ABSTRACT
Introduction
Clinical proteomics studies of Alzheimer’s disease (AD) research aim to identify biomarkers useful for clinical research, diagnostics, and improve our understanding of the pathological processes involved in the disease. The rapidly increasing performance of proteomics technologies is likely to have great impact on AD research.
Areas covered
We review recent proteomics approaches that have advanced the field of clinical AD research. Specifically, we discuss the application of targeted mass spectrometry (MS), labeling-based and label-free MS-based as well as affinity-based proteomics to AD biomarker development, underpinning their importance with the latest impactful clinical studies. We evaluate how proteomics technologies have been adapted to meet current challenges. Finally, we discuss the limitations and potential of proteomics techniques and whether their scope might extend beyond current research-based applications.
Expert opinion
To date, proteomics technologies in the AD field have been largely limited to AD biomarker discovery. The recent development of the first successful disease-modifying treatments of AD will further increase the need for blood biomarkers for early, accurate diagnosis, and CSF biomarkers that reflect specific pathological processes. Proteomics has the potential to meet these requirements and to progress into clinical routine practice, provided that current limitations are overcome.
1. Introduction
Alzheimer’s disease (AD) affects around 50 million people worldwide and accounts for 60–80% of all dementia cases [Citation1]. It is a progressive neurodegenerative disease that gradually destroys memory and cognitive functions. Its characteristic pathological features, described by Alois Alzheimer over a century ago, are two types of protein aggregates in the brain called plaques and tangles [Citation2]. The plaques were found to mainly consist of the peptide amyloid β (Aβ) 1–42 [Citation3], while neurofibrillary tangles were discovered to be composed of extensively phosphorylated tau protein [Citation4]. In addition, around half of AD patients also have protein aggregates in the form of Lewy bodies, consisting of α-synuclein and, especially in elderly patients, transactive response DNA-binding protein 43 (TDP-43) inclusions can be found [Citation5]. A strong genetic association of AD with the gene encoding apolipoprotein E (apoE) has also been shown, with carriers of the APOE ε4 allele having a 40% increased risk of acquiring AD [Citation6].
AD starts with a long preclinical phase, with amyloid pathology commencing as early as 20 years before the onset of symptoms. In the subsequent prodromal stage, mild memory and cognitive symptoms begin to manifest, finally leading to AD dementia characterized by a marked loss of short-term memory, impaired reasoning skills and a reduced ability to perform complex tasks. The disease onset is usually above 65 years of age; however, around 5% of patients experience an earlier onset.
While it is still unknown what causes AD, the leading hypothesis stipulates that an imbalance in the production and clearance of Aβ 1–42 results in the formation of neurotoxic peptide oligomers that give rise to plaques and cause neurodegeneration [Citation7]. Recently, disease-modifying treatments based on therapeutic antibodies targeting Aβ peptides and oligomers have shown positive results in clinical trials [Citation8–11]. While these treatments efficiently removed plaques, the effect on cognition was moderate, the treatment expensive, and potentially associated with health risks. Nevertheless, the results of these trials are a strong indicator that AD may become treatable in a not-too-distant future.
Biomarkers are a key component in realizing such treatments as they make it possible to identify patients already in the pre-clinical stage, when treatments can be expected to provide the greatest benefit, to monitor disease progression and treatment effects, and to differentiate between AD and other neurological conditions. Proteomics is playing an increasingly important role in identifying biomarkers useful for clinical research as well as in improving our understanding of the mechanisms and combination of pathological processes involved in the disease. Most proteomic studies to date have been performed in cerebrospinal fluid (CSF), which is in direct contact with the cells of the brain and therefore reflects many neurological disease-related processes. CSF biomarkers have found their way into routine clinical assessment, the prime example being the core CSF biomarker signature of AD: a decreased Aβ1–42/Aβ1–40 ratio, indicative of amyloid plaque pathology, elevated phosphorylated tau (p-tau), reflecting increased neuronal tau phosphorylation and secretion, which predicts tau tangle pathology, and total tau (t-tau), which indicates neuronal degeneration [Citation12]. Blood, being more accessible than CSF, is also being explored for its biomarker potential, with recent success in the analyses of phosphorylated and brain-derived tau species [Citation13,Citation14].
Currently, the two cornerstones of proteomic methodology are affinity- and mass spectrometry (MS)-based technologies. In this report, we review how the newest proteomics techniques are being applied in clinical AD research to identify new biomarkers and to monitor disease processes in clinical research. We will also discuss the emerging next generation of proteomics, future prospects of the field as well as the current challenges and limitations of the technology.
2. Challenges and requirements
CSF and blood share common biological characteristics that complicate AD biomarker discovery by proteomics. Firstly, both biofluids exhibit high interindividual biological variability and intra-individual variation in protein concentrations over time, causing large variances in measurements [Citation15–17] and thereby necessitating large study groups to achieve high statistical significance in identified biomarker candidates. Secondly, brain-derived proteins are generally low-abundant and thus harder to detect and quantify against the complex sample matrix of CSF and blood in which proteins span more than 10 orders of magnitude in concentration, and where a relatively small number of proteins account for a large fraction of the total protein amount [Citation18]. Due to these challenges, proteomic technologies should ideally accommodate the following requirements: (1) A capacity to analyze large clinical cohorts comprising hundreds of individuals within reasonable time to achieve sufficiently powered analyses to detect disease-associated changes in protein abundances. (2) Sample preparation and analysis must be reproducible to ensure comparability across a multitude of samples. (3) High detection sensitivity and deep proteomic coverage are required to be able to detect low-abundant markers of interest. In MS-based proteomics, the two main tuning parameters to meet these requirements are the sample preparation and LC-MS method itself. Generally, a compromise must be made between analysis time (extent of sample fractionation and HPLC) and depth of proteome coverage.
3. MS-based proteomics
3.1. Labeling-based proteomics
In labeling-based proteomics, peptides obtained through tryptic digestion are conjugated to a stable isotope-labeled chemical tag and individual samples are subsequently combined into sets for multiplexed analysis. While different chemical tags have been developed for proteomic applications, the tandem mass tag (TMT) technique [Citation19] is currently the most widely used in AD biomarker discovery studies. At present, there are 18 isobaric TMTs available that differ in their distribution of the stable isotopes 13C and 15N between a reporter and balance part of the tag [Citation20]. Multiplex samples are analyzed in data-dependent acquisition (DDA) mode on a high-resolution orbitrap mass spectrometer coupled to a nano-LC system. In the MS/MS fragment ion spectra, the reporter ions from each individual sample are detected separately due to their different stable-isotope labeling, enabling peptide quantitation in up to 18 individual samples simultaneously.
In AD research, the major benefit of TMT proteomics lies within its multiplexing capability by which the overall run time can be significantly reduced, thereby enabling larger studies to be performed. Furthermore, reproducibility concerns are largely limited to TMT set to set variability as opposed to individual sample run-to-run variability. Consequently, to date, several AD biomarker studies comprising hundreds of patients have been performed utilizing the TMT labeling approach. Additionally, multiplexing decreases the required sample volume per patient, which is especially important when working with precious study cohort samples of limited availability.
To ensure sufficient proteomic depth of the MS analysis, several approaches have been developed to reduce sample complexity and enhance biomarker candidate-relevant signals. Reversed-phase HPLC at basic pH with concatenated fractionation of the TMT sets, typically into 24 or 48 fractions, prior to LC-MS analysis is frequently employed [Citation21]. Fractionation decreases the complexity of the samples loaded on the LC-MS, enabling loading of larger sample amounts, and decreasing the incidence of co-isolation, which improves quantification. The implementation of high-field asymmetric waveform ion mobility spectrometry (FAIMS) has also proven useful for TMT proteomics to separate ions into subpopulations, thereby reducing overall spectral complexity and decreasing the incidence of co-isolation [Citation22].
TMT proteomics has considerably advanced the field of AD biomarkers with several impactful studies having been published over the last decade [Citation23–30]. A recent CSF proteomic study comprising >300 individuals across the AD continuum identified proteomic signatures by unsupervised clustering that divided AD patients into three distinct groups [Citation31]. Gene ontology analysis showed that the proteins in the three groups were involved in blood-brain-barrier integrity, synaptic hyperplasticity, and neuroinflammation, respectively, indicating the existence of AD subtypes with differences in their pathological changes. Analysis of this data set also identified an APOE ε4 genotype-dependent proteomic disease signature [Citation32], helped to validate genome-scale metabolic models [Citation33], and formed the basis for the design of a method targeting synaptic proteins [Citation34], including neurogranin, synaptotagmin-1, 14-3-3 proteins, syntaxins and synucleins, which is currently being used in published [Citation35,Citation36] and ongoing clinical studies of synaptic degradation.
Despite the benefits of TMT proteomics, the method has some drawbacks that need to be carefully considered. Because MS2 spectra are recorded in the DDA mode, there is a randomness in the selection of precursor ions for fragment ion analysis. Consequently, different peptides are often selected across TMT sets, resulting in missing data, particularly for low-abundant proteins close to the detection limit. Furthermore, TMT labeled peptides are generally more hydrophobic and have different charge states as well as fragmentation characteristics than their unlabeled forms [Citation37]. Setting up targeted methods to measure the same peptides in unlabeled form often requires extra work as LC-MS parameters need to be optimized.
3.2. Label-free proteomics
In label-free proteomics, peptide abundances are typically measured via chromatographic peak areas calculated by integrating the intensity of MS1 or MS2 signals as a function of retention time, yielding an extracted ion chromatogram (XIC) [Citation38]. Sample multiplexing is not possible; each sample is prepared and analyzed separately. Although this potentially increases the analytical variation as LC-MS performance may vary over time, the method allows for MS data to be recorded in data independent acquisition (DIA) mode, circumventing DDA-related detection bias. Owing to the complexity of resulting MS2 spectra, spectral libraries are typically generated in DDA-mode from extensively fractionated samples to facilitate peptide identification [Citation39].
Since run-to-run comparability is crucial for label-free proteomics, some studies have harnessed automated sample preparation platforms and avoided off-line pre-fractionation steps [Citation15,Citation40]. Although their proteomic coverage was, on average, lower than that of most TMT studies, they showed increased throughput as well as good robustness. Additionally, increasingly robust nano-LC systems contributed to the improved comparability of single runs within label-free proteomics studies.
Multiple label-free proteomics studies have contributed to our understanding in the AD field. For example, a recent study identified a consistent glycolytic signature in the CSF of AD patients with potential clinical utility [Citation40] while another proteomics study uncovered approximately 200 proteins that differed in abundance in AD versus control brain samples [Citation41].
While most AD proteomics studies to date use the TMT approach, a notable advance in the field of label-free proteomics has been recently made by using trapped ion mobility spectrometry (TIMS)-quadrupole-TOF and the principle of parallel accumulation-serial fragmentation (PASEF), synchronizing ion mobility separation with quadrupole mass selection [Citation42]. This technique, termed diaPASEF, significantly increases ion sampling efficiency of both simple and complex proteomes, clearly surpassing DDA and conventional DIA methods in their ion sampling capabilities. It holds potential as a sensitive method requiring comparatively short gradient times and minute sample amounts – important attributes for large-scale clinical studies.
The recently developed Asymmetric Track Lossless (Astral) mass analyzer has also shown promising potential in pushing the boundaries of proteomic coverage and detection sensitivity when combined with DIA [Citation43]. Due to the novelty of both diaPASEF and the Astral, however, clinical studies demonstrating their utility are yet lacking.
3.3. Targeted mass spectrometry
The identification of numerous promising biomarker candidates through exploratory proteomics creates a bottleneck situation since biomarker validation is more time-intensive than the initial discovery phase [Citation44]. This is especially true when utilizing immunoassays for biomarker validation, in which case suitable antibodies for the target in question may have to be generated first. A conceivably faster and more efficient way to validate biomarkers is by targeted MS for which peptide targets can be easily extracted from previous proteomics studies.
In the AD biomarker field, both selected reaction monitoring (SRM) and parallel reaction monitoring (PRM) assays are widely used to precisely quantify proteins of interest [Citation34,Citation45–49]. While SRM is typically performed on a triple quadrupole MS measuring carefully selected precursor fragments (transitions), PRM assays make use of orbitrap cells simultaneously analyzing all transitions stemming from a peptide of interest. Both assays perform comparably in most settings and demonstrate a high analyte multiplexing capacity, with the potential to measure dozens of proteins in a single run.
Contributions to the AD biomarker field include PRM-based assays measuring multiple p-tau species in plasma and CSF showing that site-specific phosphorylations occur at different periods of AD disease progression as well as reflect distinct amyloid and tau pathologies [Citation45–47]. Another example includes an SRM-based synaptic biomarker panel evaluating synaptic dysfunction [Citation34–36]. Synaptic proteins such as neuronal pentraxin-2 could hold potential as AD progression marker as their CSF levels appear to correlate with cognitive decline. Furthermore, targeted MS approaches outperformed immunoassays in the measurement of plasma Aβ1–42/1–40 ratios to predict amyloid status in AD patients and controls [Citation50,Citation51], and there are also companies that offer plasma Aβ1–42/1–40 testing by immunoprecipitation (IP)-MS in clinical laboratory practice [Citation52].
4. Affinity-based proteomics
Over the past decade, affinity-based proteomics has gained increasing popularity in the field of AD research. Conceptually, DNA oligonucleotide-conjugated affinity reagents such as antibodies or aptamers bind to their protein targets with high specificity and bound proteins are detected as well as quantified via molecular genetic methods e.g. quantitative polymerase-chain reaction, microarray, or next-generation sequencing. That way, multiplex panels enable the simultaneous quantitation of hundreds to thousands of protein targets. Notable examples of affinity-based proteomics platforms that have been applied in AD research include Olink [Citation53,Citation54], and SomaScan [Citation55,Citation56] while the new NULISA platform [Citation57] has potential for future AD research applications. For instance, a recent study utilizing the Olink™ ProSeek immunoassay to measure CSF and plasma proteins across the AD continuum in the framework of the Swedish BioFINDER study identified novel biomarkers of early AD [Citation54]. Another study, employing SomaScan technology, found the plasma proteins prostate-specific antigen complexed to α1-antichymotrypsin and clusterin to be associated with AD diagnosis as well as the rate of cognitive decline, respectively [Citation55].
In theory, affinity-based assays suffer less from dynamic range-associated problems as well as missing data than MS. However, proteins are being measured indirectly and measurement specificity can be influenced by e.g. off-target binding. A comparative study evaluating the performance of the three proteomic platforms SomaLogic SomaScan, Olink proximity extension assay, and TMT-based MS in both AD CSF and plasma explored the advantages of each technology and showed that, by using a cross-platform approach, a proteomic analysis of unprecedented depth could be achieved for both biofluids [Citation58].
5. Conclusion
To date, several clinical proteomic studies of AD have been published, mostly in CSF and a smaller number in blood. Most studies are based on the TMT technique, but lately, following the technological advances in LC-MS instrumentation, label-free proteomics is increasingly applied in clinical studies. In these studies, several new biomarker candidates have been identified that could potentially reflect different pathological processes in AD and monitor disease progression. Targeted MS assays have been established that are helping to validate these biomarker candidates and, in a few, support diagnosis in the clinical laboratory. In addition, affinity-based proteomics platforms have demonstrated great utility in quantifying disease-relevant proteins in both CSF and blood, providing complementary value to MS-based techniques.
6. Expert opinion
Development of new instrumentation, analytical strategies, and bioinformatic methods is driving proteomics in clinical research of AD, resulting in a steady increase in the number of studies per year over the last two decades (). Alongside LC-MS-based methodology, the new generation of ultrasensitive affinity-based techniques is having an impact on the identification of new biomarker candidates.
Figure 1. Annual number of publications on proteomics studies of AD until 2022 (PubMed search for “Proteomic” AND “Alzheimer’s”). Total number of publications: 3169.
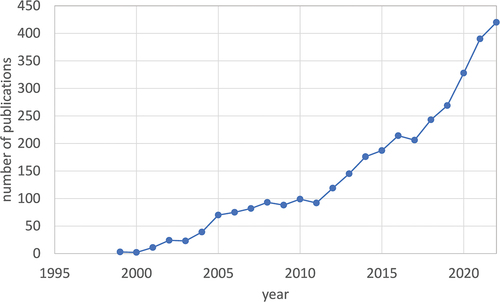
The majority of these studies are performed in CSF but proteomics studies of plasma and serum are likely to increase in number soon for several reasons. One is that sampling of CSF by lumbar puncture is perceived as being invasive and while the risk of severe adverse effects is very low, post-lumbar headache occurs in a fraction of cases [Citation59]. Furthermore, lumbar puncture is not performed at primary care units but only in specialized memory clinics, limiting the access to CSF. Therefore, the identification of blood biomarkers of AD is an important goal, particularly as disease-modifying treatments are likely to become available in the next few years. Several of the CSF biomarkers show measurable changes in plasma or serum, such as different p-tau forms [Citation60–65], amyloid-β 1–42/1–40 [Citation66], and neurofilament light polypeptide [Citation67,Citation68]. Although their concentrations are significantly lower in blood than in CSF, their presence and biomarker performance indicate feasibility of identifying other biomarkers that may be of clinical use. Such markers could serve as a first test to screen risk groups at primary care centers, and individuals testing positive could be followed up by CSF biomarker analysis and/or PET imaging [Citation69].
While numerous candidate biomarkers have been identified via proteomic approaches, the next steps, encompassing validation and characterization of these markers, typically require many further studies – a lengthy process that has created a bottleneck for the development of clinically useful biomarkers (). This problem is being addressed in different ways, both on the discovery and validation level. On the discovery level, the design of larger exploratory studies, made possible, e.g. by increased TMT multiplexing, can raise confidence in the identified candidate biomarkers. Current explorative studies comprise several hundreds of samples, and this number can be expected to increase as instruments and analytical protocols continue to be developed.
Figure 2. Biomarker development is comprised of three stages: the initial discovery phase involves the use of proteomic techniques to identify a multitude of candidate markers. These proteins then enter the verification stage, in which targeted MS-based methods such as PRM and SRM or immunoassays are established and used to replicate previous results and further characterize the markers by studying them in other cohorts. While the initial discovery phase is performed comparatively fast, sometimes yielding hundreds of potential candidates, the subsequent verification and validation stage requires significantly more time, creating a bottleneck. Protein candidates, which have been successfully validated in the second stage of the pipeline, can be employed for research purposes. Their clinical utility, however, remains to be evaluated in the third and final stage, the clinical validation phase.
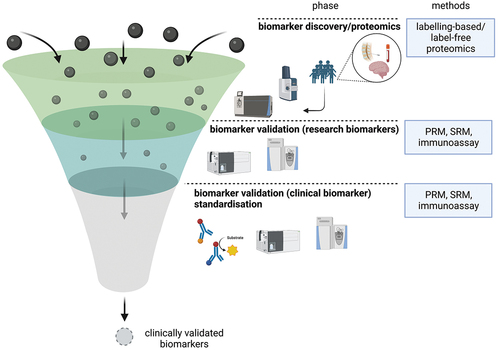
Furthermore, more well-characterized study populations are also improving the quality of the candidate markers. For example, the rapid development of amyloid- and tau-positron emission tomography (PET) imaging technology in the last decade has made it possible to detect plaque and tangle pathology in vivo. By combining proteomic and PET imaging data it is thus possible to correlate protein changes in CSF directly to measurable pathological changes in vivo instead of to a clinical diagnosis. Importantly, these imaging techniques also enable studies of AD in pre-symptomatic patients, which may lead to the detection of early AD-associated changes in CSF proteins. Such studies have the potential to discover new drug targets as well as early biomarkers that could help identify individuals at risk of developing AD before extensive neurodegeneration has occurred. Integrating proteomic data with imaging data as well as other sources such as expression analysis and genome-wide screening is an important topic in bioinformatics and will likely be an area that will develop over the next years.
Beyond the discovery level, targeted MS is speeding up biomarker validation in clinical studies due to the rapidity with which fit-for-purpose assays can be established compared with immunoassays. While SRM and PRM are the currently dominating methods because of their high detection sensitivity and specificity, an increasing number of studies are exploring the use of DIA, which has the potential to monitor a much larger number of proteins in parallel.
Another important area of use for LC-MS is as reference method, due to the high specificity of detection afforded by precise molecular mass measurement, and the unbiased quantification achieved by counting analyte ions relative to a stable-isotope-labeled standard. Reference methods are used together with reference materials to harmonize measurements of routine clinical assays. With the deployment of several new biomarkers to monitor AD in patients in clinical settings, it is likely that there will also be LC-MS-based reference methods developed for several of these biomarkers in the next few years.
The application of biomarkers for routine patient diagnostics is likely to increase soon with the recent successful development of disease-modifying treatments. While proteomics technologies are having a great impact on biomarker discovery, characterization, and validation, they have not yet made their way into the clinical routine laboratory on a large scale. This area is so far dominated by immunoassays with which many AD biomarkers can be routinely measured both in CSF and blood, rapidly and with high reproducibility. Nevertheless, LC-MS has potential advantages that might make it a competitive technique also in this area, one being its high specificity of detection, enabling selective measurement of modified or processed forms of proteins that may not be possible to distinguish using antibody-based detection. Another advantage of LC-MS is the ability to measure many targets in the same analysis run, which saves time and sample volume.
To realize the full potential of LC-MS, however, some limitations of the technique need to be overcome. One is the lack of robustness of the LC and the LC-MS interface: to achieve sufficient detection sensitivity, measurement of AD biomarkers by LC-MS often requires the use of nano-flow HPLC and nano-ESI; these techniques are still prone to interruptions and sensitive to sample impurities. The performance robustness of high-resolution TOF or Orbitrap mass spectrometers is also an issue; contamination of the ion optics by the sample matrix components may cause a multitude of performance issues that can be hard to diagnose and fix, making it difficult to meet the stringent quality criteria and response times for clinical analyses. In the next five to ten years, we will likely see the development of instrumentation that is more robust and easier to maintain and operate, which will enable a wider use of LC-MS for AD biomarker analysis in clinical routine settings.
Article highlights
Large-scale clinical proteomic studies play an important role in the field of AD, identifying biomarkers useful for clinical research and furthering our understanding of the disease pathophysiology.
To date, both labeling-based and label-free proteomics studies have been performed to study the CSF and plasma proteome of AD patients, with most studies employing TMT-based approaches.
Recent technological advances in label-free proteomics hold promise to conduct ultra-sensitive large clinical studies within short time frames, demonstrating unparalleled ion sampling capabilities.
Targeted mass spectrometry, capable of multiplexing dozens of analytes for a single analysis, is used to validate biomarker candidates in a time-efficient manner and has potential as a reference method in the context of biomarker standardization.
MS-based as well as affinity-based proteomics techniques are widely employed in clinical AD research; however, their application in clinical laboratory practice is very limited.
To further advance into a clinical routine setting, LC-MS instrumentation needs to become more robust.
Declaration of interest
H Zetterberg has served at scientific advisory boards and/or as a consultant for AbbVie, Acumen, Alector, Alzinova, ALZPath, Annexon, Apellis, Artery Therapeutics, AZTherapies, CogRx, Denali, Eisai, Nervgen, Novo Nordisk, Optoceutics, Passage Bio, Pinteon Therapeutics, Prothena, Red Abbey Labs, reMYND, Roche, Samumed, Siemens Healthineers, Triplet Therapeutics, and Wave, has given lectures in symposia sponsored by Cellectricon, Fujirebio, Alzecure, Biogen, and Roche, and is a co-founder of Brain Biomarker Solutions in Gothenburg AB (BBS), which is a part of the GU Ventures Incubator Program (outside submitted work).
K Blennow has served as a consultant and at advisory boards for Acumen, ALZPath, BioArctic, Biogen, Eisai, Lilly, Moleac Pte. Ltd, Novartis, Ono Pharma, Prothena, Roche Diagnostics, and Siemens Healthineers; has served at data monitoring committees for Julius Clinical and Novartis; has given lectures, produced educational materials and participated in educational programs for AC Immune, Biogen, Celdara Medical, Eisai and Roche Diagnostics; and is a co-founder of Brain Biomarker Solutions in Gothenburg AB (BBS), which is a part of the GU Ventures Incubator Program, outside the work presented in this paper.
The authors have no other relevant affiliations or financial involvement with any organization or entity with a financial interest in or financial conflict with the subject matter or materials discussed in the manuscript. This includes employment, consultancies, honoraria, stock ownership or options, expert testimony, grants or patents received or pending, or royalties.
Reviewer disclosures
Peer reviewers on this manuscript have no relevant financial or other relationships to disclose.
Additional information
Funding
References
- 2022 Alzheimer’s disease facts and figures. Alzheimers Dement. 2022;18(4):700–789. doi: 10.1002/alz.12638
- Alzheimer A, Stelzmann RA, Schnitzlein HN, et al. An English translation of Alzheimer’s 1907 paper, “Uber eine eigenartige Erkankung der Hirnrinde”. Clin Anat. 1995;8(6):429–431. doi: 10.1002/ca.980080612
- Masters CL, Simms G, Weinman NA, et al. Amyloid plaque core protein in Alzheimer disease and down syndrome. Proc Natl Acad Sci U S A. 1985;82(12):4245–4249. doi: 10.1073/pnas.82.12.4245
- Grundke-Iqbal I, Iqbal K, Tung Y-C, et al. Abnormal phosphorylation of the microtubule-associated protein tau (tau) in Alzheimer cytoskeletal pathology. Proc Nat Acad Sci. 1986;83(13):4913–4917. doi: 10.1073/pnas.83.13.4913
- James BD, Wilson RS, Boyle PA, et al. TDP-43 stage, mixed pathologies, and clinical Alzheimer’s-type dementia. Brain. 2016;139(11):2983–2993. doi: 10.1093/brain/aww224
- Liu CC, Liu CC, Kanekiyo T, et al. Apolipoprotein E and Alzheimer disease: risk, mechanisms and therapy. Nat Rev Neurol. 2013;9(2):106–118. doi: 10.1038/nrneurol.2012.263
- Hardy JA, Higgins GA. Alzheimer’s disease: the amyloid cascade hypothesis. Science. 1992;256(5054):184–185. doi: 10.1126/science.1566067
- McDade E, Cummings JL, Dhadda S, et al. Lecanemab in patients with early Alzheimer’s disease: detailed results on biomarker, cognitive, and clinical effects from the randomized and open-label extension of the phase 2 proof-of-concept study. Alzheimers Res Ther. 2022;14(1):191. doi: 10.1186/s13195-022-01124-2
- Mintun MA, Lo AC, Duggan Evans C, et al. Donanemab in early Alzheimer’s disease. N Engl J Med. 2021;384(18):1691–1704. doi: 10.1056/NEJMoa2100708
- Swanson CJ, Zhang Y, Dhadda S, et al. A randomized, double-blind, phase 2b proof-of-concept clinical trial in early Alzheimer’s disease with lecanemab, an anti-Abeta protofibril antibody. Alzheimers Res Ther. 2021;13(1):80. doi: 10.1186/s13195-021-00813-8
- Van Dyck CH, Swanson CJ, Aisen P, et al. Lecanemab in early Alzheimer’s disease. N Engl J Med. 2023;388(1):9–21. doi: 10.1056/NEJMoa2212948
- Blennow K, Dubois B, Fagan AM, et al. Clinical utility of cerebrospinal fluid biomarkers in the diagnosis of early Alzheimer’s disease. Alzheimers Dement. 2015;11(1):58–69. doi: 10.1016/j.jalz.2014.02.004
- Gonzalez-Ortiz F, Turton M, Kac PR, et al. Brain-derived tau: a novel blood-based biomarker for Alzheimer’s disease-type neurodegeneration. Brain. 2023;146(3):1152–1165. doi: 10.1093/brain/awac407
- Karikari TK, Ashton NJ, Brinkmalm G, et al. Blood phospho-tau in Alzheimer disease: analysis, interpretation, and clinical utility. Nat Rev Neurol. 2022;18(7):400–418. doi: 10.1038/s41582-022-00665-2
- Geyer PE, Kulak NA, Pichler G, et al. Plasma proteome profiling to Assess human Health and disease. Cell Syst. 2016;2(3):185–195. doi: 10.1016/j.cels.2016.02.015
- Liu Y, Buil A, Collins BC, et al. Quantitative variability of 342 plasma proteins in a human twin population. Mol Syst Biol. 2015;11(1):786. doi: 10.15252/msb.20145728
- Schilde LM, Kösters S, Steinbach S, et al. Protein variability in cerebrospinal fluid and its possible implications for neurological protein biomarker research. PLoS One. 2018;13(11):e0206478. doi: 10.1371/journal.pone.0206478
- Anderson NL, Anderson NG. The human plasma proteome: history, character, and diagnostic prospects. Mol & Cell Proteomics. 2002;1(11):845–867. doi: 10.1074/mcp.R200007-MCP200
- McAlister GC, Huttlin EL, Haas W, et al. Increasing the multiplexing capacity of TMTs using reporter ion isotopologues with isobaric masses. Anal Chem. 2012;84(17):7469–7478. doi: 10.1021/ac301572t
- Li J, Cai Z, Bomgarden RD, et al. Tmtpro-18plex: the expanded and complete set of TMTpro reagents for sample multiplexing. J Proteome Res. 2021;20(5):2964–2972. doi: 10.1021/acs.jproteome.1c00168
- Batth TS, Francavilla C, Olsen JV. Off-line high-Ph reversed-phase fractionation for in-depth phosphoproteomics. J Proteome Res. 2014;13(12):6176–6186. doi: 10.1021/pr500893m
- Pfammatter S, Bonneil E, McManus FP, et al. A novel differential ion mobility device expands the depth of proteome coverage and the sensitivity of Multiplex proteomic measurements. Mol & Cell Proteomics. 2018;17(10):2051–2067. doi: 10.1074/mcp.TIR118.000862
- Dey KK, Wang H, Niu M, et al. Deep undepleted human serum proteome profiling toward biomarker discovery for Alzheimer’s disease. Clin Proteomics. 2019;16(1):16. doi: 10.1186/s12014-019-9237-1
- Higginbotham L, Ping L, Dammer EB, et al. Integrated proteomics reveals brain-based cerebrospinal fluid biomarkers in asymptomatic and symptomatic Alzheimer’s disease. Sci Adv. 2020;6(43). doi: 10.1126/sciadv.aaz9360
- Johnson ECB, Carter EK, Dammer EB, et al. Large-scale deep multi-layer analysis of Alzheimer’s disease brain reveals strong proteomic disease-related changes not observed at the RNA level. Nat Neurosci. 2022;25(2):213–225. doi: 10.1038/s41593-021-00999-y
- Johnson ECB, Dammer EB, Duong DM, et al. Large-scale proteomic analysis of Alzheimer’s disease brain and cerebrospinal fluid reveals early changes in energy metabolism associated with microglia and astrocyte activation. Nat Med. 2020;26(5):769–780. doi: 10.1038/s41591-020-0815-6
- Johnson ECB, Dammer EB, Duong DM, et al. Deep proteomic network analysis of Alzheimer’s disease brain reveals alterations in RNA binding proteins and RNA splicing associated with disease. Mol Neurodegener. 2018;13(1):52. doi: 10.1186/s13024-018-0282-4
- Ping L, Kundinger SR, Duong DM, et al. Global quantitative analysis of the human brain proteome and phosphoproteome in Alzheimer’s disease. Sci Data. 2020;7(1):315. doi: 10.1038/s41597-020-00650-8
- Sathe G, Na CH, Renuse S, et al. Quantitative proteomic profiling of cerebrospinal fluid to identify candidate biomarkers for Alzheimer’s disease. Proteomics Clin Appl. 2019;13(4):e1800105. doi: 10.1002/prca.201800105
- Tijms BM, Gobom J, Reus L, et al. Pathophysiological subtypes of Alzheimer’s disease based on cerebrospinal fluid proteomics. Brain. 2020;143(12):3776–3792. doi: 10.1093/brain/awaa325
- Roche S, Gabelle A, Lehmann S. Clinical proteomics of the cerebrospinal fluid: Towards the discovery of new biomarkers. Proteomics Clin Appl. 2008;2(3):428–436. doi: 10.1002/prca.200780040
- Konijnenberg E, Tijms BM, Gobom J, et al. APOE epsilon4 genotype-dependent cerebrospinal fluid proteomic signatures in Alzheimer’s disease. Alzheimers Res Ther. 2020;12(1):65. doi: 10.1186/s13195-020-00628-z
- Wang H, Robinson JL, Kocabas P, et al. Genome-scale metabolic network reconstruction of model animals as a platform for translational research. Proc Natl Acad Sci U S A. 2021;118(30). doi: 10.1073/pnas.2102344118
- Nilsson J, Cousins KAQ, Gobom J, et al. A novel antibody-free mass spectrometry panel of CSF biomarkers for synaptic dysfunction. Alzheimer’s Dementia. 2021;17(S5):e054818. doi: 10.1002/alz.054818
- Nilsson J, Cousins KAQ, Gobom J, et al. Cerebrospinal fluid biomarker panel of synaptic dysfunction in Alzheimer’s disease and other neurodegenerative disorders. Alzheimers Dement. 2023;19(5):1775–1784. doi: 10.1002/alz.12809
- Nilsson J, Gobom J, Sjodin S, et al. Cerebrospinal fluid biomarker panel for synaptic dysfunction in Alzheimer’s disease. Alzheimers Dement (Amst). 2021;13(1):e12179. doi: 10.1002/dad2.12179
- Tsai CF, Smith JS, Krajewski K, et al. Tandem mass tag labeling facilitates reversed-phase liquid chromatography-mass spectrometry analysis of hydrophilic phosphopeptides. Anal Chem. 2019;91(18):11606–11613. doi: 10.1021/acs.analchem.9b01814
- Megger DA, Bracht T, Meyer HE, et al. Label-free quantification in clinical proteomics. Biochim Biophys Acta. 2013;1834(8):1581–1590. doi: 10.1016/j.bbapap.2013.04.001
- Zhang F, Ge W, Ruan G, et al. Data-independent acquisition mass spectrometry-based proteomics and software tools: a glimpse in 2020. Proteomics. 2020;20(17–18):e1900276. doi: 10.1002/pmic.201900276
- Bader JM, Geyer PE, Müller JB, et al. Proteome profiling in cerebrospinal fluid reveals novel biomarkers of Alzheimer’s disease. Mol Syst Biol. 2020;16(6):e9356. doi: 10.15252/msb.20199356
- Andreev VP, Petyuk VA, Brewer HM, et al. Label-free quantitative LC-MS proteomics of Alzheimer’s disease and normally aged human brains. J Proteome Res. 2012;11(6):3053–3067. doi: 10.1021/pr3001546
- Meier F, Brunner AD, Frank M, et al. diaPASEF: parallel accumulation-serial fragmentation combined with data-independent acquisition. Nat Methods. 2020;17(12):1229–1236. doi: 10.1038/s41592-020-00998-0
- Heil LR, Damoc E, Arrey TN, et al. Evaluating the performance of the Astral mass analyzer for quantitative proteomics using data independent acquisition. bioRxiv, (2023).
- Meng Z, Veenstra TD. Targeted mass spectrometry approaches for protein biomarker verification. J Proteomics. 2011;74(12):2650–2659. doi: 10.1016/j.jprot.2011.04.011
- Barthélemy NR, Li Y, Joseph-Mathurin N, et al. A soluble phosphorylated tau signature links tau, amyloid and the evolution of stages of dominantly inherited Alzheimer’s disease. Nat Med. 2020;26(3):398–407. doi: 10.1038/s41591-020-0781-z
- Gobom J, Benedet AL, Mattsson-Carlgren N, et al. Antibody-free measurement of cerebrospinal fluid tau phosphorylation across the Alzheimer’s disease continuum. Mol Neurodegener. 2022;17(1):81. doi: 10.1186/s13024-022-00586-0
- Montoliu-Gaya L, Benedet AL, Tissot C, et al. Mass spectrometric simultaneous quantification of tau species in plasma shows differential associations with amyloid and tau pathologies. Nat Aging. 2023;3(6):661–669. doi: 10.1038/s43587-023-00405-1
- Weiner S, Sauer M, Brinkmalm G, et al. SCRN1: a cerebrospinal fluid biomarker correlating with tau in Alzheimer’s disease. Alzheimers Dement. 2023. doi: 10.1002/alz.13042
- Zhou M, Haque RU, Dammer EB, et al. Targeted mass spectrometry to quantify brain-derived cerebrospinal fluid biomarkers in Alzheimer’s disease. Clin Proteomics. 2020;17(1):19. doi: 10.1186/s12014-020-09285-8
- Brand AL, Lawler PE, Bollinger JG, et al. The performance of plasma amyloid beta measurements in identifying amyloid plaques in Alzheimer’s disease: a literature review. Alzheimer’s Res Ther. 2022;14(1):195. doi: 10.1186/s13195-022-01117-1
- Ovod V, Ramsey KN, Mawuenyega KG, et al. Amyloid β concentrations and stable isotope labeling kinetics of human plasma specific to central nervous system amyloidosis. Alzheimers Dement. 2017;13(8):841–849. doi: 10.1016/j.jalz.2017.06.2266
- Kirmess KM, Meyer MR, Holubasch MS, et al. The PrecivityAD™ test: accurate and reliable LC-MS/MS assays for quantifying plasma amyloid beta 40 and 42 and apolipoprotein E proteotype for the assessment of brain amyloidosis. Clin Chim Acta. 2021;519:267–275.
- Carlyle BC, Kitchen RR, Mattingly Z, et al. Technical performance evaluation of olink proximity extension assay for blood-based biomarker discovery in longitudinal studies of alzheimer’s disease. Front Neurol. 2022;13:889647doi: 10.3389/fneur.2022.889647
- Whelan CD, Mattsson N, Nagle MW, et al. Multiplex proteomics identifies novel CSF and plasma biomarkers of early Alzheimer’s disease. Acta Neuropathol Commun. 2019;7(1):169. doi: 10.1186/s40478-019-0795-2
- Sattlecker M, Kiddle SJ, Newhouse S, et al. Alzheimer’s disease biomarker discovery using SOMAscan multiplexed protein technology. Alzheimers Dement. 2014;10(6):724–734. doi: 10.1016/j.jalz.2013.09.016
- Shi L, Westwood S, Baird AL, et al. Discovery and validation of plasma proteomic biomarkers relating to brain amyloid burden by SOMAscan assay. Alzheimers Dement. 2019;15(11):1478–1488. doi: 10.1016/j.jalz.2019.06.4951
- Feng W, Beer J, Hao Q, et al. NULISA: a novel proteomic liquid biopsy platform with attomolar sensitivity and high multiplexing. bioRxiv. 2023. doi: 10.1101/2023.04.09.536130
- Dammer EB, Ping L, Duong DM, et al. Multi-platform proteomic analysis of Alzheimer’s disease cerebrospinal fluid and plasma reveals network biomarkers associated with proteostasis and the matrisome. Alzheimer’s Res Ther. 2022;14(1):174. doi: 10.1186/s13195-022-01113-5
- Costerus JM, Brouwer MC, van de Beek D. Technological advances and changing indications for lumbar puncture in neurological disorders. Lancet Neurol. 2018;17(3):268–278. doi: 10.1016/S1474-4422(18)30033-4
- Ashton NJ, Pascoal TA, Karikari TK, et al. Plasma p-tau231: a new biomarker for incipient Alzheimer’s disease pathology. Acta Neuropathol. 2021;141(5):709–724. doi: 10.1007/s00401-021-02275-6
- Janelidze S, Berron D, Smith R, et al. Associations of plasma phospho-Tau217 levels with tau positron emission tomography in early Alzheimer disease. JAMA Neurol. 2021;78(2):149–156. doi: 10.1001/jamaneurol.2020.4201
- Karikari TK, Benedet AL, Ashton NJ, et al. Diagnostic performance and prediction of clinical progression of plasma phospho-tau181 in the Alzheimer’s disease Neuroimaging Initiative. Mol Psychiatry. 2021;26(2):429–442. doi: 10.1038/s41380-020-00923-z
- Karikari TK, Pascoal TA, Ashton NJ, et al. Blood phosphorylated tau 181 as a biomarker for Alzheimer’s disease: a diagnostic performance and prediction modelling study using data from four prospective cohorts. Lancet Neurol. 2020;19(5):422–433. doi: 10.1016/S1474-4422(20)30071-5
- Lantero Rodriguez J, Karikari TK, Suárez-Calvet M, et al. Plasma p-tau181 accurately predicts Alzheimer’s disease pathology at least 8 years prior to post-mortem and improves the clinical characterisation of cognitive decline. Acta Neuropathol. 2020;140(3):267–278. doi: 10.1007/s00401-020-02195-x
- Mattsson-Carlgren N, Janelidze S, Bateman RJ, et al. Soluble P-tau217 reflects amyloid and tau pathology and mediates the association of amyloid with tau. EMBO Mol Med. 2021;13(6):e14022. doi: 10.15252/emmm.202114022
- Thijssen EH, Verberk IMW, Vanbrabant J, et al. Highly specific and ultrasensitive plasma test detects Abeta(1-42) and Abeta(1-40) in Alzheimer’s disease. Sci Rep. 2021;11(1):9736. doi: 10.1038/s41598-021-89004-x
- Giacomucci G, Mazzeo S, Bagnoli S, et al. Plasma neurofilament light chain as a biomarker of Alzheimer’s disease in subjective cognitive decline and mild cognitive impairment. J Neurol. 2022;269(8):4270–4280. doi: 10.1007/s00415-022-11055-5
- Lewczuk P, Ermann N, Andreasson U, et al. Plasma neurofilament light as a potential biomarker of neurodegeneration in Alzheimer’s disease. Alzheimers Res Ther. 2018;10(1):71. doi: 10.1186/s13195-018-0404-9
- Gonzalez-Ortiz F, Kac PR, Brum WS, et al. Plasma phospho-tau in Alzheimer’s disease: towards diagnostic and therapeutic trial applications. Mol Neurodegener. 2023;18(1):18. doi:10.1186/s13024-023-00605-8