ABSTRACT
Transcriptional timing is inherently influenced by gene length, thus providing a mechanism for temporal regulation of gene expression. While gene size has been shown to be important for the expression timing of specific genes during early development, whether it plays a role in the timing of other global gene expression programs has not been extensively explored. Here, we investigate the role of gene length during the early transcriptional response of human fibroblasts to serum stimulation. Using the nascent sequencing techniques Bru-seq and BruUV-seq, we identified immediate genome-wide transcriptional changes following serum stimulation that were linked to rapid activation of enhancer elements. We identified 873 significantly induced and 209 significantly repressed genes. Variations in gene size allowed for a large group of genes to be simultaneously activated but produce full-length RNAs at different times. The median length of the group of serum-induced genes was significantly larger than the median length of all expressed genes, housekeeping genes, and serum-repressed genes. These gene length relationships were also observed in corresponding mouse orthologs, suggesting that relative gene size is evolutionarily conserved. The sizes of transcription factor and microRNA genes immediately induced after serum stimulation varied dramatically, setting up a cascade mechanism for temporal expression arising from a single activation event. The retention and expansion of large intronic sequences during evolution have likely played important roles in fine-tuning the temporal expression of target genes in various cellular response programs.
Introduction
Human genes come in a wide variety of lengths; protein-coding genes range in length from less than a hundred to over 2 million base pairs.Citation1 One consequence of this extensive range in gene size is that the time needed to complete transcription elongation of a full-length transcript is also highly variable among genes. Hypothetically, at a constant transcription elongation rate of 1.4 kb/min,Citation2-5 a 100 bp gene would take only a few seconds to finish while a 2 Mb long gene at the same rate would take over 24 hours to complete elongation. These gene size differences are largely driven by variations in the lengths of intronic sequences. Long genes tend to be mostly comprised of intronic sequences that are transcribed but then excised by the spliceasome and degraded by the nuclear RNA exosome.Citation6 Additionally, introns tend to be longer in humans than in other vertebrate species,Citation7 and longer in tissue-specific genes compared to housekeeping genes.Citation8 Furthermore, very long genes promote genomic instability through increased risks for the formation of copy number variations (CNVs) and common fragile sites.Citation9 Why have long genes survived evolutionary pressures for shortening despite the time and energy burden and the threat to genomic integrity they present? While introns undoubtedly play critical roles in genome organization, hosting ncRNA genes, RNA processing and isoform diversity,Citation10-12 their contribution to gene size variability suggests that intron length may also serve an important biological function in regulating the temporal expression of genes induced simultaneously in response to stress or cell stimuli.
Early observations of large introns in Drosophila developmental genes led to the “intron delay hypothesis” which postulates that intron length may play a role in gene expression timing.Citation13 Indeed, it has been demonstrated that during Drosophila early development, the gap gene kni is expressed while its cognate gene knrl, which has more intronic sequence, does not have enough time to be expressed due to rapid cell divisions disrupting its transcription.Citation14 More recently, it was shown that intronic length is important during somite segmentation of mouse embryos.Citation15 During this time, the Hes7 gene is cyclically expressed as a result of regulation through a negative feedback loop involving the Hes7 protein. Removal of introns from Hes7 led to earlier expression and abolishment of oscillatory expression, and resulted in developmental defects. While intron length is implicated in the regulation of expression timing for certain genes during development, whether variable intron lengths are important for coordinating gene expression programs outside of development is unknown.
Gene expression programs allow cells to react to specific changes in their environment through temporally coordinated transcription of response genes. Various signaling cascades activate preexisting transcription factors, which in turn induce primary response genes. Immediate-early genes induced as part of the primary response are often rapidly and transiently expressed, and many of these genes encode transcription factors.Citation16 The target genes of these transcription factors are part of the secondary response in which gene induction requires de novo protein synthesis and therefore necessitates a delay before transcription initiation of these genes. Initiation of transcription is classically viewed as a key regulatory point for the timing of gene expression, however other transcriptional events such as pre-positioned RNA polymerase II (RNAPII) at promoter proximal pause sites and rate of transcriptional elongation which is affected by intron base composition, repetitive sequences, and RNA splicing complex formation, can also influence temporal expression patterns during the early activation stages of gene programs.Citation17,18
The early response to serum stimulation provides an excellent model for studying immediate-early gene expression programs. While this response involves many triggers and signal transduction pathways making it difficult to elucidate mechanisms responsible for transcriptional regulation, it is a well-studied response for inducing robust transcriptional reprogramming. Fibroblasts grown in serum-free media enter a G0 state of minimal metabolic activity, and subsequent addition of serum to these cells results in global gene expression changes as cells prepare to re-enter the cell cycle.Citation19 While the genome-wide early serum response has been previously explored, these studies focused on expression changes based on steady-state mRNA levels.Citation20,21 Because such analyses only provide information about the steady-state of completed transcripts, they do not directly indicate timing of upstream transcriptional events such as induction or repression of transcription initiation. Some studies have explored nascent transcription of early response genes using qRT-PCR,Citation17,22 but this approach is limited to the assessment of transcription of a handful of selected genes. We recently used nascent RNA Bru-seq to assess the dynamics of transcription initiation and elongation during the first 2 hours after serum stimulation.Citation23 In the present study we used Bru-seq and BruUV-seq to assess induction and repression of transcription initiation, and changes in the activity of enhancer elements immediately after serum stimulation.Citation24-26 Because Bru-seq allows for the assessment of transcription initiation without the need for the generation of a mature mRNA product, our study provides a comprehensive profile of the immediate transcriptional response to serum. Our results confirm the rapid induction of many previously described serum-response genes and provide a novel list of genes immediately repressed by serum stimulation. We also show that many of the highly induced genes were associated with activated putative enhancers while downregulated genes were rarely associated with inactivated enhancers, suggesting that enhancer regulation primarily plays a key role in the regulation of rapid gene induction. Finally, serum-induced genes were found to be on average significantly longer than housekeeping genes and serum-repressed genes, highlighting the importance of gene size variability and the role of gene length in temporal global expression timing.
Results
Identification of immediate serum response genes using Bru-seq
To investigate the immediate transcriptional response to serum, we compared nascent RNA expression in starved and serum activated normal human fibroblasts using Bru-seq.Citation23-25 Cells were grown in serum-free media for 48 hours. Serum was then added back to the media (or not for the starved control), and nascent RNA was immediately labeled with bromouridine (Bru) for 30 minutes (). The Bru-RNA was isolated, used to prepare cDNA libraries, deep sequenced, and mapped to the reference genome (hg19). The experiment was repeated and the transcription profiles of the 2 biological experiments were highly correlated (Fig. S1). The mapped reads typically give a jagged appearance, which is quite reproducible and most likely stem from an inherent feature of library preparations primarily caused by variations in PCR amplification. Among the genes that were immediately induced, we observed known immediate-early genes such as the transcription factor gene FOS (). This was evident through an increase in reads across the entire gene. In long induced genes, such as PDE7B (∼344 kb) which encodes a nucleotide phosphodiesterase, the increase in reads was present at the beginning of the gene but only extended partially into the body of the gene (). We previously measured a median elongation rate of ∼1.4kb/min in the first 40 kb of genes of our fibroblast cell line.Citation2 Consistent with this rate, the 30-minute labeling period did not allow enough time for newly initiated PDE7B transcripts to be completed. In contrast to RNA techniques based on steady-state RNA analysis, the Bru-seq approach allowed us to identify genes that were transcriptionally repressed immediately after serum addition, such as TRIB2 (Tribbles pseudokinase 2) (). For long repressed genes, such as the signaling gene GNG2 (guanine nucleotide binding protein 2) (109 kb), we observed a decrease in reads at the beginning of the gene and a receding wave of reads toward the 3′ end of the gene (). This receding wave corresponds to transcripts initiated prior to serum addition that continued to elongate toward the 3′-end of the gene during the labeling period.
Figure 1. (A) Experimental outline. Bromouridine (2 mM) labeling was performed for 30 minutes on serum starved human fibroblasts or together with serum for 30 min on previously starved human fibroblasts. Nascent RNA sequencing reads expressed as RPKM are shown for (B) FOS, (C) PDE7B, (D) TRIB2 and (E) GNG2 in starved cells (orange trace) and in cells serum stimulated for 30 min (blue trace).
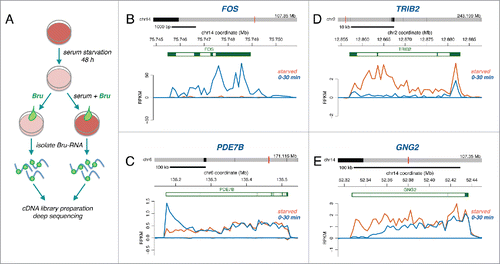
For our genome-wide analysis, we calculated nascent RNA expression levels for all genes in starved cells and during the 30-minute serum stimulation period. For genes over 30 kb, we calculated expression levels based on the first 30 kb of the genes because we predicted that newly induced or repressed long genes would exhibit read changes within this region but not at the end of these genes. Out of the 6958 genes fitting our expression criteria (at least 300 bp long and expressed above 0.5 RPKM), we identified 873 significantly induced and 209 significantly repressed genes (adjusted p-values < 0.05, n = 2) during the first 30 min of serum stimulation (Table S1). Our results demonstrate that serum stimulation of starved human fibroblasts results in extensive, immediate transcriptional changes that are detectable by Bru-seq, including a novel set of immediately repressed genes.
Comparisons between Bru-seq and microarray technologies for serum-induced transcription timing
Previously defined groups of immediate-early genes based on increases in steady-state mRNA levels tend to be dominated by small genes.Citation16,22 This is partially due to timing constraints on elongation since these techniques are biased toward detection of full-length transcripts. Bru-seq allows for the assessment of instantaneous changes in transcription, and by analyzing reads just downstream of transcription start sites (TSSs), changes in transcription initiation can be inferred independently of gene length. Such immediate assessment of transcriptional changes is not afforded by microarray or conventional RNA-seq analysis because changes are slow to appear in steady state RNA pools due to the “noise” of pre-existing RNA. Furthermore, if RNA is captured through poly(A) selection, changes will only be detected after completion of full-length transcripts.
A previous study used the microarray approach and polyA+ enriched RNA to assess the timing of transcriptional changes in human fibroblasts following serum stimulation,Citation20 and we compared this dataset to our Bru-seq dataset. For short genes, both Bru-seq and the microarray assay detected rapid increases in transcription levels by the first 30 minutes following serum stimulation (Fig. S2). In contrast, for longer genes, the microarray analysis detected increased transcript levels at later labeling periods following serum addition while Bru-seq detected an immediate increase of nascent transcription at the beginning of the gene within the first 30 minutes. This timing discrepancy can be explained by the delay between productive initiation of RNAPII and the time needed to elongate across the entire gene to produce full length transcripts detected by the microarray assay in the total RNA pool. These comparisons of transcriptional response timing demonstrate how different methods capture distinct stages of transcription.
Gene size-dependent induction of functional pathways
We next tested the hypothesis that gene length may play a functional role in the temporal regulation of gene expression during cellular responses. Although a large set of genes may be induced simultaneously, variations in gene size allow for staggered generation of full-length RNA products. This would lead to temporally ordered translation of mRNAs at ribosomes and staggered production of proteins involved in different response pathways. We performed gene set enrichment analysis (GSEA) on our list of expressed genes which were pre-ranked according to fold change in the serum-stimulated sample compared to the starved sample (Table S2). The pathways which showed enrichment among genes induced by serum stimulation included “TNF signaling via the transcription factor NFκB,” “TGFβ signaling,” “apical junction,” and “hypoxia” (). Pathways which showed enrichment among genes suppressed by serum included “meiotic recombination,” “RNA pol I transcription,” “peroxisome,” and “oxidative phosphorylation” (). To examine whether induced genes of different sizes were enriched for specific biological pathways, we grouped the 873 serum-induced genes based on size and performed DAVID functional annotation analysis (Table S3, , S3). The induced gene groups were compared to a background of all expressed genes within the same size category in order to eliminate biases of pathways that may be overrepresented in certain size categories. Pathways such as “focal adhesion” and “actin cytoskeleton” were enriched in all size categories while “MAPK signaling pathway” was enriched in 4 out of 5 size groups. “Regulation of transcription” was enriched in the 3 smallest size classes, while “pathways in cancer” was enriched in the 2 largest gene categories. For genes repressed by serum stimulation, we grouped the 209 genes into 2 size groups, smaller or larger than 15 kb. Among the smallest gene group, enriched gene sets included “transcription,” “mitochondrial oxidative phosphorylation,” and “chromatin assembly” (), while for the larger genes, enrichment for “actin cytoskeleton,” “focal adhesion,” “pathways in cancer,” and “endocytosis” was found ().
Figure 2. Gene set enrichment analysis of immediate serum-response genes. Normalized enrichment scores calculated by GSEA for pathways identified in induced (A) and repressed (B) serum response genes. Enriched pathways identified by DAVID in induced (C-G) and repressed (H-I) gene sets grouped based on gene size. The data is expressed as -log10 of p-values from two independent biological experiments.
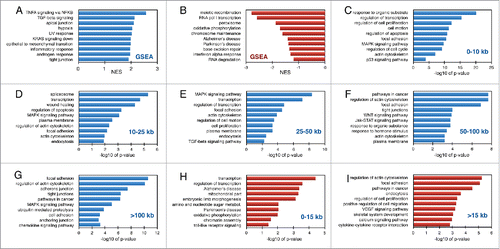
Primary transcription factors responding to serum stimulation
To explore what transcription factors may be responsible for regulating the immediate serum response in human fibroblasts, we performed GSEA analysis to look for transcription factor-binding motifs enriched among the induced genes (Table S4). As expected, the transcription factor binding motif for serum response factor (SRF) had the highest enrichment score, followed by CREBP1 (ATF2), and other members of the ATF/CREB transcription factor family (). Among repressed genes, we found enrichment of 3 motifs that have not yet been associated with any known transcription factor (). Because our Bru-seq set of immediately repressed genes is novel, much less is known about the transcription factors and regulatory mechanisms controlling the suppression of these genes.
Rapid activation of enhancer elements following serum stimulation
We recently developed BruUV-seq, which allows for the genome-wide identification of putative active enhancer elements.Citation26 Here we used BruUV-seq to investigate the relationship between immediately induced serum response genes and concurrently activated enhancer elements. In BruUV-seq, cells are exposed to UV-irradiation prior to Bru-labeling, resulting in increased signal for certain classes of non-coding RNAs such as RNA generated at active enhancer elements (eRNA). This enhanced eRNA signal is likely due to UV-induced transcription-blocking lesions and inhibition of RNA exosome components, which together allow us to capture unstable RNA species.Citation26-28 We identified several induced genes that had corresponding increases in activity at nearby putative enhancer elements. For example, Bru-seq identified a 9-fold induction of the FOS gene (Table S1), which was accompanied by an increase in reads at nearby UV-enriched peaks that overlap with an annotated lncRNA (). These peaks were not seen in the Bru-seq data, reflecting the unstable nature of these lncRNAs, presumably due to rapid degradation by the RNA exosome, which suggests that they may represent eRNAs.Citation26 Similar findings of nearby putative enhancer elements were made for the highly induced genes NR4A1, TNC, ID1, ID2, and ID3 (). Out of the top 50 most highly induced genes, we found evidence that 39 of these were adjacent to at least one putative activated enhancer element, and out of these, 18 genes were adjacent to 4 or more putative activated enhancer peaks (Table S1). In sharp contrast, only one of the top 15 most highly repressed genes following serum stimulation coincided with suppression of nearby putative enhancer elements (Table S1). Thus, during the serum response, immediate induction of many genes appears to be associated with rapid activation of nearby enhancer elements, while genes rapidly repressed by serum stimulation appear to be inhibited in an enhancer-independent manner.
Figure 4. Putative enhancer activation following serum stimulation. Bru-seq (top) and BruUV-seq (bottom) traces are shown for starved (blue) and serum stimulated cells (orange) for (A) FOS, (B) NR4A1, (C) TNC, (D) ID1, (E) ID2, and (F) ID3. Genes are shown on top in green for plus strand genes and red for minus strand genes. Transcripts and histone mark peaks from ENCODE data for normal human lung fibroblasts (NHLF) are shown below. Red arrows point at intergenic transcription peaks enhanced in BruUV-seq compared to Bru-seq, which align with peaks for the enhancer marks H3K4me1 and H3K27ac.
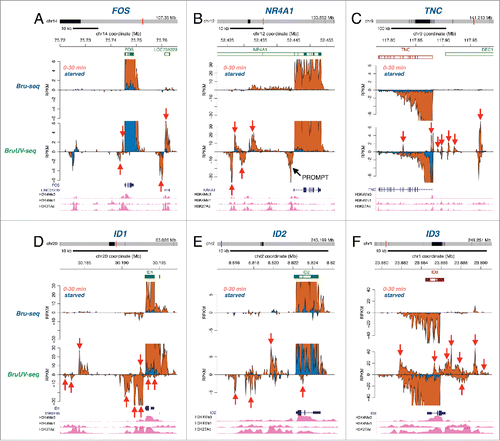
Diverse sizes of serum-induced transcription factor and miRNA genes
A prominent group of immediately induced genes following serum stimulation identified by Bru-seq were transcription factor genes (Table S4). Of the 873 immediately induced genes, 111 were transcription factor genes, while 21 of the 209 repressed genes encoded transcription factors. These transcription factor genes were of various sizes and expected to complete transcription at different times. Based on size, small transcription factor genes such as FOSB, NR4A1, and NR4A2, are expected to produce full-length transcripts within about 6 minutes (), medium-sized genes such as BACH1, TSC22D2, and ZNF131 are expected to produce full-length transcript after about 30 minutes (), and large genes such as NFKB1, KLF7, and HIVEP2 are expected to produce finished transcripts after 1-3 hours following serum addition ().
Figure 5. Transcriptional delays as a result of gene length. Bru-seq traces for (A) FOSB, (B) NR4A1, (C) NR4A2, (D) CDK7, (E) REL, (F) LIMA1, (G) PDLIM5, (H) BTAF1, and (I) UBR4 during starved conditions (orange trace) and following serum stimulation (blue trace), along with estimated times required for transcription completion (right) based on length and an elongation rate of 1.4 kb/min.
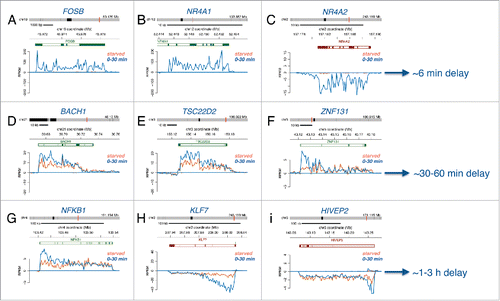
MicroRNAs (miRNAs) are small non-coding RNAs that can target specific mRNAs for degradation or inhibit translation.Citation29 Annotation of miRNA genes has been difficult because rapid processing of miRNA precursors into mature miRNA do not allow traditional RNA-seq techniques to capture miRNA primary transcripts. We have used Bru-seq to identify and annotate primary miRNA gene sequences genome-wide in multiple cell lines and found that they vary dramatically in size (manuscript in preparation). In this study, we found several miRNAs genes that were transcriptionally upregulated in response to serum in human fibroblasts. Examples of miRNA genes that were rapidly induced following serum stimulation are the gene pairs MIR143:1/ MIR143:2 () and MIR30B/MIR30D (). Other induced miRNA genes appeared to be regulated by transcription run-on past the termination sites of the upstream genes, such as MIR21 located downstream of VMP1 () and MIR3193 located downstream of ID1 (). Because these primary miRNA transcripts are short, they are expected to be completely transcribed within the 30 min labeling period. In contrast, a miRNA cluster on chromosome 14 consisting of 43 miRNAs is transcribed from a single promoter as one 200 kb unit,Citation26 and therefore these miRNAs are subject to a delay in production. These variably sized primary miRNA transcription units set up a type of cascade mechanism for temporal gene regulation similar to transcription factor genes. The size of the transcription unit will determine the length of time delay before these transcripts can be processed into mature miRNAs for inhibition of translation and degradation of target mRNAs.
Relative gene length is evolutionarily conserved
One possible role for long introns is to delay the completion of full-length transcripts. The simultaneous activation or repression of a set of genes will result in different completion times determined by gene length. We reasoned that if the serum response utilizes a gene length delay mechanism to regulate temporal dynamics, serum-response genes will be, on average, longer than housekeeping genes that are constitutively transcribed. To test this, we compared the median lengths among different human gene groups. The median length of transcription factor genes expressed in human fibroblasts is ∼27 kb (488 genes), similar to the median length of all genes expressed in these cells, which is ∼29.5 kb (6958 genes) (). In contrast, human housekeeping genes expressed in human fibroblasts (as defined by a study which identified as genes expressed across 19 normal tissuesCitation10) were found to be significantly shorter with a median length of just ∼9.4 kb (336 genes). Next, we compared the median length of the serum induced genes to serum repressed genes and found that induced genes were overall significantly longer than repressed genes, suggesting that temporal expression timing of induced genes relies on gene size more than repressed genes. This also held true when examining the subgroup of transcription factor genes responding to serum stimulation. We estimated transcriptional completion timing of serum response transcription factors based on length (). While we estimate that the majority of the transcription factors complete transcription within the first hour after serum stimulation, there are several transcription factors (27/131) that are likely to complete transcription later, some not until 2 hours following serum addition. Following translation, induced transcription factors likely activate another set of target response genes, and expression timing of these target genes would depend on gene size as well. This sets up a complex regulatory mechanism by which successive series of gene induction events set up precise temporal expression patterns as a function of gene size. We found that expressed genes in human fibroblasts and corresponding mouse orthologs exhibited similar size distributions when grouped as above (). Overall, relative gene size is well-conserved between human and mouse (). Previous studies have shown that genes tend to be larger in humans compared to other animals, largely due to intron lengthening.Citation7,30,Citation31 A study comparing orthologous introns between human and mouse found that 70% of human introns are longer than corresponding mouse introns.Citation30 However, although human introns are longer, there is a strong conservation of relative lengths of specific introns. Therefore, the longest intron of a gene in human tends to be the longest intron of a gene in mouse. These results show that gene size distribution is conserved and suggest that the maintenance of relative gene size may be important for proper expression timing.
Figure 7. Serum response genes and their mouse orthologs exhibit a broad range of gene sizes. (A) Boxplots displaying the distributions of gene sizes for various gene sets: all genes expressed in either starved or serum stimulated cells (6958 genes), transcription factor genes expressed in either starved or serum stimulated cells (488 genes), housekeeping genes expressed in either starved or serum stimulated cells (336 genes), genes induced after serum stimulation (873 genes), genes repressed after serum stimulation (210 genes), transcription factor genes induced after serum stimulation (111 genes), and transcription factor genes repressed after serum stimulation (21 genes). Stars indicate significance of Mann-Whitney-Wilcoxon statistical tests comparing the medians of the different groups, with p-values *< 2 × 10-5, **< 2 × 10-7 and ***< 3 × 10-16. (B) Induced and repressed transcription factor genes displayed according to estimated time needed to complete transcription. (C) Boxplots displaying the distributions of gene sizes for mouse orthologs of the gene sets shown in A. Only orthologs with one-to-one homology were assessed. All genes (5719 genes), all TFs (385 genes), housekeeping genes expressed (272 genes), induced genes (753 genes), repressed genes (169 genes), induced TFs (102 genes), and repressed TFs (17 genes). (D) Correlation plot of sizes of all human protein-coding genes and sizes of their mouse orthologs (15845 genes). Only orthologs with one-to-one homology were assessed.
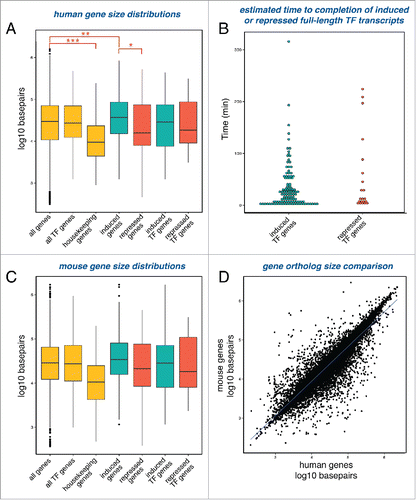
Temporal expression for optimal protein complex assembly
Assembly of multi-protein complexes may benefit from temporal synthesis of individual components. To explore this, we focused on the GO term category “cellular components,” which includes complexes that we found to be enriched among our induced genes such as “actin cytoskeleton” and “plasma membrane” (). Many of these complexes contained genes within several size categories (Fig. S3). Examples of transcriptional induction of 4 genes of varying lengths that encode actin cytoskeleton proteins are shown in . The ACTC1 gene contains short introns allowing for rapid transcription after induction, while the other 3 genes contain large introns causing transcriptional delays. These genes range in size from 7.6 kb for ACTC1 () to 122 kb for VCL (), which translates into an estimated transcript completion times of 5 and 81 minutes, respectively. String interaction networks for the 4 different gene products are provided to show their physiological interactions.Citation32 It would be of interest to study the relationship between gene expression timing and the temporal assembly of multi-protein complexes, such as actin cytoskeleton. Perhaps the temporal gene expression pattern driven by evolutionary selection of particular gene sizes could teach us about the importance of the order by which these complexes are assembled. Additional studies are needed to elucidate the importance of temporal expression of different protein components for optimal assembly of actin cytoskeleton and other structural complexes in cells entering the cell cycle following serum stimulation or exposed to other environmental cues.
Figure 8. Transcription of actin cytoskeleton genes following serum stimulation. Bru-seq traces for (A) ACTC1, (B) DSTN, (C) ACTN4, and (D) VCL during starved conditions (orange) and following serum addition (blue). String interaction networks are shown below.
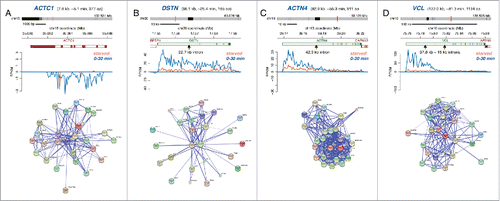
Figure 9. Model for the role of gene length in establishing temporal expression patterns following serum stimulation. (A) Transcription induction occurs simultaneously for several genes (blue arrow), but gene length influences the completion timing of the transcript (red lines). These immediately induced transcription factors (colored circles) then go on to activate their own gene targets (red arrows), whose expression timing is also influenced by gene length. (B) Temporal, staggered expression timing established by gene length.
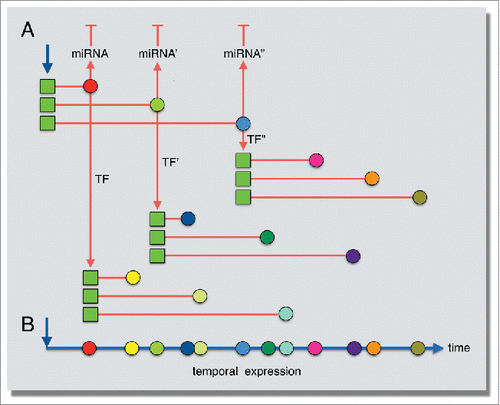
Discussion
Initiation of transcription is a key regulatory step for gene expression, where recruitment of RNAPII and promoter proximal pausing contribute to variations in temporal expression patterns during early cellular responses.Citation17,18 Gene length and transcription elongation rates also act as regulatory mechanisms controlling gene expression timing. Human genes span in size from a few hundred to over 2 million base pairs. The presence of introns in human genes necessitates splicing, which allows for the generation of alternative splice variants leading to the generation of multiple protein forms encoded by an individual gene. This diversification of the proteome is an important function of introns, but why do introns exhibit such diverse sizes? The time and energy exerted while transcribing large introns as well as the increased threat of genomic instability from very large transcription unitsCitation9 would be good reasons for evolutionary pressures to shape genes into more compact sizes. Instead, the retention and expansion of large and variably-sized introns within genes could have been selected to stagger the production of their full-length transcripts following transcription initiation to temporally regulate specific gene programs.
Immediate-early response genes have been typified by their short length and rapid induction. Our genome-wide profiling of nascent transcription using Bru-seq indicated that for the serum response, these common traits are not due to selective initiation of small genes but instead are a result of elongation constraints on longer genes which results in the staggered generation of full-sized transcripts. If genes in a particular response pathway were of similar sizes, the completion of their mRNAs would be expected to occur at the same time, and this could perhaps cause a “traffic jam” at ribosomes. Instead, evolutionary selection of different sized genes within a specific response pathway allows for temporal spacing of mature mRNA arrival at ribosomes for protein synthesis. Considering that many immediate response genes encode transcription factors that in turn regulate sets of different-sized genes, this potentially allows for one initial triggering event to induce a complex network of temporal expression patterns through combinatory regulation by gene length and signaling cascades (). Additionally, induction of primary miRNAs transcription units of different sizes is expected to temporally space the production of mature miRNA and the subsequent downregulation of target gene expression. We predict that immediately transcribed genes for other cellular responses would also have a broad size range based on the complexity of the induced pathways and whether the response occurs over a longer period of time. In our previous study, we treated cells with TNF for 1 hour to stimulate an immune response,Citation24 and induced genes identified by Bru-seq in that study were also longer overall compared to all expressed genes (data not shown).
Previous studies investigating gene expression changes following serum stimulation have been performed using steady-state RNA, and in many studies the mature RNA was obtained via poly(A) tail selection. One advantage of using nascent RNA Bru-seq for gene expression analysis is that the data is not confounded by the presence of pre-existing RNA. As a result, the Bru-seq analysis captured both immediate induction and repression of transcription. The data obtained for immediately repressed genes is novel because it reflects transcription rate changes and is not linked to the turnover of pre-existing RNA. We identified certain gene ontology terms not previously linked to repression following serum addition, including “meiotic recombination,” “RNA pol I transcription,” “peroxisome,” “oxidative phosphorylation,” “base excision repair,” and “RNA degradation” ().
We recently developed BruUV-seq to map active enhancer elements based on UV-mediated repression of eRNA degradation by the RNA exosome.Citation26 Here we used BruUV-seq to assess the modulation of enhancer activity immediately following serum stimulation. Our results revealed that immediate changes in enhancer activity were common in neighborhoods of induced genes but not around suppressed genes. Of the 50 most highly induced genes, 18 genes were associated with 4 or more activated enhancer elements, 20 genes were associated with 1-3 activated enhancers, and only 12 genes were not associated with any activated enhancer element. In contrast, only one out of the 25 most highly downregulated genes was associated with an enhancer element with reduced activity. Thus, transcriptional induction immediately following serum stimulation is likely a result of enhancer activation, while immediate transcriptional suppression is likely achieved in an enhancer activity-independent manner, however further investigation of enhancer transcription and identification of enhancer target genes is necessary. It is likely that signal transduction pathways that respond to serum stimulation result in the activation of a set of transcription factors that primarily drive transcription initiation by binding to nearby enhancer elements. Transcription factor motif enrichment analysis indicated that SRF, CREB and ATF transcription factors may be driving the initial wave of induction (). Interestingly, the top 3 most enriched motifs within suppressed genes did not have any identified transcription factor associated with them. This may reflect regulation of this understudied gene set by novel transcription factors.
If gene length plays an important role in coordinating expression following activation of particular pathways, we would predict that these gene length relationships are conserved across different species. Although genes tend to be larger in humans compared to other eukaryotes due to intron lengtheningCitation7,31,Citation33 we observed a strong correlation between gene lengths of orthologous genes in human and mouse. Therefore, we hypothesize that relative gene sizes may be important for the temporal regulation of gene expression in mammals. Though gene sizes may change over evolutionary time, the maintenance of relative gene sizes across species allows for a group of simultaneously induced genes to be expressed in a predetermined and presumably optimal order.
While we speculate that gene length acts as a biological timer for proper expression during the serum response (), the consequences of altering gene lengths and therefore the order of protein expression is unknown. The temporal order of gene expression during the serum response may only be important for certain sets of genes or for those involved in specific pathways. The immediately induced transcription factor genes showed a broad distribution of gene sizes, with gene lengths uniformly spread across a wide range as opposed to concentrated around a median value. Because this broad distribution may set up a distinct order of gene expression, we would predict that shuffling expression order would have significant biological effects. The fact that housekeeping genes are significantly shorter on average compared to all genes or transcription factor genes strongly supports the notion that the expansion of gene size of non-housekeeping genes has been selected for during evolution. Alternatively, introns in housekeeping genes have been shortened during evolution since these genes require constitutive rather than temporal expression. The larger sizes of serum-induced compared to serum-repressed genes implies that it is more important for cells to have a mechanism for temporally separating expression of genes that are induced compared to genes that are repressed. In addition to regulating the workflow at ribosomes, the temporal production of proteins as a consequence of different gene sizes may orchestrate their ordered assembly into multi-protein structures. Evolutionary selection of larger introns may also have been promoted by the advantage of placing regulatory elements and functional ncRNAs in the non-coding introns. Thus, introns can act as molecular timers with important biological regulatory functions both for protein production and assembly, and their size expansion has served as a critical element for evolutionary optimization.
In conclusion, our study provides a comprehensive profile of the early transcriptional response following serum stimulation. Early serum response genes display a wide range of lengths, which influences their transcriptional timing and allows for coordinated expression of functionally related genes. We speculate that gene size likely plays an important role in gene expression timing for many different cellular responses.
Materials and methods
Cell culture and serum stimulation
HF1, hTERT immortalized foreskin-derived human fibroblasts,Citation2,9,Citation23-26,Citation34 were grown in MEM supplemented with 10% FBS, L-glutamine, vitamin mix, and antibiotics. Starved cells were grown in MEM without the supplementation of FBS for 48 hrs. For serum stimulation experiments, FBS was added to the media of starved cells together with bromouridine to obtain 10% and 2 mM final concentrations of serum and bromouridine, respectively.
Bru-seq and BruUV-seq analyses
Bru-seq was performed as previously described.Citation24,25 Briefly, bromouridine (Bru) (Aldrich) was added to the media of starved or serum stimulated cells to a final concentration of 2 mM and incubated at 37°C for 30 min. BruUV-seq was performed as previously described Citation26 using a UV dose of 100 J/m2 prior to Bru-labeling. Total RNA was isolated using TRIzol reagent (Invitrogen) and Bru-labeled RNA was isolated from total RNA by incubation with anti-BrdU antibodies (BD Biosciences) conjugated to magnetic Dynabeads (Invitrogen) under gentle agitation at room temperature for 1h. cDNA libraries were generated from the isolated Bru-labeled RNA using the Illumina TruSeq kit, modified as described previously to produce strand-specific and size-selected libraries which were then sequenced using the Illumina HiSeq 2500 platform (50 base, single-ended reads).Citation24,25
Gene expression and serum response analysis
RPKM (reads per kilobase per million mapped reads) values were calculated for individual genes that were at least 300 bp long. For genes of lengths of 30 kb and less, RPKM values were calculated using read counts from the entire gene. For genes longer than 30 kb, an RPKM value was calculated using read counts from the first 30 kb downstream of the TSS. The R package DESeqCitation35 was used to test differential expression of genes whose mean RPKM between samples was greater than 0.5. Serum-response genes were defined as having a significant change in transcription initiation (adjusted p-values < 0.05, n = 2). Repressed genes were required to be expressed (RPKM value > 0.5) in the starved sample.
Ortholog size comparison
Mouse and human ortholog sizes were obtained using Ensembl Biomart.Citation1 The coefficient of determination (r2) was calculated. Genes with one-to-one orthologs were assessed.
Gene enrichment analysis
DAVID was used to analyze functional annotation enrichment.Citation36 We grouped induced genes into 5 categories based on gene length: 0-10 kb, 10-25 kb, 25-50 kb, 50-100 kb, and >100 kb. We grouped repressed genes into 2 categories: 0-15 kb and >15 kb. For the background gene list in each analysis, we used all expressed genes (RPKM > 0.5) which fell within the given size range. The pathways displayed have a p-value < 0.05. We also performed gene set enrichment analysis (GSEA).Citation37 Expressed genes were rank-ordered according to log2-fold changes compared to the gene expression in serum-starved cells. The top 10 pathways with the highest NESs were presented in .
Abbreviations
Bru | = | Bromouridine |
CNV | = | Copy Number Variation |
DAVID | = | Database for Annotation, Visualization and Integrated Discovery |
GSEA | = | Gene Set Enrichment Analysis |
hTERT | = | human Telomerase Reverse Transcriptase |
MAPK | = | Mitogen-Activated Protein Kinase |
qRT-PCR | = | quantitative Reverse Transcription Polymerase Chain Reaction |
RPKM | = | Reads Per Kilobase of transcript per Million mapped reads |
seq | = | sequencing |
ST | = | Starved condition |
SE | = | Serum-stimulated condition |
UV | = | Ultra Violet light |
Disclosure of potential conflict of interest
The authors have no conflict of interest with this study.
Supplemental Files
Download Zip (2.7 MB)Acknowledgments
We thank the personnel at University of Michigan Sequencing Core for professional technical assistance and Manhong Dai and Fan Meng for administration and maintenance of the University of Michigan Molecular and Behavioral Neuroscience Institute (MBNI) computing cluster. We thank Dr. Artur Veloso and Dr. Thomas E. Wilson for development of bioinformatics analysis and visualization tools.
Funding
This work was supported by research grants from the National Institute of Environmental Health Sciences (1R21ES020946), National Human Genome Research Institute (1R01HG006786) and the National Institute of Health (P50CA130810). KSK was supported, in part, by an NIH training grant (T32GM07544) and BM was supported by the University of Michigan School of Public Health Environmental Toxicology and Epidemiology Program, National Institute of Environmental Health Sciences (T32ES007062).
References
- Yates A, Akanni W, Amode MR, Barrell D, Billis K, Carvalho-Silva D, Cummins C, Clapham P, Fitzgerald S, Gil L, et al. Ensembl 2016. Nucleic Acids Res 2016; 44:D710-6; PMID:26687719; http://dx.doi.org/10.1093/nar/gkv1157
- Veloso A, Kirkconnell KS, Magnuson B, Biewen B, Paulsen MT, Wilson TE, Ljungman M. Rate of elongation by RNA polymerase II is associated with specific gene features and epigenetic modifications. Genome Res 2014; 24:896-905; PMID:24714810; http://dx.doi.org/10.1101/gr.171405.113
- Jonkers I, Kwak H, Lis JT. Genome-wide dynamics of Pol II elongation and its interplay with promoter proximal pausing, chromatin, and exons. Elife 2014; 3:e02407; PMID:24843027; http://dx.doi.org/10.7554/eLife.02407
- Fuchs G, Voichek Y, Benjamin S, Gilad S, Amit I, Oren M. 4sUDRB-seq: measuring genomewide transcriptional elongation rates and initiation frequencies within cells. Genome Biol 2014; 15:R69; PMID:24887486; http://dx.doi.org/10.1186/gb-2014-15-5-r69
- Saponaro M, Kantidakis T, Mitter R, Kelly GP, Heron M, Williams H, Soding J, Stewart A, Svejstrup JQ. RECQL5 controls transcript elongation and suppresses genome instability associated with transcription stress. Cell 2014; 157:1037-49; PMID:24836610; http://dx.doi.org/10.1016/j.cell.2014.03.048
- Kilchert C, Wittmann S, Vasiljeva L. The regulation and functions of the nuclear RNA exosome complex. Nat Rev Mol Cell Biol 2016; 17:227-39; PMID:26726035; http://dx.doi.org/10.1038/nrm.2015.15
- Gelfman S, Burstein D, Penn O, Savchenko A, Amit M, Schwartz S, Pupko T, Ast G. Changes in exon-intron structure during vertebrate evolution affect the splicing pattern of exons. Genome Res 2012; 22:35-50; PMID:21974994; http://dx.doi.org/10.1101/gr.119834.110
- Eisenberg E, Levanon EY. Human housekeeping genes are compact. Trends Genet 2003; 19:362-5; PMID:12850439; http://dx.doi.org/10.1016/S0168-9525(03)00140-9
- Wilson TE, Arlt MF, Park SH, Rajendran S, Paulsen M, Ljungman M, Glover TW. Large transcription units unify copy number variants and common fragile sites arising under replication stress. Genome Res 2015; 25:189-200; PMID:25373142; http://dx.doi.org/10.1101/gr.177121.114
- Swinburne IA, Miguez DG, Landgraf D, Silver PA. Intron length increases oscillatory periods of gene expression in animal cells. Genes Dev 2008; 22:2342-6; PMID:18703678; http://dx.doi.org/10.1101/gad.1696108
- Swinburne IA, Silver PA. Intron delays and transcriptional timing during development. Dev Cell 2008; 14:324-30; PMID:18331713; http://dx.doi.org/10.1016/j.devcel.2008.02.002
- Chorev M, Carmel L. The function of introns. Front Genet 2012; 3:55; PMID:22518112; http://dx.doi.org/10.3389/fgene.2012.00055
- Gubb D. Intron-delay and the precision of expression of homoeotic gene products in Drosophila. Dev Genet 1986; 7:119-31; PMID:NOT_FOUND; http://dx.doi.org/10.1002/dvg.1020070302
- Rothe M, Pehl M, Taubert H, Jackle H. Loss of gene function through rapid mitotic cycles in the Drosophila embryo. Nature 1992; 359:156-9; PMID:1522901; http://dx.doi.org/10.1038/359156a0
- Takashima Y, Ohtsuka T, Gonzalez A, Miyachi H, Kageyama R. Intronic delay is essential for oscillatory expression in the segmentation clock. Proc Natl Acad Sci U S A 2011; 108:3300-5; PMID:21300886; http://dx.doi.org/10.1073/pnas.1014418108
- Herschman HR. Primary response genes induced by growth factors and tumor promoters. Annu Rev Biochem 1991; 60:281-319; PMID:1883198; http://dx.doi.org/10.1146/annurev.bi.60.070191.001433
- Morris DP, Lei B, Longo LD, Bomsztyk K, Schwinn DA, Michelotti GA. Temporal Dissection of Rate Limiting Transcriptional Events Using Pol II ChIP and RNA Analysis of Adrenergic Stress Gene Activation. PLoS One 2015; 10:e0134442; PMID:26244980; http://dx.doi.org/10.1371/journal.pone.0134442
- Fuda NJ, Ardehali MB, Lis JT. Defining mechanisms that regulate RNA polymerase II transcription in vivo. Nature 2009; 461:186-92; PMID:19741698; http://dx.doi.org/10.1038/nature08449
- Winkles JA. Serum- and polypeptide growth factor-inducible gene expression in mouse fibroblasts. Prog Nucleic Acid Re 1997; 58:41-78; PMID:NOT_FOUND; http://dx.doi.org/10.1016/S0079-6603(08)60033-1
- Iyer VR, Eisen MB, Ross DT, Schuler G, Moore T, Lee JC, Trent JM, Staudt LM, Hudson J, Jr., Boguski MS, et al. The transcriptional program in the response of human fibroblasts to serum. Science 1999; 283:83-7; PMID:9872747; http://dx.doi.org/10.1126/science.283.5398.83
- Amit I, Citri A, Shay T, Lu Y, Katz M, Zhang F, Tarcic G, Siwak D, Lahad J, Jacob-Hirsch J, et al. A module of negative feedback regulators defines growth factor signaling. Nat Genet 2007; 39:503-12; PMID:17322878; http://dx.doi.org/10.1038/ng1987
- Tullai JW, Schaffer ME, Mullenbrock S, Sholder G, Kasif S, Cooper GM. Immediate-early and delayed primary response genes are distinct in function and genomic architecture. J Biol Chem 2007; 282:23981-95; PMID:17575275; http://dx.doi.org/10.1074/jbc.M702-044200
- Kirkconnell KS, Paulsen MT, Magnuson B, Bedi K, Ljungman M. Capturing the dynamic nascent transcriptome during acute cellular responses: The serum response. Biol Open 2016; 5:837-47; PMID:27230646; http://dx.doi.org/10.1242/bio.019323
- Paulsen MT, Veloso A, Prasad J, Bedi K, Ljungman EA, Tsan YC, Chang CW, Tarrier B, Washburn JG, Lyons R, et al. Coordinated regulation of synthesis and stability of RNA during the acute TNF-induced proinflammatory response. Proc Natl Acad Sci U S A 2013; 110:2240-5; PMID:23345452; http://dx.doi.org/10.1073/pnas.1219192110
- Paulsen MT, Veloso A, Prasad J, Bedi K, Ljungman EA, Magnuson B, Wilson TE, Ljungman M. Use of Bru-Seq and BruChase-Seq for genome-wide assessment of the synthesis and stability of RNA. Methods 2014; 67:45-54; PMID:23973811; http://dx.doi.org/10.1016/j.ymeth.2013.08.015
- Magnuson B, Veloso A, Kirkconnell KS, Lima LC, Paulsen MT, Ljungman EA, Bedi K, Prasad J, Wilson TE, Ljungman M. Identifying transcription start sites and active enhancer elements using BruUV-seq. Sci Rep 2015; 5:17978; PMID:26656874; http://dx.doi.org/10.1038/srep17978
- Blasius M, Wagner SA, Choudhary C, Bartek J, Jackson SP. A quantitative 14-3-3 interaction screen connects the nuclear exosome targeting complex to the DNA damage response. Genes Dev 2014; 28:1977-82; PMID:25189701; http://dx.doi.org/10.1101/gad.246272.114
- Tiedje C, Lubas M, Tehrani M, Menon MB, Ronkina N, Rousseau S, Cohen P, Kotlyarov A, Gaestel M. p38MAPK/MK2-mediated phosphorylation of RBM7 regulates the human nuclear exosome targeting complex. RNA 2015; 21:262-78; PMID:25525152; http://dx.doi.org/10.1261/rna.048090.114
- Huntzinger E, Izaurralde E. Gene silencing by microRNAs: contributions of translational repression and mRNA decay. Nat Rev Genet 2011; 12:99-110; PMID:21245828; http://dx.doi.org/10.1038/nrg2936
- Batzoglou S, Pachter L, Mesirov JP, Berger B, Lander ES. Human and Mouse Gene Structure: Comparative Analysis and Application to Exon Prediction. Genome Res 2000; 10:950-8; PMID:10899144; http://dx.doi.org/10.1101/gr.10.7.950
- Yandell M, Mungall CJ, Smith C, Prochnik S, Kaminker J, Hartzell G, Lewis S, Rubin GM. Large-scale trends in the evolution of gene structures within 11 animal genomes. PLoS Comput Biol 2006; 2:e15; PMID:16518452; http://dx.doi.org/10.1371/journal.pcbi.0020015
- Szklarczyk D, Franceschini A, Wyder S, Forslund K, Heller D, Huerta-Cepas J, Simonovic M, Roth A, Santos A, Tsafou KP, et al. STRING v10: protein-protein interaction networks, integrated over the tree of life. Nucleic Acids Res 2015; 43:D447-52; PMID:2535fs2553; http://dx.doi.org/10.1093/nar/gku1003
- Batzoglou S, Pachter L, Mesirov JP, Berger B, Lander ES. Human and mouse gene structure: comparative analysis and application to exon prediction. Genome Res 2000; 10:950-8; PMID:10899144; http://dx.doi.org/10.1101/gr.10.7.950
- Andrade-Lima LC, Veloso A, Paulsen MT, Menck CF, Ljungman M. DNA repair and recovery of RNA synthesis following exposure to ultraviolet light are delayed in long genes. Nucleic Acids Res 2015; 43:2744-56; PMID:25722371; http://dx.doi.org/10.1093/nar/gkv148
- Anders S, Huber W. Differential expression analysis for sequence count data. Genome Biol 2010; 11:R106; PMID:20979621; http://dx.doi.org/10.1186/gb-2010-11-10-r106
- Huang CN, Chan KC, Lin WT, Su SL, Wang CJ, Peng CH. Hibiscus sabdariffa inhibits vascular smooth muscle cell proliferation and migration induced by high glucose–a mechanism involves connective tissue growth factor signals. J Agric Food Chem 2009; 57:3073-9; PMID:19301817; http://dx.doi.org/10.1021/jf803911n
- Subramanian A, Tamayo P, Mootha VK, Mukherjee S, Ebert BL, Gillette MA, Paulovich A, Pomeroy SL, Golub TR, Lander ES, et al. Gene set enrichment analysis: a knowledge-based approach for interpreting genome-wide expression profiles. Proc Natl Acad Sci U S A 2005; 102:15545-50; PMID:16199517; http://dx.doi.org/10.1073/pnas.0506580102