ABSTRACT
Introduction
An avalanche of early stage cancer clinical trials is coming. The majority of these solely use surrogate outcomes that have not been validated against a target outcome of interest (e.g. overall survival). Current HTA guidance on surrogate outcome validation are not methodologically or practically conducive to this scenario.
Areas covered
We provide a high-level overview of methods, approaches, and conceptual thinking for making better use of limited evidence within early stage cancer HTA submissions. We outline regulatory and HTA issues and emphasize how evidence transitions from one to another, what major gaps currently exist, and how these may be bridged. We summarize current methodologies and practices, their pros and cons. We outline how complementary measurements strengthen evaluations and address fallacies and biases of conventional statistical methods for surrogate outcomes validation. The value of real-world data to support some of the necessary validity components is discussed. Lastly, we address the importance of the patient voice for better understanding which surrogate outcomes may appropriately inform HTA.
Expert opinion
Conventional surrogate outcome validation represents a fraught and sub-optimal framework for HTA purposes, particularly for early stage cancer. Tools for optimizing use of limited evidence exist. Education of stakeholders is highly needed.
1. Introduction
Several hundreds of early stage cancer trials are currently being conducted or planned [Citation1]. Unlike clinical trials for later stage cancers, establishing comparative efficacy for conventional and broadly accepted outcomes like overall survival (OS) or progression-free survival (PFS) may not be feasible for most randomized clinical trials (RCT) where allotted resources only allow for a few years of follow-up. Manufacturers, contract research organizations and trial investigators are therefore increasingly utilizing surrogate outcomes as the trials’ primary outcomes – many of which are not validated against target outcomes of interest like OS, PFS, or well-established health related quality of life (HRQoL) measures [Citation2]. Further, in many ongoing or planned early stage cancer clinical trials, the surrogate outcome may be the only primary outcome (or even the only efficacy outcome) [Citation1].
The advantage of surrogate outcomes is that the time to collect the required number of events to sufficiently power the trial is substantially shorter [Citation3], thus reducing both research and development (R&D) costs and the time needed to get a medication to patients (i.e. get regulatory approval and payer reimbursement). The disadvantage is that data cut offs from surrogate outcomes may often not be able to demonstrate long-term benefits such as OS or PFS [Citation4]. For example, it is estiamted that less than 20% of oncology therapeutics that receive regulatory approval based on surrogate outcome efficacy (e.g. from the FDA) later demonstrate long term benefit in confirmatory post-approval trials. [Citation5–7]. Surrogate outcomes may also lack generalizability across cancers or even specific patient populations within the same type of cancer. For example, while 12 months pathological complete response (pCR) has been shown to be a surrogate outcome for several longer term outcomes in breast cancer, the strength of surrogate association varies substantially across breast cancer subtypes [Citation8]. Consequently, HTA submissions based on primary surrogate outcomes are often unable to meet the rigor of HTA agencies’ evidence standards. Without substantial change of practice in handling surrogate outcomes, many early stage oncology HTA submissions over the next decade may be unsuccessful. An unfortunate consequence is that millions of early stage oncology patients will be left without access to potentially effective therapeutics.
The solution to this problem is not obvious as various stakeholders may not align on priorities. Industry is generally supportive of the widespread use of surrogate outcomes to attain faster approvals while regulatory agencies are predominantly concerned with balancing patient needs with demonstrated efficacy and safety. HTA agencies must consider uncertainty, long-term benefits, and the economic value of such benefits, and lastly, patient advocates are pushing for increased access to available therapeutics. To facilitate a productive and efficient debate leading to rapid meaningful action, it is imperative that all stakeholders become aware of what challenges with early stage clinical trials currently exist, identify which outcomes are used appropriately during the various stages of therapeutic development, and how market access processes can best view and value evidence from surrogate outcomes.
In this article, we provide an in-depth review and perspective of the current state of surrogate outcomes in early stage cancers, the limitations of approaches currently used to correlate surrogate outcomes to target outcome of interest like OS or PFS, as well as recent developments in the field of surrogate outcome validation. We address opportunities for decreasing uncertainty with real-world data, novel methodological frameworks, and incorporating the patient voice. We discuss how HTA agencies may seize the opportunity to update current guidelines on surrogate outcomes given how rapidly the field is evolving. Lastly, we address how HTA submissions may better involve clinicians and patients in an evaluation.
2. Surrogate outcomes – what they are and what they are not
2.1. Classic definition
The classic definition of a surrogate outcome goes back to the 1989 paper by Prentice [Citation9]:
‘the surrogate must be a correlate of the true clinical outcome and fully capture the net effect of treatment on the clinical outcome.’
Equally important, in their classic overview of surrogate outcomes Fleming and DeMets state that:3
‘a correlate does not a surrogate make.’
The reason for the latter is that for an outcome to be a valid surrogate outcome, it must necessarily be the dominant causal pathway from disease, through the treatment, to the ‘true’ clinical outcome. Note that throughout this article we will use the term target outcome of interest (or the shorter version, target outcome) rather than ‘true’ clinical outcome, or even the frequently used alternative ‘hard’ clinical outcome. This is to avoid misconceptions that a surrogate outcome necessarily represents an ‘untrue’ or a ‘soft’ clinical outcome.
In practice, the causality involved with the surrogate outcome pathway is often forgotten or ignored and reduced to a simple correlation coefficient. In reality, several causal scenarios may be plausible. As illustrated in , an outcome is a proper surrogate outcome if the treatment impacts the target outcome only by directly impacting the surrogate outcome, which in turn impacts the target outcome of interest. Both of these causal effects must be strong. However, as also illustrated in , several other potential causal pathways may be present to alter or confound the observed strength surrogacy. First, in addition to impacting the surrogate outcome, the treatment may also directly impact the target outcome of interest, thus rendering a weaker strength of surrogacy. Second, prognostic factors may confound the treatment’s impact on both the surrogate and target outcome. Third, particularly in cancer, the surrogate outcome may affect the decision to continue or terminate the treatment, thereby directly confounding its own strength [Citation10,Citation11]. For example, an earlier than expected observed pathological response may cause the oncologist to terminate the treatment (e.g. after six months compared to a full course of 12 months) in belief that the cancer has been resolved. However, if this surrogate outcome is not a highly accurate predictor of the target outcome, such clinical action may negatively impact the target outcome.
Figure 1. Depicts the general directed acyclic graph of causal pathways involved with surrogate outcome evaluation and validity. The solid lines are those directly involved with surrogate validity, whereas the dashed lines all represent potential confounding pathways. Surrogate- mediated treatment changes represent events where a surrogate outcome leads the clinician to alter an effective treatment (e.g. terminating treatment due to early pathological response).
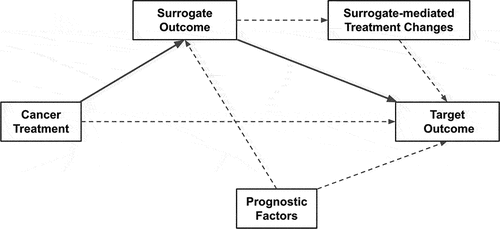
For oncology in particular, OS tends to be the target outcome of interest which a surrogate must both correlate to and capture the net effect of. In many cases, PFS has already also been validated to possess these properties, i.e. be a validated surrogate outcome. However, it should be noted that this is not true for all cancers.
2.2. Treatment-level and trial-level surrogacy
For an outcome to qualify as a surrogate outcome, the surrogacy must be validated with respect to both the ‘treatment-level’ and the ‘trial-level’ [Citation12]. illustrates how these two segments of validation are connected. Treatment-level validity should be validated for all treatments that are anticipated to affect the target outcome of interest via the surrogate pathway. If the surrogate pathway is not well-understood or defined, treatment-level surrogacy should be validated for all treatment arms (including control). For a surrogate outcome to be valid at the treatment-level, it must predict the target outcome within a treatment arm [Citation12]. For example, if 6-month pCR is the surrogate outcome and the target outcome of interest is 2-year overall survival, then across all patients in one treatment arm the majority of patients with 6-months pCR should also survive for at least 2 years. Similarly, the majority without 6-month pCR should not survive. For a surrogate outcome to be valid at the ‘trial-level,’ there should be clear evidence that a comparative effect on the surrogate outcome translates into a comparative effect on the target outcome of interest. For time-to-event outcomes, a comparatively longer time-to-event for the surrogate outcome should predict a comparatively longer time-to-event for the target outcome, and vice versa, for shorter time-to-events. Surrogate outcomes have generally been shown to overestimate the comparative treatment effect compared to the target outcomes of interest [Citation13]. The use of surrogacy threshold effects have therefore been recommended. That is, researchers should a priori establish the minimally important comparative effect on the target outcome (e.g. a hazard ratio of 0.8) that the surrogate outcome should be able to predict and how large the comparative surrogate effect must be to do so. Regulatory and HTA agencies will generally require that the surrogate outcome is associated with a larger comparative effect than the minimally important difference on the target outcome. This is simply because the surrogate outcome is invariably considered of less clinical importance. For example, in the recent ADAURA trial comparing adjuvant treatment with osimertinib versus placebo in resected epidermal growth factor receptor (EGFR) positive stage I-IIIa non-small cell lung cancer (NSCLC) the interim analysis on disease-free survival at 24 months yielded a highly positive comparative effect in favor of osimertinib (HR = 0.17, 99.06% Confidence Interval 0.11–0.26, p < 0.001), but overall survival data were immature with nine deaths in the osimertinib group and 20 in the placebo group [Citation14]. Based on these data, osimertinib received a conditional recommendation by CADTH with an 82% price reduction justified by the OS data being immature and an assessment that DFS could be used as a proxy for patients maintaining good quality of life for the same duration [Citation15]. Three years later, the mature (5-year) overall survival data was published, yielding a statistically significant benefit of adjuvant osimertinib therapy over placebo (HR = 0.49, 95.03% CI 0.33–0.73, p < 0.001) [Citation16].
3. The oncoming avalanche of early stage cancer trials using surrogate outcomes
An avalanche of early stage cancer clinical trials is coming. A recent whitepaper prepared by IQVIA and AstraZeneca systematically searched clinicaltrials.gov for early stage cancer clinical trials in the top 10 solid tumors (Lung, Breast, Prostate, Melanoma, Ovarian, Colorectal, Pancreatic, Esophageal, Gastric, Bladder) that were initiated between 2017–2022, not withdrawn, suspended, or terminated and identified a total of 387 trials [Citation1]. Among these, 70% of all trials had a single primary endpoint, of which 83% (225 out of 272) have a surrogate endpoint as their primary endpoint. The median enrollment of these trials was 77 participants (IQR: 36–328), 80% were open-label, and 37% had single-group assignment. Over 50 different surrogate outcomes were identified, none of which had previously been validated in early stage cancer populations. This review only covers a proportion of all early stage cancer trials as hematological cancers and less prevalent cancers were not included. As such, it seems reasonable to estimate that in the coming years, HTA agencies across the globe may collectively receive over 100 HTA submissions a year for early stage cancer therapeutics with evidence anchored in surrogate outcomes, of which many are not supported by good evidence to suggest they predict and correlate with a traditionally valued target outcome (e.g. PFS, OS).
4. The gap between regulatory and HTA agencies
4.1. Regulatory standards for assessing surrogate outcomes
There is a clear and well-recognized gap between regulatory and HTA agencies when clinical trials only use surrogate endpoints as their primary endpoints. Regulatory agencies such as the Food and Drug Administration (FDA) and the European Medicines Agency (EMA) are predominantly concerned with striking a balance between patient need and the demonstrated efficacy and safety of a novel intervention. In circumstances of high unmet patient need, regulatory agencies may grant conditional approvals, requiring manufacturers to produce more compelling evidence post-approval. For early stage cancers in particular, the evidence available prior to completion of post-approval studies is commonly limited to trials with surrogate outcomes supported by limited follow-up, insufficient number of events (statistical power), and often no comparator arm [Citation1]. Some early stage cancer trials may even focus solely on key safety outcomes as the efficacy is assumed from available later stage cancer clinical trials. While this strength of evidence may be acceptable for conditional regulatory approvals, post-approval studies often may not subsequently deliver the required strength of evidence. Reviews have found that less than 20% of post-approval studies demonstrate long-term benefit of the experimental therapeutic due to crossover and other study design issues [Citation5], and over half of post-approval studies solely continue to record and report data on the original surrogate outcome, albeit with longer follow-up, rather than a clinically more relevant target outcome of interest. Furthermore, a substantial proportion of planned or ongoing clinical trials are either terminated or results may not be publicly reported following conditional regulatory approvals.
4.2. HTA standards for assessing surrogate outcomes
For HTA agencies, novel therapeutics are evaluated on a broader set of criteria compared to the regulatory settings. Overall efficacy and safety are necessary but ‘strong’ evidence should be available to demonstrate long term benefits so that these may be implemented in pharmacoeconomic evaluations to calculate its cost-effectiveness. What constitutes ‘strong’ evidence is commonly rooted in traditional evidence-based medicine (EBM) where large well-designed randomized trials are considered to demonstrate a low risk of bias. In order for surrogate outcomes in HTA submissions to be considered valid and backed by strong evidence, the evidence standards for comparative effect estimates must equally apply to surrogacy correlation estimates (or other justified metrics of surrogacy). For example, the European network for Health Technology Assessment (EUnetHTA) recommends that surrogate outcome evidence can be considered strong (level 1) if it: ‘demonstrates that treatment effects on the surrogate endpoint correspond to effects on the patient-related clinical outcome (from clinical trials); comprises a meta-analysis of several RCTs and establishment of correlation between effects on the surrogate and clinical endpoint’ [Citation17,Citation18]. By contrast, moderate level evidence (level 2) is achieved ‘if evidence demonstrating a consistent association between surrogate endpoint and final patient related endpoint (from epidemiological/observational studies).’ A recent review of guidance documents from HTA agencies globally revealed a total of 29 HTA agencies (20 countries) providing methodological guidance that considered surrogate outcomes (26 European, 2 Australian, 1 Canadian) [Citation17]. For those agencies specifically addressing the strength of surrogacy evidence, all recommend a similar type of strength of evidence categorization (e.g. strong, moderate or low), where strong evidence requires individual patient data analysis from multiple RCTs or meta-analysis (i.e. meta-regression) for trial-level surrogacy. Under such criteria, the vast majority of oncoming early stage cancer clinical trials would be graded a low or moderate strength evidence and HTA agencies would be highly hesitant to issue a positive recommendation without conditions. In many instances, HTA agencies may view surrogate outcome strength of evidence low or insufficient and thereby issue recommendations that are conditional upon both a price reduction and stronger evidence being gathered. This has been the case in NICE and CADTH submissions for conventional surrogate outcomes like progression-free survival (PFS), and in a few cases, disease response (e.g. response rate) [Citation19].
Unfortunately, a low to moderate strength of evidence as judged by conventional EBM standards may result in HTA agencies issuing unfavorable funding recommendations and criteria, which often dissuade manufacturers from pursuing market approval in the given country. In the UK, the National Health Services’ Cancer Drug Fund was established to provide interim funding for a period up to two years for new cancer drugs targeting unmet needs when the clinical evidence was not yet sufficient to support conclusive cost-effectiveness analysis. For example, pembrolizumab plus chemotherapy with or without bevacizumab was recently (May 2023) approved to be funded under the Cancer Drug Fund for the treatment of recurrent or metastatic cervical cancer [Citation20]. The supporting clinical trials yielded suggestive but not convincing evidence of long-term benefits in prolonging time to progression. The Cancer Drug Fund provides interim funding recommendations to inform managed access agreements, which not only allows patients to access drugs where HTA bodies still require more evidence but also for post-market collection of real-world data or occasionally continuation of clinical trials to collect long term outcomes. In other countries, such post-conditional HTA approval data collection mechanisms have not been coordinated to the same extent. Uncertainty is often cited as the main reason for rejection, and a lack of general set of processes to collect data to supplement conditional approvals may often delay time to recommendations or even dissuade manufacturers from pursuing further local data collection [Citation19].
4.3. Opportunities for bridging the gap
In the scenario where regulatory agencies fail to enforce rigorous post-approval studies and where HTA agencies stay the course of requiring the strength of evidence be judged by conventional EBM standards and do not broadly adopt post-recommendation data collection, access to innovative interventions may be limited. A better understanding of how data can best be managed is highly needed.
There are three areas that represent immediate opportunities for drastically advancing the use and evaluation of surrogate outcome evidence. Firstly, the simplistic and singular requirement that a surrogate outcome only needs to correlate reasonably with some target outcome of interest only provides a limited view of the available data. Several other simple metrics like positive predictive value (PPV) and negative predictive value (NPV) (i.e. what percentage of ‘positive’ surrogate outcomes yield ‘positive’ target outcomes and similar for ‘negative’ outcomes) can readily be incorporated in any surrogacy evaluation [Citation21]. Further, albeit under somewhat complex statistical models like causal models and Bayesian hierarchical models, simple metrics like the percent contribution that the surrogate outcome has on the target outcome within the causal pathway can be extracted [Citation22,Citation23]. While none of these are conclusive on their own, they provide greater nuance with the available data and have the potential to reduce uncertainty without further data collection. Second, the vast advances in the size and quality of real-world data (particularly in the oncology space) that have occurred over the past decade now facilitates reliable validation of surrogate outcomes against long-term target outcomes of interest. The growing discipline of Target Trial Emulation (TTE) [Citation24], which was endorsed by NICE [Citation25], combined with a broadly better understanding of causal inference models, may to a large extent obviate the need for long-term clinical trials for surrogate outcome validation. For the purpose of obtaining comparative effectiveness estimates, TTEs have already been validated empirically against existing RCT results [Citation26]. They work by employing well-planned causal models to real-world data (typically very large databases) to emulate the protocol of a target trial. As such, they emulate the results of either a hypothetical or an actual completed trial. While these techniques have not yet been applied to surrogate outcome validation, it is likely that they will become necessary due to challenging and limited evidence-bases like the one currently seen in early stage cancer. Third and lastly, the patient’s voice is still relatively unheard in HTA submissions. Many patient-important outcomes may be overlooked both in clinical trial design or undervalued in HTA submissions. For the latter, the patient’s voice serves more as supplementary qualitative evidence to augment the strength of clinical trial evidence.
5. Oncology surrogate outcome validation part I – current practices and their gaps
5.1. Current statistical practices documented across the literature
Across the surrogate outcome validation literature, the most commonly used criterion for asserting surrogacy is a positive statistical correlation (R, Pearson correlation) of at least 0.7 [Citation27–29]. However, perusal of the surrogate oncology outcomes literature reveals that several other thresholds have been utilized and consistent guidance is missing. Most surrogate outcome evaluation frameworks and HTA guidelines stay away from firm recommendations about correlation thresholds, but those who do make firm recommendations, also frequently do not agree [Citation17,Citation30]. For example, the Biomarker-Surrogacy Evaluation Schema (BSES) recommends R2 >0.6 both at the trial-level and treatment-level to constitute ‘excellent’ surrogacy. Here, R2 represents the proportion of variation in target outcome (dependent variable) that can be attributed to the surrogate outcome (independent variable). In addition, BSES also requires that ‘the proportion of the total range of the surrogate that is equal or larger than the surrogate [minimally important] threshold effect’ exceeds 30%. To achieve ‘good’ level of surrogacy, BSES recommends R2 >0.4 and a proportion exceeding the surrogate threshold effect be at least 20%. By contrast, Institute of Quality and Efficiency in Health Care (IQWiG) in Germany a 2011 rapid report outlined that lower 95% confidence interval bounds of R > 0.85 would constitute high correlation, values between 0.7–0.85 moderate, and values below 0.7 low [Citation31]. A 2017 update to the IQWiG further suggested a minimum R2 >0.49 for trial level surrogacy to be considered valid [Citation32]. To potentially add to the confusion, it is also possible that non-statistician researchers dealing with surrogate outcomes may occasionally misinterpret R2 as (Pearson) correlation. Similarly, there are examples of other surrogate outcome validation studies where other correlation metrics like Spearman’s rank correlation coefficient or Kendall’s tau were preferred.
Validation of trial-level surrogacy is essential. The most common approach is the meta-analytic approach (due to data availability). Here, aggregate data estimates of comparative effects of the surrogate outcome and target outcome are modeled using meta-regression (typically univariate), which produces an R2 estimate [Citation12]. Arguably, the gold-standard approach is statistical modeling of individual patient-level data from multiple RCTs. For oncology surrogate outcome validations, the underlying statistical model is typically a Cox proportional hazards model with the target outcome as the dependent variable, but including the surrogate outcome as a predictor (independent variable). Analysis of individual patient level data also allows for controlling for other variables, thus aiding in reducing bias from prognostic factors on the surrogate effect. It has long been known that meta-regression is subject to bias from the ecological fallacy associated with regression analysis of aggregate data (rather than individual patient level data) [Citation12,Citation29,Citation33,Citation34]. depicts a conceptual example of how ecological fallacy imposes bias on the R2 estimate compared to analysis of individual patient level data. The ecological fallacy often biases toward the null, meaning that many surrogate effects may be missed due to bias. In a review of 15 surrogate validation analyses conducted by the FDA from 2014–2022, 10 analyses were performed on aggregate data and 5 with individual patient level data [Citation35]. Almost all correlation estimates (i.e. R) obtained from patient level analyses were moderate to high (0.6 yo 0.95), whereas correlations (R) from 6 of 10 aggregate data analyses were low (<0.5).
Figure 3. Illustration of ecological fallacy in aggregate data meta-analytic surrogate outcome validation versus individual patient level meta-analytic surrogate outcome.
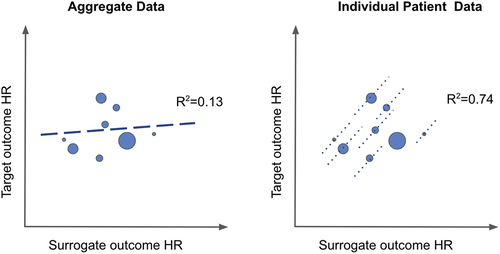
The target outcome in oncology surrogate outcome validation is always time-to-event, and the Cox proportional hazard model is almost ubiquitous in RCT analysis of time-to-event data. As such, trial-level surrogate outcome validation has traditionally also relied on the Cox proportional hazard model. Yet, in oncology, the underlying assumption of proportional hazards has been put into question recently as several RCT scenarios with non-proportional hazards have surfaced. Over the past decade, oncology RCTs have increasingly evaluated the efficacy of novel treatment classes. These treatment classes have vastly different clinical and biological properties than conventional chemotherapy. Immunotherapies, for example, frequently produce faster tumor response, but often have a time-lag in drug-induced adverse events, thus yielding progression and survival curves of vastly different shape than those commonly observed for standard-of-care chemotherapy [Citation10,Citation11]. Since all statistical models are based on assumptions, the extent to which these are violated the validity of the model suffers. As such, trial-level validity may suffer. We were only able to identify two articles in the literature where surrogate outcome metrics were obtained under the non-proportional hazards assumption [Citation21,Citation36]. However, both of these were empirical methods studies rather than individual applications. Their findings are discussed in the next section.
6. Oncology surrogate outcome validation part II – adapting to better practices
6.1. Beyond correlation
The statistical correlation between the surrogate outcome and the target outcome of interest is a one-dimensional and highly limited metric. Judging surrogacy by correlation alone is akin to judging the evidence of comparative effect between two treatments by the p-value alone. HTA agencies typically state uncertainty as their key reasons for issuing negative recommendations. With the availability of individual patient data from the manufacturer, much of the uncertainty surrounding a surrogate outcome can often be alleviated by employing additional, supplementary methods.
6.1.1. Positive and negative predictive values
At its very core, a surrogate outcome is valid at the treatment-level if 1) a large proportion of the patients with a positive surrogate outcome also have a positive target outcome; and conversely, 2) if a large proportion of patients with a negative surrogate outcome have a negative target outcome. The one-dimensional correlation estimate provides very limited insight into this property. Depending on the event rate of both surrogate and target outcomes in the data, a high correlation may easily be obtained from a surrogate outcome with poor predictive properties and vice versa.
The use of positive predictive values and negative predictive values are also valuable in understanding any potential difference in strength and behaviors of surrogacy across treatments [Citation21]. In oncology, treatments targeting different pathways tend to elicit different surrogate behaviors. For example, with conventional chemotherapy, treatment-related toxicity typically occurs almost immediately, and thus, lack of improvement is a good surrogate indicator for lack of long-term benefit. By contrast, with immunotherapies where toxicities often occur with a lag, many patients may respond well to treatment without this conferring any comparative long-term benefits [Citation11].
Positive predictive values and negative predictive values can similarly be utilized to understand the strength of surrogacy at the trial-level (i.e. with respect to the comparative effect). Steward et al. demonstrate the behavior of positive predictive values and negative predictive values both on the treatment-level and trial level by retrospectively analyzing 139 oncology clinical trials published in the New England Journal of Medicine between 2012 to 2017. At the treatment-level, for example, different cut-points of median PFS (1.5, 2, 3, 4, and 5 months) are used to predict OS at 2, 3, 4, 5, and 6 months [Citation21]. By contrast, across multiple trials different hazard ratio (HR) cut-points for PFS (e.g. 0.5, 0.6, 0.7, and 0.8) are used to predict whether the HR for OS is larger or smaller than 0.8. This approach can readily be utilized to establish or evaluate minimal surrogacy thresholds recommended in several guidelines.
6.1.2. Percent attributable causality
RCTs are the most accepted way of truly establishing causality between treatment and efficacy. This is because all prognostic factors (known and unknown) are equally distributed and randomized across treatment groups. However, with surrogate outcome validation, all prognostic factors (known and unknown) may not be equally distributed across those patients experiencing a positive versus negative surrogate outcome. As such, some statistical model to adjust for suspected confounders is recommended. Because surrogacy operates on causal pathways (see ), the ideal statistical approach is a causal model [Citation22]. A further advantage of this approach is that each causal pathway contributes to the target outcome of interest, and therefore the percentage contribution of the surrogate outcome can be estimated and provide an intuitive metric for surrogate validity. The percent attributable causality can be approximated and gauged by comparing standard errors from multiple causal models for the surrogate effect, with and without direct effect (i.e. treatment directly to target outcome) and confounding effects (see causal diagram breakdown in ). The disadvantage of this approach is that causal models can be complex, resource intensive to implement, and often rely on many assumptions. Perhaps for this reason, the applications of causal models for surrogate outcome validation have been sparse to date.
6.2. Beyond proportional hazards
In oncology, where the target outcome of interest is typically time-to-event, the data must be analyzed accordingly. To date, surrogate outcome validation with time-to-event data has invariably been modeled using the Cox proportional hazards model. However, in oncology where novel treatments target different pathways, the behavior of patients responding to treatment over time is likely to vary across different treatment classes [Citation10]. Therefore, many examples of non-proportional hazards have been observed in recent randomized clinical trials of oncology therapeutics, and this trend is likely to increase.
A proportional hazards model is an inaccurate model when comparative hazards from time-to-event data are not proportional. Modeling the data incorrectly also leads to biased estimates of surrogacy validation metrics (typically statistical correlation). Pang et al. explored the correlation between PFS and OS in 14 randomized clinical trials, using both the proportional hazards model as well as non-proportional hazards models [Citation36]. Using restricted mean survival time (mean life expectancy), they showed an average correlation estimate of 0.5 under the proportional hazards model (comparative effect measure: hazard ratio) compared to an average correlation estimate of approximately 0.35 under the non-proportional hazard model (comparative effect measure: life expectancy ratio). This is a substantial bias introduced solely by the choice of statistical model. Thus, it may be reasonable to question the validity of many surrogate outcomes previously validated under the Cox proportional hazards model – particularly where the two interventions being compared were from different drug classes. For example, one of the studies analyzed by Pang et al. (albeit not a surrogate outcome study) looked at both PFS and OS associated with Durvalumab after chemoradiotherapy in Stage 3 non-small cell lung cancer [Citation37,Citation38]. While the relative benefit on PFS was approximately 50% both under a proportional hazards model and a non-proportional hazards model, the relative benefits of Durvalumab on OS was approximately 33% under the proportional hazards model and only 13% under the non-proportional hazards model.
6.3. Sharing of individual patient-level data
It is well known that aggregation of individual patient level data from multiple large well-designed RCTs is the gold standard for surrogate outcome validation. This requires trial investigators and sponsors to share the data, a practice which has been advocated for decades for several purposes beyond (and including) surrogate outcome validation, but has seen limited uptake. Trial data sharing comes with further complexities when commercial interests are involved, and simple academic encouragement in journal publications has repeatedly been proved futile. In the context of HTA, at a minimum, guidance on use of surrogate outcomes need to include firm recommendations that individual patient level data analyses either be conducted by the manufacturer or that the data are made available for analysis by the HTA agency itself or affiliated academic institutions. For early stage cancers, a call to HTA agencies for incentivizing data sharing may have its limitations since one of the key challenges with early stage cancer RCT evidence is that the sample sizes and follow-up durations are not available to support surrogacy validation at the level of evidence typically required by HTA agencies. However, within the next 5 to 10 years as more early stage cancer trials complete, the picture may change.
One example of a successful application of surrogate outcome validation using a large individual patient data set from several international RCTs is presented in . Due to substantial heterogeneity in pCR across breast cancer subtypes, it had previously been challenging to demonstrate surrogate association to PFS and OS using aggregate data meta-analysis [Citation8]. However, the availability of a large individual patient data set allowed accurate modeling by cancer subtype. For early stage cancers, similar stories may likely unfold because several RCTs include a smaller subgroup of early stage cancer patients which do not provide sufficient sample sizes on their own, but may likely do so combined.
Table 1. Presents three distinct examples of limitations associated with conventional surrogate outcome methodology, practice, and interpretation, as well as proposed solutions.
6.4. Beyond OS and PFS
In early stage cancers, waiting for survival or progression to be observed is often not feasible. Not only do these outcomes take a long time to observe, but several ‘interfering’ events may occur during the first years of treatment which makes it close to impossible to validate the surrogate outcome in relation to the intervention [Citation39]. For example, novel immunotherapies are often associated with a time lag in safety risks. Since approximately one in five cancer patients experience serious adverse events, the treatment course may be altered accordingly in a substantial proportion of patients, often resulting in mixed and unpredictable treatment-switching patterns. For one, terminating a therapy due to a drug-induced adverse event impedes the ability to record the time of progression unless that adverse event is resolved and the patient can resume therapy. Even if the event is eventually resolved and the treatment is resumed, several clinical trials truncate the data from such patients. Second, adverse events may cause trial physicians to alter the therapy (if allowed in the trial protocol). For example, when a novel therapy is given in combination with another therapy such as chemotherapy, the latter may be terminated while the novel therapy is continued. While serious drug-induced adverse events leading to treatment discontinuation or treatment switching are not good surrogates for PFS, they do provide insights into the potential biases. Thus, for HTA evaluations they should be considered as an important complementary source of evidence in the evaluation of both comparative efficacy and comparative safety. In the recent whitepaper summarized earlier, roughly 10% of the primary ‘surrogate endpoints’ were conventional adverse events [Citation1].
When OS or PFS data are either not available or only supported by a limited number of events, it becomes highly important to evaluate outcomes that are important to the patient. The field of patient reported outcomes and quality of life is currently subjected to notable developments [Citation40], and it is estimated that close to 50% of recent trials (including oncology trials) include PROs [Citation41]. Although quality of life (QoL) and patient reported outcome (PRO) scales have been criticized for failing to capture the individual patient’s voice, they provide a well-recognized framework for quantitatively studying comparative efficacy on patient important domains in RCTs. In the absence of OS and PFS, evidence on QoL becomes highly relevant to HTA submissions for the purpose of cost-effectiveness evaluations. In early stage cancers, a disproportionately low number of trials appear to include PROs. In the previously mentioned white paper, of 70% of early stage cancer trials that used surrogate outcomes, only one used QoL as their surrogate efficacy outcome, which was also the only primary outcome [Citation1]. Given the increasing uptake of PROs in RCTs, it stands to reason that the conventional practical reasons for excluding PROs from clinical trials (burden on patients being interviewed, added resource requirements, etc.) do not apply well to early stage cancer trials, particularly due to the enhanced need for non-OS/non-PFS data. Perhaps one of the reasons are that examples of surrogate outcome validation studies for PROs and PFS/OS are still relatively rare [Citation4,Citation42].
7. Reducing uncertainty - real world data opportunities
While focusing on increasing use of shorter-term patient important outcomes, challenges and skepticism still remain. Further, the majority of planned and ongoing early stage cancer trials do not include such outcomes, and therefore the avalanche of non-conventional surrogate outcomes that will reach regulatory and HTA agencies is inevitable. Facilitating post-approval and post-recommendation studies and data collection with emphasis on patient important outcomes may be part of the solution, but a better understanding of what many of these outcomes mean to patients and society is still needed. Additional sources of evidence will be required to reduce the uncertainty surrounding long-term benefits of the many utilized surrogate outcomes that face evaluation in the near future.
The two best options are proactive planning of long-term clinical trials as well as managed care access data collection. However, both may be absent or substantially delayed in many instances. Delays are likely as the number of patients that can feasibly be enrolled in a clinical trial may be limited, and the number of patients that will receive treatment after funding approval will be limited to the country where a decision was made to reimburse it. Therefore, turning to the many sources of oncology real-world evidence that have emerged over the past decade may facilitate a substantial reduction of uncertainty. Retrospective analysis of real-world data should be feasible for most outcomes and can aid in validating treatment-level surrogacy for the control arm. This serves as a good complement to treatment-level surrogacy validation of the experimental treatment which comes out of data collection from managed care access programs or phase IV single arm studies. In scenarios where the experimental intervention belongs to a drug class where other therapeutics of the same class already exist in the market, real-world evidence can similarly be used to validate treatment-level surrogacy for the experimental intervention arm, as long as the assumption of a drug class effect is acceptable. For example, a large-scale real world data study using electronic health record data from 2015 to 2017 found moderate evidence for treatment-level surrogacy of PFS for OS (R = 0.75) for patients receiving PD-(L)1 inhibitors for the treatment of advanced non-small cell lung cancer [Citation43]. This study included a total of 5,257 patients that all had at least 6 months of follow-up. It also found no compelling evidence for time-to-progression as a surrogate outcome for OS (R = 0.6). However, given that both of these correlation estimates fell close to the conventional 0.7 threshold and that the data was not randomized, other measures like the positive predictive value and negative predictive value could have been useful to cast further light on the performance of the candidate surrogate outcomes (see also ).
For trial-level surrogacy, no real-world data analysis can replace well-designed RCT. However, for early stage cancer where the latter is typically not an option, recent popularized methods like Target Trial Emulation (TTE) could well find their application in surrogate outcome validation [Citation24]. TTE is a systematic application and framework for emulating the results of a ‘target’ randomized clinical trial using a suitable observational data set combined with causal inference models. TTEs have both been applied to large and moderate sized data sets with success, and studies have generally found that thorough application of TTE yields consistent results with RCTs when estimating comparative efficacy [Citation26]. Because surrogate outcome validation must take into account the surrogate pathway (and other pathways), analysis of observational data (real-world data) should incorporate all such causal pathways, i.e. perform mediation analysis. The literature on such analyses is limited, particularly for cancer. It is further worth noting that new causal models need to be developed for each surrogate outcome validation scenario (e.g. for each population, sub-population, treatment class, and outcomes), and that strong model assumptions are often required. For example, previous examples of surrogate outcome validation models applied to clinical trial data or meta-analytic data have utilized the principal stratification framework, under which researchers have had to rely on the monotonicity assumption, i.e. that is impossible for a patient in the treatment group to do better in the control group [Citation44,Citation45]. The need for unique but similar models potentiates a future scenario that is analogous to the many Markov models for cost-effectiveness analyses that have been constructed over the past two decades in order to map long term cost and quality of life under several country-specific populations and patient population settings as novel drugs continually came to market.
8. The importance of the patient voice
Decisions to fund new therapeutics are rooted in average population effects (and cost-effectiveness). This is despite the fact that patient preferences may vary substantially based on experiences, hopes, and expectations. For example, cancer-related loss of fertility and libido typically matter to younger individuals, whereas it may not be of importance to older individuals. Conversely, the high risk of serious infections caused by immunotherapies are likely more of a concern to older individuals with already weakened immune systems, whereas younger individuals, despite undergoing cancer treatment, may be naturally more able to fight off infections.
While many HTA agencies now include the perspectives of a few patients in the final HTA report, these experiences are often read by a member of the decision-making committee, not an actual patient, which means the authentic patient voice is not at the table. This also means that patients often do not learn how decisions are made and what information is most pertinent to the decision-makers. To the best of our knowledge, individual patient demographic-dependent preferences are never considered in the decision making. Since funding decisions are heavily correlated with the incremental cost-effectiveness ratios (i.e. cost per quality-adjusted life year) produced in the pharmacoeconomics’ report, this practice seems suboptimal since the patient preference is also highly correlated with the quality of life that is derived from the new therapeutic.
9. Discussion
In this perspective article, we have outlined how the current practices for evaluating evidence of surrogate outcomes in the context of HTA fall short methodologically and inferentially and thus may often leave reviewers with highly uncertain or even biased evidence. We have outlined how such shortcomings will particularly be problematic in HTA of early stage cancer therapeutics as this area faces an oncoming avalanche of clinical trials relying on non-conventional surrogate outcomes. Lastly, we have outlined a number of practices and methods that can readily complement current practices and thereby reduce both uncertainty and bias in the evaluation of evidence based on surrogate outcomes.
10. Expert opinion
Surrogate outcomes offer a solution to an unavoidable and challenging scenario. As this article focused on HTA evaluations of surrogate outcomes, we have refrained from discussing the practice of surrogate outcomes in clinical trial design and planning. The truth is that surrogate outcomes serve as the middle station at a point in the R&D process where the evidence-base is not yet sufficient for making strong recommendations or decisions. The reason surrogate outcomes are endorsed or allowed in many clinical trials is because of the recognition that therapeutic R&D is a multi-year long process and that patients should be given an option to access novel treatments as soon as it is possible in this process. Ironically, the degree of evidence required to validate a surrogate outcome is often greater than that required to demonstrate comparative efficacy on the target outcome of interest.
In our humble opinion, the field of surrogate outcomes is ready for changes. First, conventional surrogate outcome validation represents a fraught and sub-optimal framework, particularly when emphasis is on a single primary surrogate outcome. With the absence or sparsity of OS or PFS data from RCTs in early stage cancer, HTA evaluations would fare better from considering all available outcomes recorded. While traditional evidence based medicine frameworks like GRADE allow reviewers to assess the importance of outcomes [Citation46], in practice, their unfortunate consequence is that all outcomes that are not (subjectively) rated as important tend to be ignored or heavily down weighted. A more structured framework involving systematic input both from clinicians and patients that have some degree of familiarity with the HTA processes is required. In some cases, there may be a strong rationale for identifying a good surrogate outcome for PFS or OS. Often this is because a valid health economic evaluation is required and cost-effectiveness cannot be established without some extrapolation of shorter term surrogate outcomes to longer term PFS or OS. To the extent that there is a strong rationale for identifying such an outcome, proactive efforts should be taken to identify which of the surrogate outcomes available in current and future clinical trials are the best candidates, and subsequently undertake efforts to validate these. For example, in newly diagnosed multiple myeloma, minimal residual disease (MRD) has been evaluated on the treatment-level and validated as a strong surrogate outcome for PFS and OS [Citation47]. This was validated meta-analytically showing highly statistically significant associations between negative MRD and both PFS and OS.
While the example of MRD is encouraging, it also represents an uncommon scenario for early stage cancers. The majority of ongoing early stage cancer trials that utilize surrogate outcomes make use of non-conventional surrogate outcomes. Thus, evidence from clinical trials will not be available for meta-analytic validation. In such scenarios, the best answer may lie either in the proactive analysis of existing real-world data or in the prospective collection of real-world data. The latter relies on a functional data collection infrastructure tied to managed access care programs, but such infrastructure is not yet developed for the majority of countries engaging in HTA evaluations. For example, in many countries, data across regions, provinces, or states are not well integrated. Rather, manufacturers in particular could proactively engage in the retrospective analysis of real-world data to support the limited surrogate outcome data which will be available from ongoing clinical trials over the next few years. In this context, it is important to note that complex outcomes are rarely captured in real-world data. Tumor response based on the RECIST criteria, for example, often have to be approximated from the available electronic medical record data. Similarly, an outcome like MRD is complex and not widely available from most real-world data sources. As such, proactive surrogate outcome validation using real-world data is more likely to succeed when the candidate surrogate outcome is not complex nor resource intensive to collect. Uncommon biomarkers or genetic biomarkers are therefore often poor candidates for real-world data surrogacy validation. Similarly, outcomes requiring thorough clinician adjudication are unlikely to be available from most real-world data sources. Quality of life and patient reported outcomes unfortunately face the same fate as these require a set of resource intensive and regulatory compliant patient interviews.
Throughout this perspective article, we have repeatedly outlined the gap that exists between regulatory and HTA agencies. Adequacy of choice of surrogate outcomes for RCTs of novel early stage cancer therapeutics is beyond the scope of this article. As is the adequacy of the RCT design and the accompanying statistical analyses for regulatory purposes. Clinical development at phase II and III faces several regulatory challenges. Given the financial incentives to optimize for regulatory approval, and the relatively smaller incentive to optimize for positive HTA recommendations, it seems unrealistic to expect or require that trials at these phases also be designed with potential HTA submissions in mind. For example, in the previously mentioned example of osimertinib for unresectable EGFR positive NSCLC, an additional 3-year interim analysis would likely have provided sufficiently compelling OS data combined with the overwhelming evidence for DFS. However, such an interim analysis would simultaneously put the trial at risk of having to stop the trial early for benefit, which would have left the strength of evidence at moderate for the primary outcome of OS. There are nonetheless potential solutions to overcome this. For example, unblinded interim data reads could be made available from sponsors only to HTA stakeholders, or additional interim analyses could be planned without allowing the possibility of stopping early for benefit (see also for discussion) [Citation48]. In early stage cancer the implications on HTA recommendations from use of non-conventional surrogate outcomes appear to be more pronounced than what is generally the case for later stage cancers or other disease areas. Ensuring access to novel therapeutics that may be the only hope of rapidly progressing cancer patients is essential in this setting, but is also highly challenging from a HTA perspective if the evidence-base is plagued by uncertainty. We therefore encourage relevant stakeholders such as regulatory agencies, manufacturers, patient advocates, and trial investigators to think beyond what the chosen primary surrogate outcomes means for sample size implications and probability of reaching statistical significance. By the same token, we strongly encourage stakeholders on the HTA side to consider as many approaches as possible to make the best of the available evidence, considering evidence from multiple efficacy outcomes, multiple approaches from measuring strength of surrogacy, and lastly, proactively engaging in real-world data collection and surrogate outcome validation analyses to the furthest extent possible.
Declaration of interest
The authors have no relevant affiliations or financial involvement with any organization or entity with a financial interest in or financial conflict with the subject matter or materials discussed in the manuscript. This includes employment, consultancies, honoraria, stock ownership or options, expert testimony, grants or patents received or pending, or royalties.
Reviewer disclosures
Peer reviewers on this manuscript have no relevant financial or other relationships to disclose.
Additional information
Funding
References
- Championing oncology relevant endpoints (CORE) in Canada: surrogate endpoints in clinical trials and reimbursement decisions for early-stage cancers. Whitepaper. 2023. Available from: https://www.iqvia.com/-/media/iqvia/pdfs/canada/white-paper/championing-oncology-relevant-endpoints-in-canada-en.pdf
- Bruce CS, Brhlikova P, Heath J, et al. The use of validated and nonvalidated surrogate endpoints in two European Medicines Agency expedited approval pathways: a cross-sectional study of products authorised 2011-2018. PLOS Med. 2019 Sep 10;16(9):e1002873.
- Fleming TR, DeMets DL. Surrogate end points in clinical trials: are we being misled? Ann Intern Med. 1996 Oct 01;125(7):605–613. doi: 10.7326/0003-4819-125-7-199610010-00011
- Gyawali B, Hey SP, Kesselheim AS. Evaluating the evidence behind the surrogate measures included in the FDA’s table of surrogate endpoints as supporting approval of cancer drugs. EClinicalMedicine. 2020 Apr;21:100332. doi: 10.1016/j.eclinm.2020.100332
- Gyawali B, Hey SP, Kesselheim AS. Assessment of the clinical benefit of cancer drugs receiving accelerated approval. JAMA Intern Med. 2019 Jul 1;179(7):906–913. doi: 10.1001/jamainternmed.2019.0462
- Hey SP, Gyawali B, D’Andrea E, et al. A systematic review and meta-analysis of bevacizumab in first-line metastatic breast cancer: lessons for research and regulatory enterprises. J Natl Cancer Inst. 2020 Apr 1;112(4):335–342. doi: 10.1093/jnci/djz211
- Schnog JB, Samson MJ, Gans ROB, et al. An urgent call to raise the bar in oncology. Br J Cancer. 2021 Nov;125(11):1477–1485. doi: 10.1038/s41416-021-01495-7
- Cortazar P, Zhang L, Untch M, et al. Pathological complete response and long-term clinical benefit in breast cancer: the CTNeoBC pooled analysis. Lancet. 2014 Jul 12;384(9938):164–172. doi: 10.1016/S0140-6736(13)62422-8
- Prentice RL. Surrogate endpoints in clinical trials: definition and operational criteria. Stat Med. 1989 Apr;8(4):431–440. doi: 10.1002/sim.4780080407
- Buyse M, Burzykowski T, Saad ED. The search for surrogate endpoints for immunotherapy trials. Ann Transl Med. 2018 Jun;6(11):231. doi: 10.21037/atm.2018.05.16
- Hamada T, Kosumi K, Nakai Y, et al. Surrogate study endpoints in the era of cancer immunotherapy. Ann Transl Med. 2018 Nov;6(Suppl 1):S27. doi: 10.21037/atm.2018.09.31
- Buyse M, Saad ED, Burzykowski T, et al. Surrogacy beyond prognosis: the importance of “trial-level” surrogacy. Oncology. 2022 Apr 5;27(4):266–271. doi: 10.1093/oncolo/oyac006
- Ciani O, Buyse M, Garside R, et al. Comparison of treatment effect sizes associated with surrogate and final patient relevant outcomes in randomised controlled trials: meta-epidemiological study. BMJ. 2013 Jan 29;346(jan29 1):f457. doi: 10.1136/bmj.f457
- Wu YL, Tsuboi M, He J, et al. Osimertinib in resected EGFR-Mutated non-small cell lung cancer. N Engl J Med. 2020 Oct 29;383(18):1711–1723. doi: 10.1056/NEJMoa2027071
- CADTH Reimbursement Recommendations. Osimertinib. Ottawa, Ontario. 2020.
- Tsuboi M, Herbst RS, John T, et al. Overall survival with osimertinib in resected EGFR-Mutated NSCLC. N Engl J Med. 2023 Jul 13;389(2):137–147. doi: 10.1056/NEJMoa2304594
- Grigore B, Ciani O, Dams F, et al. Surrogate endpoints in health technology assessment: an international review of methodological guidelines. PharmacoEconomics. 2020 Oct;38(10):1055–1070. doi: 10.1007/s40273-020-00935-1
- Pavlovic M, Teljeur C, Wieseler B, et al. Endpoints used for relative effectiveness assessment clinical endpoints amended JA1 guideline final. Int J Technol Assess Health Care. 2014. Nov;30(5):508–13. doi: 10.1017/S0266462314000592
- Pinto A, Naci H, Neez E, et al. Association between the use of surrogate measures in pivotal trials and health technology assessment decisions: a retrospective analysis of NICE and CADTH reviews of cancer drugs. Value Health. 2020 Mar;23(3):319–327.
- NICE. Pembrolizumab plus chemotherapy with or without bevacizumab for persistent, recurrent or metastatic cervical cancer. 2023.
- Stewart DJ, Bossé D, Goss G, et al. A novel, more reliable approach to use of progression-free survival as a predictor of gain in overall survival: the Ottawa PFS predictive model. Crit Rev Oncol Hematol. 2020 Apr;148:102896.
- Joffe MM, Greene T. Related causal frameworks for surrogate outcomes. Biometrics. 2009 Jun;65(2):530–538. doi: 10.1111/j.1541-0420.2008.01106.x
- Vandenberghe S, Duchateau L, Slaets L, et al. Surrogate marker analysis in cancer clinical trials through time-to-event mediation techniques. Stat Methods Med Res. 2018 Nov;27(11):3367–3385. doi: 10.1177/0962280217702179
- Zuo H, Yu L, Campbell SM, et al. The implementation of target trial emulation for causal inference: a scoping review. J Clin Epidemiol. 2023 Aug 9;162:29–37.
- NICE. NICE real-world evidence framework. [cited 2023 Jul]. Available from: https://www.nice.org.uk/corporate/ecd9/resources/nice-realworld-evidence-framework-pdf-1124020816837
- Wang SV, Schneeweiss S, Franklin JM, et al. Emulation of randomized clinical trials with nonrandomized database analyses: results of 32 clinical trials. JAMA. 2023 Apr 25;329(16):1376–1385. doi: 10.1001/jama.2023.4221
- Ciani O, Grigore B, Blommestein H, et al. Validity of surrogate endpoints and their impact on coverage recommendations: a retrospective analysis across International Health Technology Assessment Agencies. Med Decis Making. 2021 May;41(4):439–452. doi: 10.1177/0272989X21994553
- Kim C, Prasad V. Strength of validation for surrogate end points used in the US Food and Drug Administration’s approval of oncology drugs. Mayo Clin Proc. 2016 May 10;91(6):713–725.
- Buyse M. Use of meta-analysis for the validation of surrogate endpoints and biomarkers in cancer trials. Cancer J. 2009;15(5):421–425. doi: 10.1097/PPO.0b013e3181b9c602
- Ciani O, Davis S, Tappenden P, et al. Validation of surrogate endpoints in advanced solid tumors: systematic review of statistical methods, results, and implications for policy makers. Int J Technol Assess Health Care. 2014 Jul;30(3):312–324. doi: 10.1017/S0266462314000300
- Gesundheitswesen(IQWiG). IfrQuWi. Aussagekraft von surrogatendpunkten in der onkologie.[Validity of surrogate parameters in oncology. 2011. (Rapid report).IQWiG-Berichte 80.
- Institute for Quality and Efficiency in Health Care (IQWiG). Allgemeine Methoden Version 5.0. Version. 2017.
- Prasad V, Kim C, Burotto M, et al. The strength of association between surrogate end points and survival in oncology: a systematic review of trial-level meta-analyses. JAMA Intern Med. 2015 Aug;175(8):1389–1398. doi: 10.1001/jamainternmed.2015.2829
- Ciani O, Buyse M, Garside R, et al. Meta-analyses of randomized controlled trials show suboptimal validity of surrogate outcomes for overall survival in advanced colorectal cancer. J Clin Epidemiol. 2015 Jul;68(7):833–842.
- Walia A, Haslam A, Prasad V. FDA validation of surrogate endpoints in oncology: 2005-2022. J Cancer Policy. 2022 Dec;34:100364. doi: 10.1016/j.jcpo.2022.100364
- Pang H, Yang G, Ho JC, et al. Assessing surrogacy using restricted mean survival time ratio for overall survival in non-small cell lung cancer immunotherapy studies. Chin Clin Oncol. 2022 Feb;11(1):7. doi: 10.21037/cco-21-110
- Antonia SJ, Villegas A, Daniel D, et al. Durvalumab after Chemoradiotherapy in stage III non-small-cell lung cancer. N Engl J Med. 2017 Nov 16;377(20):1919–1929. doi: 10.1056/NEJMoa1709937
- Antonia SJ, Villegas A, Daniel D, et al. Overall survival with Durvalumab after Chemoradiotherapy in stage III NSCLC. N Engl J Med. 2018 Dec 13;379(24):2342–2350. doi: 10.1056/NEJMoa1809697
- Hashim M, Pfeiffer BM, Bartsch R, et al. Do surrogate endpoints better correlate with overall survival in studies that did not allow for crossover or reported balanced postprogression treatments? An application in advanced non-small cell lung cancer. Value Health. 2018 Jan;21(1):9–17. doi: 10.1016/j.jval.2017.07.011
- Meadows KA, Reaney M. Bringing the patient’s perspectives forward in drug development and health-care evaluation. Expert Rev Pharmacoecon Outcomes Res. 2023 Mar;23(3):267–271. doi: 10.1080/14737167.2023.2166492
- Kim Y, Gilbert MR, Armstrong TS, et al. Clinical outcome assessment trends in clinical trials-contrasting oncology and non-oncology trials. Cancer Med. 2023 Aug;12(16):16945–16957. doi: 10.1002/cam4.6325
- Hudgens S, Forsythe A, Kontoudis I, et al. Evaluation of quality of life at progression in patients with soft tissue sarcoma. Sarcoma. 2017;2017:2372135. doi: 10.1155/2017/2372135
- Khozin S, Miksad RA, Adami J, et al. Real-world progression, treatment, and survival outcomes during rapid adoption of immunotherapy for advanced non-small cell lung cancer. Cancer. 2019 Nov 15;125(22):4019–4032. doi: 10.1002/cncr.32383
- Tanaka S, Matsuyama Y, Ohashi Y. Validation of surrogate endpoints in cancer clinical trials via principal stratification with an application to a prostate cancer trial. Stat Med. 2017 Aug 30;36(19):2963–2977.
- Li Y, Taylor JM, Elliot MR, et al. Causal assessment of surrogacy in a meta-analysis of colorectal cancer trials. Biostatistics. 2011;12(3):15. doi: 10.1093/biostatistics/kxq082
- Guyatt G, Oxman AD, Akl EA, et al. GRADE guidelines: 1. Introduction-GRADE evidence profiles and summary of findings tables. J Clin Epidemiol. 2011 Apr;64(4):383–394.
- Munshi NC, Avet-Loiseau H, Anderson KC, et al. A large meta-analysis establishes the role of MRD negativity in long-term survival outcomes in patients with multiple myeloma. Blood Adv. 2020 Dec 08;4(23):5988–5999. doi: 10.1182/bloodadvances.2020002827
- Freidlin B, Korde LA, Korn EL. Timing and reporting of secondary overall survival end points for phase III trials in advanced/metastatic disease. J Clin Oncol. 2023 Oct 10;41(29):4616–4620.