ABSTRACT
Oncogene mutations are primarily thought to facilitate uncontrolled cell growth. However, overexpression of oncoproteins likely leads to apoptosis in a feed forward mechanism, whereby a certain level of oncoprotein leads to the activation of pro-proliferation effector genes and higher levels lead to activation of pro-apoptotic effector genes. TCGA STAD barcodes having no oncoprotein coding region mutations represented reduced expression of the apoptosis-effector genes compared with barcodes with multiple oncoprotein coding region mutations. Furthermore, STAD barcodes in a “no-subsequent tumor” group, representing 224 samples, and in a “positive outcome” group, had more oncoprotein coding regions mutated, on average, than barcodes of the new tumor and negative outcome groups, respectively. BRAF, CTNNB1, KRAS and MTOR coding region mutations (as a group) had the strongest association with the no-subsequent tumor group. Tumor suppressor coding region mutations were also correlated with no-subsequent tumor. These results are consistent with an oncoprotein-mediated, feed-forward mechanism of apoptosis in patients. Importantly, the no-subsequent tumor group also had more overall mutations. This result leads to considerations of unhealthy cells or cells with more neo-antigens for immune rejection. However, a probabilistic aspect of mutagenesis is also consistent with more oncoprotein and tumor suppressor protein mutations, in cases of more overall mutations, and thus a higher likelihood of activation of feed forward apoptosis pathways.
Introduction
The capacity of oncoproteins to stimulate pro-proliferative effector genes and cell division has overwhelmed cancer research. However, oncoproteins also have the capacity to effect apoptosis, particularly at high levels of expression. There are numerous settings where the pro-apoptotic role for what have traditionally been referred to as oncoproteins comes into effect. For example, E2F1 knock-out mice develop tumors,Citation1,2 despite the role of E2F1 in activating pro-proliferation effector genes such as the histone genes and dihydrofolate reductase.Citation3-5 Over-activation of the T-cell receptor, in the course of deletion of self-reactive T-cells, in the thymus, leads to T-cell apoptosis.Citation6-8 Over-expression of POU2F1 (Oct1) leads to apoptosis,Citation9-13 although POU2F1 activates histone genes.Citation14,15 Overexpression of NF-kappaB leads to blast cell apoptosis in anaplastic large cell lymphoma.Citation16,17
While there are several potential mechanisms for the pro-apoptotic effect of overexpressed, pro-proliferative regulatory proteins,Citation18,19 a very credible possibility is represented by the difference in the gene sizes of pro-proliferative versus pro-apoptotic effector genes. McKay et al.Citation20 in particular noticed the unusually small size of apoptosis genes, and later work by our group confirmed the relative size relationships between pro-proliferative and apoptosis-effector genes.Citation21 Furthermore, it has been demonstrated that pro-proliferative effector genes are much bigger “sinks” for pro-proliferative transcription factors, e.g., MYC.Citation21 Pro-proliferative effector genes also have more regions of open chromatin, i.e., more functionally effective regulatory regions than do apoptosis-effector genes.Citation21,22
The above data and paradigm are well represented in cancer medicine by only one case, whereby it has been established that MYCN amplification represents a relatively good prognosis for neuroblastoma specifically in cases where the caspase 8 apoptosis-effector gene is present.Citation23-27 This case prompted us to re-address this issue but by focusing on activating mutations rather than amplification, particularly after having noted that there are patient cases where there are multiple oncoprotein mutations and other cases where cancer develops in the absence of any known oncoprotein mutation, a relatively unexpected situation considering the comprehensive wealth of knowledge known about the available oncoproteins and their considerable role in the theory of oncogenesis. The results for the analysis of the TCGA STAD set indicate that indeed, more oncoprotein mutations correlate with a more favorable outcome.
Results
Apoptosis-effector gene expression for the TCGA STAD dataset was compared for barcodes having mutations in 4 or more oncoprotein coding regions vs. 3 independent sets of randomly selected barcodes having no oncogene, coding region mutations (). For the subsequent analyses in this report, we grouped the oncoprotein and tumor suppressor coding regions, keeping in mind the clear degeneracy of aberrant signaling pathway activation that sustains cancer growth, particular apparent recently with the activation of alternative signaling pathways in designer drug resistant cancer cells.Citation28,29 The barcodes with oncoprotein coding region mutations had a greater level of RNA expression for a previously and rigorously defined set of apoptosis-effector genesCitation21,22,30 compared to the randomly selected barcodes lacking mutations in the indicated oncogene set (; Random Set 1 p < 0.018, Set 2 p < 0.018, Set 3 p < 7.76E-05).
Table 1. HUGO symbols for the oncoprotein, tumor suppressor and apoptosis-effector protein coding region sets used in the analyses. (See SOM file labeled, “SOM , source file.”).
Table 2. Comparison of the expression of apoptosis-effector genes in the samples representing the high oncoprotein mutation barcodes vs. randomly selected barcodes having no oncoprotein mutations. (See SOM file labeled, “SOM STAD, apoptosis.”).
To determine whether there was a clinical distinction between more or fewer oncoprotein coding region mutations, we established groups of barcodes from the STAD and BLCA cancer sets, as stated above, according to the clinical outcome methods 1 and 2 (see Methods): the development of a new tumor versus no-subsequent tumor; and negative and positive (treatment) outcome, respectively. The groups were compared based on the number of oncoprotein coding regions mutated, i.e. the coding region for that barcode was mutated at least once (). The no-subsequent tumor and positive outcome groups for the STAD data set had a higher number of oncoprotein coding regions mutated (p < 0.004 and p < 0.026, respectively), while the BLCA cancer set did not show any differences in oncoprotein coding region mutations for the 2 different clinical outcome groups.
Figure 1. (A) Average number of oncoproteins mutated for New Tumor vs. No-subsequent tumor (STAD p < 0.004, BLCA not significant) (detailed in SOM file labeled, “SOM , source file”). The new tumor group represents 74 barcodes and the no-subsequent tumor group represents 224 barcodes. (B) Average number of oncoproteins mutated for Negative vs. Positive outcome (STAD p < 0.026, BLCA not significant) (detailed in SOM file labeled, “SOM , source file”). The negative outcome group represents 32 barcodes and the positive outcome group represents 71 barcodes.
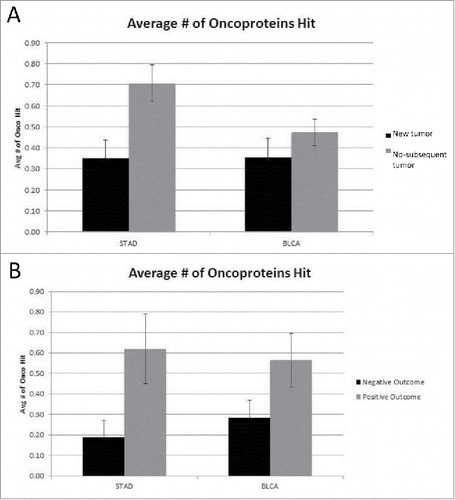
Inspection of the new tumor and no-subsequent tumor data for the STAD dataset revealed 4 oncoprotein coding regions (BRAF, CTNNB1, KRAS and MTOR) that were mutated in the greatest number of barcodes (). A difference in the number of mutations representing this group of oncoproteins, between the new tumor and no-subsequent tumor barcodes, represented a statistical significance of p < 2.24E-05.
Table 3. Reduced oncoprotein set for comparison of the new tumor vs. no-subsequent tumor in the STAD data set. Comparison made based on the oncoprotein being mutated at least once or not (detailed in the SOM file labeled, “SOM STAD, new tumor_onco hit or not”). The p-value represents more oncoprotein mutations in the no-subsequent tumor group. The new tumor group represents 74 barcodes and the no-subsequent tumor group represents 224 barcodes.
Mutation rates for the oncoprotein coding region set were recalculated to consider all mutations in a given coding region (rather than whether the coding region was “hit” or not), along with the mutation rates for the tumor suppressor gene set and total genome mutations. A comparison of the new tumor and no-subsequent tumor barcodes showed an increase in the average number of oncoprotein, tumor suppressor and total coding region mutations (p < 0.007, p < 0.019 and p < 0.004, respectively) for the no-subsequent tumor group in the STAD data set (). The BLCA cancer sets representing new tumor and no-subsequent tumor, respectively, did not differ significantly in the number of oncoprotein, tumor suppressor or total genome mutations. Using the same approach of considering all mutations, a comparison of the negative and positive outcome barcodes was conducted (). The positive outcome group in the STAD dataset had a higher average number of oncoprotein coding region mutations compared to the negative outcome group (p < 0.042).
Figure 2. (A) Oncoprotein mutation averages for New Tumor vs. No-subsequent tumor (STAD p < 0.007, BLCA not significant) (detailed in SOM file labeled, “SOM , source file”). The new tumor group represents 74 barcodes and the no-subsequent tumor group represents 224 barcodes. (B) Tumor suppressor mutation averages for New Tumor vs. No-subsequent tumor (STAD p < 0.019, BLCA not significant) (detailed in SOM file labeled, “SOM , source file”). The new tumor group represents 74 barcodes and the no-subsequent tumor group represents 224 barcodes. (C) Total mutation averages for New Tumor vs. No-subsequent tumor (STAD p< 0.004, BLCA not significant) (detailed in the SOM file labeled, “SOM , source file”). The new tumor group represents 74 barcodes and the no-subsequent tumor group represents 224 barcodes.
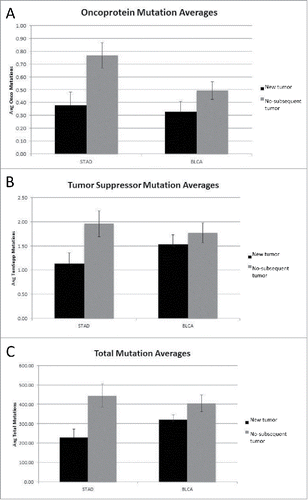
Figure 3. (A) Oncoprotein mutation averages for Negative and Positive outcome (STAD p < 0.042, BLCA not significant) (detailed in SOM file labeled, “SOM , source file”). The negative outcome group represents 32 barcodes and the positive outcome group represents 71 barcodes. (B) Tumor suppressor mutation averages for Negative and Positive outcome (Both STAD and BLCA are not significant) (detailed in SOM file labeled, “SOM , source file”). The negative outcome group represents 32 barcodes and the positive outcome group represents 71 barcodes. (C) Total mutation averages for Negative vs. Positive outcome (STAD and BLCA are not significant) (detailed in SOM file labeled, “SOM , source file”). The negative outcome group represents 32 barcodes and the positive outcome group represents 71 barcodes.
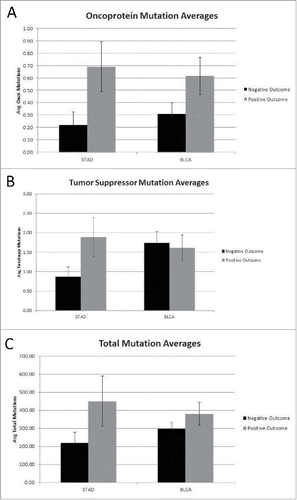
Previous work has indicated that the ratio of silent to amino acid altering mutations is highly constant within a TCGA cancer data set, e.g., regardless of whether a barcode has few mutations or a large number of mutations.Citation31 That result is consistent with the overwhelming stochastic nature of somatic mutagenesis. Thus, to a very significant degree, total mutations track amino acid altering mutations. Regardless, we repeated the above analyses with all of the silent mutations removed (). The new tumor vs. no-subsequent tumor, and negative versus positive outcome groups, were compared for the oncoprotein, tumor suppressor protein and total coding region mutation sets. As expected, the above described, statistically significant correlations of STAD oncoprotein, tumor suppressor protein, and overall coding region mutations, with the indicated clinical parameters, were reproduced and verified.
Table 4. Indications of the p-values for comparison of mutation occurrences, in the oncoprotein, tumor suppressor protein and total coding region sets, for the STAD dataset, when silent mutations are either included or removed. (These data are compiled from the SOM files labeled, “SOM STAD, new tumor_results” and “SOM STAD, neg and pos outcome_results.”) In all cases, the p-values represent higher numbers of mutations in the no-subsequent tumor group and in the positive outcome group. The new tumor group represents 74 barcodes and the no-subsequent tumor group represents 224 barcodes. The negative outcome group represents 32 barcodes and the positive outcome group represents 71 barcodes.
To further consider the functional impact of the higher level of mutations correlating with a favorable course of disease, the number of deleterious amino acid changes for the barcodes representing the new tumor vs. no-subsequent tumor, and negative versus positive outcome, were determined for the tumor suppressor coding region set in the STAD cancer data set (). The no-subsequent tumor and positive outcome groups show an approximate 2-fold increase in the number deleterious amino acid changes compared to the new tumor and negative outcome groups, respectively. The number of deleterious amino acid changes was also calculated for the oncoprotein gene set in the STAD cancer dataset. The no-subsequent tumor and positive outcome groups, again, showed an approximate 2-fold increase in the number of deleterious amino acid changes compared to the new tumor and positive outcome groups, respectively ().
Table 5. Average number of deleterious amino acid changes per barcode for each clinical outcome group for the STAD cancer set using PROVEAN (data compiled from the SOM files labeled, “SOM STAD, PROVEAN_new tumor_onco,” “SOM STAD, PROVEAN_new tumor_tumsupp,” “SOM STAD, PROVEAN_negandpos outcome_onco,” “SOM STAD, PROVEAN_neg and pos outcome_tumsupp”). The new tumor group represents 74 barcodes and the no-subsequent tumor group represents 224 barcodes. The negative outcome group represents 32 barcodes and the positive outcome group represents 71 barcodes.
Discussion
For decades, there has been an apparent contradiction with regard to the dual functions of oncoproteins and pro-proliferative signaling pathways in proliferation and apoptosis. There is very little acceptance in the scientific community regarding a mechanism to unify these functions, but one very credible proposal is that over-expression or over-activation of oncoproteins leads to apoptosis. In addition to the extensive data consistent with this mechanism, the mechanism represents a certain biologic elegance, in that apoptosis largely results from a failure to transit S-phase, and such a failure may prevent the negative feedback that would ordinarily be in effect with the completion of cell-division that would dampen pro-S-phase activation processes. Without the negative feedback, S-phase activation processes continue. The prolongation of oncoprotein-mediated, S-phase stimulation processes, e.g., prolonged because of the absence of needed metabolites and S-phase block, would be conveniently exploited to engineer cell apoptosis when practical for the organism, because of the pending S-phase failure.
Despite the extensive scientific literature confirming the feed-forward mechanism of apoptosis, regardless of the debate over molecular details, very little of this knowledge has led to progress in cancer patient treatments or prognosis, almost certainly because of the complexity of assessing and exploiting degrees of oncoprotein expression or activity, as opposed to the discreet, presence or absence of an oncoprotein. Interestingly, decades ago, high dose estrogen strategy was employed for breast cancer treatment,Citation32 possibly due to hints of breast cancer cell susceptibility with over-activation of what would otherwise be a pro-proliferative pathway. And, as indicated above, MYCN amplification has been noted as prognostic of a more favorable outcome for neuroblastoma. And, it is relatively common for rapidly dividing tumors to respond well to therapy.Citation33,34 Such results have been attributed to the assumption that rapidly dividing cells rapidly acquire a high mutation burden from mutagenic therapy. However, it remains possible that rapidly dividing cells are close to the tipping point, with regard to over-activation of oncproteins, thereby being well poised to enter apoptosis following mutagenic therapy that halts or significantly delays S-phase. Given the likely complications involved in treating patient tumors with drugs meant to stimulate tumor growth, in the hopes of “over-treatment” and driving tumor cells into apoptosis, a feed-forward mechanism of apoptosis does not immediately suggest therapeutic options. But it is interesting to note that decades ago, high dose estrogen therapies were used to treat breast cancer.Citation32
For the first time, the above analysis indicates a likely setting whereby more oncoprotein coding region mutations represent a better outcome, in retrospect not surprising given the pioneering work indicating the association of MYCN amplification with a better outcome and the decades old work indicating that rapidly dividing cancers are more vulnerable to chemotherapy.
The oncoprotein coding region mutation associations with a more favorable disease course for STAD is verified by analogous results presented above for tumor suppressor protein coding region mutations. However, the results did not apply to the BLCA data set, either because of distinct cancer development mechanisms or simply for technical reasons, such as the use of an oncoprotein set that is not as relevant to BLCA as STAD.
Methods
Apoptosis-effector gene expression, oncoprotein set, tumor suppressor set
Clinical and somatic mutation data were collected for the stomach adenocarcinoma (STAD) dataset from the TCGA data portal (http://cancergenome.nih.gov/), under NIH/dbGAP project approval number #6300. Tumor sample barcodes in the somatic mutation (exome) file were truncated to contain only the following characters, TCGA-##-####. Mutation data from the comprehensive mutation file, including truncated tumor sample barcodes and mutation type (amino acid altering or silent), were collected for the oncoprotein and tumor suppressor coding region sets (HUGO symbols listed in ) and a Microsoft Excel COUNTIF function was used to determine the number of oncoprotein coding region mutations per barcode for the barcodes collected from the clinical patient file. The oncoprotein and tumor suppressor sets have been previously described.Citation31,35,36 Barcodes having 4 or more oncoprotein coding region mutations were considered to be high oncoprotein coding region mutation barcodes and served as the experimental group. Barcodes having no known oncoprotein coding region mutations, based on the above referenced oncoprotein set, were sorted randomly using a random number generator function and grouped into 3 sets (SET 1-3) to serve as control groups (“SOM STAD, apoptosis”).
Level 3 BCGSC IlluminaHiSeq RNASeq data was collected from the TCGA data portal for the barcodes in the experimental group and each of the 3 control groups (“SOM STAD, apoptosis”). From the gene quantification file, RPKM values of the apoptosis-effector gene set () were collected for each barcode. This () set of apoptosis genes is referred to as “Set A” in several previous publications,Citation21,22,30 and represents a high stringency standard for the definition of apoptosis-effector gene, as described.Citation21,22,30 However, due to issues related to data availability for the STAD RNASeq files, the following 3 genes were eliminated from the apoptosis-effector gene set described in refs. Citation21, 22, 30: COX6B2, COX7B2, and AIFM3.
Clinical outcome method 1: Developing new tumor vs. no-subsequent tumor
The TCGA STAD clinical follow up file was used to categorize barcodes based on the new tumor event data. Barcodes having developed a new tumor status post initial treatment were grouped and labeled “new tumor,” and those not having developed a new tumor were grouped and labeled “no-subsequent tumor.” Any barcode with no associated mutation data was eliminated and a comparison between the remaining new tumor and no-subsequent tumor barcodes was conducted based on the total number of oncoprotein coding regions mutated, i.e., based on the coding region being mutated at least once. (Additional detail of methods is provided in SOM file labeled, “SOM STAD, new tumor_onco hit or not”). We then considered the total number of mutations, i.e. included all mutations occurring in each coding region per barcode, and compared the new tumor and no-subsequent tumor barcodes. Using this same approach of considering all mutations per coding region, we compared the new tumor and no-subsequent tumor barcodes based on the tumor suppressor gene set () and total mutation rates (Additional detail of method provided in SOM file labeled, “SOM STAD, new tumor results”).
Clinical outcome method 2: Treatment best response
The TCGA STAD clinical drug file was used to categorize barcodes into negative and positive outcome groups based on the treatment best response data. Barcodes with stable or clinical progressive disease were grouped and labeled “negative outcome,” while barcodes with partial or complete response were grouped and labeled “positive outcome.” Any barcode appearing in multiple treatment, best response categories or with no corresponding mutation data was eliminated. The above methods of comparison outlined in clinical outcome method 1 were used to compare the negative and positive outcome groups. (Additional detail for methods is provided in SOM files labeled, “SOM STAD, neg and pos outcome_onco hit or not” and “SOM STAD, neg and pos outcome_results.”) The above methods for clinical outcome methods 1 and 2 were then repeated for the TCGA BLCA cancer set from TCGA. The corresponding SOM files are labeled, “SOM BLCA,” with the same corresponding suffix as used for the STAD SOM files.
Deleterious amino acid changes: PROVEAN
The average number of deleterious amino acid changes was determined for each clinical outcome group, i.e., new tumor, no-subsequent tumor, negative outcome and positive outcome, for the oncoprotein and tumor suppressor coding region sets for the STAD data set. The chromosome number, start position, reference allele and tumor sequence allele data were collected for the oncoprotein and tumor suppressor coding region setsCitation31,35,36 for each clinical outcome group. The data was then copied into PROVEAN under the “Human Genome Variants” protocol. The removal of duplicates from the “#ROW_NO.” column in the PROVEAN output was used to determine the number of deleterious amino acid changes for the oncoprotein and tumor suppressor coding region sets for each of the clinical outcome groups. The number of deleterious amino acid changes was then divided by the sample size to determine the average number of deleterious amino acid changes per barcode for each clinical outcome group for the STAD cancer dataset (Additional detail provided in the SOM files labeled, “SOM STAD, PROVEAN_new tumor_onco,” “SOM STAD, PROVEAN_new tumor_tumsupp,” “SOM STAD, PROVEAN_neg and pos outcome_onco,” and “SOM STAD, PROVEAN_neg and pos outcome_tumsupp”).
Disclosure of potential conflicts of interest
No potential conflicts of interest were disclosed.
1195532_Supplemental_Material.zip
Download Zip (7.6 MB)Funding
Authors acknowledge the financial contributions of the Anna Valentine Fund and the taxpayers of the State of Florida.
References
- Field SJ, Tsai FY, Kuo F, Zubiaga AM, Kaelin WG, Jr, Livingston DM, Orkin SH, Greenberg ME. E2F-1 functions in mice to promote apoptosis and suppress proliferation. Cell 1996; 85(4):549-61; PMID:8653790; http://dx.doi.org/10.1016/S0092-8674(00)81255-6
- Yamasaki L, Jacks T, Bronson R, Goillot E, Harlow E, Dyson NJ. Tumor induction and tissue atrophy in mice lacking E2F-1. Cell 1996; 85(4):537-48; PMID:8653789; http://dx.doi.org/10.1016/S0092-8674(00)81254-4
- Oswald F, Dobner T, Lipp M. The E2F transcription factor activates a replication-dependent human H2A gene in early S phase of the cell cycle. Mol Cell Biol 1996; 16(5):1889-95; PMID:8628255; http://dx.doi.org/10.1128/MCB.16.5.1889
- Banerjee D, Ercikan-Abali E, Waltham M, Schnieders B, Hochhauser D, Li WW, Fan J, Gorlick R, Goker E, Bertino JR. Molecular mechanisms of resistance to antifolates, a review. Acta Biochimica Polonica 1995; 42(4):457-64; PMID:8852336
- Kirch HC, Putzer B, Schwabe G, Gnauck HK, Schulte Holthausen H. Regulation of adenovirus 12 E1A transcription: E2F and ATF motifs in the E1A promoter bind nuclear protein complexes including E2F1, DP-1, cyclin A and/or RB and mediate transcriptional (auto)activation. Cell Mol Biol Res 1993; 39(8):705-16; PMID:7951410
- Gatzka M, Walsh CM. Apoptotic signal transduction and T cell tolerance. Auto Immunity 2007; 40(6):442-52
- Ramos SJ, Hernandez JB, Gatzka M, Walsh CM. Enhanced T cell apoptosis within Drak2-deficient mice promotes resistance to autoimmunity. J Immunol 2008; 181(11):7606-16; PMID:19017949; http://dx.doi.org/10.4049/jimmunol.181.11.7606
- Carreno LJ, Gonzalez PA, Kalergis AM. Modulation of T cell function by TCR/pMHC binding kinetics. Immuno Biol 2006; 211(1-2):47-64; http://dx.doi.org/10.1016/j.imbio.2005.09.003
- Zhao H, Jin S, Fan F, Fan W, Tong T, Zhan Q. Activation of the transcription factor Oct-1 in response to DNA damage. Cancer Res 2000; 60(22):6276-80; PMID:11103783
- Hafezi F, Marti A, Grimm C, Wenzel A, Reme CE. Differential DNA binding activities of the transcription factors AP-1 and Oct-1 during light-induced apoptosis of photoreceptors. Vision Res 1999; 39(15):2511-8; PMID:10396620; http://dx.doi.org/10.1016/S0042-6989(98)00313-7
- Szekeres K, Koul R, Mauro J, Lloyd M, Johnson J, Blanck G. An Oct-1-based, feed-forward mechanism of apoptosis inhibited by co-culture with Raji B-cells: towards a model of the cancer cell/B-cell microenvironment. Exp Mol Pathol 2014; 97(3):585-9; PMID:25236570; http://dx.doi.org/10.1016/j.yexmp.2014.09.010
- Sikora E, Grassilli E, Radziszewska E, Bellesia E, Barbieri D, Franceschi C. Transcription factors DNA-binding activity in rat thymocytes undergoing apoptosis after heat-shock or dexamethasone treatment. Biochem Biophys Res Commun 1993; 197(2):709-15; PMID:8267607; http://dx.doi.org/10.1006/bbrc.1993.2537
- Berry DE, Lu Y, Schmidt B, Fallon PG, O'Connell C, Hu SX, Xu HJ, Blanck G. Retinoblastoma protein inhibits IFN-gamma induced apoptosis. Oncogene 1996; 12(8):1809-19; PMID:8622902
- Zheng L, Roeder RG, Luo Y. S phase activation of the histone H2B promoter by OCA-S, a coactivator complex that contains GAPDH as a key component. Cell 2003; 114(2):255-66; PMID:12887926; http://dx.doi.org/10.1016/S0092-8674(03)00552-X
- La Bella F, Heintz N. Histone gene transcription factor binding in extracts of normal human cells. Mol Cell Biol 1991; 11(12):5825-31; PMID:1944265; http://dx.doi.org/10.1128/MCB.11.12.5825
- Hirsch B, Hummel M, Bentink S, Fouladi F, Spang R, Zollinger R, Stein H, Durkop H. CD30-induced signaling is absent in Hodgkin's cells but present in anaplastic large cell lymphoma cells. Am J Pathol 2008; 172(2):510-20; PMID:18187570; http://dx.doi.org/10.2353/ajpath.2008.070858
- Nishikori M, Ohno H, Haga H, Uchiyama T. Stimulation of CD30 in anaplastic large cell lymphoma leads to production of nuclear factor-kappaB p52, which is associated with hyperphosphorylated Bcl-3. Cancer Sci 2005; 96(8):487-97; PMID:16108830; http://dx.doi.org/10.1111/j.1349-7006.2005.00078.x
- Lu H, Hallstrom TC. The nuclear protein UHRF2 is a direct target of the transcription factor E2F1 in the induction of apoptosis. J Biol Chem 2013; 288(33):23833-43; PMID:23833190; http://dx.doi.org/10.1074/jbc.M112.447276
- Lu H, Liang X, Issaenko OA, Hallstrom TC. Jab1/CSN5 mediates E2F dependent expression of mitotic and apoptotic but not DNA replication targets. Cell Cycle 2011; 10(19):3317-26; PMID:21937878; http://dx.doi.org/10.4161/cc.10.19.17618
- McKay BC, Stubbert LJ, Fowler CC, Smith JM, Cardamore RA, Spronck JC. Regulation of ultraviolet light-induced gene expression by gene size. Proc Natl Acad Sci U S A 2004; 101(17):6582-6; PMID:15087501; http://dx.doi.org/10.1073/pnas.0308181101
- Mauro JA, Blanck G. Functionally distinct gene classes as bigger or smaller transcription factor traps: a possible stochastic component to sequential gene expression programs in cancer. Gene 2014; 536(2):398-406; PMID:24291030; http://dx.doi.org/10.1016/j.gene.2013.11.013
- Mauro JA, Butler SN, Ramsamooj M, Blanck G. Copy number loss or silencing of apoptosis-effector genes in cancer. Gene 2015; 554(1):50-7; PMID:25307873; http://dx.doi.org/10.1016/j.gene.2014.10.021
- Teitz T, Wei T, Liu D, Valentine V, Valentine M, Grenet J, Lahti JM, Kidd VJ. Caspase-9 and Apaf-1 are expressed and functionally active in human neuroblastoma tumor cell lines with 1p36 LOH and amplified MYCN. Oncogene 2002; 21(12):1848-58; PMID:11896617; http://dx.doi.org/10.1038/sj.onc.1205180
- Teitz T, Wei T, Valentine MB, Vanin EF, Grenet J, Valentine VA, Behm FG, Look AT, Lahti JM, Kidd VJ. Caspase 8 is deleted or silenced preferentially in childhood neuroblastomas with amplification of MYCN. Nat Med 2000; 6(5):529-35; PMID:10802708; http://dx.doi.org/10.1038/75007
- Peirce SK, Findley HW. High level MycN expression in non-MYCN amplified neuroblastoma is induced by the combination treatment nutlin-3 and doxorubicin and enhances chemosensitivity. Oncology Reports 2009; 22(6):1443-9; PMID:19885598; http://dx.doi.org/10.3892/or_00000586
- Fulda S, Lutz W, Schwab M, Debatin KM. MycN sensitizes neuroblastoma cells for drug-induced apoptosis. Oncogene 1999; 18(7):1479-86; PMID:10050884; http://dx.doi.org/10.1038/sj.onc.1202435
- Huang M, Weiss WA. Neuroblastoma and MYCN. Cold Spring Harbor Perspectives Med 2013; 3(10):a014415; ; http://dx.doi.org/10.1101/cshperspect.a014415
- Frangione ML, Lockhart JH, Morton DT, Pava LM, Blanck G. Anticipating designer drug-resistant cancer cells. Drug Discov Today 2015; 20(7):790-3; PMID:25697478; http://dx.doi.org/10.1016/j.drudis.2015.02.005
- Bucheit AD, Davies MA. Emerging insights into resistance to BRAF inhibitors in melanoma. Biochem Pharmacol 2014; 87(3):381-9; PMID:24291778; http://dx.doi.org/10.1016/j.bcp.2013.11.013
- Garcia M, Mauro JA, Ramsamooj M, Blanck G. Tumor suppressor genes are larger than apoptosis-effector genes and have more regions of active chromatin: Connection to a stochastic paradigm for sequential gene expression programs. Cell Cycle 2015; 14(15):2494-500; PMID:25945879; http://dx.doi.org/10.1080/15384101.2015.1044179
- Parry ML, Ramsamooj M, Blanck G. Big genes are big mutagen targets: A connection to cancerous, spherical cells? Cancer Letters 2015; 356(2 Pt B):479-82
- Stoll BA. Hypothesis: breast cancer regression under oestrogen therapy. British Medical J 1973; 3(5877):446-50; http://dx.doi.org/10.1136/bmj.3.5877.446
- Pantazis P, Early JA, Kozielski AJ, Mendoza JT, Hinz HR, Giovanella BC. Regression of human breast carcinoma tumors in immunodeficient mice treated with 9-nitrocamptothecin: differential response of nontumorigenic and tumorigenic human breast cells in vitro. Cancer Res 1993; 53(7):1577-82; PMID:8453626
- Kessel D. Modes of resistance to antitumor agents. Vivo 1994; 8(5):829-34; PMID:7727732
- Fawcett TJ, Parry ML, Blanck G. A Novel Approach to Evaluating Cancer Driver Gene Mutation Densities: Cytoskeleton-related Gene Candidates. Cancer Genomics Proteomics 2015; 12(6):283-90; PMID:26543077
- Long K, Abuelenen T, Pava L, Bastille M, Blanck G. Size matters: sequential mutations in tumorigenesis may reflect the stochastic effect of mutagen target sizes. Genes Cancer 2011; 2(10):927-31; PMID:22701759