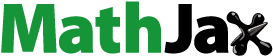
ABSTRACT
MicroRNAs (miRs) are short noncoding RNA molecules that regulate expression of target mRNAs. Many published sources provide information about miRs and their targets. However, bioinformatic tools elucidating higher level impact of the established total miR profiles, are still largely missing. Recently, we developed a method termed OncoFinder enabling quantification of the activities of intracellular molecular pathways basing on gene expression data. Here we propose a new technique, MiRImpact, which enables to link miR expression data with its estimated outcome on the regulation of molecular pathways, like signaling, metabolic, cytoskeleton rearrangement, and DNA repair pathways. MiRImpact uses OncoFinder rationale for pathway activity calculations, with the major distinctions that (i) it deals with the concentrations of miRs - known regulators of gene products participating in molecular pathways, and (ii) miRs are considered as negative regulators of target molecules, if other is not specified. MiRImpact operates with 2 types of databases: for molecular targets of miRs and for gene products participating in molecular pathways. We applied MiRImpact to compare regulation of human bladder cancer-specific signaling pathways at the levels of mRNA and miR expression. We took 2 most complete alternative databases of experimentally validated miR targets – miRTarBase and DianaTarBase, and an OncoFinder database featuring 2725 gene products and 271 signaling pathways. We showed that the impact of miRs is orthogonal to pathway regulation at the mRNA level, which stresses the importance of studying posttranscriptional regulation of gene expression. We also report characteristic set of miR and mRNA regulation features linked with bladder cancer.
Introduction
MicroRNAs (miRs) are 19 to 24 nucleotides long noncoding RNA molecules that regulate the expression of target mRNAs both at the transcriptional and translational levels.Citation1,2 Since the discovery of lin-4 gene producing a small noncoding RNA, which affected the development of C. elegans,Citation3 thousands of miRs in eukaryotes were identified. They influence all major physiological processes such as development, growth, differentiation, immune reaction and adaptation to stress.Citation4,5 Diverse disease states such as cancer, infection and heart failure are associated with distinct miR signatures suggesting that specific miR programs are activated in pathophysiological processes.Citation6,7
Each miR may have tens and even hundreds of different targets and it is a conservative estimate that nearly 30% of the genes are regulated by at least one miR.Citation1 An example is shown on , depicting a molecular signalization pathway laying at the interface between Akt and ERK signaling. Effector miRs targeting gene products participating in this pathway, identified using miRTarBase,Citation8 are schematized on the figure. For example, gene AKT1 has 11 effector miRs, among them one - “has-miR-124-3p” also targets genes AKT2 and AKT3. These and other complex influence patterns lead to unique regulatory networks specific to each molecular pathway.
Figure 1. MiR interactions in OncoFinder pathway ”AKT_Pathway_ERK_Pathway.“ AKT_Pathway_ERK_Pathway is a terminal branch of AKT pathway responsible for the interaction with ERK signaling. It consists of 6 functional nodes including 24 individual gene products. Diagram shows 25 effector microRNAs targeting them, basing on the information from the database miRTarBase
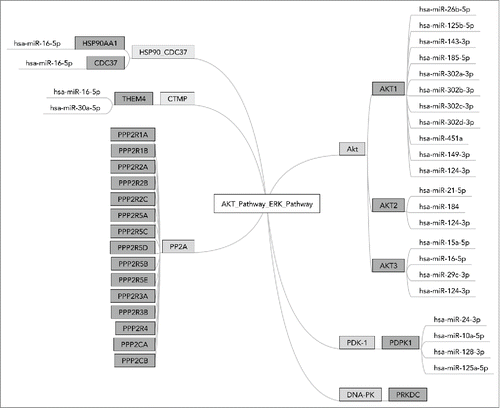
To the date, many databases were published, which accumulate information on individual miRs and their targets.Citation8,9 However, there are no currently available bioinformatic tools which allow to estimate higher-level impact of the overall miR profile in biosamples, e.g. in cell lines and in tissue samples.
Recently, we developed a bioinformatic technique termed OncoFinder.Citation10,11 It allows analyzing activity of intracellular signaling pathways based on gene expression profiles, e.g., came from transcriptomic or proteomic data. The method makes it possible to establish pathway activation strength (PAS) profiles corresponding to intracellular molecular pathways. Several approaches were published previously by us and others to measure PAS basing on large scale gene expression data, which may deal with either transcriptomes or proteomes. Khatri et al.Citation12 classified those methods into 3 major groups: Over-Representation Analysis (ORA), Functional Class Scoring (FCS) and Pathway Topology (PT)-based approaches. ORA-based methods calculate if the pathway is significantly enriched with differentially expressed genes.Citation13 These methods have many limitations, as they ignore all non-differentially expressed genes and don't take into account many gene-specific characteristics. FCS-based approaches partially tackle aforementioned problems by calculating fold change-based scores for each gene and then combining them into a single pathway enrichment score.Citation14 PT-based analysis also takes into account topological characteristics of each given pathway, assigning additional weights to the genes (for a review, see ref. Citation15). To account for gene expression variability within a pathway, a set of differential variability methods has been developed.Citation16 Differential variability analysis determines a group of genes with a significant change in variance of gene expression between case and control groups.Citation17 This approach was further extended and applied on the pathway level.Citation18 Finally, based on the kinetic models that use the “low-level” approach of mass action law, OncoFinder performs quantitative and qualitative enrichment analysis of the signaling pathways. For each investigated sample, it performs a case-control pairwise comparison and calculates the Pathway Activation Strength (PAS), a value which serves as a qualitative measure of pathway activation. Unlike most other methods this approach determines if the signaling pathway is significantly up- or down-regulated compared to the reference. Negative and positive overall PAS values correspond, respectively, to inhibited or activated state of signaling pathway.Citation10
OncoFinder is also, to our knowledge, a unique PAS calculating method, which was reported to provide output data with significantly reduced noise introduced by the experimental transcriptome profiling systems.Citation19 It was shown to be efficient in finding new cancer biomarkers, more stable than individual gene expression patterns.Citation11 To date, OncoFinder was applied for many objects including induced pluripotent stem cells,Citation20 leukemia and solid cancers,Citation21,22,23 Hutchinson Gilford Disease,Citation24 and Age-Related Macular Degeneration Disease.Citation25
Here, we propose a new biomathematical method named MiRImpact, which enables to perform quantitative and qualitative analysis of miRs influence on the activation of intracellular signaling pathways. MiRImpact may be regarded as an extension of OncoFinder technique. The enclosed algorithm distinguishes the positive/activator and negative/repressor roles of every gene product in each pathway. By combining impacts of targeting miR with each individual gene role in a pathway, we explore dependencies between gene expression levels and miR profiles and identify trends in up- and downregulation of intracellular signaling pathways. We took 2 most complete alternative databases of experimentally validated miR targets – miRTarBase and DianaTarBase, and an OncoFinder database featuring 2725 gene products and 271 signaling pathways.
We applied MiRImpact to assess the regulation of signaling pathways in human bladder cancer (BC). Globally, BC is the ninth most common cancer. Approximately 356,000 new cases are reported annually worldwide.Citation26 BC accounts for 3.1% and 1.8% of the overall cancer mortality in males and females, respectively. Early diagnosis of BC can significantly prolong lifespan and improve the quality of life of the patients. Routinely used methods of clinical diagnostics are, in general, not efficient for detecting BC at the early stages. There is an urgent need to develop novel diagnostic tools that would efficiently detect and monitor BC progression.Citation27,28,29 Recently, we identified a number of molecular pathways that may be used as the stable markers of BC.Citation21 These results were based on the high-throughput detection of mRNA levels in the pathological tissues. However, the patient's bladder tissues are not commonly available for a regular screening. From this point of view, urea might be an ideal source of biomaterial for the analysis. Unlike mRNAs which are prone to quick degradation in biological fluids, miRs are known to be stable because of binding with specific proteins that protect them from cleavage by RNases.Citation30 A fraction of known miR species has been reported to be among the major molecular markers of BC.Citation30,31 Here, we applied MiRImpact to compare trends in regulation of signaling pathways at mRNA and miR levels. For the same biosamples, we found a general orthogonal trend in the regulation of pathway activity at those 2 levels. However, for a fraction of molecular pathways that were previously identified as the stable BC biomarkers, we found significantly more congruent regulation at both mRNA and miR levels. We conclude, therefore, that MiRImpact may be beneficial for finding new types of stable biomarkers of BC progression compatible with the routinely available source of biomaterial. For bladder cancer, for the first time, we report the signalome-wide data on the regulation of signaling pathway activity at the levels of mRNA and miR expression.
Results
MiRImpact method
MiRImpact biomathematical algorithm was built to enable quantization of the effects, caused by the changes in overall miR concentrations, on the activity of intracellular molecular pathways. The algorithm was created on the basis of a rationale previously published for the OncoFinder technique.Citation19 As the input information, OncoFinder digests gene expression data (microarray or next generation sequencing transcriptomic data, or proteomic data), and calculates for each molecular pathway a so-called pathway activation strength (PAS) value. PAS values may serve as the measure of pathological changes in an intracellular signaling network.Citation10,32 To calculate PAS, for each gene product participating in a pathway, its molecular function is defined, which may be either overall activator, unknown or repressor role in a pathway. For each gene of a pathway p, its activator-repressor role () is defined, which depends on the functional role of this gene product in a pathway:
This is also important to identify control sample or a group of control samples, which will be used as the norms for PAS calculation. Next, for each gene i, case to normal ratio () is calculated for the respective concentrations of mRNA or for protein concentrations, depending on the origin of input data:
In addition, 2 auxiliary coefficients are introduced:
beyond tolerance interval flag (
) determines if the difference between case and norm is significant:
where the accepted interval of
values (tol) is defined by the user depending to the criteria of differential gene expression used;
activator-repressor role (
), which reflects role of gene product i in pathway p, see above.
value is expressed by the formula:
where summation is made for all the genes
, whose products participate in a molecular pathway
.Positive
values indicate activation of a pathway
compared to norms, whereas negative ones denote downregulation of a pathway.
Similarly to OncoFinder approach, in MiRImpact method, for each miR, a case to normal ratio is calculated for the respective miR concentrations ():
The miR beyond tolerance interval flag () marker determines if the difference between case and norm is significant:
The unique coefficient termed miR involvement index () determines, if a given mRNA transcript of a gene i is a molecular target of a miR
:
The value of miR-defined activation strength of a pathway p () is calculated according to the following:
Similarly to OncoFider, a positive value of indicates activation, whereas a negative one indicates repression of a pathway
, calculated basing on the available miR expression data.
As the intracellular molecular pathway database, we took previously published Oncofinder signaling database featuring 2725 unique genes and 271 signaling pathways.Citation21,22 These data are needed to identify genes involved in each pathway and their functional roles expressed by ARR values. To find out mII indexes, a database covering target gene product specificities of miRs is needed. In this study, we used the most recent updates of the 2 alternative knowledge bases on miRs and their experimentally validated targets: miRTarBaseCitation8 and Diana TarBase.Citation9 The target specificities of miRs cataloged there cover, respectively, 72% and 18% of the genes listed in the OncoFinder database, that was used here for the analysis of signaling pathways (). Both databases include information on more than 50 thousands of molecular interactions of miRs with target mRNA molecules, in case of miRTarBase - for 18 species, in case of Diana-TarBase – for 24 species, including human. This information is manually curated by the database developers basing on published literature on functional experimental studies of miRs. The most commonly used experimental approaches for validating molecular targets of miRs are luciferase reporter assay, Western blots and next generation sequencing approaches.Citation8,9
Table 1. Characteristics of validated miR target databases, based on the data collected from miRTarBase, Diana TarBase and OncoFinder pathway databases.
Biosamples
The bladder cancer (BC) samples were obtained from transurethral resection tissue material taken from 8 individual patients treated in Moscow Oncological Research Institute. All patients provided written informed consent to participate in this study. The consent procedure was approved by the ethical committee of the P.A. Hertzen Moscow Clinical Oncology Institute. Four tissue samples for non-cancer controls were collected at the Department of Pathology at the Faculty of Medicine, Moscow State University, from autopsies from normal bladder tissues taken from 4 independent healthy donors killed in road accidents. Both the tumors and normal tissues were evaluated by a pathologist to confirm the diagnosis and estimate the tumor cell numbers. Gene expression in the BC and normal samples was analyzed using the Illumina HumanHT-12v4 Expression Bead array (Illumina, USA). This gene expression platform contains more than 25,000 annotated genes and more than 48,000 probes derived from the National Center for Biotechnology Information RefSeq (build 36.2, release 22) and the UniGene (build 199) databases. The matching data on miR expression for the same patients were obtained using massive sequencing on an Illumina GAIIx platform. The number of reads varied between the samples, with the mean value of 5,04 million reads per sample. The respective data on gene and miR expression were published by us previously.Citation21,31
Experimental design
Here, we re-analyzed the above BC gene expression and miR expression data using OncoFinder and MiRImpact methods. For the first time, we compared profiles of intracellular signaling pathway activities at the levels of mRNA (OncoFinder) and miR (MiRImpact) regulation. The outline of the data analysis is schematized on .
Figure 2. Schematic outline of bladder cancer miR and gene expression data analysis. Corresponding mRNA and miR expression data were preprocessed for Oncofinder and MIRImpact calculations. Output data were used to generate heatmaps and statistical dependencies between the results obtained using these 2 methods, and to identify up- and downregulated molecular pathways.
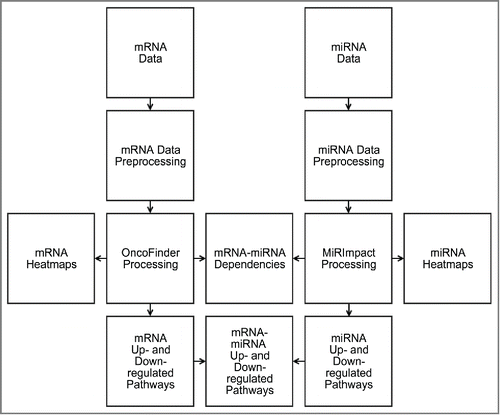
Signaling pathway activation analysis
Overall pathway activation profiles obtained using OncoFinder for mRNA regulation level, and using MiRImpact for miR regulation level, differed dramatically. This was reflected by the apparent differences between the PAS (Supplementary dataset 1) and miPAS (Supplementary datasets 2,3) scores. These two values characterize pathway activity in a biosample at the mRNA and miR levels, respectively. For the PAS scores, 48% of the investigated signaling pathways were downregulated, and 34% were upregulated in all BC samples. Approximately 2% of the pathways showed zero values in all the samples, and ˜16% of the pathways showed opposite trends of regulation in the different BC samples (Supplementary dataset 4).
At the level of miPAS scoring, the results depended greatly on the database used to establish molecular targets of miRs (miRTarBase or Diana-TarBase). For a more complete database miRTarBase, the distribution of up-, down-, intact and contrarily regulated pathways was similar to that observed for the PAS scores (). In contrast, for a database Diana-TarBase having far less molecular targets overlapping with the OncoFinder signaling pathway database (only 18% versus 72% in miRTarBase), significantly greater proportion of unaffected pathways showing zero miPAS scores was seen (21% compared to only 2% for PAS scoring). This may be due to the relatively low number of differentially regulated targets within the pathways that may be captured using the latter database.
Figure 3. Regulation of molecular pathways at the PAS and miPAS levels. The MiRImpact and OncoFinder data were processed for 8 bladder cancer tissue samples. Upregulated or downregulated states of pathway activation were defined as those if at least 5 over total 8 samples showed the respective trends, and the other samples did not show contrary trends. “Inconclusive” results were obtained when either less when 5 samples displayed a certain trend, or when the different samples showed contrary trends for a pathway activation. “Unchanged” states of the pathways mean that the absolute values of PASes or miPASes were lower than the defined significance threshold. The data for miPAS calculation are shown for the 2 alternative miR target databases: miRTarBase (mTB) or Diana-TarBase (DTB).
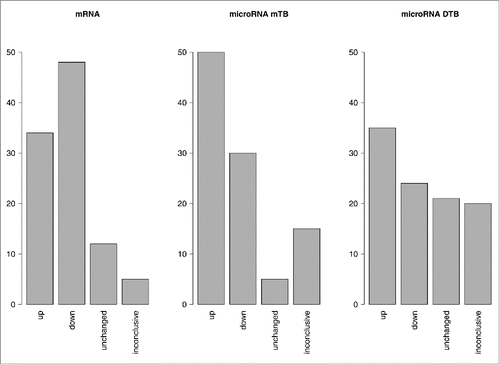
Previously, we identified 44 molecular signaling pathways which may serve as potent biomarkers of BC progression in humans.Citation21 For 21 of them, we found literature data connecting miR expression and pathway activation abnormalities in cancer. We identified for them forty-four published scientific reports describing regulation of signaling pathways by various miRs (Supplementary data set 5). Basing on our own experimental analysis, for miRTarBase we observed congruence with finding of pathway up/downregulated state in 10/21 molecular pathways, and for Diana-Tarbase – in only 5/21 pathways. Note that for both databases, there were no miPAS levels regulated oppositely compared to the published data (Supplementary dataset 5). The remaining pathways that did not coincide with both miRTarBase- and Diana-Tarbase-based versions of MiRImpact, were either apparently inconclusively (bidirectionally) regulated in BC, or were unchanged according to miPAS data. It should be mentioned here that the results of the literature search are typically based on published reports on very few individual effector miRs, which may have distinct activities compared to the whole pool of miRs associated with a given pathway, which has never been previously investigated.
We next compared pathway activation signatures for the 44 above characteristic BC-associated pathways at the mRNA and miR levels. In the case of miRTarBase version, 20 pathways had contrary trends, and only 10 had common trends at the miPAS and PAS levels. For Diana-Tarbase version, 9 pathways had contrary trends, and 10 pathways – common trends on mRNA and miR regulation levels. This suggests that the regulation of many characteristic BC-linked pathways differs dramatically at the mRNA and miR levels. For 11 and 6 characteristic pathways we observed, respectively, common and contradictory trends in pathway regulation using miRTarBase and Diana-Tarbase databases (Supplementary dataset 5). Pathways commonly upregulated according to both databases were ILK pathway_wound healing and mTOR_Pathway_VEGF_pathway activation. Downregulated pathways were 2 branches of AHR pathway: AHR_Pathway_C_Myc_Expression and AHR_Pathway_Cath_D_Repression, a terminal branch of CREB pathway (CREB_Pathway_Gene_Expression), a branch of Glucocorticoid receptor pathway (Glucocorticoid Receptor Pathway Cell cycle arrest), 2 branches of ILK pathway: ILK_Pathway_Cell_motility and ILK_Pathway_G2_phase_arrest regulation, a branch of JAK-STAT pathway (JAK mStat Pathway JAK degradation), and an RNA Polymerase II Complex Pathway. Five pathways were unchanged at the level of miR regulation, according to both databases.
A fraction of consensus data obtained using both databases, demonstrates that 3 molecular pathways previously shown to be aberrantly regulated at the mRNA level,Citation21 are congruently regulated at the miR level as well. These are the branches of the integrin-linked kinase (ILK) signaling pathway, responsible for the cell motility and wound healing, and a branch of the mTOR pathway, responsible for the activation of VEGF signaling (Supplementary dataset 5).
Similar figure was seen when comparing miPAS values for both miRTarBase and Diana-Tarbase versions of MiRImpact, for all available pathways (). For the average miPAS values among all the samples, the correlation was statistically significant, but rather low (0.27), whereas for the different individual BC samples it varied from 0.08 till 0.53 with the mean value of 0.26 (Supplementary dataset 6). Overall, this suggests that the results of miPAS scoring, although roughly correlated, depend greatly on the origin of miR target database used. Both databases used in this study, although reported to include only experimentally validated interactions with targets, produce output results which are poorly compatible to each other. At present, we still don't know what database is better in terms of realistic miR-target mRNA interactions, although miRTarBase may look better because it is far more complete in terms of greater number of miR targets and because it produced output results which were closer to the literature-reported experimental data on pathway activation in response to miRs (10 pathways coincided, vs. only 5 in the case of Diana-Tarbase, see above). We conclude, that further studies are needed to refine the existing miR target databases and to eliminate the apparent disagreements between them.
Figure 4. Comparison of microRNA Pathway Activation Strength (miPAS) values calculated using miRTarBase and Diana TarBase databases of miR targets, for an averaged miR expression between all the samples under investigation. The resulting virtual sample is the result of averaging of miR expression measured by deep sequencing for 8 bladder cancer samples. The results for each individual sample are given on Supplementary dataset 6, showing correlation coefficients varying between 0.06 and 0.53 with the mean value of 0.26.
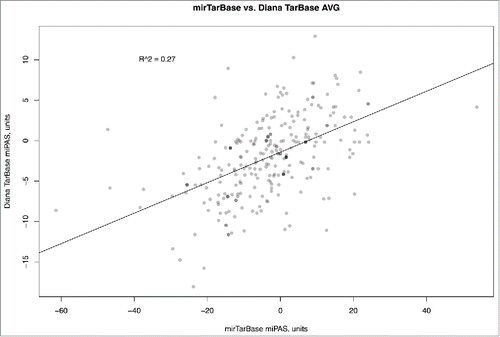
Comparison of pathway activation features at the mRNA and miR levels also showed quite distinct peculiarities in terms of variation between the individual samples. We observed relatively uniform regulation of pathways at the mRNA level, with relatively small number of pathways showing significant variations between the individual samples. In addition, a significant fraction of the pathways showed little or no difference in regulation compared to the normal samples (Supplementary dataset 7). In contrast, at the level of miR regulation, the apparently observed differences between the samples were significantly more sound, as established for both miRTarBase and Diana-Tarbase databases (Supplementary data sets 8 and 9, respectively). In the latter cases, the majority of the pathways were also strongly differential between the normal and cancer samples. These peculiarities of miPAS scores suggest that they may be more sensitive compared to the PAS values to discriminate between the individual cancer samples. This may be highly beneficial for finding new diagnostic markers, e.g. linked with the individual sensitivity of patient to treatment.
Finally, using both abovementioned miR target databases, for the whole set of signaling pathways, the regulation at the miR and mRNA levels, reflected by the miPAS and PAS scores, was not reciprocally correlated (). This characteristic trend was seen for all individual samples (Supplementary datasets 10 and 11), and for the averaged samples shown on , as well. Of note, many molecular pathways showing zero PAS scores, at the same time had quite distinct miPAS scores (). This lack of correlation shown for both alternative databases clearly suggests that transcriptional profiling at the mRNA level alone may be not sufficient to estimate the activation of molecular pathways.
Figure 5. Pathway Activation Strength (PAS) versus microRNA Pathway Activation Strength (miPAS) for an averaged miR and mRNA expression between all the samples under investigation. The resulting virtual sample is the result of averaging of miR expression measured by deep sequencing and mRNA expression measured using microarrays, for 8 bladder cancer samples. The results for each individual sample are given on Supplementary datasets 10 and 11 for both miR target databases. “AVG” samples were averaged at the level of mRNA/miR expression across all 8 BC samples, whereas “PAS AVG” was averaged at the level of PAS/miPAS values across all BC samples.
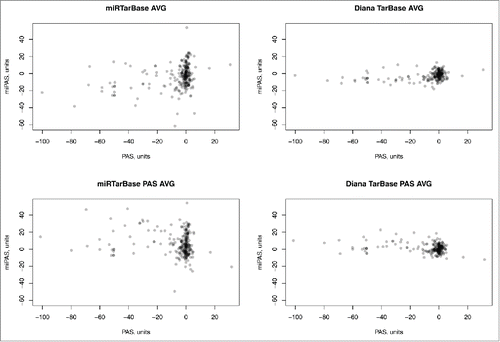
Discussion
In this study, we used 2 alternative databases of experimentally validated miR targets, miRTarBase and Diana-TarBase. We observed a weak, but still statistically significant correlation between the miPAS data calculated for both databases (). However, the high level of noise () reflects a big difference between their content and completeness. The results obtained suggest that the method MiRImpact may be compatible with various databases collecting data on miR specificities and on their specific activities. This means that the future developments based on the MiRImpact method may utilize any kind of new miR target databases, either based on computational prediction, or on experimental validation of miR interactions. Similarly, the enclosed OncoFinder database of signaling pathways may be updated, extended or replaced by another database of molecular pathways, in a user-definitive way. Furthermore, knowledge of the qualitative aspects of molecular interactions between miRs and their targets, and between the molecules participating in molecular pathways, may be used to tune the databases in order to assign specific weighting coefficients to each miR and/or gene product. The mathematical algorithm used here is rather universal and can be employed to trace also metabolic, cytoskeleton rearrangement, DNA repair and other types of intracellular molecular pathways, in any organism or species of the interest. We provide evidence that miPAS values are more variable between the samples than the conventional, mRNA concentrations-based, PAS values. This feature of miPAS can make it an especially useful tool for finding markers of the processes, which are hardly distinguishable according to the current molecular approaches. For example, miPAS values may be a new type of somewhat more sensitive biomarkers for accurate molecular diagnostics of various pathologies, or for predicting response to drug treatment.
We propose here a new biomathematical method, MiRImpact, which makes it possible to link large-scale miR expression data and their estimated outcome on the regulation of intracellular molecular pathways. MiRImpact utilizes a previously published mathematical apparatus for pathway activity calculations, with the major distinctions that (i) it deals with the concentrations of miRs - known regulators of individual gene products participating in molecular pathways, and (ii) miRs are considered by default to be negative regulators of target molecules, if other is not specified. MiRImpact operates with 2 types of databases: for molecular targets of miRs and for gene products participating in molecular pathways. We applied MiRImpact to compare regulation of human bladder cancer-specific signaling pathways at the levels of mRNA and miR expression. We took 2 most complete alternative databases of experimentally validated miR targets – miRTarBase and DianaTarBase, and a previously published OncoFinder database featuring 2725 gene products and 271 signaling pathways. The apparently seen correlation between the data calculated using miRTarBase and Diana-TarBase suggests that the algorithm works in the same manner for both miR target databases. We compared the obtained results with the literature data on the impact of particular miRs on the respective signaling pathways. For the data calculated using the miRTarBase, we observed a greater congruence between the experimental and the literature data (in 47% of the cases), whereas for Diana-TarBase, the data were compatible in only 23% of the cases. We suggest, therefore, that the miRTarBase is currently a database of choice for the estimation of molecular pathways regulation by miRs in humans. The higher variability of miPAS clouds among the samples, compared to previously published PAS values, can make them superior biomarkers of various biological and pathological processes because of greater sensitivity.
We demonstrate here that at least for the human bladder cancer (BC) tissues, the intracellular pathway regulation at the miR level differs greatly from that at the mRNA level, thus showing orthogonal dependencies for the extents of pathway activation. So far, we cannot quantitatively compare the effects of PAS and miPAS scores on the pathway activation. We presume that this will be done in the future by comparing high-throughput miR, mRNA and proteomic expression data, at the level of molecular pathways. To this end, a combination of MiRImpact approach communicated here and of OncoFinder technique published previously, may provide a feasible methodological solution. MiRImpact method would provide information on the activation of molecular pathways at the miR level, whereas OncoFinder – at the whole-transcriptome mRNA and proteomic levels. In addition, ribosome profiling dataCitation33 may be processed with these bioinformatic tools to uncover crosstalk between mRNA concentration, quantitative measure of protein translation efficiency and final protein concentrations. Finally, we propose that other types of noncoding RNAs than miRs can be also analyzed using MiRImpact method, when their regulatory roles and target\effector gene products are known. Connecting these data on pathway activation will be a matter of our further studies.
Methods
Biosamples
Gene expression microarray data were taken from the previous publication,Citation21 and the deep sequencing data for the pools of miRs corresponding to the same biosamples were taken from Zabolotneva et al.Citation31
Mapping of miRs
Following FASTQ to FASTA conversion, the adapter sequence (TGGAATTCTCGGGTGCCAAGG) was clipped from the 3′-end of the read. Remaining sequences were mapped to the databases of miR targets using the short-read aligner Bowtie,Citation34 and processed by SAMTools.Citation35
OncoFinder and MiRImpact software calculation
Quantile normalization was done for both microarray and mapped deep sequencing data. A new project was created and run for mRNA microarray expression according to the software developer recommendations. In MiRImpact software, a new miR project was created and run for with the following default parameters, for both miRTarBase and Diana TarBase: target database - Diana Tarbase, miRTarBase; sigma amount: 2. CNR lower threshold: 0.67; CNR upper threshold: 1.5
Pathway activation data analysis
Heatmaps. Function heatmaps.2 (R package gplots) was used for building heatmaps (Additional Materials 7, 8, 9).
Up- and downregulated pathways. We analyzed activations of 271 intracellular signaling pathways. For mRNA data, we chose pval_threshold equal 0.05 and assigned labels for each pathway according to the following:
– unchanged, if if absolute PAS/miPAS value was less than threshold
– upregulated, if the absolute PAS/miPAS value is higher than the threshold and PAS/miPAS is positive
– downregulated, if the absolute PAS/miPAS value is lower than the threshold and PAS/miPAS is negative
We chose threshold value at the level of approximately 1/10 of a minimum difference among all samples between maximum and minimum PAS/miPAS value within a sample. We assigned pathways the following labels:
We formed a consensus sample for 8 bladder cancer samples. Pathway was assigned quality if more than half (> 4) of all samples had this quality. Otherwise we assigned quality inconclusive. () miRTarBase miPAS vs. Diana-TarBase miPAS dependency was plotted using standard R plot function (). PAS vs. miPAS dependencies were calculated with both miRTarBase and Diana Tarbase validated targets and were plotted using standard R plot function ().
Inspection of literature databases
To validate the method MiRImpact, we performed literature search of miR involvement in intracellular signaling pathway regulation. We analyzed articles indexed by National Center for Biotechnology Information (NCBI), for 44 signaling intracellular pathways which were previously identified as the efficient biomarkers for BC using OncoFinder method.Citation21 We used the following search criteria: “(name of the pathway) + pathway + miRNA” and “(name of the main pathway effector) + pathway + miRNA”. We selected articles which described regulation of a particular signaling pathway by the the miRs in the context of human cancer. The results were compared to the pathway regulation data obtained using MiRImpact method for bladder cancer samples (Supplementary Dataset 5).
Disclosure of potential conflicts of interest
No potential conflicts of interest were disclosed.
2015CC6843R-s12.xlsx
Download MS Excel (17.9 KB)2015CC6843R-s11.xlsx
Download MS Excel (57.1 KB)2015CC6843R-s10.xlsx
Download MS Excel (59.2 KB)2015CC6843R-s09.xlsx
Download MS Excel (77.7 KB)2015CC6843R-s08.pdf
Download PDF (138.7 KB)2015CC6843R-s07.pdf
Download PDF (143.5 KB)2015CC6843R-s06.pdf
Download PDF (37.6 KB)2015CC6843R-s05.pdf
Download PDF (38 KB)2015CC6843R-s04.pdf
Download PDF (40.2 KB)2015CC6843R-s03.pdf
Download PDF (145.2 KB)2015CC6843R-s02.docx
Download MS Word (32.9 KB)Funding
Alina Artcibasova, Mikhail Korzinkin, Maksim Sorokin and Alex Zhavoronkov were supported by the Pathway Pharmaceuticals (Hong-Kong) and First Oncology Research and Advisory Center (Russia) Joint Research Initiative and by the Program of the Presidium of the Russian Academy of Sciences “Dynamics and Conservation of Genomes.”
References
- Bartel DP. MicroRNAs: genomics, biogenesis, mechanism, and function. Cell 2004; 0:281-97; PMID:14744438; http://dx.doi.org/10.1016/S0092-8674(04)00045-5
- Denli AM, Tops BBJ, Plasterk RHA, Ketting RF, Hannon GJ. Processing of primary microRNAs by the Microprocessor complex. Nature 2004; 432:231-5; PMID:15531879; http://dx.doi.org/10.1038/nature03049
- Lee RC, Feinbaum RL, Ambros V. The C. elegans heterochronic gene lin-4 encodes small RNAs with antisense complementarity to lin-14. Cell 1993; 75:843-54; PMID:8252621; http://dx.doi.org/10.1016/0092-8674(93)90529-Y
- Van Rooij E, Sutherland LB, Qi X, Richardson JA, Hill J, Olson EN. Control of stress-dependent cardiac growth and gene expression by a microRNA. Science 2007; 316:575-9; PMID:17379774; http://dx.doi.org/10.1126/science.1139089
- Xiao C, Calado DP, Galler G, Thai T-H, Patterson HC, Wang J, Rajewsky N, Bender TP, Rajewsky K. MiR-150 controls B cell differentiation by targeting the transcription factor c-Myb. Cell 2007; 131:146-59; PMID:17923094; http://dx.doi.org/10.1016/j.cell.2007.07.021
- Calin GA, Ferracin M, Cimmino A, Di Leva G, Shimizu M, Wojcik SE, Iorio M V, Visone R, Sever NI, Fabbri M, et al. A MicroRNA signature associated with prognosis and progression in chronic lymphocytic leukemia. N Engl J Med 2005; 353:1793-801; PMID:16251535; http://dx.doi.org/10.1056/NEJMoa050995
- Zabolotneva A, Tkachev V, Filatov F, Buzdin A. How many antiviral small interfering RNAs may be encoded by the mammalian genomes? Biol Direct 2010; 5:62; PMID:21059241; http://dx.doi.org/10.1186/1745-6150-5-62
- Hsu S-D, Tseng Y-T, Shrestha S, Lin Y-L, Khaleel A, Chou C-H, Chu C-F, Huang H-Y, Lin C-M, Ho S-Y, et al. miRTarBase update 2014: an information resource for experimentally validated miRNA-target interactions. Nucleic Acids Res 2014; 42:D78-85; PMID:24304892; http://dx.doi.org/10.1093/nar/gkt1266
- Vergoulis T, Vlachos IS, Alexiou P, Georgakilas G, Maragkakis M, Reczko M, Gerangelos S, Koziris N, Dalamagas T, Hatzigeorgiou AG. TarBase 6.0: capturing the exponential growth of miRNA targets with experimental support. Nucleic Acids Res 2012; 40:D222-9; PMID:22135297; http://dx.doi.org/10.1093/nar/gkr1161
- Buzdin AA, Zhavoronkov AA, Korzinkin MB, Venkova LS, Zenin AA, Smirnov PY, Borisov NM. Oncofinder, a new method for the analysis of intracellular signaling pathway activation using transcriptomic data. Front Genet 2014; 5:55; PMID:24723936; http://dx.doi.org/10.3389/fgene.2014.00055
- Borisov NM, Terekhanova NV, Aliper AM, Venkova LS, Smirnov PY, Roumiantsev S, Korzinkin MB, Zhavoronkov AA, Buzdin AA. Signaling pathways activation profiles make better markers of cancer than expression of individual genes. Oncotarget 2014; 5:10198-205; PMID:25415353; http://dx.doi.org/10.18632/oncotarget.2548
- Khatri P, Sirota M, Butte AJ. Ten years of pathway analysis: current approaches and outstanding challenges. PLoS Comput Biol 2012; 8:e1002375; PMID:22383865; http://dx.doi.org/10.1371/journal.pcbi.1002375
- Khatri P, Drăghici S. Ontological analysis of gene expression data: current tools, limitations, and open problems. Bioinformatics 2005; 21:3587-95; PMID:15994189; http://dx.doi.org/10.1093/bioinformatics/bti565
- Tian L, Greenberg SA, Kong SW, Altschuler J, Kohane IS, Park PJ. Discovering statistically significant pathways in expression profiling studies. Proc Natl Acad Sci U S A 2005; 102:13544-9; PMID:16174746; http://dx.doi.org/10.1073/pnas.0506577102
- Mitrea C, Taghavi Z, Bokanizad B, Hanoudi S, Tagett R, Donato M, Voichiţa C, Drăghici S. Methods and approaches in the topology-based analysis of biological pathways. Front Physiol 2013; 4:278; PMID:24133454; http://dx.doi.org/10.3389/fphys.2013.00278
- Afsari B, Geman D. Learning dysregulated pathways in cancers from differential variability analysis. Cancer Inform 2014; 13:61; PMID:25392694
- Ho JWK, Stefani M, dos Remedios CG, Charleston MA. Differential variability analysis of gene expression and its application to human diseases. Bioinformatics 2008; 24:i390-8; PMID:18586739; http://dx.doi.org/10.1093/bioinformatics/btn142
- Eddy JA, Hood L, Price ND, Geman D. Identifying tightly regulated and variably expressed networks by Differential Rank Conservation (DIRAC). PLoS Comput Biol 2010; 6:e1000792; PMID:20523739; http://dx.doi.org/10.1371/journal.pcbi.1000792
- Buzdin AA, Zhavoronkov AA, Korzinkin MB, Roumiantsev SA, Aliper AM, Venkova LS, Smirnov PY, Borisov NM. The OncoFinder algorithm for minimizing the errors introduced by the high-throughput methods of transcriptome analysis. Front Mol Biosci 2014; 1:8; PMID:25988149; http://dx.doi.org/10.3389/fmolb.2014.00008
- Makarev E, Fortney K, Litovchenko M, Braunewell KH, Zhavoronkov A, Atala A. Quantifying signaling pathway activation to monitor the quality of induced pluripotent stem cells. Oncotarget 2015; 6:23204-12; PMID:26327604; http://dx.doi.org/10.18632/oncotarget.4673
- Lezhnina K, Kovalchuk O, Zhavoronkov AA, Korzinkin MB, Zabolotneva AA, Shegay P V, Sokov DG, Gaifullin NM, Rusakov IG, Aliper AM, et al. Novel robust biomarkers for human bladder cancer based on activation of intracellular signaling pathways. Oncotarget 2014; 5:9022-32; PMID:25296972; http://dx.doi.org/10.18632/oncotarget.2493
- Spirin PV, Lebedev TD, Orlova NN, Gornostaeva AS, Prokofjeva MM, Nikitenko NA, Dmitriev SE, Buzdin AA, Borisov NM, Aliper AM, et al. Silencing AML1-ETO gene expression leads to simultaneous activation of both pro-apoptotic and proliferation signaling. Leukemia 2014; 28:2222-8; PMID:24727677; http://dx.doi.org/10.1038/leu.2014.130
- Aliper AM, Frieden-Korovkina VP, Buzdin A, Roumiantsev SA, Zhavoronkov A. Interactome analysis of myeloid-derived suppressor cells in murine models of colon and breast cancer. Oncotarget 2014; 5:11345-53; PMID:25294811; http://dx.doi.org/10.18632/oncotarget.2489
- Aliper AM, Csoka AB, Buzdin A, Jetka T, Roumiantsev S, Moskalev A, Zhavoronkov A. Signaling pathway activation drift during aging: Hutchinson-Gilford Progeria Syndrome fibroblasts are comparable to normal middle-age and old-age cells. Aging (Albany NY) 2015; 7:26-37; PMID:25587796
- Makarev E, Cantor C, Zhavoronkov A, Buzdin A, Aliper A, Csoka AB. Pathway activation profiling reveals new insights into age-related macular degeneration and provides avenues for therapeutic interventions. Aging (Albany NY) 2014; 6:1064-75; PMID:25543336
- Parkin DM, Bray F, Ferlay J, Pisani P. Global cancer statistics, 2002. CA Cancer J Clin 55:74-108; PMID:15761078; http://dx.doi.org/10.3322/canjclin.55.2.74
- Kim W-J, Bae S-C. Molecular biomarkers in urothelial bladder cancer. Cancer Sci 2008; 99:646-52; PMID:18377416; http://dx.doi.org/10.1111/j.1349-7006.2008.00735.x
- Majewski T, Lee S, Jeong J, Yoon D-S, Kram A, Kim M-S, Tuziak T, Bondaruk J, Lee S, Park W-S, et al. Understanding the development of human bladder cancer by using a whole-organ genomic mapping strategy. Lab Investig 2008; 88:694-721; PMID:18458673; http://dx.doi.org/10.1038/labinvest.2008.27
- Sánchez-Carbayo M, Cordon-Cardo C. Applications of array technology: identification of molecular targets in bladder cancer. Br J Cancer 2003; 89:2172-7; PMID:14676790; http://dx.doi.org/10.1038/sj.bjc.6601406
- Zabolotneva AA, Zhavoronkov A, Garazha A V, Roumiantsev SA, Buzdin AA. Characteristic patterns of microRNA expression in human bladder cancer. Front Genet 2012; 3:310; PMID:23316212; http://dx.doi.org/10.3389/fgene.2012.00310
- Zabolotneva AA, Zhavoronkov AA, Shegay P V, Gaifullin NM, Alekseev BY, Roumiantsev SA, Garazha A V, Kovalchuk O, Aravin A, Buzdin AA. A systematic experimental evaluation of microRNA markers of human bladder cancer. Front Genet 2013; 4:247; PMID:24298280; http://dx.doi.org/10.3389/fgene.2013.00247
- Zhavoronkov A, Buzdin AA, Garazha A V. Borisov NM, Moskalev AA. Signaling pathway cloud regulation for in silico screening and ranking of the potential geroprotective drugs. Front Genet 2014; 5:49; PMID:24624136; http://dx.doi.org/10.3389/fgene.2014.00049
- Andreev DE, O'Connor PB, Fahey C, Kenny EM, Terenin IM, Dmitriev SE, Cormican P, Morris DW, Shatsky IN, Baranov PV. Translation of 5′ leaders is pervasive in genes resistant to eIF2 repression. Elife 2015; 4:e03971; PMID:25621764; http://dx.doi.org/10.7554/eLife.03971
- Langmead B, Trapnell C, Pop M, Salzberg SL. Ultrafast and memory-efficient alignment of short DNA sequences to the human genome. Genome Biol 2009; 10:R25; PMID:19261174; http://dx.doi.org/10.1186/gb-2009-10-3-r25
- Li H, Handsaker B, Wysoker A, Fennell T, Ruan J, Homer N, Marth G, Abecasis G, Durbin R. The Sequence Alignment/Map format and SAMtools. Bioinformatics 2009; 25:2078-9; PMID:19505943; http://dx.doi.org/10.1093/bioinformatics/btp352