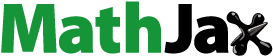
ABSTRACT
In this work, an analytical study of type-2 fuzzy fractional-order PD-PI (T2FFOPDPI) controller in a microgrid system (MGS) has been carried out for frequency regulation. The MGS contains a combination of renewable generations, different storage and electric vehicles. The controller parameters are optimised by enhanced Harris hawks optimisation (EHHO) technique. The efficiency of EHHO method is compared with HHO and other optimization techniques for the PID controller. The MGS analysis is executed under increased load, decreased renewable powers, unavailability of renewable sources, and reduction in system parameters. The performance of the suggested EHHO-based T2FFOPDPI method is examined by fuzzy PID (FPID), T2FPID and a recent approach. Finally, the competency of the suggested method is investigated through OPAL-RT real-time simulator.
1. Introduction
The indecisive character of solar, load demand and wind sources makes recent micro-grids (MGs) more intricate and establishes the frequency variation. In modern power systems (MPSs), renewable energy sources (RESs) are a more attractive choice due to the environmental issues, rising power demand, and growing expenses of electricity. Nevertheless, RESs like solar and wind exhibit immensely random behaviour. Also, the disordered nature of the demand initiates deviation in the frequency. While the MGs are working in isolated form, it is a hard job to preserve the steady frequency in the existence of irregular RESs and normal load variations. The normally recognised answer for this problem in isolated MGs is using the support systems like energy storage systems (EESs) and microturbines. Energy-storing devices, for example, flywheel energy storage systems (FESs), ultra-capacitors (UC) and battery energy storage systems (BESSs) stock the surplus power from the RESs over the demanded load and supply the energy to the grid when the demand is higher than the generation (Pan and Das Citation2015, Citation2016b; Hasanien Citation2018; Khooban et al. Citation2018a, Citation2018b; Soliman et al. Citation2019).
To manage frequency control of MGs for appropriate control, different approaches are demonstrated by various researchers. In Ray, Mohanty, and Kishor (Citation2010), the author studied a hybrid distribution system for quick load deviation. Also, they have examined wind turbine generators with the variation of wind speed and photovoltaic structure with a variation of temperature and irradiance. The author employed UC as a substitute energy storage device and included hybrid systems for better performance. In Shankar and Mukherjee (Citation2016), the author examined a hybrid power system model including different generating units and EESs employing a quasi-oppositional harmony search algorithm. The author engaged PI and PID controllers, and the results are compared. In Elsisi and Soliman (Citation2020), the – future search algorithm (FSA)-based PID for an automatic voltage regulator has been proposed. In Elsisi (Citation2020), the author proposed a novel variable structure gain scheduling to the frequency stability problem.
A fuzzy logic controller (FLC) is competent in managing uncertainties. FLC assisted with the optimisation method enhances the dynamic performance of the system. Later, diverse FLC-based controllers, i.e. hPSO-PS (Sahu, Panda, and Sekhar Citation2015) and ICA-tuned Fuzzy PID with derivative filter (FPIDN)/fuzzy PIDN-FOI (Arya Citation2018) have been suggested. Khooban et al. Citation2018a studied multi-objective improved black hole algorithm to optimise FOFPD + I for MGs. In Khooban et al. (Citation2017), the authors have suggested optimal general type-2 fuzzy PI (OGT2FPI)-based modified harmony search algorithm in MGs with electrical vehicles (EVs). In Khooban et al. (Citation2018a, Citation2018b), the authors have studied the multi-objective FOFPID method for MGs with EVs. In Gheisarnejad and Khooban (Citation2019), the authors have analysed enhanced JAYA-based various controller structures in the multi-MGs. Recently Patel, Mohanty, and Hasanien (Citation2020) examined competition over resources (COR)-based FTIDF controller for frequency stabilisation of hybrid MGs. The advantage of COR-based FTIDF is demonstrated over other approaches. In Khamari, Sahu, and Panda (Citation2020), the authors have suggested modified moth swarm algorithm (mMSA)-tuned hybrid FPD-PI controller for frequency control of distributed power generation system (DPGS) through EVs. The benefit of the mMSA over MSA is evaluated. The traditional fuzzy PID is established to be operative for getting a response in different disturbances (Fereidouni, Masoum, and Moghbel Citation2015; Mohanty et al. Citation2016). Under huge uncertainties, the traditional fuzzy is less effectual and may not offer the anticipated system performances. In the presence of uncertainties, improved dynamic performance can be obtained with twin membership function-based type-2 fuzzy PID (T2FPID) controllers (Sahu et al. Citation2018). The present article proposes a type-2 fuzzy fractional-order PD-PI (T2FFOPD-PI) controller to regulate the frequency of MGs.
Heuristic techniques are gaining popularity among researchers for their ability to optimal controller parameter tuning. Several artificial intelligence (AI) methods have been engaged in the area of power system, i.e. mayfly optimisation algorithm (MOA), bat-inspired algorithm (BIA), genetic algorithm (GA), whale optimisation algorithm (WOA), imperialist competitive algorithm (ICA), crow search algorithm (CSA), and future search algorithm (Elsisi Citation2018; Elsisi et al. Citation2018; Elsisi Citation2019a, Citation2019b, Citation2019c, Citation2019d; Elsisi et al. Citation2020). The different optimisation technique is adopted for different optimisation problem having their strengths and weaknesses. Thus, there may not be any best optimisation technique which will provide the best result among all others for all kind of research problems. This shows the path for researchers to always develop, examine and apply new optimisation techniques for various real-world problems. Consequently, a large quantity of investigations is taking place day by day for developing innovative optimisation methods. Furthermore, existing methods are sometimes developed by proposing a hybrid technique having benefits of two different techniques. Other modifications to existing techniques also have been recommended by various authors to get better effectiveness of the current schemes or to pertain to a particular problem. Little variations to the existing optimisation method can be planned as improved whale optimization algorithm (Xiong et al. Citation2019), Improved grey wolf optimization (Sahoo and Panda Citation2018), binary butterfly optimization algorithm (Arora and Anand Citation2019), hybrid whale optimization algorithm with simulated annealing (Mafarja and Mirjalili Citation2017), hybrid adaptive ‘gbest’-based gravitational search and pattern search (PS) method (Khadanga and Kumar Citation2017), cosine adopted modified WOA (Saha and Panda Citation2020) and least mean the square root of exponential algorithm (Mohammed et al. Citation2021).
No optimisation problem is appropriate for all problems. Harris hawks optimization (HHO) algorithm is recently suggested based on nature-inspired (Heidari et al. Citation2019). The supremacy of HHO is established over GA, DE, PSO, BBO, CS, TLBO, FPA, FA, BA/BAT, GWO, and MFO in Heidari et al. (Citation2019). In HHO, the transition from exploration to exploitation is governed by E, i.e. fleeing energy of prey which decreases linearly. For E ≥ 1, the exploration phase is followed, and for E < 1, the exploration phase is followed. Thus, the original HHO has a drawback in that it overlooks the influence of accurate balance between them to deliver a global solution. This limitation has been taken care of in the proposed enhanced HHO (EHHO), which was a more exact equilibrium among the exploration and exploitation to get a better solution. Also, scaling factors are introduced to avoid the random movement of search agents in the initial stages of the search procedure.
This paper proposes a newly recognised and competent technique named EHHO algorithm to tune gains of T2FFOPD-PI controller. The performance of EHHO-based T2FFOPD-PI is compared with the GA, PSO, GSA, GWO, HHO-based PID and EHHO-based FPID, T2FPID controllers. Additional examinations are carried out to confirm the efficiency and sturdiness character of the recommended approach against the deviations of participation, load, renewable powers, and in system parameters.
The contributions of the paper are concise as follows:
A novel plan robust T2FFOPD-PI controller is applied for frequency stability in MGS.
EHHO technique is proposed where the more accurate equilibrium between the exploration and exploitation stage is maintained, and scaling factors are introduced to improve the algorithm performance.
The parameters of the controllers are optimised by a recent intelligence algorithm named EHHO.
The superiority of EHHO is demonstrated over the HHO technique as well as other recognised optimisation methods like a genetic algorithm (GA), grey wolf optimisation (GWO), gravitational search algorithm (GSA), and particle swarm optimisation (PSO).
The usefulness of the planned T2FFOPD-PI controller is authenticated by appropriate relative study with PID, FPID, T2FPID, and fuzzy PD-PI controllers (Khamari, Sahu, and Panda Citation2020).
The suggested approach is tested against the uncertainties of system parameters for robustness.
The simulation results and OPAL-RT results are confirmed for real-time validation.
2. Enhanced Harris hawks optimisation (EHHO)
Harris’s hawk’s optimisation (HHO) method is based on the cooperative search activities of Harris’s hawk's birds in haunting (Heidari et al. Citation2019). HHO operates in the following stages (Heidari et al. Citation2019).
2.1. Exploration stage
The populations of Harris’s hawks are taken as possible results. Prey is the finest result in a single iteration. Harris's hawks follow a double approach of the same worth to search the search area. The first method for the succeeding position of hawks is obtained on alteration between the current and random location of a hawk. The following method is based on the difference of location of the best solution achieved till now and the average location of all hawks. These strategies are expressed as follows:
(1)
(1) where H(k+1) is the position of hawks in the successive stage, Hrabbit (k) is the position of prey, H(k) is the current site of hawks, c1, c2, c3, c4, and p are random values in the range (0,1), ub and lb are the limits of variables, Hrand(k) is an arbitrarily designated hawk, and Hm(k) is the average location of all hawks in the present iteration. The Hm(k) is valued as:
(2)
(2) N is the population of hawks.
2.2. Crossover of stages (exploration to exploitation)
The reduction of energy of the prey is expressed as
(3)
(3) Fleeing energy of prey is E, Eo is the energy at the starting stage, k and l are present and last iterations. Eo varies randomly in between (−1, 1) at every iteration as
(4)
(4)
for the exploration phase and
for the exploitation stage.
2.3. Exploitation phase
The prey tries to escape from insecure locations. If e represents the chance of prey’s successful escape (e < 0.5) or unsuccessful escape (e ≥ 0.5), various hunting strategies by hawks can be expressed as follows:
2.3.1. Soft besiege
If e ≥ 0.5 and , the prey take random bewildering steps to escape but fails to do so. It is presented as:
(5)
(5)
(6)
(6) where
match to different steps strength of prey differs with every iteration, and c5 is the random value in a range (0, 1).
2.3.2. Hard besiege
If e ≥ 0.5 and, the prey is exhausted. The hawks enclose prey hardly. The locations of hawks are updated as:
(7)
(7)
2.3.3. Soft besiege with a rapid jump
If e < 0.5 and , the prey is capable to escape successfully. Soft besiege is performed by the hawks with the fast attack. Levy flight (LF) shape is employed to repeat puzzling efforts of prey and fast jumps of hawks. The positions of hawks are expressed as:
(8)
(8) where J is the objective.
The hawks perform soft besiege Sb at the beginning, and if the result of this fall is not improved, then the LF pattern constructed plunge is done. Mathematically, it is expressed as
(9)
(9)
(10)
(10) Dimension of task (d), random path of size 1×d is v and levy flight is expressed as LF and stated as:
(11)
(11) Here
and u,v are random values between (0–1).
2.3.4. Hard besiege with a rapid jump
If e < 0.5 and , hard besiege with rapid jump happens by hawks as:
(12)
(12)
(13)
(13) Here, SLF is defined in a new way
(14)
(14) In HHO (Heidari et al. Citation2019), the switch between exploration and exploitation segment is done depending on the fleeing energy of prey (E). Exploration takes place when |E| ≥ 1, and exploitation takes place when |E| < 1. Excessively exploration of search space might help to evade being getting stuck in local optima but it brings together additional randomness and the best solution may not be attained. Also, surplus exploitation is associated with less randomness. The algorithm may not deliver the optimum solution. Therefore, equilibrium amongst exploration and exploitation stages should be preserved throughout the iterations. Also, in HHO, the value of E is governed by Equation (3) and E0 has varied arbitrarily as Equation (4). In the present study, E and E0 are varied by Equations (15) and (16).
(15)
(15)
(16)
(16) Equation (15) ensures that a greater number of iterations are devoted to the exploitation phase and less for the exploration phase. The reduction in the number of iterations of the exploration phase is compensated by Equation (16) where a cosine function is introduced. The cyclic function shape of the cosine function makes a solution to be relocated. This practice can guarantee appropriate exploration of search space identified among two solutions, and the technique is capable to search elsewhere in the space and also among their corresponding positions.
In the HHO method, all other hawks try to alter their positions based on the best position of hawks. In the initial search stages, the best position of hawks is unidentified. Thus, the method of modifying using big steps primarily may outcome in affecting whales far off the optimum value. Therefore, scaling factors are involved to limit the movement of hawks during the early phases of the algorithm. Before the start of every iteration, the modified position of hawks is adjusted as:
(17)
(17) scaling factor is presented as
(18)
(18) The inclusion of scaling factors allows the hawks to move slowly during the beginning of the search procedure. Thus, the search capability of the algorithm is refined. During the advanced stages, as improved results are found, hawks travel at a normal rate.
3. Modeling of the system under study
The hybrid power system includes solar and wind energy with different storage components like BESS and FESS, which is presented in this study. BESS and FESS are having higher time constants than EVs, DEG and FC are maintained. From the results shown in the paper, it can be concluded that the suggested approach is competent in an actual power system and maintains complete system dynamics. Proposed MGS using diverse generations and storage units along with EVs is presented in and , respectively. Every component is expressed with its transfer function parameter values in . Total generation is from two FCs, three WTGs, one DEG and one PV. To the load side, one from every storage element BEESs, FESSs, and EVs are linked.
Table 1. Nominal Parameters of MGS [3, 15, 26].
3.1. Modeling of different generation sources
Modeling of PV, FC, DEG and WTG is given by their first-order transfer functions (Equations (19–22)) with their corresponding gains (,
,
,
) and time constants (
,
,
,
) reported in (Pan and Das Citation2015, Citation2016a; Khamari, Sahu, and Panda Citation2020) where n is the no. of elements of every source.
(19)
(19)
(20)
(20)
(21)
(21)
(22)
(22)
AE utilises a portion i.e. () of the generated power. It discharges hydrogen as needed by the fuel cell (Pan and Das Citation2015, Citation2016a; Khamari, Sahu, and Panda Citation2020).
is 0.6 in the current work.
(23)
(23) For a steady function of the hybrid structure, the role of energy storing elements such as FESSs and BEESs is very important as power availability during generation deficiency can be made possible by these units. FESSs and BEESs are the two storage structures given by Equations (24) and (25)
(24)
(24)
(25)
(25) EV system is expressed by a first-order transfer function (Pham, Trinh, and Hien Citation2016; Khamari, Sahu, and Panda Citation2020).
(26)
(26) where
and
are the gain and time constant of the EV.
shows energy storage elements with their rate constraint non-linearity.
Figure 3. Rate constraint-type non-linearity for an energy storing element having a gain of K and time constant.
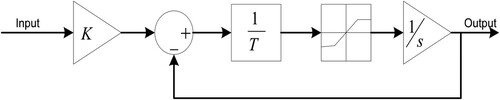
and
represent their rate constraint nonlinearity which refers to the inability to store or release power very fast for any energy storage.
The equivalent power system is expressed as
(27)
(27) where D is the equivalent damping (=0.03) and M is the equivalent inertia (=0.4) that signify rotating machines existing in studied MGS.
3.2. Controller structure
Under huge uncertainties, the traditional fuzzy logic control (FLC) is the slightest effectual and incapable of progressing system performances. Due to huge qualms, the twin (MFs)-based type-II fuzzy PID offers enhanced dynamic performance of electrical systems (Sahu et al. Citation2018). The current work recommends a type-2 fuzzy FO PD-PI (T2FFOPD-PI) structure for frequency stabilisation in MGS. The MFs are prepared by coordination of minor membership function and higher membership function. The MFs of the type-2 FLC use upper MF (UMF) and lower MF (LMF). Conjoining UMF and LMF a barrier is established as revealed in . A footprint of uncertainty (FOU) is constructed constrained between UMF and LMF. The MFs for inputs () are presented in . Fuzzification is the first stage of FLC. It processes inputs and generates required structured fuzzy sets using MFs. The linguistic variables employed for MFs are Extreme Negative (EXN), Least Negative (LN), Zero (ZER), Least Positive (LP) and Extreme Positive (EXP).
The type-2 fuzzy set (FS) may be expressed as
(28)
(28) where
is the UMF, Var is the main variable, a is the added variable of domain JVar
The universe of discourse is expressed as
(29)
(29) where
= Union on ACE and a
Now, the equations can be written as
(30)
(30) where JVar is expressed as
(31)
(31)
The assembly of the MF with FOU is illustrated in .
The knowledge base includes a rule base and interface engine. The rule base is illustrated in . Both Var and dAVar are the input signals to type-2 FLC which generates output y.
Table 2. Rule base of fuzzy controller.
Type-2 FLC characteristic can be expressed as
(32)
(32)
(33)
(33) The related FS firing forte is
(34)
(34)
(35)
(35)
(36)
(36)
TR is employed to change type-2 to type-1 FS for defuzzification. The approaches for defuzzification are the center of sums, centroid, the center of sets (SOC), etc., out of which SOC is reported as the best approach. The outputs are:
(37)
(37)
(38)
(38)
(39)
(39)
where and
are related to two MF of type-1 FLC. By averaging, the output of type-2 FLC is found. In fuzzy PD-PI structure, a double-stage controller is realised. shows T2FFOTID controller structure.
3.3. Objective function
The cost function assists to choose suitable gains of the best controller. It has to be a huge confront for planning engineers to choose the correct cost function so that system performances might be modified appreciably. An integral square error (ISE) criterion, which targets to reduce objective function, is taken as:
(40)
(40) where simulation time is t, the frequency deviation is ΔF, and control efforts (Δu). To formulate the two terms in Equation (40) spirited in the search method, kn and w are taken as 200 and 0.5, respectively.
4. Results and discussions
To establish the efficacy of the recommended approach, the frequency regulation problem of MGS (Pan and Das Citation2016a; Khamari, Sahu, and Panda Citation2020) is formulated and a study is carried out. The parameters of MGS are given in . Different sources and load demand patterns of MGS are shown in (a,b). presents renewable power along with the power imbalance of MGS for nominal loading. The frequency of the system gets influenced by the power imbalance. Suitable control action needs to be performed to suppress deviation in frequency by the suitable controller action.
Both PID, FPID, T2FPID, and T2FFOPD-PI controllers are considered, and gains are optimised using HHO and EHHO techniques. The structure of FPID is similar to T2FFOPD-PI shown in , but the fractional derivatives/integer is exchanged by integer ordered derivatives/integers. and Type-2 FLC is used in place of Type 1 FLC. The performance of the suggested approach is also equated by various current meta-heuristic methods like GA, GWO, GSA and PSO. To make a fair comparison for each algorithm, the number of functions is taken, several search agents, and the number of iterations are taken as3000, 30 and 100, respectively. The parameter of algorithms is delivered in . The range of the controller parameters is picked in between [0, 2] for PID, FPID, T2FPID and T2FFOPD-PI controllers. All the suggested techniques are performed in 20 instances, and the finest result which will give a minimum J value is obtained in 20 runs. These values are employed as the gains of the controller. The tuned values are located in . It is noticed from that with PID, a smaller amount of J value is found by HHO (J = 1.5242) evaluated to GA (J = 1.5489), PSO (J = 1.5419), GSA (J = 1.5352) and GWO (J = 1.5284). The J value is reduced to 1.5156 while EHHO is engaged. J value with the proposed EHHO technique is improved from PSO, GA, GSA, GWO and HHO by 2.19%, 1.73%, 1.29%, 0.84% and 0.56%, respectively. Thus, EHHO outperforms, HHO, GA, PSO, GSA and GWO techniques. Further reduced J values are found when FPID, T2FPID and T2FFOPD-PI controllers are applied and the smallest amount J value (J = 0.4187) is found by EHHO-tuned T2FFOPD-PI. With the same EHHO technique, enhancement in J value with recommended T2FFOPD-PI controller evaluated to PID, FPID and T2FPID is 72.37%, 56.75% and 9.31%, respectively. From , this can be concluded that suggested EHHO-tuned T2FFOPD-PI controller provides less J value (0.4187) compared to the published mMSA-based FPD-PI (Khamari, Sahu, and Panda Citation2020) controller (J = 0.8242). To assess the system performance, the time-domain simulation of different cases is studied.
Table 3. Parameter setting of dissimilar techniques (no. of function evaluations=3000 for each technique).
Table 4. Optimal controller parameters.
Case 1: Normal operation
Renewable generations (solar and wind) and load patterns as given in (a,b) are considered. The response with proposed EHHO optimised PID, FPID, T2FPID, T2FFOPD-PI and mMSA- based FPD-PI (Khamari, Sahu, and Panda Citation2020) are exhibited in . It can be perceived from that, the system performance with the proposed T2FFOPD-PI is better than that with PID, FPID, T2FPID and mMSA based FPD-PI (Khamari, Sahu, and Panda Citation2020) controllers. It can be noticed from that the integral error values are less with the recommended method compared to others. The input powers of controllable sources with the proposed EHHO optimised T2FFOTID controller are presented in . It can be perceived from that when the power imbalance is negative (up to 8 s and between 11 and 25 s), input powers of controllable sources are also negative i.e. they supply the difference power to minimise the power imbalance. From 25 to 82 s, when the power imbalance is positive i.e. generation is more than load, input powers of controllable sources became positive, i.e. they receive power from the MGS to minimise the power imbalance. In the last period, from 82 to 90 s, the power imbalance becomes negative and positive and the controllable sources also became negative and positive during this period.
Table 5. Performance comparison of EHHO optimised controllers.
Case 2: Load is increased by 100%
In this study, it is assumed that the solar and wind generations are there, and at the same time, the load demand pattern is improved by 100%. The whole renewable power and new power mismatch of MGS are shown in . The response of frequency deviation is illustrated in for the above case. From and , the suggested T2FFOPD-PI controller is observed to be superior in performance than that of PID, FPID, T2FPID, T2FFOPD-PI and mMSA-based FPD-PI (Khamari, Sahu, and Panda Citation2020) controllers. The input powers of controllable sources with proposed EHHO optimised T2FFOPD-PI controller are illustrated in . It can be seen from that when the power imbalance is negative (0–25, 42–45, 70–72 and 81–90 s), input powers of controllable sources are also negative i.e. they supply the difference power to minimise the power imbalance. When the power imbalance is positive (25–42, 48–66, 66–70 and 72–81 s), i.e. generation is more than load, input powers of controllable sources turn into positive, i.e. they obtain power from the MGS to diminish the power imbalance.
Case 3: Decreased participation of renewable sources
Solar and wind powers are considered to be reduced by 50% in this case. The new full renewable power and power difference of MGS are shown in . The frequency variation response for this case is illustrated in . It can be noticed from that the system performance with recommended T2FFOPD-PI is better than that by PID, FPID, T2FPID, T2FFOPD-PI and mMSA-based FPD-PI (Khamari, Sahu, and Panda Citation2020) controllers. The input powers of controllable sources with proposed EHHO optimised T2FFOPD-PI is illustrated in . It can be observed from that when the power imbalance is negative (0–25 and 81–90 s), input powers of controllable sources are also negative; i.e. they supply the difference power to minimise the power imbalance. When the power imbalance is positive (25–42, 48–66, 66–70 and 72–81 s), i.e. generation is more than load, input powers of controllable sources turn into positive, i.e. they obtain power from the MGS to diminish the power imbalance.
Figure 14. Total renewable power and power imbalance of MGS for case 3 (renewable powers are decreased by 50%).
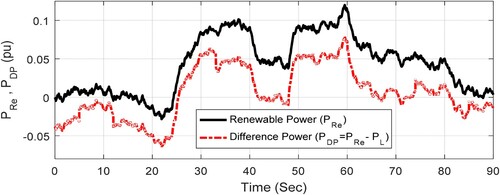
Case 4: Unavailability of renewable sources
shows frequency response in the case of unavailability of solar and wind powers. In such a situation, load demand constantly exceeds the generation. Input powers of controllable sources (with EHHO tuned T2FFOPD-PI) are shown in . As generation by renewables is less than demand, the input powers of controllable sources are always negative, i.e. they supply energy to MGS. The higher the power imbalance occurs, the larger is the energy supplied by controllable sources.
Figure 18. Controllable sources with T2FFOPD-PI for case 4 (unavailability of renewable power sources).
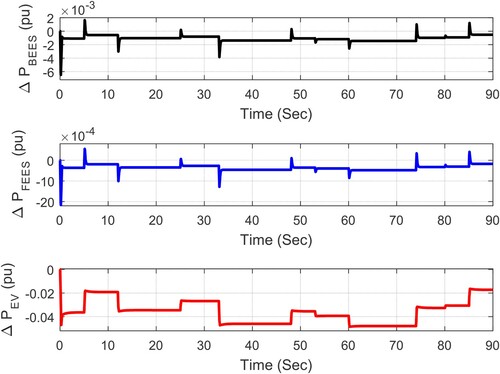
Case 5: 50% reduction in M and D parameters
In this case, it is assumed M and D parameters are decreased by 50%. The usefulness of the suggested T2FFOPD-PI controller is validated by considering variation in M and D system parameters to examine the sturdiness of MGS. In , the frequency variation is illustrated for a 50% reduction in M and D parameters. It can be noticed from that the frequency deviation has lees fluctuation with suggested T2FFOPD-PI compared to PID, FPID, T2FPID and mMSA-based FPD-PI (Khamari, Sahu, and Panda Citation2020). This reveals that the suggested EHHO-based T2FFOPD-PI controller achieved adequately for frequency variation in MGS even when system parameters variation to a larger value is considered. The imbalance between total renewable and the new power of MGS is shown in . The input powers of controllable sources with proposed EHHO optimised T2FFOTID are displayed in . presents that when the power imbalance is negative, input powers of controllable sources are also negative; i.e. they supply the difference power to minimise the power imbalance. When power difference is positive, i.e. generation is more than load, input powers of controllable sources became positive, i.e. they receive power from the MGS to minimise the power imbalance. The performance comparison of EHHO optimised controllers for all the cases is summarised in . For performance comparison, various integral errors like ISE, ITAE, IAE, ITSE, overshoots (Os) and undershoots (Us) with EHHO optimised PID, FPID, T2FPID, T2FFOPD-PI and mMSA-based FPD-PI (Khamari, Sahu, and Panda Citation2020) controller are summarised. It can be noticed from that significantly less integral errors, Os and Us are found with the proposed T2FFOPD-PI controller compared to PID, FPID, T2FPID controllers and mMSA-based FPD-PI (Khamari, Sahu, and Panda Citation2020). This concludes that the T2FFOPD-PI formulates the MGS healthier and works adequately under different operating circumstances.
5. Experimental validation based on OPAL-RT
The simulation results based on OPAL-RT pertain to experimental confirmation of the suggested method (EHHO based T2FFOPD-PI) as illustrated in . The real-time simulation technique replicates the delays, and faults that are intrinsically there but unnoticed in the traditional off-line simulations (Khadanga and Kumar Citation2017; Mafarja and Mirjalili Citation2017). Comparison of the frequency response of SIMULINK and OPAL-RT based-Real-Time Simulator (RTS) are illustrated in for normal operation. It can be seen from the MATLAB/SIMULINK results equal with OPAL-RT real-time simulation results.
6. Conclusion
This work presents a frequency regulation of the micro-grid system with storage units along with EVs and numerous renewable sources. A novel type-2 fuzzy fractional-order pd-pi (T2FFOPD-PI) controller based on the EHHO technique is employed to get better system performance. It is observed that the EHHO technique outperforms HHO, GA, PSO, GWO and GSA techniques. The percentage enhancement in J value with suggested EHHO related to GA, PSO, GSA, GWO and HHO is 2.19%, 1.73%, 1.29%, 0.84% and 0.56%, respectively. It is seen that under normal operation, with the same EHHO technique, an increase in J value with recommended T2FFOPD-PI controller evaluated to PID, FPID and T2FPID are 72.37%, 56.75% and 9.31%, respectively. Also, with proposed EHHO-based T2FFOPD-PI controller provides less J value compared to the published mMSA-based FPD-PI (Khamari, Sahu, and Panda Citation2020) controller (J = 0.8242). The performance of EHHO-based T2FFOPD-PI is equated with PID FPID, T2FPID and modified moth swarm algorithm (mMSA) based fuzzy PD-PI controller (Khamari, Sahu, and Panda Citation2020) under uncertain cases like increased/decreased renewable participation, increased load, and varied system parameters. It is also noticed that lower value of integral errors, overshoots and undershoots are found with the proposed T2FFOPD-PI controller compared to PID FPID T2FPID and mMSA-based FPD-PI (Khamari, Sahu, and Panda Citation2020) controllers in all cases. Finally, the proposed approach is examined by OPAL-RT real-time simulation for validation. It is perceived that the MATLAB results are similar to that of OPAL-RT real-time results. The proposed approach can be extended with other soft computing techniques and intelligent controller structures. Also, the state of charge (SOC) of EV may be included for a more realistic approach of frequency control with EV.
Disclosure statement
No potential conflict of interest was reported by the author(s).
References
- Arora, S., and P. Anand. 2019. “Binary Butterfly Optimization Approaches for Feature Selection.” Expert Systems with Applications 116: 147–160.
- Arya, Y. 2018. “Improvement in Automatic Generation Control of Two-Area Electric Power Systems via a new Fuzzy Aided Optimal PIDN-FOI Controller.” ISA Transactions 80: 475–490.
- Elsisi, M. 2018. “Future Search Algorithm for Optimization.” Evolutionary Intelligence. doi:https://doi.org/10.1007/s12065-018-0172-2
- Elsisi, M. 2019a. “Design of Neural Network Predictive Controller Based on Imperialist Competitive Algorithm for Automatic Voltage Regulator.” Neural Computing and Applications. doi:https://doi.org/10.1007/s00521-018-03995-9
- Elsisi, M. 2019b. “New Design of Adaptive Model Predictive Control for Energy Conversion System with Wind Torque Effect.” Journal of Cleaner Production 240: 118265.
- Elsisi, M. 2019c. “New Design of Robust PID Controller Based on Meta-Heuristic Algorithms for Wind Energy Conversion System.” Wind Energy. doi:https://doi.org/10.1002/we.2439
- Elsisi, M. 2019d. “Optimal Design of Non-fragile PID Controller.” Asian Journal of Control. doi:https://doi.org/10.1002/asjc.2248
- Elsisi, M. 2020. “New Variable Structure Control Based on Different Meta-Heuristics Algorithms for Frequency Regulation Considering Nonlinearities Effects.” International Transactions on Electrical Energy Systems. doi:https://doi.org/10.1002/2050-7038.12428
- Elsisi, M., M. Aboelela, M. Soliman, and W. Mansour. 2018. “Design of Optimal Model Predictive Controller for LFC of Nonlinear Multi-Area Power System with Energy Storage Devices.” Electric Power Components and Systems 46 (11-12): 1300–1311.
- Elsisi, M., and M. Soliman. 2020. “Optimal Design of Robust Resilient Automatic Voltage Regulators.” ISA Transactions. doi:https://doi.org/10.1016/j.isatra.2020.09.003
- Elsisi, M., M. Tran, K. Mahmoud, M. Lehtonen, and M. F. Darwish. 2020. “Robust Design of ANFIS-Based Blade Pitch Controller for Wind Energy Conversion Systems Against Wind Speed Fluctuations.” IEEE access. doi:https://doi.org/10.1109/ACCESS.2021.3063053
- Fereidouni, A., A. S. Masoum, and M. Moghbel. 2015. “A new Adaptive Configuration of PID Type Fuzzy Logic Controller.” ISA Transactions 56: 222–240.
- Gheisarnejad, M., and M. H. Khooban. 2019. “Secondary Load Frequency Control for Multi-Microgrids: HiL Real-Time Simulation.” Soft Computing 23: 5785–5798.
- Hasanien, H. M. 2018. “Whale Optimisation Algorithm for Automatic Generation Control of Interconnected Modern Power Systems Including Renewable Energy Sources.” IET Generation, Transmission & Distribution 12 (3): 607–614.
- Heidari, A. A., S. Mirjalili, H. Faris, I. Aljarah, M. Mafarja, and H. Chen. 2019. “Harris Hawks Optimization: Algorithm and Applications.” Future Generation Computer Systems. 97: 849–872.
- Khadanga, R. K., and A. Kumar. 2017. “Hybrid Adaptive ‘Gbest’-Guided Gravitational Search and Pattern Search Algorithm for Automatic Generation Control of Multi-Area Power System.” IET Generation, Transmission & Distribution 11 (13): 3257–3267.
- Khamari, D., R. K. Sahu, and S. Panda. 2020. “A Modified Moth Swarm Algorithm-Based Hybrid Fuzzy PD–PI Controller for Frequency Regulation of Distributed Power Generation System with Electric Vehicle.” Journal of Control, Automation and Electrical Systems 31: 675–692.
- Khooban, M. H., T. Dragicevic, F. Blaabjerg, and M. Delimar. 2018a. “Shipboard Microgrids: A Novel Approach to Load Frequency Control.” IEEE Transactions on Sustainable Energy 9 (2): 843–852.
- Khooban, M. H., T. Niknam, F. Blaabjerg, and T. Dragicevic. 2017. “A new Load Frequency Control Strategy for Micro-Grids with Considering Electrical Vehicles.” Electric Power Systems Research 143: 585–598.
- Khooban, M. H., T. Niknam, M. Shasadeghi, T. Dragicevic, and F. Blaabjerg. 2018b. “Load Frequency Control in Microgrids Based on a Stochastic Noninteger Controller.” IEEE Transactions on Sustainable Energy 9 (2): 853–861.
- Mafarja, M. M., and S. Mirjalili. 2017. “Hybrid Whale Optimization Algorithm with Simulated Annealing for Feature Selection.” Neurocomputing 260: 302–312.
- Mohammed, H., H. Qais, M. Hasanien, and S. Alghuwainem. 2021. “A Novel LMSRE-Based Adaptive PI Control Scheme for Grid-Integrated PMSG-Based Variable-Speed Wind Turbine.” International Journal of Electrical Power & Energy Systems 125: 106505.
- Mohanty, P. K., B. K. Sahu, T. K. Pati, S. Panda, and S. K. Kar. 2016. “Design and Analysis of Fuzzy PID Controller with Derivative Filter for AGC in Multi-Area Interconnected Power System.” IET Generation, Transmission & Distribution 10: 3764–3776.
- Pan, I., and S. Das. 2015. “Kriging Based Surrogate Modeling for Fractional Order Control of Microgrids.” IEEE Transactions on Smart Grid 6 (1): 36–44.
- Pan, I., and S. Das. 2016a. “Fractional Order AGC for Distributed Energy Resources Using Robust Optimization.” IEEE Transactions on Smart Grid 7 (5): 2175–2186.
- Pan, I., and S. Das. 2016b. “Fractional Order Fuzzy Control of Hybrid Power System with Renewable Generation Using Chaotic PSO.” ISA Transactions 62: 19–29.
- Patel, S., B. Mohanty, and H. M. Hasanien. 2020. “Competition Over Resources Optimized Fuzzy TIDF Controller for Frequency Stabilization of Hybrid Micro-Grid System.” International Transactions on Electrical Energy Systems. doi:https://doi.org/10.1002/2050-7038.12513
- Pham, T. N., H. Trinh, and L. V. Hien. 2016. “Load Frequency Control of Power Systems with Electric Vehicles and Diverse Transmission Links Using Distributed Functional Observers.” IEEE Transactions on Smart Grid 7 (1): 238–252.
- Ray, P., S. Mohanty, and N. Kishor. 2010. “Small-Signal Analysis of Autonomous Hybrid Distributed Generation Systems in Presence of Ultracapacitor and Tie-Line Operation.” Journal of Electrical Engineering 61 (4): 205–214.
- Saha, N., and S. Panda. 2020. “Cosine Adapted Modified Whale Optimization Algorithm for Control of Switched Reluctance Motor.” Computational Intelligence. doi:https://doi.org/10.1111/coin.12310
- Sahoo, B. P., and S. Panda. 2018. “Improved Grey Wolf Optimization Technique for Fuzzy Aided PID Controller Design for Power System Frequency Control.” Sustainable Energy, Grids and Networks 16: 278–299.
- Sahu, P. C., S. Mishra, R. C. Prusty, and S. Panda. 2018. “Improved-salp Swarm Optimized Type-II Fuzzy Controller in Load Frequency Control of Multi Area Islanded AC Microgrid.” Sustainable Energy, Grids and Networks 16: 380–392.
- Sahu, R. K., S. Panda, and G. T. C. Sekhar. 2015. “A Novel Hybrid PSO-PS Optimized Fuzzy PI Controller for AGC in Multi Area Interconnected Power Systems.” International Journal of Electrical Power & Energy Systems 64: 880–893.
- Shankar, G., and V. Mukherjee. 2016. “Load Frequency Control of an Autonomous Hybrid Power System by Quasi-Oppositional Harmony Search Algorithm.” International Journal of Electrical Power & Energy Systems 78: 715–734.
- Soliman, M. A., H. M. Hasanien, S. Alghuwainem, and A. Al-Durra. 2019. “Symbiotic Organisms Search Algorithm-Based Optimal Control Strategy for Efficient Operation of Variable-Speed Wind Generators.” IET Renewable Power Generation 13 (14): 2684–2692.
- Xiong, G., J. Zhang, D. Shi, and Y. He. 2019. “Parameter Extraction of Solar Photovoltaic Models Using an Improved Whale Optimization Algorithm.” Energies 174: 388–405.