ABSTRACT
RNA binding proteins mediate global regulation at the level of transcriptome and translatome of a cell. We studied the global level expression changes regulated by IMP3 in transcriptome and translatome by performing microarray using total cellular RNA and heavy polysome derived RNA of IMP3 silenced glioma cells respectively. Differentially regulated transcripts at the transcriptome level (n = 2388) and at the level of translatome (n = 479) were identified. Further, these transcripts were classified as direct and indirect targets on the basis of presence of IMP3 binding site. Additional investigation revealed that direct targets at transcriptome level were found to be associated with processes related to cell cycle, whereas direct targets at the translatome level participated in apoptosis related pathways. Probable mechanism of indirect regulation at both the levels is also investigated. Collectively, our study reveals multi-level gene expression regulation imposed by IMP3 in glioma cells.
Introduction
Gene expression is determined by concerted effects of transcriptional regulation, post transcriptional regulation and post translational regulation. Cancer cells hijack the normal cellular processes of RNA metabolism to alter the proliferation, apoptosis, migration and invasion of these cells. Various steps in the RNA metabolism include mRNA transcription, localization, stability and translation. Post transcriptional regulation is imposed by cis-acting elements (regulatory elements on mRNA) and trans-acting factors (ncRNAs and RBPs).
mRNA stability and translation are regulated by synergistic or antagonistic effects of ncRNA and RBPs. Mutations in regulatory sequences in mRNA, deregulation or aberrant activity of RBPs and trans-acting factors are being established to contribute to carcinogenesis.Citation1 Furthermore, collaborative effect of RBPs in regulating RNA stability and translation has been increasingly documented.Citation2-5 Many RBPs have been reported to determine the steady-state levels of RNAs by controlling the rate of transcript degradation. Several oncogenic transcripts are protected from degradation by binding of these trans-factors to their respective sites in UTRs. Translation control has also been established as an important regulator of cancerous state of cells. Proteins are considered as effectors of gene function, and contribute directly in regulation of phenotypes. Merely 40% of the variation in the protein expression can be explained by mRNA levels, indicating the importance of translation.Citation6 The role of translation in cancers has been clearly documented, where it has been shown to contribute in growth and apoptosis related processes.Citation5 Few RBPs like PABP1 bind to poly (A) tail of mRNA and also interact with translation initiation factor eIF4G. This interaction bridges the 3′ and 5′ end of the mRNA, thus facilitating translation and protecting mRNA degradation.Citation7 Another example of an RBP regulating plethora of cellular targets by determining their mRNA stability and translation is HuR.Citation2,4,8 IMP3 is yet another example of an oncofetal RNA binding protein, which has been associated with various malignancies.Citation9-14 Few of the bonafide targets of the protein are emerging from the recent research. These targets include CCND1, EIF4EBP, MYC, SLUG, ABCG2 and IGF2.Citation3,15-20 Some of these target proteins are regulated at the level of RNA stability, while others are regulated at the level of translation.
These findings inspired us to explore the effects of IMP3 on global transcriptome and translatome of glioma cells. In this study, we have performed global gene expression profiling on IMP3 knockdown and identified genes that are regulated at the level of transcript. We have also performed polysome profiling after depleting glioma cells of IMP3, and by performing an integrated analysis, we identified targets regulated by IMP3 only at the level of translation. Moreover, incorporating the information about IMP3 binding sites from literature in our analysis, we also revealed genes getting directly regulated by IMP3 in transcriptome and translatome of glioma cells.
Results
Overall work-flow used to unravel the global level regulation of transcriptome and translatome by IMP3
RNA binding proteins may have global effects both on transcriptome and translatome.Citation2-5 The number of putative targets of IMP3 (n = ∼10,000 genes) as indicated by Hafner et al.,Citation21 suggests the possible magnitude of global regulation executed by IMP3. To assess the effect of IMP3 on transcriptome, we had performed transcriptome profiling (using total RNA) of IMP3 silenced U251 glioma cells (siIMP3) and control silenced U251 glioma cells (siControl) using microarray (). The differentially expressed genes (Log2 ratio ≤ −0.58 and ≥ 0.58, p < 0.05) were identified. Further, we classified the differentially expressed genes as direct and indirect targets as per the presence or absence of IMP3 binding sites (as per PAR-CLIP data derived from Hafner et al., 2010) (). We were also interested in investigating the significantly affected transcripts at the level of translation by IMP3. To achieve this aim, RNA from heavy polysome fractions of IMP3 depleted U251 cells and siControl cells were subjected to microarray analysis (). The extent of translation level changes termed as ‘translation efficiency’ was measured by calculating H /T ratio [ratio of differential intensity of a gene in a heavy polysome fraction (H) (siControl vs. siIMP3) and differential intensity of a gene in a total RNA (T) (siControl vs. siIMP3)]. The following strategy was adopted to find the list of mRNAs that are regulated by IMP3 only at the level of translation. In the first step, we selected those mRNAs that are not altered at the level of transcripts (Log2 ratio ≥ −0.58 and ≤ 0.58, p > 0.05; p ≤ 0.05) derived from the analysis of total RNA (siControl vs. siIMP3). Secondly, the differentially regulated genes at the level of translation (Log2 ratio ≤ −0.58 and ≥ 0.58, p < 0.05), derived from the analysis of heavy polysome bound RNA (siControl vs. siIMP3) were taken. Next, from these 2 lists, genes which were differentially regulated at the level of translation, but not regulated at the level of transcript were identified. For these genes, H/T ratios were calculated and only those genes having Log2 ratio ≤ −1 and ≥ 1 were selected. These genes are regulated by IMP3 exclusively at the level of translation, without having any effect on the levels of their transcripts. Direct and indirect translation targets of IMP3 were also identified on the basis of the presence or absence of IMP3 binding sites (as per PAR-CLIP data derived from Hafner et al., 2010) (). Taken together, using an integrated approach of global profiling total RNA and heavy polysome RNA and a thorough bioinformatics analysis, we identified the IMP3 modulated transcriptome and translatome.
Figure 1. Data analysis strategy used to investigate the effects of IMP3 on transcriptome and translatome in glioma cells. Differentially expressed genes in transcriptome were identified (log2 ratio ≥ 0.58 or ≤−0.58). Out of these, genes having IMP3 binding sites were termed as ‘direct targets’, while those with no binding sites were termed as ‘indirect targets’. Direct targets may be possibly regulated at the level of RNA stability, while indirect targets may be secondary targets of IMP3. To identify genes only regulated at the level of translation, we had taken unregulated genes at the transcript level (Log2 ratio between 0.58 and −0.58) and checked for the differentially regulated genes at the translation level. Again, direct and indirect targets on the basis of presence and absence of IMP3 binding sites were identified. Here, direct targets may be regulated at the level of translation, while indirect targets may be regulated as secondary effects.
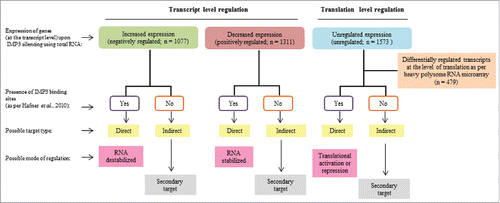
Regulation of global transcriptome by IMP3
We first went ahead to investigate the role of IMP3 in regulation of transcriptome in GBM cells. Global profiling of transcript levels was performed using microarray in U251 control cells (siControl) and in IMP3 silenced cells (siIMP3). Differential Log2 ratios of transcript levels of genes, whose intensity was detected with significance in microarray, were calculated. Microarray analysis revealed that 2388 genes had significant differential expression in these 2 conditions. 1077 genes were upregulated in IMP3 depleted cells, indicating a possible negative regulation imposed by IMP3 on these genes, while 1311 genes were downregulated, implying a positive regulation by IMP3 on the transcript level of these genes (, Supplementary Table 1). To identify the subset of differentially regulated transcripts that may be regulated by IMP3 in GBM tumors; we checked for the correlation between expressions of these genes with IMP3 transcript levels by using microarray (Agilent platform) data from TCGA. Out of the 2388 differentially regulated genes, the gene expression data for 1940 genes (873 upregulated genes and 1067 genes from downregulated genes in microarray performed) was available in TCGA. Genes having significant correlation with IMP3 transcript (p-value < 0.05) and having correlation coefficient above and below 0.1 and −0.1 (r ≥ 0.1 and r ≤ −0.1) respectively were considered. The genes which were upregulated in IMP3 silenced condition, were analyzed for negative correlation with IMP3 transcript levels in TCGA GBM data. Our analysis revealed that 220 of these 873 upregulated transcripts showed a significant negative correlation coefficient (r ≤ −0.1) in GBM tumors (; red dots). As these targets are expected to be downregulated in GBM tumors, they were termed as “IMP3_down.” Conversely, 308 of 1077 downregulated transcripts showed a significant positive correlation with IMP3 transcript in GBM tumors (r ≥ 0.1) (; green dots). These targets are expected to be upregulated in GBM tumors, hence are termed as “IMP3_up.” To delineate the possible mechanism of regulation for the differentially expressed genes under IMP3 silenced conditions, we checked for the presence of IMP3 binding sites on these transcripts. We hypothesize that the transcripts harbouring putative IMP3 binding sites on their RNA have a higher probability of being a “direct target." These direct targets may be positively or negatively regulated by IMP3 at the level of RNA stability. The differentially regulated genes which do not have IMP3 binding sites may be regulated by secondary effects and were thus termed as “indirect targets.” For this analysis, we acquired the IMP3 binding site information from a published data set (GSE21918).Citation21 We found a larger proportion of genes to be direct targets in both the differentially regulated groups (78.5% for IMP3_down genes and 79.9% for IMP3_up genes) ( and ). Thus, we have identified targets of IMP3 regulated at the level of transcriptome in GBM tumors.
Figure 2. IMP3 transcriptomic targets in U251 cells and GBM tumors. a. Volcano graph depicting the differentially regulated genes in IMP3 knockdown condition. The black dotted vertical lines and horizontal line represent Log2 ratio −0.58 and 0.58 expression cut-off values and p-value < 0.05 respectively applied to identify the significantly differentially regulated genes in IMP3 silenced condition. Red dots represent the significantly upregulated genes, while green dots represent the significantly downregulated genes. Black dots depict the non-significant genes. b. Graph representing correlation coefficient values of IMP3 transcriptomic target transcripts with IMP3 transcripts (x-axis) and expression values (y-axis) of upregulated and downregulated genes upon IMP3 silencing. Each dot represents an IMP3 target gene. Dotted line in quadrants discriminate the non-correlating (black dots) and correlating dots (colored) after applying 0.1 correlation coefficient for the analysis. The colored dots in each quadrant represent significantly correlated genes in GBM tumors. Upregulated and negatively correlating targets upon IMP3 silencing are represented in red, while downregulated and positively correlating targets are represented in green. c,d. Proportion of IMP3_down (negatively regulated by IMP3) (c) and IMP3_up (positively regulated by IMP3) (d) Genes classified as direct and indirect targets of IMP3 as per the binding site information from PAR-CLIP data. e,f. Venn diagrams depicting distribution of IMP3 binding sites on 5′UTR, coding sequence (CDS) and 3′UTR on IMP3_down (e) and IMP3_up (f) target transcripts. The number and percentage of transcripts falling in each category are mentioned along with the region of the transcript represented. Venn diagram was drawn using http://bioinformatics.psb.ugent.be/webtools/Venn/.
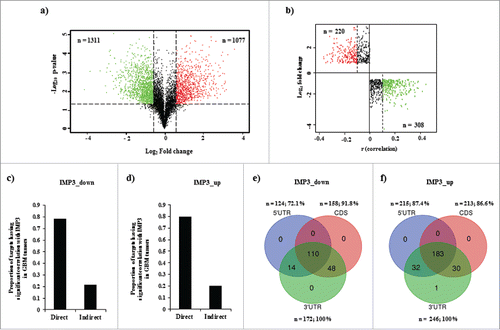
The binding sites at 5′UTR and 3′UTR are implicated in regulation of RNA stability and translation of various targets by RBPs.Citation1, 22, 23 Hence, the distribution of IMP3 binding sites on the regulated transcripts was also investigated. We observed that the distribution of the target sites on the IMP3_down target transcripts was: 72.1% at 5′UTR, 91.8 % at CDS and 100% at 3′UTR (). A similar trend was observed for IMP3_up targets also (87.4% at 5′UTR, 86.6 % at CDS and 100% at 3′UTR) (). Thus, a preference for 3′UTR sites on the regulated transcripts with IMP3 binding sites was observed ( and ). We also observed that some of these direct targets had multiple IMP3 binding sites, present together in both UTR regions, in either of 2 UTR region and CDS region, and in all the 3 together ( and ). Taken together, we observed that major proportion of differentially regulated genes harboured IMP3 binding sites.
To broadly understand the cellular functions altered by IMP3 modulated direct transcriptome targets (which significantly correlated with IMP3 transcript), we performed an unbiased functional enrichment analysis using DAVID (Database for Annotation, Visualization, and Integrated Discovery).Citation24 This analysis revealed that various biologic processes linked to cell cycle regulation were significantly enriched (, Supplementary Table 4). This observation was in coherence with our previously published results, where IMP3 silenced glioma cells showed reduced metabolically active cells and also showed diminished capacity of colony formation.Citation17 Among the IMP3 regulated targets that are enriched in cell cycle related pathways, we observed some of the established gene players in cell cycle regulation like AURKA, CDC25B, CDC25C, GTSE1 and CENPE. Validation of the expression profile of these genes in the total RNA of IMP3 depleted glioma cells using qRT-PCR revealed a similar downregulated expression pattern as observed in microarray (). Hence, in GBM tumors, they were expected to show positive correlation with IMP3 transcript as shown in . Thus, IMP3 regulated direct targets at the transcript level may play important role in cell proliferation of GBMs.
Figure 3. Enrichment of biologic processes in IMP3 modulated direct targets at the transcriptome level. (a) Enriched biologic processes in direct transcriptomic targets of IMP3 at transcript level as identified by DAVID. The grey colored bars correspond to the processes associated with cell cycle. (b) Transcript levels of mentioned genes in total RNA from IMP3 silenced U251 glioma cells relative to control silenced U251 cells as assessed by microarray and qRT-PCR. (c) Correlation graphs of AURKA, CDC25C, CDC25B, CENPE and GTSE1 transcript levels with IMP3 transcript level in GBM tumors using TCGA Agilent data set.
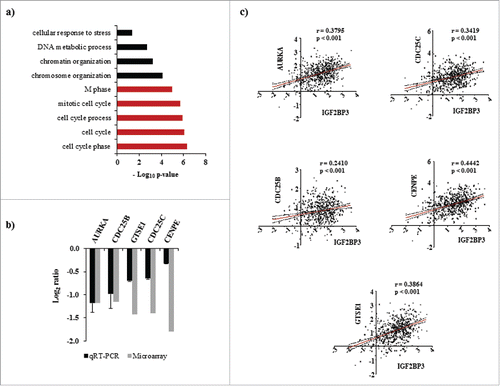
Regulation of global translation by IMP3
The varied number of transcripts regulated by IMP3 intrigued us to check if any regulation is imposed by the molecule at the level of translation. Moreover, literature and our own published work suggest that IMP3 regulates the translation of various molecules.Citation17, 25 To answer this question, we performed polysome analysis on cell lysates of IMP3 knockdown U251 cells (Figure S1a). The fractions collected were divided into monosome, light polysome and heavy polysome fractions. RNA from heavy polysome fraction was subjected to microarray (Figure S1b). We were interested in the genes solely affected at the translation level by IMP3. Thus, the genes unregulated at their transcript levels (from total RNA) in IMP3 depleted cells (n = 1573; Log2 ratio ≥ −0.58 and ≤ 0.58; p > 0.05, p ≤ 0.05; Supplementary Table S5), but showing significant differential expression in heavy polysome fraction (siControl vs. siIMP3) were selected (n = 916; Log2 ratio ≤ −0.58 and ≥ 0.58, p < 0.05). To further shortlist the genes, we calculated H/T ratios [Transcript expression in heavy polysome fraction (H) / transcript expression in total RNA (T)] of these genes. Transcripts with positive H/T ratios represent those undergoing heavy translation out of the total cellular pool of transcript in question. Genes having H/T ratios more than Log2 ratio 1 or less than Log2 ratio −1 (n = 479) were considered significant and selected for further investigation (, Table S6). 268 genes were upregulated and 220 genes showed downregulation in heavy polysome fraction of IMP3 silenced cells. We next wanted to analyze the direct and indirect targets of IMP3 at the level of translation. Again, transcripts which were modulated at the level of translation and containing the IMP3 binding sites were categorized as direct targets, while the ones without IMP3 binding sites were categorized as indirect targets ( and ). Out of 268 upregulated and 220 downregulated genes, 165 (64.1%) and 148 (70.4%) were found to be direct targets respectively ( and ). Differentially regulated direct and indirect target genes of IMP3 at the level of translation are enlisted in Supplementary Table 7 and 8 respectively. Similar to transcriptome data, further investigation revealed that direct targets had sites predominantly on the 3′UTR ( and ). Moreover, presence of multiple sites at different regions on the same transcript was observed in direct translation targets as well ( and ). 107 upregulated target transcripts and 109 downregulated target transcripts had sites on all the 3 regions, namely 5′UTR, CDS and 3′UTR ( and ).
Figure 4. Differentially regulated genes in IMP3 modulated translatome (a) Heat map representing differential H/T ratios of translationally differentially regulated genes upon IMP3 depletion in U251 cells. A dual-color code was used wherein red represents genes having H/T ratio ≥ 1, while green represents genes having H/T ratio ≤ −1. (b,c) Percentage of IMP3_down (negatively regulated by IMP3) (b) and IMP3_up (positively regulated by IMP3) (c) genes classified as direct and indirect targets of IMP3 as per the binding site information from PAR-CLIP data. (d,e) Venn diagrams depicting distribution of IMP3 binding sites on 5′UTR, coding sequence (CDS) and 3′UTR on IMP3_down (d) and IMP3_up (e) target transcripts. The number and percentage of transcripts falling in each category are mentioned along with the region of the transcript represented. Venn diagram was drawn using http://bioinformatics.psb.ugent.be/webtools/Venn//.
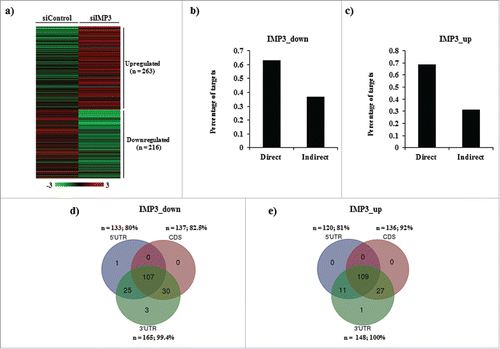
Figure 5. Enrichment of biologic processes in IMP3 modulated direct targets at the translatome level. (a) Enriched biologic processes in direct targets of IMP3 at the level of translation as identified by DAVID. The grey colored bars correspond to the processes associated with apoptosis and cell death. (b) Transcript levels of the mentioned cell death related genes in total RNA fraction of IMP3 silenced U251 cells relative (siIMP3) to the control cells (siControl) U251 cells as assessed using microarray and qRT-PCR. (c) H/T ratios as calculated in IMP3 silenced U251 cells of the same genes are depicted as a bar graph. The levels of these genes in heavy polysome fractions and total RNA from control and siIMP3 cells were assessed using microarray and qRT-PCR.
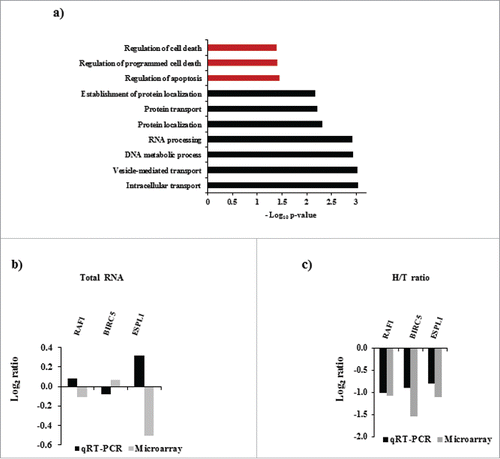
To broadly understand the cellular functions altered by IMP3 modulated direct translatome targets, we performed an unbiased functional enrichment analysis using DAVID (Database for Annotation, Visualization, and Integrated Discovery).Citation24 This analysis revealed that various biologic processes linked to regulation of apoptosis were significantly enriched (, Table S9). Several well-known apoptosis regulators like BIRC5, RAF1 and ESPL1 were downregulated in polysome fraction upon IMP3 silencing (), whereas their transcript levels were unaltered in total RNA as per our microarray analysis (). Assessment of the levels of these genes using qRT-PCR in heavy polysome fractions of the IMP3 silenced cells validated our results of the microarray ( and ). Additionally, we could provide protein level data for RAF1. By using protein data from Reverse Phase Protein Array (RPPA) from TCGA, we obtained RAF1 protein levels and correlated it with IMP3 transcript levels in GBM. This analysis revealed that RAF1 protein has significant positive correlation (p < 0.05; r = 0.22) with IMP3 transcript levels (Fig. S2a). RAF1 transcript levels were unaltered in IMP3 silenced cells, as seen in our microarray results suggesting that no regulation is imposed by IMP3 on RAF1 transcript in regulating its stability (). Our conclusion was validated by our observation that there was no significant difference in the RNA levels of RAF1 in IMP3 overexpressing or IMP3 silenced glioma cells as quantified using qRT-PCR (Fig. S2b and S2c). We further obtained additional evidence for RAF1 being a direct target of IMP3. Investigation of IMP3 PAR-CLIP data,Citation21 revealed a significantly higher enrichment of IMP3 bound reads on the 3′ UTR of the RAF1 transcript (Fig. S2d). These observations suggest that IMP3 may contribute in enhancing the translation of RAF1 transcript, without bringing any significant changes in transcript level of RAF1. Collectively, we identified genes that are exclusively regulated at the level of translation upon IMP3 depletion. Additionally, we also found that the regulation of cell death by IMP3 may be attributed to the direct targets regulated at this level. We also established RAF1 as a potential direct target of IMP3 regulated at the level of translation in GBM tumors.
Insights into the mechanism of regulation of indirect targets by IMP3
We were intrigued to explore the mechanisms by which IMP3 regulates the indirect targets. The indirect targets may be regulated at the secondary level as a consequence of the regulation imposed by IMP3 on the direct targets. We hypothesized that global regulators like transcription factors (TFs) and RNA binding proteins (RBPs) regulated directly by IMP3 either at the transcript or protein (translation) levels may be contributing in modulating the pool of indirect targets. Firstly, we checked for the possible mechanisms affecting the expression levels of indirect targets of IMP3 at the transcript level. To begin with, we checked for the TFs that may regulate the indirect target genes (n = 110) using iRegulon plugin of Cytoscape.Citation26,27 iRegulon predicts the transcription factors and co-factors that may regulate a list of genes. A network of 107 nodes and 449 edges was generated. The network contained nodes associated with transcription factors (Fig. S3a; n = 22; represented in yellow octagonal nodes) and a subset of indirect targets (Fig. S3a; n = 85; represented in gray rectangle nodes) which may be transcriptional targets of these TFs. Next, we checked whether any of the TFs from this subgroup were direct targets of IMP3 either regulated at the level of RNA stability () or translation (). This analysis revealed STAT1, SIN3A, UBP1, JUND and SMAD3 as the transcription factors directly regulated by IMP3. A sub-network representing the interaction between these TFs (shown in yellow octagonal nodes) and the indirect targets (shown in blue rectangle nodes) of IMP3 was created (). We hypothesize that these TFs may be regulating the indirectly regulated genes of IMP3 at their transcription level.
Figure 6. Regulation of indirect targets of IMP3 at transcriptome level. (a) A sub-network created from network shown in Fig. S3a depicting the transcription factors which are direct target of IMP3 (n = 5) and the indirect targets of IMP3 putatively regulated by these 5 transcription factors (n = 41). (b) A sub-network created from Fig. 3b depicting the RNA binding proteins which are direct target of IMP3 (n = 5) and the indirect targets of IMP3 putatively regulated by these 5 RNA binding proteins (n = 39).
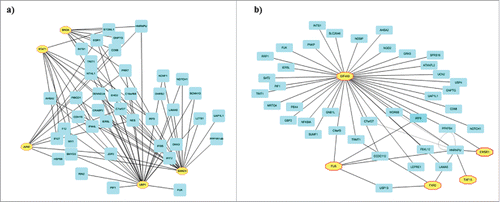
Figure 7. Regulation of indirect targets of IMP3 at the level of translatome. Sub-network created from depicting the RNA binding proteins which are direct target of IMP3 (n = 4) and the indirect targets of IMP3 putatively regulated by these 4 RNA binding proteins (n = 59).
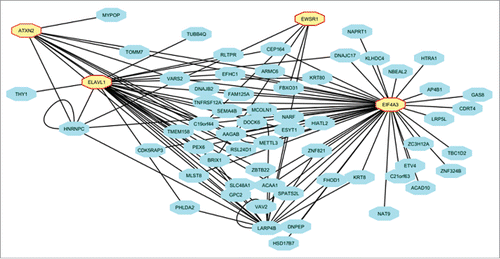
Next, we also envisaged that RBPs which are direct targets of IMP3 may also contribute to the regulation of indirect targets. We used AURA 2Citation28 to identify the putative post-transcriptional regulators of this indirect pool of targets. A network encompassing these regulators (Fig. S3b; yellow octagonal nodes) and the regulated targets (Fig. S3b; gray rectangle nodes) was generated using Cytoscape. The network contained 119 nodes and 346 edges. Next, we checked whether any of the selected regulators (RBPs) are the direct targets of IMP3 regulated at either the RNA stability () or translation level (). This analysis revealed 5 RBPs (FUS, EWSR1, TAF15, EIF4A3 and FXR2) of the 32 post-transcriptional regulators to be direct targets of IMP3. A sub-network representing the interaction between these RBPs (shown in yellow octagonal nodes) and the indirect IMP3 targets (shown in blue rectangle nodes) was created (). These RBPs may be regulating the IMP3 indirect targets at the level of RNA stability. From these results, we enlist the potential TFs and RBPs that may be regulating the transcription and RNA stability of these indirect targets respectively.
Further to unravel the probable mechanism of regulation of indirect targets by IMP3 at translation level, we checked for the IMP3 regulated RBPs that may play a role in regulating these genes. Using AURA 2Citation28, we identified the putative post transcriptional regulators (n = 18) of the indirect targets regulated at the level of translation (n = 109). The network generated constituted of the post-transcriptional regulators (shown in yellow octagonal nodes) and the indirect targets of IMP3 predicted to be targeted by them (shown in gray rectangle nodes) (Fig. S4). Next, we checked whether the selected regulators (RBPs) are the direct targets of IMP3 regulated at either the RNA stability () or translation level (). This analysis revealed 4 RBPs (ELAVL1, EWSR1, ATXN2 and EIF4A3) to be the direct targets of IMP3 (shown in yellow octagonal nodes) and hence may be regulating the translation of these indirect targets of IMP3 (shown in blue rectangle nodes) (). Taken together, we explored the possible regulatory mechanisms by which IMP3 may be regulating its indirect targets at both transcript and translation levels.
Discussion
RNA binding proteins (RBPs) are emerging as key regulators of posttranscriptional mechanisms.Citation29,30 Various steps like mRNA 5′ capping, 3′ polyadenylation, splicing, localization and translation are affected by RBPs.Citation29,30 Aberrant regulation of gene expression at transcript and protein levels has been extensively documented.Citation2-5 Post transcriptional regulation has been ascertained as one of the important regulatory mechanisms in integrating micro-environmental cues with rapid gene expression changes. Indeed, various oncogenic signals (PIK3CA, KRAS and MYC) and tumor suppressive signals (TP53, TSC2 and PTEN) control global and specific mRNA translation.Citation31, 32 Moreover, increased rates of protein synthesis and translation of various oncogenes is accompanied by cell proliferation in response to mitogenic signals.Citation33,34
We have taken IMP3 as an example to study the magnitude of effects that may be brought about by an RBP on the transcriptome and translatome of cells. Gene expression analysis using microarray profiling of total cellular RNA from cells depleted of IMP3 revealed that a large number of transcripts were deregulated upon IMP3 modulation. Earlier studies have identified thousands of bound targets for IMP3 in different non-cancer and cancer cell lines.Citation3,20,21,25 Recent studies have also identified IMP3 modulated targets at the transcript level.Citation15,28,30 A comparison of the existing literature revealed a partial overlap between the targets identified, suggesting the existence of cell line specific targets of IMP3.Citation15,28,30 In the light of this evidence, we performed global microarray profiling of transcripts to reveal a subset of transcriptome regulated by IMP3. Our results revealed that more number of transcripts were downregulated (n = 1388; 54.9%) than upregulated (n = 1077; 45.1%) upon IMP3 silencing in U251 glioma cells.
IMP3 has also been established as a translational regulator for targets like IGF2, ARF6, and ARHGEF4.Citation17,25 To identify the transcripts that are regulated by IMP3 at the level of translation, we performed microarray of RNA isolated from heavy polysome fractions of IMP3 silenced glioma cells. Our analysis determines that almost equal proportion of genes were upregulated (n = 210; 44.8%) and downregulated (n = 259; 55.2%) at the level of translation by IMP3. Among any cancer cell type, our experiments give the first insight in the IMP3 regulated translatome of cancer cells.
IMP3 being an RNA binding protein and a global regulator may have directly and indirectly regulated genes. Integrating data of differentially expressed genes under IMP3 silenced conditions with published data from IMP3 PAR-CLIP dataCitation21 has shed light on the directly and indirect regulated targets of IMP3. Among the identified IMP3 targets, the major proportion of them was found to be direct targets. We also elucidated probable mechanisms by which IMP3 may be modulating the indirect pool of targets. This analysis revealed a list of putative regulators of these genes which consisted of transcription factors (STAT1, UBP1, SMAD3, JUND and SIN3A) and RBPs (ATXN2, FUS, ELAVL1, TAF15, EIF4A3, FXR2 and EWSR1) as potential mediators of indirect regulation by IMP3. It was interesting to note that EIF4A3 and EWSR1 were able to regulate IMP3 indirect targets at both transcriptome and translatome level.Citation35 EIF4A3 functions as an ATP-dependent RNA helicase and as a part of exon junction complex. EWSR1 belongs to the EWS family of proteins which have been shown to play a role in splicing, transcription genotoxic stress and thus contributing to cancer progression.Citation36
IMP3 regulons include a repertoire of genes involved in various cellular functions. We determined important and enriched hallmark biologic processes associated with cancer that are influenced by direct targets of IMP3. Pathways involved in regulation of cell cycle and apoptosis were most significantly enriched in direct targets of IMP3 at transcriptome and translatome level respectively. IMP3 may modulate genes involved in different regulatory networks in different cell types. Evidence for this observation is derived from the migration and invasion related genes regulated by IMP3 in PDAC cell lines,Citation37 whereas cell cycle related genes in B-ALLCitation3 and glioma as shown by our data. Our analysis revealed genes including AURKA, CENPE, CDC25B, CDC25C and GTSE1 as direct transcriptome targets of IMP3 participating in regulation of cell cycle. Similar to IMP3 expression pattern, AURKA, CENPE, CDC25B, CDC25C and GTSE1 are upregulated in GBM tumors (data not shown). Inhibition of AURKA has been shown to reduce glioma cell line growth and has been proposed as a promising target of therapeutic intervention.Citation38 Inhibition of CENPE, a motor kinesin component of the spindle assembly checkpoint decreased the tumor burden in many cancers.Citation39 Silencing of GTSE1 significantly reduces the proliferation of hepatocarcinoma cells.Citation40 CDC25B and CDC25C have also shown to be critical for cell cycle regulation in several cancers.Citation41-46 These phosphatases have also been implicated in glioma biology as positive regulators of proliferation.Citation46 Among translatome direct targets, anti-apoptotic factors including BIRC5, ESPL1 and RAF1 were identified. BIRC5 is a known anti-apoptotic factor in several cancers, and its silencing leads to apoptosis in several types of cancer cells.Citation47-49 ESPL1 is a proposed oncogene and its high expression is associated with poor prognosis in luminal B breast cancer patients.Citation50 It is also implicated in apoptosis related pathways and in chemoresistance of ovarian cancer cells.Citation51,52
To conclude, we have identified the IMP3 regulated transcriptome and translatome in glioma cells. We have also delineated the putative directly regulated targets of the molecule at both the transcript and translation level. We also enlist the various cellular processes regulated by these direct targets of IMP3. Hence, IMP3 may be regulating proliferation and apoptosis by regulating the enlisted targets and thus fuelling GBM aggressiveness. Regulation of multiple important targets and pathways by IMP3, along with its selective and specific expression in tumor tissues underscores its importance as a promising therapeutic target.
Materials and methods
Cell lines and siRNA transfection
U251 cell line was purchased from Sigma (USA). The cell line was grown in DMEM Dulbecco's modified Eagle Medium (DMEM) supplemented in 10% FBS, penicillin (Sigma, USA), gentamicin (Sigma, USA) and streptomycin (Sigma, USA) at 37° C in a humidified atmosphere with 5% CO2. Control siRNA and siRNA used against IMP3 were purchased from GE HealthCare Dharmacon Inc. (On-TARGET plus Human siRNA SMART pool). SMARTpool siRNA is a mixture of 4 different siRNA duplexes targeting distinct coding region sequences of IMP3 (GenbankTM accession number NM_006547).
For siRNA transfection, cells were plated at 70–80 % confluency 16–24 hours before transfection. siRNA transfections were performed using 200 nM siRNA against IMP3. Transfections were performed using Dharmafect 1 as per the manufacturer's instructions. 48 hours after transfection, optiMEM is replaced with complete medium containing 10% FBS. 96 hours post-transfection polysome analysis was performed on cell lysates.
Polysome analysis
U251 cells transfected with control siRNA or siRNA against IMP3 (after 96 hours of transfection) were treated with 100 µg/mL cycloheximide (Sigma, USA) for 10 min at 37° C. Cells were washed with ice cold PBS containing 100 µg/mL cycloheximide and a wash with 1X hypotonic buffer (5 mM TRIS-HCl pH-7.5, 1.5 mM KCl and 5 mM MgCl2 and 100 µg/mL cycloheximide) was given. Cells were harvested in ice cold lysis buffer (5 mM Tris-HCl pH-7.5, 1.5 mM KCl, 5 mM MgCl2, 100 µg/mL cycloheximide, 1mM DTT, 200 U/mL RNasein (RNase inhibitor, Promega, USA), 200µg t-RNA, 0.5% Triton X -100, 0.5% Sodium deoxycholate, 1X protease inhibitor cocktail). After 15 min. of incubation on ice, KCl concentration was adjusted to 150 mM in the lysates. Equal OD of supernatants from the 2 conditions was loaded on 15–50% sucrose gradient containing 100µg/mL cycloheximide and gradients were centrifuged at 36,000 rpm for 2 h at 4° C in SW41 rotor (Beckman). Multiple fractions were collected using automated density Gradient Fractionation System (ISCO). RNA was isolated from total cellular lysate and pooled heavy polysome fractions using TRI reagent (Sigma, USA). RNA level of genes was quantified using real time PCR.
RNA isolation and quantitative real time PCR (qRT-PCR)
Total RNA and heavy polysome RNA was isolated using TRI Reagent® (Sigma, USA) was isolated The relative quantification of the expression levels of genes was performed using a 2-step strategy: In the first step, cDNA was generated from RNA derived from different cell lines or tissue samples using a cDNA archive kit (ABI PRISM); subsequently, real-time quantitative PCR was performed in an ABI PRISM 7900 (Applied Biosystems, USA) sequence detection system with the cDNA as template using gene-specific primer sets and a Dynamo kit containing SYBR green dye (Catalog # F-410L, Thermo Scientific, USA). Measurements were made in duplicates. 18s rRNA (18s rRNA) was used as internal control. ΔΔCTmethod was used for the calculation of expression ratios. Statistical significance was tested by Student's t-test. Primers used in this study are: GTSE1 (forward): CTCTACCAGCAATCTCGCAAGG, GTSE1 (reverse): GACTTGCTGATGTTTGACAGAGG, AURKA (forward): GCAACCAGTGTACCTCATCCTG, AURKA (reverse): AAGTCTTCCAAAGCCCACTGCC, CENPE (forward): GGAGAAAGATGACCTACAGAGGC, CENPE (reverse): AGTTCCTCTTCAGTTTCCAGGTG, CDC25C (forward): AGAAGCCCATCGTCCCTTTGGA, CDC25C (reverse): GCAGGATACTGGTTCAGAGACC, CDC25B (forward): AGAACCTCCTGGACAGTGACCA, CDC25B (reverse): GCTGAACTTGCCCGTCAATAGG, BIRC5 (forward): CCACTGAGAACGAGCCAGACTT, BIRC5 (reverse): GTATTACAGGCGTAAGCCACCG, RAF1 (forward): TCAGGAATGAGGTGGCTGTTCTG, RAF1 (reverse): CTCGCACCACTGGGTCACAATT, TWIST1 (forward): GCCAGGTACATCGACTTCCTCT, TWIST1 (reverse): TCCATCCTCCAGACCGAGAAGG, ESPL1(forward): ATCTCTGTCAGTCGGACCTGCA, ESPL1 (reverse): CAGGTGGACCTTCTTCACAGAG
Bioanalyzer based quantification of RNA samples
RNA quantification was performed using Agilent RNA 6000 pico Kit (Agilent Technologies, USA) by Bioanalyzer (Agilent Technologies) as per manufacturer's instructions.
RNA/DNA quantitation was done using Qubit (Catalog # Q32851, Life technologies, USA). The quantitation of nucleic acids was done as per manufacturer's protocol. Briefly, the assay reagent was diluted in the dilution buffer and then sample was added. The concentrations of the samples were read using Qubit® Fluorometer.
mRNA microarray and data analysis
Sample preparation for microarray was done with 50 pg of RNA using TargetAmp-PicoLabeling Kit (Illumina, USA). Microarray hybridization was performed using Illumina's HumanHT-12 v4 Expression BeadChip Kit as per manufacturer's instructions. The beadchip was scanned using Illumina's HiScanSQ machine. The quantified signals were quantile normalized and only the genes having significant signal intensity were taken. The difference in log2 ratio for each transcript (subtracting the log2 ratio of a given sample from the average log2 ratio of control samples) was calculated. For calculating the H/T ratio, the differential log2 ratio between heavy polysome sample and total RNA samples from the indicated conditions were subtracted. Genes having Log2 ratio ≤ −0.58 and ≥ 0.58 and p-value < 0.05 were considered significantly differentially regulated at transcriptomic data. For determining the IMP3 modulated gene at the level of translatome, genes between Log2 ratio ≤ 0.58 and ≥ −0.58 (unregulated at transcript level) were used for calculation of H/T ratios. Genes having H/T ratio ≤ −1 or ≥ 1 were considered to be differentially regulated at the level of translation. Classification of direct and indirect targets of IMP3 at both levels was performed using information as per the presence or absence of IMP3 binding sites given by Hafner et al., 2010.Citation21
Bioinformatic analysis
Unbiased functional enrichment analysis of IMP3 direct targets at transcriptome and transltome level was performed using DAVID (Database for Annotation, Visualization, and Integrated Discovery).Citation24
iRegulon plugin of CytoscapeCitation26,27 was used to identify transcription factors which may putatively regulate indirect target transcripts. Indirect transcriptomics targets were used as an input and transcription factors and co-factors regulating these pool of targets were predicted. Cytoscape v3.0 was used to visualize the network. AURA 2Citation28 was used to identify the putative post-transcriptional regulators of this indirect pool of transcriptomic and translatomic IMP3 targets. Either of the 2 gene sets was used as an input and only the ones interacting with 3′UTR were selected. These interactions were visualized using Cytoscape v3.0.
Venn diagrams in this study were drawn using an online tool -http://bioinformatics.psb.ugent.be/webtools/Venn//
Disclosure of potential conflicts of interest
No potential conflicts of interest were disclosed.
Author contributions
SB and KS conceived and wrote the paper; SB and VS designed, performed and analyzed all the experiments. RAS helped in performing the experiment shown in Fig. S1a. VP for bioinformatics and statistics related experiments. All authors reviewed the results and approved the final version of the manuscript.
Supplemental_Materials.zip
Download Zip (2.2 MB)Acknowledgments
The results published here are in whole or part based upon data generated by The Cancer Genome Atlas pilot project established by the NCI and NHGRI. Information about TCGA and the investigators and institutions that constitute the TCGA research network can be found at http://cancergenome.nih.gov/. Prof. Varshney is acknowledged for his help in performing polysome experiments and for his critical inputs. SB acknowledges CSIR, Government of India for the research fellowship. VP thanks DBT, Government of India for financial support. KS acknowledges CSIR and DBT, Government of India for research grant. Infrastructure support by funding from DST-FIST, DBT grant-in-aid and UGC (Center for Advanced Studies in Molecular Microbiology) to MCB is acknowledged. KS is a J. C. Bose Fellow of the Department of Science and Technology.
References
- Diederichs S, Bartsch L, Berkmann JC, Frose K, Heitmann J, Hoppe C, Iggena D, Jazmati D, Karschnia P, Linsenmeier M, et al. The dark matter of the cancer genome: Aberrations in regulatory elements, untranslated regions, splice sites, non-coding RNA and synonymous mutations. EMBO Mol Med 2016; 8:442-57; PMID: 26992833; https://doi.org/10.15252/emmm.201506055
- St Laurent G, 3rd, Shtokalo D, Heydarian M, Palyanov A, Babiy D, Zhou J, Kumar A, Urcuqui-Inchima S. Insights from the HuR-interacting transcriptome: ncRNAs, Ubiquitin pathways, and patterns of secondary structure dependent RNA interactions. Mol Genet Genomics 2012; 287:867-79; PMID: 23052832; https://doi.org/10.1007/s00438-012-0722-8
- Palanichamy JK, Tran TM, Howard JM, Contreras JR, Fernando TR, Sterne-Weiler T, Katzman S, Toloue M, Yan W, Basso G, et al. RNA-binding protein IGF2BP3 targeting of oncogenic transcripts promotes hematopoietic progenitor proliferation. J Clin Invest 2016; 126:1495-511; PMID: 26974154; https://doi.org/10.1172/JCI80046
- Lebedeva S, Jens M, Theil K, Schwanhausser B, Selbach M, Landthaler M, Rajewsky N. Transcriptome-wide analysis of regulatory interactions of the RNA-binding protein HuR. Mol Cell 2011; 43:340-52; PMID: 21723171; https://doi.org/10.1016/j.molcel.2011.06.008
- Wurth L, Gebauer F. RNA-binding proteins, multifaceted translational regulators in cancer. Biochim Biophys Acta 2015; 1849:881-6; PMID: 25316157; https://doi.org/10.1016/j.bbagrm.2014.10.001
- Schwanhausser B, Busse D, Li N, Dittmar G, Schuchhardt J, Wolf J, Chen W, Selbach M. Global quantification of mammalian gene expression control. Nature 2011; 473:337-42; PMID: 21593866; https://doi.org/10.1038/nature10098
- Kahvejian A, Svitkin YV, Sukarieh R, M'Boutchou MN, Sonenberg N. Mammalian poly(A)-binding protein is a eukaryotic translation initiation factor, which acts via multiple mechanisms. Genes Dev 2005; 19:104-13; PMID:15630022; https://doi.org/10.1101/gad.1262905
- Wang J, Guo Y, Chu H, Guan Y, Bi J, Wang B. Multiple functions of the RNA-binding protein HuR in cancer progression, treatment responses and prognosis. Int J Mol Sci 2013; 14:10015-41; PMID: 23665903; https://doi.org/10.3390/ijms140510015
- Kobel M, Xu H, Bourne PA, Spaulding BO, Shih Ie M, Mao TL, Soslow RA, Ewanowich CA, Kalloger SE, Mehl E, et al. IGF2BP3 (IMP3) expression is a marker of unfavorable prognosis in ovarian carcinoma of clear cell subtype. Mod Pathol 2009; 22:469-75; PMID: 19136932; https://doi.org/10.1038/modpathol.2008.206
- Xie C, Li Y, Li Q, Chen Y, Yao J, Yin G, Bi Q, O'Keefe RJ, Schwarz EM, Tyler W. Increased insulin mRNA binding Protein-3 expression correlates with vascular enhancement of renal cell carcinoma by intravenous Contrast-CT and is associated with bone metastasis. J Bone Oncol 2015; 4:69-76; PMID: 26478857; https://doi.org/10.1016/j.jbo.2015.07.001
- Schaeffer DF, Owen DR, Lim HJ, Buczkowski AK, Chung SW, Scudamore CH, Huntsman DG, Ng SS, Owen DA. Insulin-like growth factor 2 mRNA binding protein 3 (IGF2BP3) overexpression in pancreatic ductal adenocarcinoma correlates with poor survival. BMC cancer 2010; 10:59; PMID: 20178612; https://doi.org/10.1186/1471-2407-10-59
- Lin CY, Chen ST, Jeng YM, Yeh CC, Chou HY, Deng YT, Chang CC, Kuo MY. Insulin-like growth factor II mRNA-binding protein 3 expression promotes tumor formation and invasion and predicts poor prognosis in oral squamous cell carcinoma. J Oral Pathol Med 2011; 40:699-705; PMID:23647076; https://doi.org/10.1111/j.1600-0714.2011.01019.x
- Tantravahi SK, Albertson D, Agarwal AM, Ravulapati S, Poole A, Patel SB, Hawatmeh JS, Straubhar AM, Liu T, Stenehjem DD, et al. Survival outcomes and tumor IMP3 expression in patients with sarcomatoid metastatic renal cell carcinoma. J Oncol 2015; 2015:181926; PMID: 25688268; https://doi.org/10.1155/2015/181926
- Kazeminezhad B, Mirafsharieh SA, Dinyari K, Azizi D, Ebrahimi A. Usefulness of insulin-like growth factor II mRNA-binding protein 3 (IMP3) as a new marker for the diagnosis of esophageal adenocarcinoma in challenging cases. Turk J Gastroenterol 2014; 25:253-6; PMID: 25141312; https://doi.org/10.5152/tjg.2014.5454
- Bell JL, Wachter K, Muhleck B, Pazaitis N, Kohn M, Lederer M, Hüttelmaier S. Insulin-like growth factor 2 mRNA-binding proteins (IGF2BPs): Post-transcriptional drivers of cancer progression?. Cell Mol Life Sci 2013; 70:2657-75; PMID: 23069990; https://doi.org/10.1007/s00018-012-1186-z
- Lederer M, Bley N, Schleifer C, Huttelmaier S. The role of the oncofetal IGF2 mRNA-binding protein 3 (IGF2BP3) in cancer. Semin Cancer Biol 2014; 29:3-12; PMID: 25068994; https://doi.org/10.1016/j.semcancer.2014.07.006
- Suvasini R, Shruti B, Thota B, Shinde SV, Friedmann-Morvinski D, Nawaz Z, Prasanna KV, Thennarasu K, Hegde AS, Arivazhagan A, et al. Insulin growth factor-2 binding protein 3 (IGF2BP3) is a glioblastoma-specific marker that activates phosphatidylinositol 3-kinase/mitogen-activated protein kinase (PI3K/MAPK) pathways by modulating IGF-2. J Biol Chem 2011; 286:25882-90; PMID: 21613208; https://doi.org/10.1074/jbc.M110.178012
- Samanta S, Sun H, Goel HL, Pursell B, Chang C, Khan A, Greiner DL, Cao S, Lim E, Shultz LD, et al. IMP3 promotes stem-like properties in triple-negative breast cancer by regulating SLUG. Oncogene 2015; 35:1111-21; PMID: 25982283; https://doi.org/10.1038/onc.2015.164
- Samanta S, Pursell B, Mercurio AM. IMP3 protein promotes chemoresistance in breast cancer cells by regulating breast cancer resistance protein (ABCG2) expression. J Biol Chem 2013; 288:12569-73; PMID: 23539627; https://doi.org/10.1074/jbc.C112.442319
- Mizutani R, Imamachi N, Suzuki Y, Yoshida H, Tochigi N, Oonishi T, Suzuki Y, Akimitsu N. Oncofetal protein IGF2BP3 facilitates the activity of proto-oncogene protein eIF4E through the destabilization of EIF4E-BP2 mRNA. Oncogene 2015; 35:3495-502; PMID:26522719; https://doi.org/10.1038/onc.2015.410
- Hafner M, Landthaler M, Burger L, Khorshid M, Hausser J, Berninger P, Rothballer A, Ascano M Jr, Jungkamp AC, Munschauer M, et al. Transcriptome-wide identification of RNA-binding protein and microRNA target sites by PAR-CLIP. Cell 2010; 141:129-41; PMID: 20371350; https://doi.org/10.1016/j.cell.2010.03.009
- Wilkie GS, Dickson KS, Gray NK. Regulation of mRNA translation by 5′- and 3′-UTR-binding factors. Trends Biochem Sci 2003; 28:182-8; PMID: 12713901; https://doi.org/10.1016/S0968-0004(03)00051-3
- Turner JD, Vernocchi S, Schmitz S, Muller CP. Role of the 5'-untranslated regions in post-transcriptional regulation of the human glucocorticoid receptor. Biochim Biophys Acta 2014; 1839:1051-61; PMID: 25150144; https://doi.org/10.1016/j.bbagrm.2014.08.010
- Dennis G, Jr., Sherman BT, Hosack DA, Yang J, Gao W, Lane HC, Lempicki RA. DAVID: Database for annotation, visualization, and integrated discovery. Genome Biol 2003; 4:P3; PMID: 12734009; https://doi.org/10.1186/gb-2003-4-5-p3
- Taniuchi K, Furihata M, Hanazaki K, Saito M, Saibara T. IGF2BP3-mediated translation in cell protrusions promotes cell invasiveness and metastasis of pancreatic cancer. Oncotarget 2014; 5:6832-45; PMID: 25216519; https://doi.org/10.18632/oncotarget.2257
- Janky R, Verfaillie A, Imrichova H, Van de Sande B, Standaert L, Christiaens V, Hulselmans G, Herten K, Naval Sanchez M, Potier D, et al. iRegulon: From a gene list to a gene regulatory network using large motif and track collections. PLoS Comput Biol 2014; 10:e1003731; PMID: 25058159; https://doi.org/10.1371/journal.pcbi.1003731
- Shannon P, Markiel A, Ozier O, Baliga NS, Wang JT, Ramage D, Amin N, Schwikowski B, Ideker T. Cytoscape: A software environment for integrated models of biomolecular interaction networks. Genome Res 2003; 13:2498-504; PMID: 14597658; https://doi.org/10.1101/gr.1239303
- Dassi E, Re A, Leo S, Tebaldi T, Pasini L, Peroni D, Quattrone A. AURA 2: Empowering discovery of post-transcriptional networks. Translation 2014; 2:e27738; PMID: 26779400; https://doi.org/10.4161/trla.27738
- Brinegar AE, Cooper TA. Roles for RNA-binding proteins in development and disease. Brain Res 2016; PMID: 26972534; 1647:1-8; https://doi.org/10.1016/j.brainres.2016.02.050
- Glisovic T, Bachorik JL, Yong J, Dreyfuss G. RNA-binding proteins and post-transcriptional gene regulation. FEBS Lett 2008; 582:1977-86; PMID: 18342629; https://doi.org/10.1016/j.febslet.2008.03.004
- Blagden SP, Willis AE. The biological and therapeutic relevance of mRNA translation in cancer. Nat Rev Clin Oncol 2011; 8:280-91; PMID: 21364523; https://doi.org/10.1038/nrclinonc.2011.16
- Silvera D, Formenti SC, Schneider RJ. Translational control in cancer. Nat Rev Cancer 2010; 10:254-66; PMID: 20332778; https://doi.org/10.1038/nrc2824
- Stolovich M, Tang H, Hornstein E, Levy G, Cohen R, Bae SS, Birnbaum MJ, Meyuhas O. Transduction of growth or mitogenic signals into translational activation of TOP mRNAs is fully reliant on the phosphatidylinositol 3-kinase-mediated pathway but requires neither S6K1 nor rpS6 phosphorylation. Mol Cell Biol 2002; 22:8101-13; PMID: 12417714; https://doi.org/10.1128/MCB.22.23.8101-8113.2002
- Gao B, Roux PP. Translational control by oncogenic signaling pathways. Biochim Biophys Acta 2015; 1849:753-65; PMID: 25477072; https://doi.org/10.1016/j.bbagrm.2014.11.006
- Chan CC, Dostie J, Diem MD, Feng W, Mann M, Rappsilber J, Dreyfuss G. eIF4A3 is a novel component of the exon junction complex. RNA 2004; 10:200-9; PMID: 14730019; https://doi.org/10.1261/rna.5230104
- Paronetto MP. Ewing sarcoma protein: A key player in human cancer. Int J Cell Biol 2013; 2013:642853; PMID: 24082883; https://doi.org/10.1155/2013/642853
- Ennajdaoui H, Howard JM, Sterne-Weiler T, Jahanbani F, Coyne DJ, Uren PJ, Dargyte M, Katzman S, Draper JM, Wallace A, et al. IGF2BP3 modulates the interaction of Invasion-Associated transcripts with RISC. Cell Rep 2016; 15:1876-83; PMID: 27210763; https://doi.org/10.1016/j.celrep.2016.04.083
- Barton VN, Foreman NK, Donson AM, Birks DK, Handler MH, Vibhakar R. Aurora kinase A as a rational target for therapy in glioblastoma. J Neurosurg Pediatr 2010; 6:98-105; PMID: 20593995; https://doi.org/10.3171/2010.3.PEDS10120
- Kung PP, Martinez R, Zhu Z, Zager M, Blasina A, Rymer I, Hallin J, Xu M, Carroll C, Chionis J, et al. Chemogenetic evaluation of the mitotic kinesin CENP-E reveals a critical role in triple-negative breast cancer. Mol Cancer Ther 2014; 13:2104-15; PMID: 24928852; https://doi.org/10.1158/1535-7163.MCT-14-0083-T
- Guo L, Zhang S, Zhang B, Chen W, Li X, Zhang W, Zhou C, Zhang J, Ren N, Ye Q. Silencing GTSE-1 expression inhibits proliferation and invasion of hepatocellular carcinoma cells. Cell Biol Toxicol 2016; 32:263-74; PMID:27240802; https://doi.org/10.1007/s10565-016-9327-z
- Wang M, Zhu XY, Wang L, Lin Y. Expression and significance of CDC25B, PED/PEA-15 in esophageal carcinoma. Cancer Biother Radiopharm 2015; 30:139-45; PMID:25775393; https://doi.org/10.1089/cbr.2014.1701
- Zhang Z, Zhang G, Kong C. High expression of Cdc25B and low expression of 14-3-3sigma is associated with the development and poor prognosis in urothelial carcinoma of bladder. Tumour Biol 2014; 35:2503-12; PMID: 24234332; https://doi.org/10.1007/s13277-013-1331-9
- Yu XY, Zhang Z, Zhang GJ, Guo KF, Kong CZ. Knockdown of Cdc25B in renal cell carcinoma is associated with decreased malignant features. Asian Pac J Cancer Prev 2012; 13:931-5; PMID: 22631674; https://doi.org/10.7314/APJCP.2012.13.3.931
- Cao XC, Yu Y, Hou LK, Sun XH, Ge J, Zhang B, Wang X. miR-142-3p inhibits cancer cell proliferation by targeting CDC25C. Cell Prolif 2016; 49:58-68; PMID: 26805039; https://doi.org/10.1111/cpr.12235
- Sur S, Agrawal DK. Phosphatases and kinases regulating CDC25 activity in the cell cycle: Clinical implications of CDC25 overexpression and potential treatment strategies. Mol Cell Biochem 2016; 416:33-46; PMID: 27038604; https://doi.org/10.1007/s11010-016-2693-2
- Navis AC, van den Eijnden M, Schepens JT, Hooft van Huijsduijnen R, Wesseling P, Hendriks WJ. Protein tyrosine phosphatases in glioma biology. Acta Neuropathol (Berl) 2010; 119:157-75; PMID: 19936768; https://doi.org/10.1007/s00401-009-0614-0
- Lamers F, van der Ploeg I, Schild L, Ebus ME, Koster J, Hansen BR, Koch T, Versteeg R, Caron HN, Molenaar JJ. Knockdown of survivin (BIRC5) causes apoptosis in neuroblastoma via mitotic catastrophe. Endocr Relat Cancer 2011; 18:657-68; PMID: 21859926; https://doi.org/10.1530/ERC-11-0207
- Liu Z, Zhang X, Xu X, Chen L, Li W, Yu H, Sun Y, Zeng J, Jia J. RUNX3 inhibits survivin expression and induces cell apoptosis in gastric cancer. Eur J Cell Biol 2014; 93:118-26; PMID: 24636883; https://doi.org/10.1016/j.ejcb.2014.02.002
- Li WL, Lee MR, Cho MY. The small molecule survivin inhibitor YM155 may be an effective treatment modality for colon cancer through increasing apoptosis. Biochem Biophys Res Commun 2016; 471:309-14; PMID: 26855135; https://doi.org/10.1016/j.bbrc.2016.02.009
- Finetti P, Guille A, Adelaide J, Birnbaum D, Chaffanet M, Bertucci F. ESPL1 is a candidate oncogene of luminal B breast cancers. Breast Cancer Res Treat 2014; 147:51-9; PMID: 25086634; https://doi.org/10.1007/s10549-014-3070-z
- Chen F, Kamradt M, Mulcahy M, Byun Y, Xu H, McKay MJ, Cryns VL. Caspase proteolysis of the cohesin component RAD21 promotes apoptosis. J Biol Chem 2002; 277:16775-81; PMID: 11875078; https://doi.org/10.1074/jbc.M201322200
- Jiang L, Siu MK, Wong OG, Tam KF, Lu X, Lam EW, Ngan HY, Le XF, Wong ES, Monteiro LJ, et al. iASPP and chemoresistance in ovarian cancers: Effects on paclitaxel-mediated mitotic catastrophe. Clin Cancer Res 2011; 17:6924-33; PMID: 21926165; https://doi.org/10.1158/1078-0432.CCR-11-0588