrointestinal cancer: a systematic review. Iran Red Crescent Med J. 2021;23(7):e436.
- Le Glaz A, Haralambous Y, Kim-Dufor DH, et al. Machine learning and natural language processing in mental health: systematic review. J Med Internet Res. 2021;23(5):e15708. doi: 10.2196/15708
- Lecointre L, Dana J, Lodi M, et al. Artificial intelligence-based radiomics models in endometrial cancer: a systematic review. Eur J Surg Oncol. 2021;47(11):2734–2741. doi: 10.1016/j.ejso.2021.06.023
- Lequertier V, Wang T, Fondrevelle J, et al. Hospital length of stay prediction methods: a systematic review. Med Care. 2021;59(10):929–938. doi: 10.1097/MLR.0000000000001596
- Li JX, Zhou ZJ, Dong JY, et al. Predicting breast cancer 5-year survival using machine learning: a systematic review. PLoS One. 2021;16(4):e0250370. doi: 10.1371/journal.pone.0250370
- Li MD, Ahmed SR, Choy E, et al. Artificial intelligence applied to musculoskeletal oncology: a systematic review. Skeletal Radiol. 2022;51(2):245–256. doi: 10.1007/s00256-021-03820-w
- Li Y, Wang X, Zhang J, et al. Applications of artificial intelligence (AI) in researches on non-alcoholic fatty liver disease(NAFLD): a systematic review. Rev Endocr Metab Disord. 2021;23(3):387–400. doi: 10.1007/s11154-021-09681-x
- Librenza-Garcia D, Kotzian BJ, Yang J, et al. The impact of machine learning techniques in the study of bipolar disorder: a systematic review. Neuroscience & Biobehavioral Reviews. 2017;80:538–554. doi: 10.1016/j.neubiorev.2017.07.004
- Lima CLD, da Silva ACG, Moreno GMM, et al. Temporal and spatiotemporal arboviruses forecasting by machine learning: a systematic review. Front Public Health. 2022;10: doi: 10.3389/fpubh.2022.900077
- Locquet M, Diep AN, Beaudart C, et al. A systematic review of prediction models to diagnose COVID-19 in adults admitted to healthcare centers. Arch Public Health. 2021;79(1):105. doi: 10.1186/s13690-021-00630-3
- Lopez CD, Gazgalis A, Boddapati V, et al. Artificial learning and Machine learning decision guidance applications in total hip and nnee arthroplasty: a systematic review. Arthroplast Today. 2021;11:103–112. doi: 10.1016/j.artd.2021.07.012
- Lubelski D, Hersh A, Azad TD, et al. Prediction models in degenerative spine surgery: a systematic review. Global Spine J. 2021;11(1_suppl):79s–88s. doi: 10.1177/2192568220959037
- Maile H, JPO L, Gore D, et al. Machine learning algorithms to detect subclinical keratoconus: systematic review. JMIR Med Inform. 2021;9(12):e27363. doi: 10.2196/27363
- Mangold C, Zoretic S, Thallapureddy K, et al. Machine learning models for predicting neonatal mortality: a systematic review. Neonatology. 2021;118(4):394–405. doi: 10.1159/000516891
- Mari T, Henderson J, Maden M, et al. Systematic review of the effectiveness of machine learning algorithms for classifying pain intensity, phenotype or treatment outcomes using electroencephalogram data. J Pain. 2021;23(3):349–369. doi: 10.1016/j.jpain.2021.07.011
- Matsangidou M, Liampas A, Pittara M, et al. Machine learning in pain medicine: an up-to-date systematic review. Pain Ther. 2021;10(2):1067–1084. doi: 10.1007/s40122-021-00324-2
- Mawdsley E, Reynolds B, Cullen B. A systematic review of the effectiveness of machine learning for predicting psychosocial outcomes in acquired brain injury: which algorithms are used and why? J Neuropsychol. 2021;15(3):319–339. doi: 10.1111/jnp.12244
- Medic G, Kosaner Kließ M, Atallah L, et al. Evidence-based clinical decision support systems for the prediction and detection of three disease states in critical care: a systematic literature review. F1000Res. 2019;8:1728. doi: 10.12688/f1000research.20498.2
- Mei J, Desrosiers C, Frasnelli J. Machine learning for the diagnosis of Parkinson’s disease: a review of literature. Front Aging Neurosci. 2021;13:633752. doi: 10.3389/fnagi.2021.633752
- Mellia JA, Basta MN, Toyoda Y, et al. Natural language processing in surgery a systematic review and meta-analysis. Ann Surg. 2021;273(5):900–908. doi: 10.1097/SLA.0000000000004419
- Miltiadous A, Tzimourta KD, Giannakeas N, et al. Machine learning algorithms for epilepsy detection based on published EEG databases: a systematic review. IEEE Access. 2023;11:564–594. doi: 10.1109/ACCESS.2022.3232563
- Minissi ME, Chicchi Giglioli IA, Mantovani F, et al. Assessment of the autism spectrum disorder based on machine learning and social visual attention: a systematic review. J Autism Dev Disord. 2021;52(5):2187–2202. doi: 10.1007/s10803-021-05106-5
- Miranda L, Paul R, Pütz B, et al. Systematic review of functional MRI applications for psychiatric disease subtyping. Front Psychiatry. 2021;12:665536. doi: 10.3389/fpsyt.2021.665536
- Mirzania D, Thompson AC, Muir KW. Applications of deep learning in detection of glaucoma: a systematic review. Eur J Ophthalmol. 2021;31(4):1618–1642. doi: 10.1177/1120672120977346
- Moezzi M, Shirbandi K, Shahvandi HK, et al. The diagnostic accuracy of Artificial intelligence-assisted CT imaging in COVID-19 disease: a systematic review and meta-analysis. Inform Med Unlocked. 2021;24:100591. doi: 10.1016/j.imu.2021.100591
- Moglia A, Georgiou K, Georgiou E, et al. A systematic review on artificial intelligence in robot-assisted surgery. Int J Surg. 2021;95:106151. doi: 10.1016/j.ijsu.2021.106151
- Mohan BP, Khan SR, Kassab LL, et al. High pooled performance of convolutional neural networks in computer-aided diagnosis of GI ulcers and/or hemorrhage on wireless capsule endoscopy images: a systematic review and meta-analysis. Gastrointest Endosc. 2021;93(2):356–364.e354. doi: 10.1016/j.gie.2020.07.038
- Mondal MRH, Bharati S, Podder P. Diagnosis of COVID-19 using machine learning and deep learning: a review. Curr Med Imaging. 2021;17(12):1403–1418. doi: 10.2174/1573405617666210713113439
- Montazeri M, ZahediNasab R, Farahani A, et al. Machine learning models for image-based diagnosis and prognosis of COVID-19: systematic review. JMIR Med Inform. 2021;9(4):e25181. doi: 10.2196/25181
- Moor M, Rieck B, Horn M, et al. Early prediction of sepsis in the ICU using Machine learning: a systematic review. Front Med. 2021;8:607952. doi: 10.3389/fmed.2021.607952
- Moshawrab M, Adda M, Bouzouane A, et al. Smart wearables for the detection of Cardiovascular diseases: a systematic literature review. Sensors. 2023;23(2):828. doi: 10.3390/s23020828
- Moura FSE, Amin K, Ekwobi C. Artificial intelligence in the management and treatment of burns: a systematic review. Burns Rauma. 2021;9: doi: 10.1093/burnst/tkab022
- Mpanya D, Celik T, Klug E, et al. Predicting mortality and hospitalization in heart failure using machine learning: a systematic literature review. Int J Cardiol Heart Vasc. 2021;34:100773. doi: 10.1016/j.ijcha.2021.100773
- Mughal H, Javed AR, Rizwan M, et al. Parkinson’s disease management via Wearable sensors: a systematic review. IEEE Access. 2022;10:35219–35237. doi: 10.1109/ACCESS.2022.3162844
- Musa N, AYu G, Aljojo N, et al. A systematic review and meta-data analysis on the applications of Deep learning in electrocardiogram. J Ambient Intell Humaniz Comput. 2022;14(7):1–74.
- Musulin J, Baressi Šegota S, Štifanić D, et al. Application of Artificial intelligence-based regression methods in the problem of COVID-19 spread prediction: a systematic review. Int J Environ Res Public Health. 2021;18(8):4287. doi: 10.3390/ijerph18084287
- Nadarajah R, Alsaeed E, Hurdus B, et al. Prediction of incident atrial fibrillation in community-based electronic health records: a systematic review with meta-analysis. Heart. 2021;108(13):1020–1029. doi: 10.1136/heartjnl-2021-320036
- Naemi A, Schmidt T, Mansourvar M, et al. Machine learning techniques for mortality prediction in emergency departments: a systematic review. BMJ Open. 2021;11(11):e052663. doi: 10.1136/bmjopen-2021-052663
- Nafea MS, Ismail ZH. Supervised machine learning and Deep learning techniques for epileptic seizure recognition using EEG signals—A systematic literature review. Bioeng. 2022;9(12):781. doi: 10.3390/bioengineering9120781
- Nasser M, Yusof UK. Deep learning based methods for breast cancer diagnosis: a systematic review and future direction. Diagnostics. 2023;13(1). doi: 10.3390/diagnostics13010161
- Nazarian S, Glover B, Ashrafian H, et al. Diagnostic accuracy of Artificial intelligence and Computer-aided diagnosis for the detection and characterization of colorectal polyps: systematic review and meta-analysis. J Med Internet Res. 2021;23(7):e27370. doi: 10.2196/27370
- Nguyen AV, Blears EE, Ross E, et al. Machine learning applications for the differentiation of primary central nervous system lymphoma from glioblastoma on imaging: a systematic review and meta-analysis. Neurosurg Focus. 2018;45(5):E5. doi: 10.3171/2018.8.FOCUS18325
- Ogink PT, Groot OQ, Bindels BJJ, et al. The use of machine learning prediction models in spinal surgical outcome: an overview of current development and external validation studies. Semin Spine Surg. 2021;33(2):100872. doi: 10.1016/j.semss.2021.100872
- Ogink PT, Groot OQ, Karhade AV, et al. Wide range of applications for machine-learning prediction models in orthopedic surgical outcome: a systematic review. Acta Orthop. 2021;92(5):526–531. doi: 10.1080/17453674.2021.1932928
- Ortíz-Barrios MA, Coba-Blanco DM, Alfaro-Saíz JJ, et al. Process improvement approaches for increasing the response of emergency departments against the COVID-19 pandemic: a systematic review. Int J Environ Res Public Health. 2021;18(16):8814. doi: 10.3390/ijerph18168814
- Ossai CI, Wickramasinghe N. Intelligent decision support with machine learning for efficient management of mechanical ventilation in the intensive care unit – a critical overview. Int J Med Inform. 2021;150:104469. doi: 10.1016/j.ijmedinf.2021.104469
- Paganelli AI, Mondéjar AG, da Silva AC, et al. Real-time data analysis in health monitoring systems: a comprehensive systematic literature review. J Biomed Informat. 2022;127:104009. doi: 10.1016/j.jbi.2022.104009
- Pahwa B, Bali O, Goyal S, et al. APplications of machine learning in pediatric hydrocephalus: a systematic review. Neurol India. 2021;69(8):S568–S577. doi: 10.4103/0028-3886.332287
- Patil S, Habib Awan K, Arakeri G, et al. Machine learning and its potential applications to the genomic study of head and neck cancer—A systematic review. J Oral Pathol Med. 2019;48(9):773–779. doi: 10.1111/jop.12854
- Peralta M, Jannin P, Baxter JSH. Machine learning in deep brain stimulation: a systematic review*. Artif Intell Med. 2021;122:122. doi: 10.1016/j.artmed.2021.102198
- Persad E, Jost K, Honoré A, et al. Neonatal sepsis prediction through clinical decision support algorithms: a systematic review. Acta Paediatr. 2021;110(12):3201–3226. doi: 10.1111/apa.16083
- Popa SL, Ismaiel A, Cristina P, et al. Non-alcoholic fatty liver disease: implementing complete automated diagnosis and staging. a systematic review. Diagnostics. 2021;11(6):1078. doi: 10.3390/diagnostics11061078
- Prasoppokakorn T, Tiyarattanachai T, Chaiteerakij R, et al. Application of artificial intelligence for diagnosis of pancreatic ductal adenocarcinoma by EUS: a systematic review and meta-analysis. Endosc Ultrasound. 2021;11(1):17–26.
- Quaak M, van de Mortel L, Thomas RM, et al. Deep learning applications for the classification of psychiatric disorders using neuroimaging data: systematic review and meta-analysis. NeuroImage Clin. 2021;30:102584. doi: 10.1016/j.nicl.2021.102584
- Quartuccio N, Marrale M, Laudicella R, et al. The role of PET radiomic features in prostate cancer: a systematic review. Clin Transl Imaging. 2021;9(6):579–588. doi: 10.1007/s40336-021-00436-x
- Ramesh S, Chokkara S, Shen T, et al. Applications of artificial intelligence in pediatric oncology: a systematic review. JCO Clin Cancer Inform. 2021;5(5):1208–1219. doi: 10.1200/CCI.21.00102
- Ramos-Lima LF, Waikamp V, Antonelli-Salgado T, et al. The use of machine learning techniques in trauma-related disorders: a systematic review. J Psychiatr Res. 2020;121:159–172. doi: 10.1016/j.jpsychires.2019.12.001
- Ravegnini G, Ferioli M, Morganti AG, et al. Radiomics and artificial intelligence in uterine sarcomas: a systematic review. J Pers Med. 2021;11(11):1179. doi: 10.3390/jpm11111179
- Ren M, Yi PH. Artificial intelligence in orthopedic implant model classification: a systematic review. Skeletal Radiol. 2022;51(2):407–416. doi: 10.1007/s00256-021-03884-8
- Rice P, Pugh M, Geraghty R, et al. Machine learning models for predicting stone-free status after shockwave lithotripsy: a systematic review and meta-analysis. Urology. 2021;156:16–22. doi: 10.1016/j.urology.2021.04.006
- Rowe TW, Katzourou IK, Stevenson-Hoare JO, et al. Machine learning for the life-time risk prediction of Alzheimer’s disease: a systematic review. Brain Commun. 2021;3(4):fcab246. doi: 10.1093/braincomms/fcab246
- Safaei M, Sundararajan EA, Driss M, et al. A systematic literature review on obesity: understanding the causes & consequences of obesity and reviewing various machine learning approaches used to predict obesity. Comput Biol Med. 2021;136:104754. doi: 10.1016/j.compbiomed.2021.104754
- Sajjadian M, Lam RW, Milev R, et al. Machine learning in the prediction of depression treatment outcomes: a systematic review and meta-analysis. Psychol Med. 2021;51(16):2742–2751. doi: 10.1017/S0033291721003871
- Salas-Zárate R, Alor-Hernández G, Salas-Zárate MDP, et al. Detecting depression signs on social media: a systematic literature review. Healthcare. 2022;10(2):291. doi: 10.3390/healthcare10020291
- Salem H, Soria D, Lund JN, et al. A systematic review of the applications of expert systems (ES) and machine learning (ML) in clinical urology. BMC Med Inform Decis Mak. 2021;21(1):223. doi: 10.1186/s12911-021-01585-9
- Sanmarchi F, Fanconi C, Golinelli D, et al. Predict, diagnose, and treat chronic kidney disease with machine learning: a systematic literature review. J Nephrol. 2023;36(4):1101–1117. doi: 10.1007/s40620-023-01573-4
- Sanmarchi F, Fanconi C, Golinelli D, et al. Correction to: predict, diagnose, and treat chronic kidney disease with machine learning: a systematic literature review (journal of nephrology. J Nephrol. 2023;2023(4):1219–1219. doi: 10.1007/s40620-023-01573-4
- Saputro SA, Pattanaprateep O, Pattanateepapon A, et al. Prognostic models of diabetic microvascular complications: a systematic review and meta-analysis. Syst Rev. 2021;10(1):288. doi: 10.1186/s13643-021-01841-z
- Sardar SK, Kumar N, Lee SC. A systematic literature review on machine learning algorithms for human status detection. IEEE Access. 2022;10:74366–74382. doi: 10.1109/ACCESS.2022.3190967
- Scardoni A, Balzarini F, Signorelli C, et al. Artificial intelligence-based tools to control healthcare associated infections: a systematic review of the literature. J Infect Public Health. 2020;13(8):1061–1077. doi: 10.1016/j.jiph.2020.06.006
- Segato A, Marzullo A, Calimeri F, et al. Artificial intelligence for brain diseases: a systematic review. APL Bioeng. 2020;4(4):041503. doi: 10.1063/5.0011697
- Senanayake S, White N, Graves N, et al. Machine learning in predicting graft failure following kidney transplantation: a systematic review of published predictive models. Int J Med Inform. 2019;130:130. doi: 10.1016/j.ijmedinf.2019.103957
- Senders JT, Arnaout O, Karhade AV, et al. Natural and artificial intelligence in neurosurgery: a systematic review. Neurosurgery. 2018;83(2):181–192. doi: 10.1093/neuros/nyx384
- Shi Z, Zhang Z, Liu Z, et al. Methodological quality of machine learning-based quantitative imaging analysis studies in esophageal cancer: a systematic review of clinical outcome prediction after concurrent chemoradiotherapy. Eur J Nucl Med Mol Imaging. 2021;49(8):2462–2481. doi: 10.1007/s00259-021-05658-9
- Shillan D, Sterne JAC, Champneys A, et al. Use of machine learning to analyse routinely collected intensive care unit data: a systematic review. Crit Care. 2019;23(1):284. doi: 10.1186/s13054-019-2564-9
- Shin S, Austin PC, Ross HJ, et al. Machine learning vs. conventional statistical models for predicting heart failure readmission and mortality. ESC Heart Fail. 2021;8(1):106–115. doi: 10.1002/ehf2.13073
- Shlobin NA, Baig AA, Waqas M, et al. Artificial intelligence for large-vessel occlusion stroke: a systematic review. World Neurosurg. 2021 Mar;159:207–220.
- Siddiqui S, Arifeen M, Hopgood A, et al. Deep learning models for the diagnosis and screening of COVID-19: a systematic review. SN Comput Sci. 2022;3(5):397. doi: 10.1007/s42979-022-01326-3
- Smets J, Shevroja E, Hügle T, et al. Machine learning solutions for osteoporosis—a review. J Bone Mineral Res. 2021;36(5):833–851. doi: 10.1002/jbmr.4292
- Soffer S, Klang E, Shimon O, et al. Deep learning for pulmonary embolism detection on computed tomography pulmonary angiogram: a systematic review and meta-analysis. Sci Rep. 2021;11(1):15814. doi: 10.1038/s41598-021-95249-3
- Song DY, Topriceanu CC, Ilie-Ablachim DC, et al. Machine learning with neuroimaging data to identify autism spectrum disorder: a systematic review and meta-analysis. Neuroradiology. 2021;63(12):2057–2072. doi: 10.1007/s00234-021-02774-z
- Song X, Liu X, Liu F, et al. Comparison of machine learning and logistic regression models in predicting acute kidney injury: a systematic review and meta-analysis. Int J Med Inform. 2021;151:104484. doi: 10.1016/j.ijmedinf.2021.104484
- Sorin V, Barash Y, Konen E, et al. Deep learning for natural language processing in radiology-fundamentals and a systematic review. J Am Coll Radiol. 2020;17(5):639–648. doi: 10.1016/j.jacr.2019.12.026
- Srivani M, Murugappan A, Mala T. Cognitive computing technological trends and future research directions in healthcare — a systematic literature review. Artif Intell Med. 2023;138:102513. doi: 10.1016/j.artmed.2023.102513
- Stephens ME, O’Neal CM, Westrup AM, et al. Utility of machine learning algorithms in degenerative cervical and lumbar spine disease: a systematic review. Neurosurg Rev. 2021;45(2):965–978. doi: 10.1007/s10143-021-01624-z
- Stewart J, Lu J, Goudie A, et al. Applications of machine learning to undifferentiated chest pain in the emergency department: a systematic review. PLoS One. 2021;16(8):e0252612. doi: 10.1371/journal.pone.0252612
- Stokes K, Castaldo R, Federici C, et al. The use of artificial intelligence systems in diagnosis of pneumonia via signs and symptoms: a systematic review. Biomedical Signal Processing And Control. 2022;72:103325. doi: 10.1016/j.bspc.2021.103325
- Subramanian H, Dey R, Brim WR, et al. Trends in development of Novel Machine learning methods for the identification of Gliomas in datasets that include non-glioma images: a systematic review. Front Oncol. 2021;11:788819. doi: 10.3389/fonc.2021.788819
- Syeda HB, Syed M, Sexton KW, et al. Role of machine learning techniques to tackle the covid-19 crisis: systematic review. JMIR Med Inform. 2021;9(1):e23811. doi: 10.2196/23811
- Syer T, Mehta P, Antonelli M, et al. Artificial intelligence compared to Radiologists for the initial diagnosis of prostate cancer on magnetic resonance imaging: a systematic review and recommendations for future studies. Cancers (Basel). 2021;13(13):3318. doi: 10.3390/cancers13133318
- Tabatabaei M, Razaei A, Sarrami AH, et al. Current status and quality of machine learning-based radiomics studies for glioma grading: a systematic review. Oncology. 2021;99(7):433–443. doi: 10.1159/000515597
- Tan KR, Seng JJB, Kwan YH, et al. Evaluation of machine learning methods developed for prediction of Diabetes complications: a systematic review. J Diabetes Sci Technol. 2021;17(2):474–489. doi: 10.1177/19322968211056917
- Teo YH, Lim ICZY, Tseng FS, et al. Predicting clinical outcomes in acute ischemic stroke patients undergoing endovascular thrombectomy with machine learning: a systematic review and meta-analysis. Clin Neuroradiol. 2021;31(4):1121–1130. doi: 10.1007/s00062-020-00990-3
- Tewarie IA, Senders JT, Kremer S, et al. Survival prediction of glioblastoma patients-are we there yet? a systematic review of prognostic modeling for glioblastoma and its clinical potential. Neurosurg Rev. 2021;44(4):2047–2057. doi: 10.1007/s10143-020-01430-z
- Triantafyllidis A, Polychronidou E, Alexiadis A, et al. Computerized decision support and machine learning applications for the prevention and treatment of childhood obesity: a systematic review of the literature. Artif Intell Med. 2020;104:101844. doi: 10.1016/j.artmed.2020.101844
- Ugga L, Perillo T, Cuocolo R, et al. Meningioma MRI radiomics and machine learning: systematic review, quality score assessment, and meta-analysis. Neuroradiology. 2021;63(8):1293–1304. doi: 10.1007/s00234-021-02668-0
- van Kempen EJ, Post M, Mannil M, et al. Accuracy of machine learning algorithms for the classification of molecular features of gliomas on MRI: a systematic literature review and meta-analysis. Cancers (Basel). 2021;13(11):2606. doi: 10.3390/cancers13112606
- van Kempen EJ, Post M, Mannil M, et al. Performance of machine learning algorithms for glioma segmentation of brain MRI: a systematic literature review and meta-analysis. Eur Radiol. 2021;31(12):9638–9653. doi: 10.1007/s00330-021-08035-0
- Volpe S, Pepa M, Zaffaroni M, et al. Machine learning for head and neck cancer: a safe bet?-a clinically oriented systematic review for the radiation oncologist. Front Oncol. 2021;11:772663. doi: 10.3389/fonc.2021.772663
- Wang W, Kiik M, Peek N, et al. A systematic review of machine learning models for predicting outcomes of stroke with structured data. PLoS One. 2020;15(6):e0234722. doi: 10.1371/journal.pone.0234722
- Wesselius FJ, van Schie MS, De Groot NMS, et al. Digital biomarkers and algorithms for detection of atrial fibrillation using surface electrocardiograms: a systematic review. Comput Biol Med. 2021;133:104404. doi: 10.1016/j.compbiomed.2021.104404
- Wongkoblap A, Vadillo MA, Curcin V. Researching mental Health disorders in the era of social media: systematic review. J Med Internet Res. 2017;19(6):e228. doi: 10.2196/jmir.7215
- Wu JH, Liu TYA, Hsu WT, et al. Performance and limitation of machine learning algorithms for diabetic retinopathy screening: meta-analysis. J Med Internet Res. 2021;23(7):e23863. doi: 10.2196/23863
- Xu L, He B, Zhang Y, et al. Prognostic models for amyotrophic lateral sclerosis: a systematic review. J Neurol. 2021;268(9):3361–3370. doi: 10.1007/s00415-021-10508-7
- Yan MY, Gustad LT, Ø N. Sepsis prediction, early detection, and identification using clinical text for machine learning: a systematic review. J Am Med Inform Assoc. 2021;29(3):559–575. doi: 10.1093/jamia/ocab236
- Yeo M, Tahayori B, Kok HK, et al. Review of deep learning algorithms for the automatic detection of intracranial hemorrhages on computed tomography head imaging. J Neurointerv Surg. 2021;13(4):369–378. doi: 10.1136/neurintsurg-2020-017099
- Yin J, Ngiam KY, Teo HH. Role of Artificial intelligence applications in Real-life clinical practice: systematic review. J Med Internet Res. 2021;23(4):e25759. doi: 10.2196/25759
- Yu K, Syed MN, Bernardis E, et al. Machine learning applications in the evaluation and management of Psoriasis: a systematic review. J Psoriasis Psoriatic Arthritis. 2020;5(4):147–159. doi: 10.1177/2475530320950267
- Zakhem GA, Fakhoury JW, Motosko CC, et al. Characterizing the role of dermatologists in developing artificial intelligence for assessment of skin cancer. J Am Acad Dermatol. 2021;85(6):1544–1556. doi: 10.1016/j.jaad.2020.01.028
- Zaunseder E, Haupt S, Mütze U, et al. Opportunities and challenges in machine learning-based newborn screening-a systematic literature review. JIMD Rep. 2022;63(3):250–261. doi: 10.1002/jmd2.12285
- Zeiser FA, da Costa CA, Roehe AV, et al. Breast cancer intelligent analysis of histopathological data: a systematic review. Appl Soft Comput. 2021;113:113. doi: 10.1016/j.asoc.2021.107886
- Zhang L, Sun JQ, Jiang BB, et al. Development of artificial intelligence in epicardial and pericoronary adipose tissue imaging: a systematic review. Eur J Hybrid Imaging. 2021;5(1). doi: 10.1186/s41824-021-00107-0
- Zhao Y, Wood EP, Mirin N, et al. Social determinants in machine learning Cardiovascular disease prediction models: a systematic review. Am J Prev Med. 2021;61(4):596–605. doi: 10.1016/j.amepre.2021.04.016
- Zheng QH, Yang L, Zeng B, et al. Artificial intelligence performance in detecting tumor metastasis from medical radiology imaging: a systematic review and meta-analysis. ECLINICALMEDICINE. 2021;31:100669. doi: 10.1016/j.eclinm.2020.100669
- Zheng Y, Dickson VV, Blecker S, et al. Identifying patients with hypoglycemia using natural language processing: systematic literature review. JMIR Diabetes. 2022;7(2):e34681. doi: 10.2196/34681
- Zhou Y, Ge YT, Shi XL, et al. Machine learning predictive models for acute pancreatitis: a systematic review. Int J Med Inform. 2022;157:104641. doi: 10.1016/j.ijmedinf.2021.104641
- Zhu T, Li K, Herrero P, et al. Deep learning for diabetes: a systematic review. IEEE J Biomed Health Inform. 2021;25(7):2744–2757. doi: 10.1109/JBHI.2020.3040225
- Maniah, Soewito B, Lumban Gaol F, et al. A systematic literature review: Risk analysis in cloud migration. J King Saud Univ Comput Inf Sci. 2022;34(6, Part B):3111–3120. doi: 10.1016/j.jksuci.2021.01.008
- Kitchenham B, Pearl Brereton O, Budgen D, et al. Systematic literature reviews in software engineering – a systematic literature review. Inf Software Technol. 2009;51(1):7–15. doi: 10.1016/j.infsof.2008.09.009
- Kitchenham B, Charters S Guidelines for performing systematic literature reviews in software engineering, Technical Report EBSE 2007-001. (2007).
- Brereton P, Kitchenham BA, Budgen D, et al. Lessons from applying the systematic literature review process within the software engineering domain. J Syst Software. 2007;80(4):571–583. doi: 10.1016/j.jss.2006.07.009
- Luo W, Phung D, Tran T, et al. Guidelines for developing and reporting machine learning predictive models in Biomedical research: a Multidisciplinary View. J Med Internet Res. 2016;18(12):e323. doi: 10.2196/jmir.5870
- Zeng X, Zhang Y, Kwong JS, et al. The methodological quality assessment tools for preclinical and clinical studies, systematic review and meta-analysis, and clinical practice guideline: a systematic review. J Evid Based Med. 2015;8(1):2–10. doi: 10.1111/jebm.12141
- Kable AK, Pich J, Maslin-Prothero SE. A structured approach to documenting a search strategy for publication: a 12 step guideline for authors. Nurse Educ Today. 2012;32(8):878–886. doi: 10.1016/j.nedt.2012.02.022
- Kim HR, Sung M, Park JA, et al. Analyzing adverse drug reaction using statistical and machine learning methods: a systematic review. Medicine. 2022;101(25):e29387. doi: 10.1097/MD.0000000000029387
- Paganelli AI, Mondéjar AG, da Silva AC, et al. Real-time data analysis in health monitoring systems: a comprehensive systematic literature review. J Biomed Informatics. 2022;127:104009. doi: 10.1016/j.jbi.2022.104009
- Ariji Y, Fukuda M, Kise Y, et al. Contrast-enhanced computed tomography image assessment of cervical lymph node metastasis in patients with oral cancer by using a deep learning system of artificial intelligence. Oral Surg Oral Med Oral Pathol Oral Radiol. 2019;127(5):458–463. doi: 10.1016/j.oooo.2018.10.002
- Ariji Y, Sugita Y, Nagao T, et al. CT evaluation of extranodal extension of cervical lymph node metastases in patients with oral squamous cell carcinoma using deep learning classification. Oral Radiol. 2020;36(2):148–155. doi: 10.1007/s11282-019-00391-4
- Kwon JM, Jeon KH, Kim HM, et al. Comparing the performance of artificial intelligence and conventional diagnosis criteria for detecting left ventricular hypertrophy using electrocardiography. EP Europace. 2020;22(3):412–419. doi: 10.1093/europace/euz324
- Nakajima K, Kudo T, Nakata T, et al. Diagnostic accuracy of an artificial neural network compared with statistical quantitation of myocardial perfusion images: a Japanese multicenter study. Eur J Nucl Med Mol Imaging. 2017;44(13):2280–2289. doi: 10.1007/s00259-017-3834-x
- Al-Aswad LA, Kapoor R, Chu CK, et al. Evaluation of a deep learning system for identifying glaucomatous optic neuropathy based on color fundus photographs. J Glaucoma. 2019;28(12):1029–1034. doi: 10.1097/IJG.0000000000001319
- Liu S, Graham SL, Schulz A, et al. A Deep Learning-Based Algorithm Identifies Glaucomatous Discs Using Monoscopic Fundus Photographs. Ophthalmol Glaucoma. 2018;1(1):15–22. doi: 10.1016/j.ogla.2018.04.002
- Phene S, Dunn RC, Hammel N, et al. Deep learning and glaucoma specialists: the relative importance of optic disc features to predict glaucoma referral in fundus photographs. Ophthalmol. 2019;126(12):1627–1639. doi: 10.1016/j.ophtha.2019.07.024
- Shibata N, Tanito M, Mitsuhashi K, et al. Development of a deep residual learning algorithm to screen for glaucoma from fundus photography. Sci Rep. 2018;8(1):14665. doi: 10.1038/s41598-018-33013-w
- Seo E, Jaccard N, Trikha S, et al. Automated evaluation of optic disc images for manifest glaucoma detection using a deep-learning, neural network-based algorithm. Invest Ophthalmol Visual Sci. 2018;59(9):2080–2080.
- Jammal AA, Thompson AC, Mariottoni EB, et al. Human versus Machine: comparing a Deep learning algorithm to human gradings for detecting glaucoma on fundus photographs. Am J Ophthalmol. 2020;211:123–131. doi: 10.1016/j.ajo.2019.11.006
- Brinker TJ, Hekler A, Enk AH, et al. Deep learning outperformed 136 of 157 dermatologists in a head-to-head dermoscopic melanoma image classification task. Eur J Cancer. 2019;113:47–54. doi: 10.1016/j.ejca.2019.04.001
- Brinker TJ, Hekler A, Enk AH, et al. Deep neural networks are superior to dermatologists in melanoma image classification. Eur J Cancer. 2019;119:11–17. doi: 10.1016/j.ejca.2019.05.023
- Yu C, Yang S, Kim W, et al. Acral melanoma detection using a convolutional neural network for dermoscopy images. PLoS One. 2018;13(3):e0193321. doi: 10.1371/journal.pone.0193321
- Marchetti MA, Codella NCF, Dusza SW, et al. Results of the 2016 international skin imaging collaboration international symposium on biomedical imaging challenge: comparison of the accuracy of computer algorithms to dermatologists for the diagnosis of melanoma from dermoscopic images. J Am Acad Dermatol. 2018;78(2):270–277.e271. doi: 10.1016/j.jaad.2017.08.016
- Marchetti MA, Liopyris K, Dusza SW, et al. Computer algorithms show potential for improving dermatologists’ accuracy to diagnose cutaneous melanoma: results of the international skin imaging collaboration 2017. J Am Acad Dermatol. 2020;82(3):622–627. doi: 10.1016/j.jaad.2019.07.016
- Haenssle HA, Fink C, Schneiderbauer R, et al. Man against machine: diagnostic performance of a deep learning convolutional neural network for dermoscopic melanoma recognition in comparison to 58 dermatologists. Ann Oncol. 2018;29(8):1836–1842. doi: 10.1093/annonc/mdy166
- Haenssle HA, Fink C, Toberer F, et al. Man against machine reloaded: performance of a market-approved convolutional neural network in classifying a broad spectrum of skin lesions in comparison with 96 dermatologists working under less artificial conditions. Ann Oncol. 2020;31(1):137–143. doi: 10.1016/j.annonc.2019.10.013
- Haenssle HA, Winkler JK, Fink C, et al. Skin lesions of face and scalp - classification by a market-approved convolutional neural network in comparison with 64 dermatologists. Eur J Cancer. 2021;144:192–199. doi: 10.1016/j.ejca.2020.11.034
- Tschandl P, Codella N, Akay BN, et al. Comparison of the accuracy of human readers versus machine-learning algorithms for pigmented skin lesion classification: an open, web-based, international, diagnostic study. Lancet Oncol. 2019;20(7):938–947. doi: 10.1016/S1470-2045(19)30333-X
- Maron RC, Weichenthal M, Utikal JS, et al. Systematic outperformance of 112 dermatologists in multiclass skin cancer image classification by convolutional neural networks. Eur J Cancer. 2019;119:57–65. doi: 10.1016/j.ejca.2019.06.013
- Tschandl P, Rosendahl C, Akay BN, et al. Expert-level diagnosis of nonpigmented skin cancer by combined convolutional neural networks. JAMA Dermatol. 2019;155(1):58–65. doi: 10.1001/jamadermatol.2018.4378
- Fujisawa Y, Otomo Y, Ogata Y, et al. Deep-learning-based, computer-aided classifier developed with a small dataset of clinical images surpasses board-certified dermatologists in skin tumour diagnosis. Br J Dermatol. 2019;180(2):373–381. doi: 10.1111/bjd.16924
- Jinnai S, Yamazaki N, Hirano Y, et al. The development of a skin cancer classification system for pigmented skin lesions using Deep learning. Biomolecules. 2020;10(8):1123. doi: 10.3390/biom10081123
- Han SS, Park I, Eun Chang S, et al. Augmented intelligence Dermatology: deep neural networks empower medical professionals in diagnosing skin cancer and predicting treatment options for 134 skin disorders. J Invest Dermatol. 2020;140(9):1753–1761. doi: 10.1016/j.jid.2020.01.019
- Han SS, Kim MS, Lim W, et al. Classification of the clinical images for benign and malignant cutaneous tumors using a deep learning algorithm. J Invest Dermatol. 2018;138(7):1529–1538. doi: 10.1016/j.jid.2018.01.028
- Brinker TJ, Hekler A, Enk AH, et al. A convolutional neural network trained with dermoscopic images performed on par with 145 dermatologists in a clinical melanoma image classification task. Eur J Cancer. 2019;111:148–154. doi: 10.1016/j.ejca.2019.02.005
- Han SS, Moon IJ, Kim SH, et al. Assessment of deep neural networks for the diagnosis of benign and malignant skin neoplasms in comparison with dermatologists: a retrospective validation study. PLOS Med. 2020;17(11):e1003381. doi: 10.1371/journal.pmed.1003381
- Brinker TJ, Schmitt M, Krieghoff-Henning EI, et al. Diagnostic performance of artificial intelligence for histologic melanoma recognition compared to 18 international expert pathologists. J Am Acad Dermatol. 2022;86(3):640–642. doi: 10.1016/j.jaad.2021.02.009
- Brennan M, Puri S, Ozrazgat-Baslanti T, et al. Comparing clinical judgment with the MySurgeryRisk algorithm for preoperative risk assessment: a pilot usability study. Surgery. 2019;165(5):1035–1045. doi: 10.1016/j.surg.2019.01.002
- Kambakamba P, Mannil M, Herrera PE, et al. The potential of machine learning to predict postoperative pancreatic fistula based on preoperative, non-contrast-enhanced CT: a proof-of-principle study. Surgery. 2020;167(2):448–454. doi: 10.1016/j.surg.2019.09.019
- Yala A, Schuster T, Miles R, et al. A Deep learning model to triage screening mammograms: a simulation study. Radiology. 2019;293(1):38–46. doi: 10.1148/radiol.2019182908
- McKinney SM, Sieniek M, Godbole V, et al. International evaluation of an AI system for breast cancer screening. Nature. 2020;577(7788):89–94. doi: 10.1038/s41586-019-1799-6
- Balta C, Rodríguez-Ruiz A, Mieskes C, et al. Going from double to single reading for screening exams labeled as likely normal by AI: what is the impact? In: 15th International Workshop on Breast Imaging; Leuven, Belgium; 2020.
- Dembrower K, Wåhlin E, Liu Y, et al. Effect of artificial intelligence-based triaging of breast cancer screening mammograms on cancer detection and radiologist workload: a retrospective simulation study. Lancet Digit Health. 2020;2(9):e468–e474. doi: 10.1016/S2589-7500(20)30185-0
- Kyono T, Gilbert FJ, van der Schaar M. Improving workflow efficiency for mammography using machine learning. J Am Coll Radiol. 2020;17(1 Pt A):56–63. doi: 10.1016/j.jacr.2019.05.012
- Rodriguez-Ruiz A, Lång K, Gubern-Merida A, et al. Can we reduce the workload of mammographic screening by automatic identification of normal exams with artificial intelligence? A feasibility study. Eur Radiol. 2019;29(9):4825–4832. doi: 10.1007/s00330-019-06186-9
- Geras KJ, Wolfson S, Kim SG, et al. High-resolution breast cancer screening with multi-view deep convolutional neural networks. ArXiv. 2017. abs/1703.07047.
- Lotter W, Diab AR, Haslam B, et al. Robust breast cancer detection in mammography and digital breast tomosynthesis using an annotation-efficient deep learning approach. Nat Med. 2021;27(2):244–249. doi: 10.1038/s41591-020-01174-9
- Rodríguez-Ruiz A, Krupinski E, Mordang JJ, et al. Detection of breast cancer with Mammography: effect of an Artificial intelligence support system. Radiology. 2019;290(2):305–314. doi: 10.1148/radiol.2018181371
- Schaffter T, Buist DSM, Lee CI, et al. Evaluation of combined Artificial intelligence and radiologist assessment to Interpret screening mammograms. JAMA Netw Open. 2020;3(3):e200265. doi: 10.1001/jamanetworkopen.2020.0265
- Salim M, Wåhlin E, Dembrower K, et al. External evaluation of 3 commercial artificial intelligence algorithms for independent assessment of screening mammograms. JAMA Oncol. 2020;6(10):1581–1588. doi: 10.1001/jamaoncol.2020.3321
- Cowley JB The use of knowledge discovery databases in the identification of patients with colorectal cancer. (Ed.^(Eds) (2012)
- Wei JW, Suriawinata AA, Vaickus LJ, et al. Evaluation of a deep neural network for automated classification of colorectal polyps on histopathologic slides. JAMA Netw Open. 2020;3(4):e203398. doi: 10.1001/jamanetworkopen.2020.3398
- Song Z, Yu C, Zou S, et al. Automatic deep learning-based colorectal adenoma detection system and its similarities with pathologists. BMJ Open. 2020;10(9):e036423. doi: 10.1136/bmjopen-2019-036423
- Bychkov D, Linder N, Turkki R, et al. Deep learning based tissue analysis predicts outcome in colorectal cancer. Sci Rep. 2018;8(1):3395. doi: 10.1038/s41598-018-21758-3
- Geessink OGF, Baidoshvili A, Klaase JM, et al. Computer aided quantification of intratumoral stroma yields an independent prognosticator in rectal cancer. Cell Oncol. 2019;42(3):331–341. doi: 10.1007/s13402-019-00429-z
- Kather JN, Krisam J, Charoentong P, et al. Predicting survival from colorectal cancer histology slides using deep learning: a retrospective multicenter study. PLOS Med. 2019;16(1):e1002730. doi: 10.1371/journal.pmed.1002730
- Zhao K, Li Z, Yao S, et al. Artificial intelligence quantified tumour-stroma ratio is an independent predictor for overall survival in resectable colorectal cancer. EBioMedicine. 2020;61:103054. doi: 10.1016/j.ebiom.2020.103054
- Suh HB, Choi YS, Bae S, et al. Primary central nervous system lymphoma and atypical glioblastoma: differentiation using radiomics approach. Eur Radiol. 2018;28(9):3832–3839. doi: 10.1007/s00330-018-5368-4
- Kang D, Park JE, Kim YH, et al. Diffusion radiomics as a diagnostic model for atypical manifestation of primary central nervous system lymphoma: development and multicenter external validation. Neuro Oncol. 2018;20(9):1251–1261. doi: 10.1093/neuonc/noy021
- Alcaide-Leon P, Dufort P, Geraldo AF, et al. Differentiation of enhancing glioma and primary Central nervous system lymphoma by texture-based Machine learning. AJNR Am J Neuroradiol. 2017;38(6):1145–1150. doi: 10.3174/ajnr.A5173
- Yamashita K, Yoshiura T, Arimura H, et al. Performance evaluation of radiologists with artificial neural network for differential diagnosis of intra-axial cerebral tumors on MR images. AJNR Am J Neuroradiol. 2008;29(6):1153–1158. doi: 10.3174/ajnr.A1037
- Rehm GB, Han J, Kuhn BT, et al. Creation of a robust and generalizable Machine learning classifier for patient ventilator asynchrony. Methods Inf Med. 2018;57(4):208–219. doi: 10.3414/ME17-02-0012
- Bakkes T, Montree RJH, Mischi M, et al. A machine learning method for automatic detection and classification of patient-ventilator asynchrony. Annu Int Conf IEEE Eng Med Biol Soc; Montreal, QC, Canada; 2020. p. 150–153.
- Mulqueeny Q, Redmond SJ, Tassaux D, et al. Automated detection of asynchrony in patient-ventilator interaction. Annu Int Conf IEEE Eng Med Biol Soc; Minneapolis, Minnesota; 2009. p. 5324–5327.
- Aghdam MA, Sharifi A, Pedram MM. Diagnosis of Autism spectrum disorders in young children based on resting-state functional magnetic resonance imaging data using convolutional neural networks. J Digit Imaging. 2019;32(6):899–918. doi: 10.1007/s10278-019-00196-1
- Petrucci K, Petrucci P, Canfield K, et al. Evaluation of UNIS: urological Nursing information systems. Proc Annu Symp Comput Appl Med Care. 1991;1:43–47.
- Gorman R. Expert system for management of urinary incontinence in women. Proc Annu Symp Comput Appl Med Care. 1995;1:527–531.
- Chang PL, Li YC, Wang TM, et al. Evaluation of a decision-support system for preoperative staging of prostate cancer. Med Decis Making. 1999;19(4):419–427. doi: 10.1177/0272989X9901900410
- Koutsojannis C, Hatzilygeroudis I. FESMI: A Fuzzy Expert System for Diagnosis and Treatment of Male Impotence. In: International Conference on Knowledge-Based Intelligent Information & Engineering Systems; Athens, Greece; 2004.
- Koutsojannis C, Nabil E, Tsimara M, et al. Using machine learning techniques to improve the behaviour of a medical decision support system for prostate diseases (2009).
- Altunay S, Telatar Z, Eroğul O, et al. A new approach to urinary system dynamics problems: evaluation and classification of uroflowmeter signals using artificial neural networks. Expert Syst Appl. 2009;36(3):4891–4895. doi: 10.1016/j.eswa.2008.05.051
- Koutsojannis C, Lithari C, Hatzilygeroudis I. Managing urinary incontinence through hand-held real-time decision support aid. Comput Methods Programs Biomed. 2012;107(1):84–89. doi: 10.1016/j.cmpb.2012.02.012
- Hassanien AE, Alqaheri H, El-Dahshan E-S. Prostate boundary detection in ultrasound images using biologically-inspired spiking neural network. Appl Soft Comput. 2011;11(2):2035–2041. doi: 10.1016/j.asoc.2010.07.001
- Torshizi AD, Zarandi MH, Torshizi GD, et al. A hybrid fuzzy-ontology based intelligent system to determine level of severity and treatment recommendation for benign prostatic hyperplasia. Comput Methods Programs Biomed. 2014;113(1):301–313. doi: 10.1016/j.cmpb.2013.09.021
- Xiao D, Zhang G, Liu Y, et al. 3D detection and extraction of bladder tumors via MR virtual cystoscopy. Int J Comput Assist Radiol Surg. 2016;11(1):89–97. doi: 10.1007/s11548-015-1234-x
- Hurst RE, Bonner RB, Ashenayi K, et al. Neural net-based identification of cells expressing the p300 tumor-related antigen using fluorescence image analysis. Cytometry. 1997;27(1):36–42. 3rd. doi: 10.1002/(SICI)1097-0320(19970101)27:1<36:AID-CYTO5>3.0.CO;2-J
- Hao AT, Wu LP, Kumar A, et al. Nursing process decision support system for urology ward. Int J Med Inform. 2013;82(7):604–612. doi: 10.1016/j.ijmedinf.2013.02.006
- Lopes MH, Ortega NR, Silveira PS, et al. Fuzzy cognitive map in differential diagnosis of alterations in urinary elimination: a nursing approach. Int J Med Inform. 2013;82(3):201–208. doi: 10.1016/j.ijmedinf.2012.05.012
- Koutsojannis C, Tsimara M, Nabil E. HIROFILOS: a medical expert system for prostate diseases. 7th WSEAS international conference on Computational intelligence, man-machine systems and cybernetics; 2007; Venice, Italy; 2008.
- Qiu W, Kuang H, Teleg E, et al. Machine learning for detecting early infarction in acute stroke with non-contrast-enhanced CT. Radiology. 2020;294(3):638–644. doi: 10.1148/radiol.2020191193
- Ni Q, Sun ZY, Qi L, et al. A deep learning approach to characterize 2019 coronavirus disease (COVID-19) pneumonia in chest CT images. Eur Radiol. 2020;30(12):6517–6527. doi: 10.1007/s00330-020-07044-9
- Tomita N, Cheung YY, Hassanpour S. Deep neural networks for automatic detection of osteoporotic vertebral fractures on CT scans. Comput Biol Med. 2018;98:8–15. doi: 10.1016/j.compbiomed.2018.05.011
- Murata K, Endo K, Aihara T, et al. Artificial intelligence for the detection of vertebral fractures on plain spinal radiography. Sci Rep. 2020;10(1):20031. doi: 10.1038/s41598-020-76866-w
- Cheng CT, Ho TY, Lee TY, et al. Application of a deep learning algorithm for detection and visualization of hip fractures on plain pelvic radiographs. Eur Radiol. 2019;29(10):5469–5477. doi: 10.1007/s00330-019-06167-y
- Yu JS, Yu SM, Erdal BS, et al. Detection and localisation of hip fractures on anteroposterior radiographs with artificial intelligence: proof of concept. Clin Radiol. 2020;75(3):.e237.231–.e237.239. doi: 10.1016/j.crad.2019.10.022
- Yamada Y, Maki S, Kishida S, et al. Automated classification of hip fractures using deep convolutional neural networks with orthopedic surgeon-level accuracy: ensemble decision-making with antero-posterior and lateral radiographs. Acta Orthop. 2020;91(6):699–704. doi: 10.1080/17453674.2020.1803664
- Jiménez-Sánchez A, Kazi A, Albarqouni S, et al. Precise proximal femur fracture classification for interactive training and surgical planning. Int J Comput Assist Radiol Surg. 2020;15(5):847–857. doi: 10.1007/s11548-020-02150-x
- Adams M, Chen W, Holcdorf D, et al. Computer vs human: deep learning versus perceptual training for the detection of neck of femur fractures. J Med Imaging Radiat Oncol. 2019;63(1):27–32. doi: 10.1111/1754-9485.12828
- Mawatari T, Hayashida Y, Katsuragawa S, et al. The effect of deep convolutional neural networks on radiologists’ performance in the detection of hip fractures on digital pelvic radiographs. Eur J Radiol. 2020;130:109188. doi: 10.1016/j.ejrad.2020.109188
- Urakawa T, Tanaka Y, Goto S, et al. Detecting intertrochanteric hip fractures with orthopedist-level accuracy using a deep convolutional neural network. Skeletal Radiol. 2019;48(2):239–244. doi: 10.1007/s00256-018-3016-3
- Chung SW, Han SS, Lee JW, et al. Automated detection and classification of the proximal humerus fracture by using deep learning algorithm. Acta Orthop. 2018;89(4):468–473. doi: 10.1080/17453674.2018.1453714
- Olczak J, Fahlberg N, Maki A, et al. Artificial intelligence for analyzing orthopedic trauma radiographs. Acta Orthop. 2017;88(6):581–586. doi: 10.1080/17453674.2017.1344459
- Lindsey R, Daluiski A, Chopra S, et al. Deep neural network improves fracture detection by clinicians. Proc Natl Acad Sci U S A. 2018;115(45):11591–11596. doi: 10.1073/pnas.1806905115
- Qazi AA, Pekar V, Kim J, et al. Auto-segmentation of normal and target structures in head and neck CT images: a feature-driven model-based approach. Med Phys. 2011;38(11):6160–6170. doi: 10.1118/1.3654160
- Misra-Hebert AD, Milinovich A, Zajichek A, et al. Natural language processing improves detection of nonsevere hypoglycemia in medical records versus coding alone in patients with type 2 diabetes but does not improve prediction of severe hypoglycemia events: an analysis using the electronic medical record in a large health system. Diabetes Care. 2020;43(8):1937–1940. doi: 10.2337/dc19-1791
- Hekler A, Utikal JS, Enk AH, et al. Deep learning outperformed 11 pathologists in the classification of histopathological melanoma images. Eur J Cancer. 2019;118:91–96. doi: 10.1016/j.ejca.2019.06.012
- Kim HE, Kim HH, Han BK, et al. Changes in cancer detection and false-positive recall in mammography using artificial intelligence: a retrospective, multireader study. Lancet Digit Health. 2020;2(3):e138–e148. doi: 10.1016/S2589-7500(20)30003-0
- European Patent Office. (Ed.^(Eds)
- FDA. Artificial intelligence and Machine learning (AI/ML)-enabled medical devices. (Ed.^(Eds) (2022)
- Muehlematter UJ, Daniore P, Vokinger KN. Approval of artificial intelligence and machine learning-based medical devices in the USA and Europe (2015–20): a comparative analysis. Lancet Digital Health. 2021;3(3):e195–e203. doi: 10.1016/S2589-7500(20)30292-2
- Varoquaux G. Cross-validation failure: Small sample sizes lead to large error bars. Neuroimage. 2018;180(Pt A):68–77. doi: 10.1016/j.neuroimage.2017.06.061
- Vabalas A, Gowen E, Poliakoff E, et al. Machine learning algorithm validation with a limited sample size. PLoS One. 2019;14(11):e0224365. doi: 10.1371/journal.pone.0224365
- Campello VM, Gkontra P, Izquierdo C, et al. Multi-centre, multi-vendor and multi-disease cardiac segmentation: the M&Ms challenge. IEEE Trans Med Imaging. 2021;40(12):3543–3554. doi: 10.1109/TMI.2021.3090082
- De Fauw J, Ledsam JR, Romera-Paredes B, et al. Clinically applicable deep learning for diagnosis and referral in retinal disease. Nat Med. 2018;24(9):1342–1350. doi: 10.1038/s41591-018-0107-6
- Celi LA, Cellini J, Charpignon M-L, et al. Sources of bias in artificial intelligence that perpetuate healthcare disparities—A global review. PLOS Digital Health. 2022;1(3):e0000022. doi: 10.1371/journal.pdig.0000022
- Khan SM, Liu X, Nath S, et al. A global review of publicly available datasets for ophthalmological imaging: barriers to access, usability, and generalisability. Lancet Digit Health. 2021;3(1):e51–e66. doi: 10.1016/S2589-7500(20)30240-5
- de Groof AJ, Struyvenberg MR, van der Putten J, et al. Deep-learning system detects neoplasia in patients with barrett’s esophagus with higher accuracy than endoscopists in a multistep training and validation study with benchmarking. Gastroenterology. 2020;158(4):915–929.e914. doi: 10.1053/j.gastro.2019.11.030
- Nagendran M, Chen Y, Lovejoy CA, et al. Artificial intelligence versus clinicians: systematic review of design, reporting standards, and claims of deep learning studies. BMJ. 2020;368:m689. doi: 10.1136/bmj.m689
- Liu X, Faes L, Kale AU, et al. A comparison of deep learning performance against health-care professionals in detecting diseases from medical imaging: a systematic review and meta-analysis. Lancet Digital Health. 2019;1(6):e271–e297. doi: 10.1016/S2589-7500(19)30123-2
- Congress. CBONaftUS. Research and development in the Pharmaceutical Industry. (Ed.^(Eds) (2023)
- Associations. EFooPIa. The root cause of unavailability and delay to innovative medicines: reducing the time before patients have access to innovative medicines. (Ed.^(Eds) (2022)
- de Hond AAH, Leeuwenberg AM, Hooft L, et al. Guidelines and quality criteria for artificial intelligence-based prediction models in healthcare: a scoping review. NPJ Digit Med. 2022;5(1):2. doi: 10.1038/s41746-021-00549-7
- Parikh RB, Helmchen LA. Paying for artificial intelligence in medicine. NPJ Digit Med. 2022;5(1):63. doi: 10.1038/s41746-022-00609-6
- Abràmoff MD, Roehrenbeck C, Trujillo S, et al. A reimbursement framework for artificial intelligence in healthcare. NPJ Digit Med. 2022;5(1):72. doi: 10.1038/s41746-022-00621-w